Bayesian Semiparametric Regression
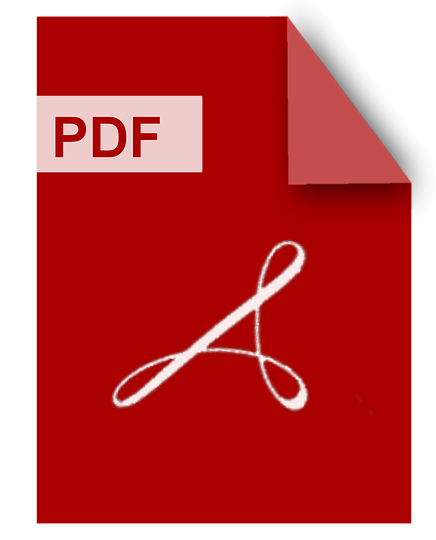
DOWNLOAD
Download Bayesian Semiparametric Regression PDF/ePub or read online books in Mobi eBooks. Click Download or Read Online button to get Bayesian Semiparametric Regression book now. This website allows unlimited access to, at the time of writing, more than 1.5 million titles, including hundreds of thousands of titles in various foreign languages. If the content not found or just blank you must refresh this page
Semiparametric Regression With R
DOWNLOAD
Author : Jaroslaw Harezlak
language : en
Publisher: Springer
Release Date : 2018-12-12
Semiparametric Regression With R written by Jaroslaw Harezlak and has been published by Springer this book supported file pdf, txt, epub, kindle and other format this book has been release on 2018-12-12 with Mathematics categories.
This easy-to-follow applied book on semiparametric regression methods using R is intended to close the gap between the available methodology and its use in practice. Semiparametric regression has a large literature but much of it is geared towards data analysts who have advanced knowledge of statistical methods. While R now has a great deal of semiparametric regression functionality, many of these developments have not trickled down to rank-and-file statistical analysts. The authors assemble a broad range of semiparametric regression R analyses and put them in a form that is useful for applied researchers. There are chapters devoted to penalized spines, generalized additive models, grouped data, bivariate extensions of penalized spines, and spatial semi-parametric regression models. Where feasible, the R code is provided in the text, however the book is also accompanied by an external website complete with datasets and R code. Because of its flexibility, semiparametric regression has proven to be of great value with many applications in fields as diverse as astronomy, biology, medicine, economics, and finance. This book is intended for applied statistical analysts who have some familiarity with R.
Bayesian Semiparametric Regression
DOWNLOAD
Author : Michael Smith
language : en
Publisher:
Release Date : 1996
Bayesian Semiparametric Regression written by Michael Smith and has been published by this book supported file pdf, txt, epub, kindle and other format this book has been release on 1996 with Regression analysis categories.
Semiparametric Regression
DOWNLOAD
Author : David Ruppert
language : en
Publisher: Cambridge University Press
Release Date : 2003-07-14
Semiparametric Regression written by David Ruppert and has been published by Cambridge University Press this book supported file pdf, txt, epub, kindle and other format this book has been release on 2003-07-14 with Mathematics categories.
Even experts on semiparametric regression should find something new here.
Bayesian Semiparametric Regression Models With Mixtures Of Constrained Polya Tree Priors
DOWNLOAD
Author : Jun Qin
language : en
Publisher:
Release Date : 2005
Bayesian Semiparametric Regression Models With Mixtures Of Constrained Polya Tree Priors written by Jun Qin and has been published by this book supported file pdf, txt, epub, kindle and other format this book has been release on 2005 with categories.
Bayesian Smoothing And Regression For Longitudinal Spatial And Event History Data
DOWNLOAD
Author : L. Fahrmeir
language : en
Publisher:
Release Date : 2011
Bayesian Smoothing And Regression For Longitudinal Spatial And Event History Data written by L. Fahrmeir and has been published by this book supported file pdf, txt, epub, kindle and other format this book has been release on 2011 with Bayesian statistical decision theory categories.
Bringing together recent advances in smoothing and semiparametric regression from a Bayesian perspective, this book demonstrates, with worked examples, the application of these statistical methods to a variety of fields including forestry, development economics, medicine and marketing.--[Source inconnue].
Bayesian Statistical Methods
DOWNLOAD
Author : Brian J. Reich
language : en
Publisher: CRC Press
Release Date : 2019-04-12
Bayesian Statistical Methods written by Brian J. Reich and has been published by CRC Press this book supported file pdf, txt, epub, kindle and other format this book has been release on 2019-04-12 with Mathematics categories.
