Automatic Methods For Multiple Sclerosis New Lesions Detection And Segmentation
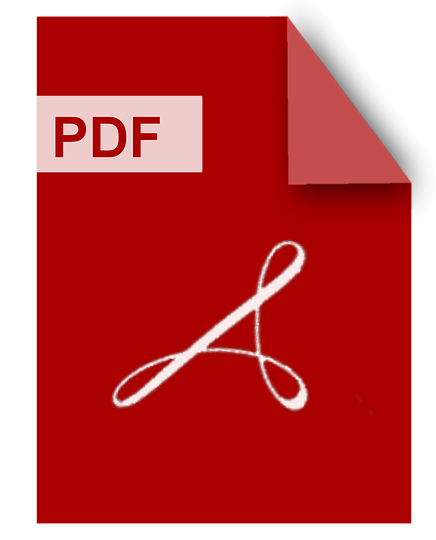
DOWNLOAD
Download Automatic Methods For Multiple Sclerosis New Lesions Detection And Segmentation PDF/ePub or read online books in Mobi eBooks. Click Download or Read Online button to get Automatic Methods For Multiple Sclerosis New Lesions Detection And Segmentation book now. This website allows unlimited access to, at the time of writing, more than 1.5 million titles, including hundreds of thousands of titles in various foreign languages. If the content not found or just blank you must refresh this page
Automatic Methods For Multiple Sclerosis New Lesions Detection And Segmentation
DOWNLOAD
Author : Olivier Commowick
language : en
Publisher: Frontiers Media SA
Release Date : 2023-04-11
Automatic Methods For Multiple Sclerosis New Lesions Detection And Segmentation written by Olivier Commowick and has been published by Frontiers Media SA this book supported file pdf, txt, epub, kindle and other format this book has been release on 2023-04-11 with Science categories.
Deep Learning Methods For Automated Detection Of New Multiple Sclerosis Lesions In Longitudinal Magnetic Resonance Images
DOWNLOAD
Author : Mostafa Salem
language : en
Publisher:
Release Date : 2020
Deep Learning Methods For Automated Detection Of New Multiple Sclerosis Lesions In Longitudinal Magnetic Resonance Images written by Mostafa Salem and has been published by this book supported file pdf, txt, epub, kindle and other format this book has been release on 2020 with categories.
This thesis is focused on developing novel and fully automated methods for the detection of new multiple sclerosis (MS) lesions inlongitudinal brain magnetic resonance imaging (MRI). First, we proposed a fully automated logistic regression-based framework forthe detection and segmentation of new T2-w lesions. The framework was based on intensity subtraction and deformation field (DF).Second, we proposed a fully convolutional neural network (FCNN) approach to detect new T2-w lesions in longitudinal brain MRimages. The model was trained end-to-end and simultaneously learned both the DFs and the new T2-w lesions. Finally, weproposed a deep learning-based approach for MS lesion synthesis to improve the lesion detection and segmentation performancein both cross-sectional and longitudinal analysis.
Automatic Segmentation Of Intramedullary Multiple Sclerosis Lesions
DOWNLOAD
Author : Charley Gros
language : en
Publisher:
Release Date : 2018
Automatic Segmentation Of Intramedullary Multiple Sclerosis Lesions written by Charley Gros and has been published by this book supported file pdf, txt, epub, kindle and other format this book has been release on 2018 with categories.
