Asymptotic Theory Of Nonlinear Regression
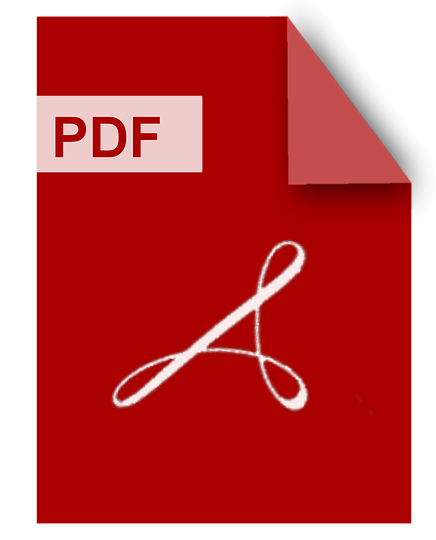
DOWNLOAD
Download Asymptotic Theory Of Nonlinear Regression PDF/ePub or read online books in Mobi eBooks. Click Download or Read Online button to get Asymptotic Theory Of Nonlinear Regression book now. This website allows unlimited access to, at the time of writing, more than 1.5 million titles, including hundreds of thousands of titles in various foreign languages. If the content not found or just blank you must refresh this page
Asymptotic Theory Of Nonlinear Regression
DOWNLOAD
Author : A.A. Ivanov
language : en
Publisher: Springer Science & Business Media
Release Date : 2013-04-17
Asymptotic Theory Of Nonlinear Regression written by A.A. Ivanov and has been published by Springer Science & Business Media this book supported file pdf, txt, epub, kindle and other format this book has been release on 2013-04-17 with Mathematics categories.
Let us assume that an observation Xi is a random variable (r.v.) with values in 1 1 (1R1 , 8 ) and distribution Pi (1R1 is the real line, and 8 is the cr-algebra of its Borel subsets). Let us also assume that the unknown distribution Pi belongs to a 1 certain parametric family {Pi() , () E e}. We call the triple £i = {1R1 , 8 , Pi(), () E e} a statistical experiment generated by the observation Xi. n We shall say that a statistical experiment £n = {lRn, 8 , P; ,() E e} is the product of the statistical experiments £i, i = 1, ... ,n if PO' = P () X ... X P () (IRn 1 n n is the n-dimensional Euclidean space, and 8 is the cr-algebra of its Borel subsets). In this manner the experiment £n is generated by n independent observations X = (X1, ... ,Xn). In this book we study the statistical experiments £n generated by observations of the form j = 1, ... ,n. (0.1) Xj = g(j, (}) + cj, c c In (0.1) g(j, (}) is a non-random function defined on e , where e is the closure in IRq of the open set e ~ IRq, and C j are independent r. v .-s with common distribution function (dJ.) P not depending on ().
Asymptotic Theory Of Nonlinear Regression
DOWNLOAD
Author : A. A. Ivanov
language : en
Publisher:
Release Date : 2014-01-15
Asymptotic Theory Of Nonlinear Regression written by A. A. Ivanov and has been published by this book supported file pdf, txt, epub, kindle and other format this book has been release on 2014-01-15 with categories.
Robust Methods And Asymptotic Theory In Nonlinear Econometrics
DOWNLOAD
Author : H. J. Bierens
language : en
Publisher: Springer Science & Business Media
Release Date : 2012-12-06
Robust Methods And Asymptotic Theory In Nonlinear Econometrics written by H. J. Bierens and has been published by Springer Science & Business Media this book supported file pdf, txt, epub, kindle and other format this book has been release on 2012-12-06 with Mathematics categories.