Bayesian Statistical Methods provides data scientists with the foundational and computational tools needed to carry out a Bayesian analysis. This book focuses on Bayesian methods applied routinely in practice including multiple linear regression, mixed effects models and generalized linear models (GLM). The authors include many examples with complete R code and comparisons with analogous frequentist procedures. In addition to the basic concepts of Bayesian inferential methods, the book covers many general topics: Advice on selecting prior distributions Computational methods including Markov chain Monte Carlo (MCMC) Model-comparison and goodness-of-fit measures, including sensitivity to priors Frequentist properties of Bayesian methods Case studies covering advanced topics illustrate the flexibility of the Bayesian approach: Semiparametric regression Handling of missing data using predictive distributions Priors for high-dimensional regression models Computational techniques for large datasets Spatial data analysis The advanced topics are presented with sufficient conceptual depth that the reader will be able to carry out such analysis and argue the relative merits of Bayesian and classical methods. A repository of R code, motivating data sets, and complete data analyses are available on the book’s website. Brian J. Reich, Associate Professor of Statistics at North Carolina State University, is currently the editor-in-chief of the Journal of Agricultural, Biological, and Environmental Statistics and was awarded the LeRoy & Elva Martin Teaching Award. Sujit K. Ghosh, Professor of Statistics at North Carolina State University, has over 22 years of research and teaching experience in conducting Bayesian analyses, received the Cavell Brownie mentoring award, and served as the Deputy Director at the Statistical and Applied Mathematical Sciences Institute.
A Bayesian Approach To Additive Semi Parametric Regression
DOWNLOAD
Author : Chi-ming Wong
language : en
Publisher:
Release Date : 1993
A Bayesian Approach To Additive Semi Parametric Regression written by Chi-ming Wong and has been published by this book supported file pdf, txt, epub, kindle and other format this book has been release on 1993 with Bayesian statistical decision theory categories.
Bayesian Non And Semi Parametric Methods And Applications
DOWNLOAD
Author : Peter Rossi
language : en
Publisher: Princeton University Press
Release Date : 2014-04-27
Bayesian Non And Semi Parametric Methods And Applications written by Peter Rossi and has been published by Princeton University Press this book supported file pdf, txt, epub, kindle and other format this book has been release on 2014-04-27 with Business & Economics categories.
This book reviews and develops Bayesian non-parametric and semi-parametric methods for applications in microeconometrics and quantitative marketing. Most econometric models used in microeconomics and marketing applications involve arbitrary distributional assumptions. As more data becomes available, a natural desire to provide methods that relax these assumptions arises. Peter Rossi advocates a Bayesian approach in which specific distributional assumptions are replaced with more flexible distributions based on mixtures of normals. The Bayesian approach can use either a large but fixed number of normal components in the mixture or an infinite number bounded only by the sample size. By using flexible distributional approximations instead of fixed parametric models, the Bayesian approach can reap the advantages of an efficient method that models all of the structure in the data while retaining desirable smoothing properties. Non-Bayesian non-parametric methods often require additional ad hoc rules to avoid "overfitting," in which resulting density approximates are nonsmooth. With proper priors, the Bayesian approach largely avoids overfitting, while retaining flexibility. This book provides methods for assessing informative priors that require only simple data normalizations. The book also applies the mixture of the normals approximation method to a number of important models in microeconometrics and marketing, including the non-parametric and semi-parametric regression models, instrumental variables problems, and models of heterogeneity. In addition, the author has written a free online software package in R, "bayesm," which implements all of the non-parametric models discussed in the book.
Semiparametric Bayesian Regression For Multivariate Responses
DOWNLOAD
Author : Samson Babatunde Adebayo
language : en
Publisher:
Release Date : 2003
Semiparametric Bayesian Regression For Multivariate Responses written by Samson Babatunde Adebayo and has been published by this book supported file pdf, txt, epub, kindle and other format this book has been release on 2003 with categories.
Statistical Modelling And Regression Structures
DOWNLOAD
Author : Thomas Kneib
language : en
Publisher: Springer Science & Business Media
Release Date : 2010-01-12
Statistical Modelling And Regression Structures written by Thomas Kneib and has been published by Springer Science & Business Media this book supported file pdf, txt, epub, kindle and other format this book has been release on 2010-01-12 with Mathematics categories.
The contributions collected in this book have been written by well-known statisticians to acknowledge Ludwig Fahrmeir's far-reaching impact on Statistics as a science, while celebrating his 65th birthday. The contributions cover broad areas of contemporary statistical model building, including semiparametric and geoadditive regression, Bayesian inference in complex regression models, time series modelling, statistical regularization, graphical models and stochastic volatility models.