Context : The spinal cord is a key component of the central nervous system, which contains neurons responsible for complex functions, and ensures the conduction of motor and sensory information between the brain and the peripheral nervous system. Damage to the spinal cord, through trauma or neurodegenerative diseases, can lead to severe impairment, including functional disabilities, paralysis and/or pain. In multiple sclerosis (MS) patients, the spinal cord is frequently affected by atrophy and/or lesions. Conventional magnetic resonance imaging (MRI) is widely used by researchers and clinicians to non-invasively assess and characterize spinal cord microstructural changes. Quantitative assessment of the structural damage to the spinal cord (e.g. atrophy severity, lesion extent) is essential for the diagnosis, prognosis and longitudinal monitoring of diseases, such as MS. Furthermore, the development of objective biomarkers is essential to evaluate the effect of new therapeutic treatments. Spinal cord and intramedullary MS lesions segmentation is consequently clinically relevant, as well as a necessary step towards the interpretation of multi-parametric MR images. However, manual segmentation is highly time-consuming, tedious and prone to intra- and inter-rater variability. There is therefore a need for automated segmentation methods to facilitate the efficiency of analysis pipelines. Automatic lesion segmentation is challenging for various reasons : (i) lesion variability in terms of shape, size and location, (ii) lesion boundaries are most of the time not well defined, (iii) lesion intensities on MR data are confounding with those of normal-appearing structures. Moreover, achieving robust segmentation across multi-center MRI data is challenging because of the broad variability of data features (e.g. resolution, orientation, field of view). Despite recent substantial developments in spinal cord MRI processing, there is still no method available that can yield robust and reliable spinal cord segmentation across the very diverse spinal pathologies and data features. Regarding the intramedullary lesions, a thorough search of the relevant literature did not yield available method of automatic segmentation. Goal : To develop a fully-automatic framework for segmenting the spinal cord and intramedullary MS lesions from conventional human MRI data. Method : The presented approach is based on a cascade of two Convolutional Neural Networks (CNN). The method has been designed to face the main challenges of 'real world' spinal cord MRI data. It was trained and validated on a private dataset made up of 1943 MR volumes, acquired in different 30 sites with heterogeneous acquisition protocols. Scanned subjects involve 459 healthy controls, 471 MS patients and 112 with other spinal pathologies. The proposed spinal cord segmentation method was compared to a state-of-the-art spinal cord segmentation method, PropSeg. Results : The CNN-based approach achieved better results than PropSeg, yielding a median (interquartile range) Dice of 94.6 (4.6) vs. 87.9 (18.3) % when compared to the manual segmentation. For the lesion segmentation task, our method provided a median Dice-overlap with the manual segmentation of 60.0 (21.4) %, a lesion-based true positive rate of 83 (34) % and a lesion-based precision de 77 (44) %. Conclusion : An original fully-automatic method to segment the spinal cord and intramedullary MS lesions on MRI data has been devised during this Master's project. The method was validated extensively against a clinical dataset. The robustness of the spinal cord segmentation has been demonstrated, even on challenging pathological cases. Regarding the lesion segmentation, the results are encouraging despite the fairly high false positive rate. I believe in the potential value of these developed tools for the research community. In this vein, the methods are integrated and documented into an open-source software, the Spinal Cord Toolbox. Some of the tools developed during this Master's project are already integrated into automated analysis pipelines of clinical studies, including MS and Amyotrophic Lateral Sclerosis patients.
Robust Segmentation Of Focal Lesions On Multi Sequence Mri In Multiple Sclerosis
DOWNLOAD
Author : Daniel García Lorenzo
language : en
Publisher:
Release Date : 2010
Robust Segmentation Of Focal Lesions On Multi Sequence Mri In Multiple Sclerosis written by Daniel García Lorenzo and has been published by this book supported file pdf, txt, epub, kindle and other format this book has been release on 2010 with categories.
Multiple sclerosis (MS) affects around 80.000 people in France. Magnetic resonance imaging (MRI) is an essential tool for diagnosis of MS and MRI-derived surrogate markers such as MS lesion volumes are often used as measures in MS clinical trials for the development of new treatments. The manual segmentation of these MS lesions is a time-consuming task that shows high inter- and intra-rater variability. We developed an automatic workflow for the segmentation of focal MS lesions on MRI. The segmentation method is based on the robust estimation of a parametric model of the intensities of the brain; lesions are detected as outliers to the model. We proposed two methods to include spatial information in the segmentation using mean shift and graph cut. We performed a quantitative evaluation of our workflow using synthetic and clinical images of two different centers to verify its accuracy and robustness.
Segmentation Of Multiple Sclerosis Lesions Using Dictionary Learning In Feature Space
DOWNLOAD
Author : Gregory Christopher Kuling
language : en
Publisher:
Release Date : 2017
Segmentation Of Multiple Sclerosis Lesions Using Dictionary Learning In Feature Space written by Gregory Christopher Kuling and has been published by this book supported file pdf, txt, epub, kindle and other format this book has been release on 2017 with categories.
Manual segmentation is used in the diagnosis, management and evaluation of clinical trials for Multiple Sclerosis (MS), but human error makes manual segmentation variable. Automatic segmentation has been proposed using a Machine Learning algorithm Dictionary Learning (DL). We explored using different feature spaces to automatically segment MS lesions from healthy brain tissue. Methods of image texture analysis quantify the spatial distribution of the voxels in multi-weighted MR scans. We present the results of using a single voxel, single voxel and standard deviation (sigma) of adjacent voxels and a large spatial patch as feature spaces. The single voxel method segments the MS lesions with a Dice Similarity Coefficient (DSC) of 0.985 on simulated Brainweb data, but performed poorly with noise in the image (0.654). The single voxel and sigma performs at a DSC of 0.943 in the presence of 3% noise. The method should be attempted on real patient data.