This Lecture Note deals with asymptotic properties, i.e. weak and strong consistency and asymptotic normality, of parameter estimators of nonlinear regression models and nonlinear structural equations under various assumptions on the distribution of the data. The estimation methods involved are nonlinear least squares estimation (NLLSE), nonlinear robust M-estimation (NLRME) and non linear weighted robust M-estimation (NLWRME) for the regression case and nonlinear two-stage least squares estimation (NL2SLSE) and a new method called minimum information estimation (MIE) for the case of structural equations. The asymptotic properties of the NLLSE and the two robust M-estimation methods are derived from further elaborations of results of Jennrich. Special attention is payed to the comparison of the asymptotic efficiency of NLLSE and NLRME. It is shown that if the tails of the error distribution are fatter than those of the normal distribution NLRME is more efficient than NLLSE. The NLWRME method is appropriate if the distributions of both the errors and the regressors have fat tails. This study also improves and extends the NL2SLSE theory of Amemiya. The method involved is a variant of the instrumental variables method, requiring at least as many instrumental variables as parameters to be estimated. The new MIE method requires less instrumental variables. Asymptotic normality can be derived by employing only one instrumental variable and consistency can even be proved with out using any instrumental variables at all.
Asymptotic Theory Of Instrumental Variables In Nonlinear Regression
DOWNLOAD
Author : José Francisco Burguete
language : en
Publisher:
Release Date : 1980
Asymptotic Theory Of Instrumental Variables In Nonlinear Regression written by José Francisco Burguete and has been published by this book supported file pdf, txt, epub, kindle and other format this book has been release on 1980 with categories.
Asymptotic Theory Of Nonlinear Least Squares Estimation
DOWNLOAD
Author : Chien-Fu WU
language : en
Publisher:
Release Date : 1979
Asymptotic Theory Of Nonlinear Least Squares Estimation written by Chien-Fu WU and has been published by this book supported file pdf, txt, epub, kindle and other format this book has been release on 1979 with categories.
Nonlinear Regression
DOWNLOAD
Author : George A. F. Seber
language : en
Publisher: John Wiley & Sons
Release Date : 2005-02-25
Nonlinear Regression written by George A. F. Seber and has been published by John Wiley & Sons this book supported file pdf, txt, epub, kindle and other format this book has been release on 2005-02-25 with Mathematics categories.
WILEY-INTERSCIENCE PAPERBACK SERIES The Wiley-Interscience Paperback Series consists of selected books that have been made more accessible to consumers in an effort to increase global appeal and general circulation. With these new unabridged softcover volumes, Wiley hopes to extend the lives of these works by making them available to future generations of statisticians, mathematicians, and scientists. From the Reviews of Nonlinear Regression "A very good book and an important one in that it is likely to become a standard reference for all interested in nonlinear regression; and I would imagine that any statistician concerned with nonlinear regression would want a copy on his shelves." –The Statistician "Nonlinear Regression also includes a reference list of over 700 entries. The compilation of this material and cross-referencing of it is one of the most valuable aspects of the book. Nonlinear Regression can provide the researcher unfamiliar with a particular specialty area of nonlinear regression an introduction to that area of nonlinear regression and access to the appropriate references . . . Nonlinear Regression provides by far the broadest discussion of nonlinear regression models currently available and will be a valuable addition to the library of anyone interested in understanding and using such models including the statistical researcher." –Mathematical Reviews
Asymptotic Theory Of Statistical Inference
DOWNLOAD
Author : B. L. S. Prakasa Rao
language : en
Publisher:
Release Date : 1987-01-16
Asymptotic Theory Of Statistical Inference written by B. L. S. Prakasa Rao and has been published by this book supported file pdf, txt, epub, kindle and other format this book has been release on 1987-01-16 with Mathematics categories.
Probability and stochastic processes; Limit theorems for some statistics; Asymptotic theory of estimation; Linear parametric inference; Martingale approach to inference; Inference in nonlinear regression; Von mises functionals; Empirical characteristic function and its applications.
Statistical Tools For Nonlinear Regression
DOWNLOAD
Author : Sylvie Huet
language : en
Publisher: Springer Science & Business Media
Release Date : 2013-04-17
Statistical Tools For Nonlinear Regression written by Sylvie Huet and has been published by Springer Science & Business Media this book supported file pdf, txt, epub, kindle and other format this book has been release on 2013-04-17 with Mathematics categories.
Statistical Tools for Nonlinear Regression presents methods for analyzing data. It has been expanded to include binomial, multinomial and Poisson non-linear models. The examples are analyzed with the free software nls2 updated to deal with the new models included in the second edition. The nls2 package is implemented in S-PLUS and R. Several additional tools are included in the package for calculating confidence regions for functions of parameters or calibration intervals, using classical methodology or bootstrap.
