Building Recommendation Engines
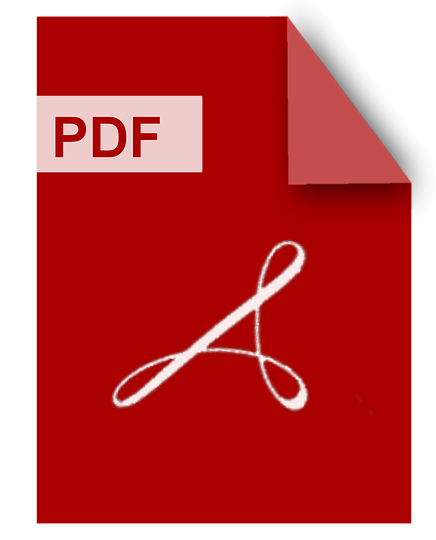
DOWNLOAD
Download Building Recommendation Engines PDF/ePub or read online books in Mobi eBooks. Click Download or Read Online button to get Building Recommendation Engines book now. This website allows unlimited access to, at the time of writing, more than 1.5 million titles, including hundreds of thousands of titles in various foreign languages. If the content not found or just blank you must refresh this page
Building Recommender Systems With Machine Learning And Ai
DOWNLOAD
Author : Frank Kane
language : en
Publisher:
Release Date : 2018
Building Recommender Systems With Machine Learning And Ai written by Frank Kane and has been published by this book supported file pdf, txt, epub, kindle and other format this book has been release on 2018 with categories.
Automated recommendations are everywhere: Netflix, Amazon, YouTube, and more. Recommender systems learn about your unique interests and show the products or content they think you'll like best. Discover how to build your own recommender systems from one of the pioneers in the field. Frank Kane spent over nine years at Amazon, where he led the development of many of the company's personalized product recommendation technologies. In this course, he covers recommendation algorithms based on neighborhood-based collaborative filtering and more modern techniques, including matrix factorization and even deep learning with artificial neural networks. Along the way, you can learn from Frank's extensive industry experience and understand the real-world challenges of applying these algorithms at a large scale with real-world data. You can also go hands-on, developing your own framework to test algorithms and building your own neural networks using technologies like Amazon DSSTNE, AWS SageMaker, and TensorFlow.
Building Practical Recommendation Engines
DOWNLOAD
Author : Suresh K. Gorakala
language : en
Publisher:
Release Date : 2017
Building Practical Recommendation Engines written by Suresh K. Gorakala and has been published by this book supported file pdf, txt, epub, kindle and other format this book has been release on 2017 with categories.
"A recommendation engine (sometimes referred to as a recommender system) is a tool that lets algorithm developers predict what a user may or may not like among a list of given items. Recommender systems have become extremely common in recent years, and are applied in a variety of applications. The most popular ones are movies, music, news, books, research articles, search queries, social tags, and products in general. This video starts with an introduction to recommendation systems and its applications. You will then start building recommendation engines straight away from the very basics. As you move along, you will learn to build recommender systems with popular frameworks such as R, Python, and more. You will get an insight into the pros and cons of different recommendation engines and when to use which recommendation. With the help of this course, you will quickly get up and running with Recommender systems. You will create recommendation engines of varying complexities, ranging from a simple recommendation engine to real-time recommendation engines."--Resource description page.
Building Recommendation Engines
DOWNLOAD
Author : Sureshkumar Gorakala
language : en
Publisher:
Release Date : 2016-12-30
Building Recommendation Engines written by Sureshkumar Gorakala and has been published by this book supported file pdf, txt, epub, kindle and other format this book has been release on 2016-12-30 with categories.