Enhancing Multiple Sclerosis Lesion Segmentation In Mri Images
DOWNLOAD
Author : Daniel Biediger
language : en
Publisher:
Release Date : 2012
Enhancing Multiple Sclerosis Lesion Segmentation In Mri Images written by Daniel Biediger and has been published by this book supported file pdf, txt, epub, kindle and other format this book has been release on 2012 with Computer science categories.
Multiple sclerosis (MS) is an autoimmune disease of the central nervous system that causes damage to the insulating myelin sheaths around the axons in the brain. It affects over 2.5 million people world-wide. The disease progresses at different rates in different people and can have periods of remission and relapse. A fast and accurate method for evaluating the number and size of MS lesions in the brain is a key component in evaluating the progress of the disease and the efficacy of treatments. MS lesion segmentation usually requires the expertise of a trained physician. Manual segmentation is slow and difficult and the results can be somewhat subjective. While many automated methods exist, they do not provide sufficiently accurate segmentation results. There exists a need for a robust, fast, and accurate method for automatically segmenting MS lesions. This thesis presents the results of an effort to improve the segmentation results of an existing system for lesion segmentation in MRI images. It includes two different strategies to improve the segmentation results by addressing opportunities missed in the existing approach. The first strategy leverages the current processing system at a granularity finer than the whole-brain to detect lesions at a local level. The existing system makes global estimates on the tissue intensities. Because these intensities vary across the brain, the global assumption provides inaccurate estimates in some cases. The first improvement combines a series of local results to produce a whole-brain lesion segmentation. This approach better captures the local lesion properties and produces encouraging results, with a general improvement in the detection rate of lesions. The second method looks at the individual voxel level and the local intensity neighborhood. As a post-processing method, it selects seed points from the results of the previous step. It uses a region growing method based on cellular automata to expand the lesion areas based on a local neighborhood similarity in intensity. While it provides some benefit, it is sensitive to initial conditions and the results depend on the implementation details.
Medical Image Computing And Computer Assisted Intervention Miccai 2015
DOWNLOAD
Author : Nassir Navab
language : en
Publisher: Springer
Release Date : 2015-09-28
Medical Image Computing And Computer Assisted Intervention Miccai 2015 written by Nassir Navab and has been published by Springer this book supported file pdf, txt, epub, kindle and other format this book has been release on 2015-09-28 with Computers categories.
The three-volume set LNCS 9349, 9350, and 9351 constitutes the refereed proceedings of the 18th International Conference on Medical Image Computing and Computer-Assisted Intervention, MICCAI 2015, held in Munich, Germany, in October 2015. Based on rigorous peer reviews, the program committee carefully selected 263 revised papers from 810 submissions for presentation in three volumes. The papers have been organized in the following topical sections: quantitative image analysis I: segmentation and measurement; computer-aided diagnosis: machine learning; computer-aided diagnosis: automation; quantitative image analysis II: classification, detection, features, and morphology; advanced MRI: diffusion, fMRI, DCE; quantitative image analysis III: motion, deformation, development and degeneration; quantitative image analysis IV: microscopy, fluorescence and histological imagery; registration: method and advanced applications; reconstruction, image formation, advanced acquisition - computational imaging; modelling and simulation for diagnosis and interventional planning; computer-assisted and image-guided interventions.
Segmentation Of Multiple Sclerosis Lesions In Brain Mri
DOWNLOAD
Author : Bassem A Abdullah
language : en
Publisher:
Release Date : 2012
Segmentation Of Multiple Sclerosis Lesions In Brain Mri written by Bassem A Abdullah and has been published by this book supported file pdf, txt, epub, kindle and other format this book has been release on 2012 with categories.