Stochastic Approximation And Nonlinear Regression
DOWNLOAD
Author : Arthur E. Albert
language : en
Publisher: MIT Press (MA)
Release Date : 2003-02-01
Stochastic Approximation And Nonlinear Regression written by Arthur E. Albert and has been published by MIT Press (MA) this book supported file pdf, txt, epub, kindle and other format this book has been release on 2003-02-01 with Science categories.
This monograph addresses the problem of "real-time" curve fitting in the presence of noise, from the computational and statistical viewpoints. It examines the problem of nonlinear regression, where observations are made on a time series whose mean-value function is known except for a vector parameter. In contrast to the traditional formulation, data are imagined to arrive in temporal succession. The estimation is carried out in real time so that, at each instant, the parameter estimate fully reflects all available data.Specifically, the monograph focuses on estimator sequences of the so-called differential correction type. The term "differential correction" refers to the fact that the difference between the components of the updated and previous estimators is proportional to the difference between the current observation and the value that would be predicted by the regression function if the previous estimate were in fact the true value of the unknown vector parameter. The vector of proportionality factors (which is generally time varying and can depend upon previous estimates) is called the "gain" or "smoothing" vector.The main purpose of this research is to relate the large-sample statistical behavior of such estimates (consistency, rate of convergence, large-sample distribution theory, asymptotic efficiency) to the properties of the regression function and the choice of smoothing vectors. Furthermore, consideration is given to the tradeoff that can be effected between computational simplicity and statistical efficiency through the choice of gains.Part I deals with the special cases of an unknown scalar parameter-discussing probability-one and mean-square convergence, rates of mean-square convergence, and asymptotic distribution theory of the estimators for various choices of the smoothing sequence. Part II examines the probability-one and mean-square convergence of the estimators in the vector case for various choices of smoothing vectors. Examples are liberally sprinkled throughout the book. Indeed, the last chapter is devoted entirely to the discussion of examples at varying levels of generality.If one views the stochastic approximation literature as a study in the asymptotic behavior of solutions to a certain class of nonlinear first-order difference equations with stochastic driving terms, then the results of this monograph also serve to extend and complement many of the results in that literature, which accounts for the authors' choice of title.The book is written at the first-year graduate level, although this level of maturity is not required uniformly. Certainly the reader should understand the concept of a limit both in the deterministic and probabilistic senses (i.e., almost sure and quadratic mean convergence). This much will assure a comfortable journey through the first fourth of the book. Chapters 4 and 5 require an acquaintance with a few selected central limit theorems. A familiarity with the standard techniques of large-sample theory will also prove useful but is not essential. Part II, Chapters 6 through 9, is couched in the language of matrix algebra, but none of the "classical" results used are deep. The reader who appreciates the elementary properties of eigenvalues, eigenvectors, and matrix norms will feel at home.MIT Press Research Monograph No. 42
Dynamic Nonlinear Econometric Models
DOWNLOAD
Author : Benedikt M. Pötscher
language : en
Publisher: Springer Science & Business Media
Release Date : 2013-03-09
Dynamic Nonlinear Econometric Models written by Benedikt M. Pötscher and has been published by Springer Science & Business Media this book supported file pdf, txt, epub, kindle and other format this book has been release on 2013-03-09 with Business & Economics categories.
Many relationships in economics, and also in other fields, are both dynamic and nonlinear. A major advance in econometrics over the last fifteen years has been the development of a theory of estimation and inference for dy namic nonlinear models. This advance was accompanied by improvements in computer technology that facilitate the practical implementation of such estimation methods. In two articles in Econometric Reviews, i.e., Pötscher and Prucha {1991a,b), we provided -an expository discussion of the basic structure of the asymptotic theory of M-estimators in dynamic nonlinear models and a review of the literature up to the beginning of this decade. Among others, the class of M-estimators contains least mean distance estimators (includ ing maximum likelihood estimators) and generalized method of moment estimators. The present book expands and revises the discussion in those articles. It is geared towards the professional econometrician or statistician. Besides reviewing the literature we also presented in the above men tioned articles a number of then new results. One example is a consis tency result for the case where the identifiable uniqueness condition fails.