Multiple Sclerosis (MS) is an autoimmune disease of central nervous system. It may result in a variety of symptoms from blurred vision to severe muscle weakness and degradation, depending on the affected regions in brain. To better understand this disease and to quantify its evolution, magnetic resonance imaging (MRI) is increasingly used nowadays. Manual delineation of MS lesions in MR images by human expert is time-consuming, subjective, and prone to inter-expert variability. Therefore, automatic segmentation is needed as an alternative to manual segmentation. However, the progression of the MS lesions shows considerable variability and MS lesions present temporal changes in shape, location, and area between patients and even for the same patient, which renders the automatic segmentation of MS lesions a challenging problem. In this dissertation, a set of segmentation pipelines are proposed for automatic segmentation of multiple sclerosis (MS) lesions from brain magnetic resonance imaging (MRI) data. These techniques use a trained support vector machine (SVM) to discriminate between the blocks in regions of MS lesions and the blocks in non-MS lesion regions mainly based on the textural features with aid of the other features. The main contribution of this set of frameworks is the use of textural features to detect MS lesions in a fully automated approach that does not rely on manually delineating the MS lesions. In addition, the technique introduces the concept of the multi-sectional views segmentation to produce verified segmentation. The multi-sectional views pipeline is customized to provide better segmentation performance and to benefit from the properties and the nature of MS lesion in MRI. These customization and enhancement leads to development of the customized MV-T-SVM. The MRI datasets that were used in the evaluation of the proposed pipelines are simulated MRI datasets (3 subjects) generated using the McGill University BrainWeb MRI Simulator, real datasets (51 subjects) publicly available at the workshop of MS Lesion Segmentation Challenge 2008 and real MRI datasets (10 subjects) for MS subjects acquired at the University of Miami. The obtained results indicate that the proposed method would be viable for use in clinical practice for the detection of MS lesions in MRI.
Automatic Segmentation Of Multiple Sclerosis Lesions In Mr Brain Images
DOWNLOAD
Author : Sherif M. Sabbah
language : en
Publisher:
Release Date : 1999
Automatic Segmentation Of Multiple Sclerosis Lesions In Mr Brain Images written by Sherif M. Sabbah and has been published by this book supported file pdf, txt, epub, kindle and other format this book has been release on 1999 with categories.
Exploring Uncertainty Measures In Deep Networks For Multiple Sclerosis Lesion Detection And Segmentation
DOWNLOAD
Author : Tanya Nair
language : en
Publisher:
Release Date : 2018
Exploring Uncertainty Measures In Deep Networks For Multiple Sclerosis Lesion Detection And Segmentation written by Tanya Nair and has been published by this book supported file pdf, txt, epub, kindle and other format this book has been release on 2018 with categories.
"This thesis presents the first exploration of multiple uncertainty estimates based on Monte Carlo (MC) dropout sampling in the context of deep networks for lesion detection and segmentation in medical images. Recently, deep learning frameworks have been shown to outperform traditional machine learning approaches to automated segmentation on a variety of public, medical-image challenge datasets, particularly for large pathologies. However, in the context of diseases such as Multiple Sclerosis (MS), monitoring all the focal lesions visible on MRI sequences, even the very small ones, is essential for disease staging, prognosis, and evaluating treatment efficacy, and deep learning models continue to underperform traditional machine learning approaches when it comes to small lesion segmentation. This, coupled with the deterministic output predictions made by deep learning models, continues to hinder their adoption into clinical routines. In order to address these barriers to deep learning's adoption in medical imaging, an approach that provides uncertainty estimates for a deep learning model's predictions is suggested, which would permit the subsequent revision by clinicians. While recent work in another domain shows the early, promising use of one uncertainty estimate in deep networks, there are several different measures of uncertainty can be calculated, and a thorough investigation of these in a clinically relevant context is lacking. The presented methodology is a 3D MS lesion segmentation CNN, augmented to provide four different voxel-based uncertainty measures based on Monte Carlo dropout. Lesion candidates are obtained from voxel-wise predictions of the network in a standard approach and a method is presented in the thesis to combine the voxel-wise uncertainties into lesion-level uncertainties. To evaluate the usefulness of the different measures, a method is presented to filter out either (a) voxels or (b) lesions for two separate comprehensive analyses such that the most uncertain regions are removed from the performance analysis of voxel segmentation or lesion detection. This filtering approach is contrasted against a standard deep learning approach of filtering predictions based on the non-probabilistic sigmoid or softmax output. The comprehensive experiments comparing the different uncertainties and their usefulness are performed with a proprietary, large-scale, multi-site, multi-scanner, clinical MS dataset. The results of the method over this data determine that across all measures, filtering out the most uncertain lesions greatly improves the lesion detection performance. Small lesions, which make up 40% of the dataset, are found to be the most uncertain and are shown to be main driver of the overall improvement when using uncertainty filtering. Even when excluding just 2% of all lesions, uncertainty based filtering improves the lesion-wise True Positive Rate from 0.75 to 0.8 at a lesion-wise False Detection Rate of 0.2 on remaining predictions. Additionally, the uncertainty-based filtering consistently performs better than sigmoid filtering. Reporting these results across the range of experiments serves as a reference to future researchers who want to apply deep learning methods in medical imaging and other safety-critical applications." --