Understand your data and preferences to make intelligent, accurate, and profitable decisionsAbout This Book* A step-by-step guide to building recommendation engines that are personalized, scalable, and real time* Get to grips with the best tool available on the market to create recommender systems* This hands-on guide shows you how to implement different tools for recommendation engines, and when to use whichWho This Book Is ForThis book caters to beginners and experienced data scientists looking to understand and build complex predictive decision-making systems, recommendation engines using R, Python, Spark, Neo4j, and Hadoop.What you will learn* Building your first recommendation engine* Discover the tools needed to build recommendation engines* Dive into the various techniques of recommender systems such as collaborative, content-based, and cross-recommendations* Familiarize yourself with machine learning algorithms in different frameworks* Build different versions of recommendation engines from practical code examplesIn DetailA recommendation engine (sometimes referred to as a recommender system) is a tool that lets algorithm developers predict what a user may or may not like among a list of given items. Recommender systems have become extremely common in recent years, and are applied in a variety of applications. The most popular ones are movies, music, news, books, research articles, search queries, social tags, and products in general.If you want to build efficient decision-making systems that will ease your work, this book is for you. This guide will take you on a unique journey of exploring various recommender systems, building them, and implementing them in popular techniques such as R, Python, Spark, and others. This book will cover all that is required to get you up and running with building recommender systems.The book starts with an introduction to recommendation systems and its applications. Then you will start building recommendation engines. As you move along, you will learn to build recommender systems with popular frameworks such as R, Python, Spark, Neo4j, and Hadoop with practical examples. You will get an insight into the pros and cons of each recommendation engine and when to use which recommendation. During the course of the book, you will create simple recommendation engine, real-time recommendation engine, scalable recommendation engine, and so on. You will familiarize yourselves with various techniques of recommender systems such as collaborative, content-based, and cross-recommendations before getting to know the best practices of building a recommender system towards the end of the book.
Hands On Recommendation Systems With Python
DOWNLOAD
Author : Rounak Banik
language : en
Publisher: Packt Publishing Ltd
Release Date : 2018-07-31
Hands On Recommendation Systems With Python written by Rounak Banik and has been published by Packt Publishing Ltd this book supported file pdf, txt, epub, kindle and other format this book has been release on 2018-07-31 with Computers categories.
With Hands-On Recommendation Systems with Python, learn the tools and techniques required in building various kinds of powerful recommendation systems (collaborative, knowledge and content based) and deploying them to the web Key Features Build industry-standard recommender systems Only familiarity with Python is required No need to wade through complicated machine learning theory to use this book Book Description Recommendation systems are at the heart of almost every internet business today; from Facebook to Netflix to Amazon. Providing good recommendations, whether it's friends, movies, or groceries, goes a long way in defining user experience and enticing your customers to use your platform. This book shows you how to do just that. You will learn about the different kinds of recommenders used in the industry and see how to build them from scratch using Python. No need to wade through tons of machine learning theory—you'll get started with building and learning about recommenders as quickly as possible.. In this book, you will build an IMDB Top 250 clone, a content-based engine that works on movie metadata. You'll use collaborative filters to make use of customer behavior data, and a Hybrid Recommender that incorporates content based and collaborative filtering techniques With this book, all you need to get started with building recommendation systems is a familiarity with Python, and by the time you're fnished, you will have a great grasp of how recommenders work and be in a strong position to apply the techniques that you will learn to your own problem domains. What you will learn Get to grips with the different kinds of recommender systems Master data-wrangling techniques using the pandas library Building an IMDB Top 250 Clone Build a content based engine to recommend movies based on movie metadata Employ data-mining techniques used in building recommenders Build industry-standard collaborative filters using powerful algorithms Building Hybrid Recommenders that incorporate content based and collaborative fltering Who this book is for If you are a Python developer and want to develop applications for social networking, news personalization or smart advertising, this is the book for you. Basic knowledge of machine learning techniques will be helpful, but not mandatory.
Building A Recommendation System With R
DOWNLOAD
Author : Suresh K. Gorakala
language : en
Publisher: Packt Publishing Ltd
Release Date : 2015-09-29
Building A Recommendation System With R written by Suresh K. Gorakala and has been published by Packt Publishing Ltd this book supported file pdf, txt, epub, kindle and other format this book has been release on 2015-09-29 with Computers categories.
Learn the art of building robust and powerful recommendation engines using R About This Book Learn to exploit various data mining techniques Understand some of the most popular recommendation techniques This is a step-by-step guide full of real-world examples to help you build and optimize recommendation engines Who This Book Is For If you are a competent developer with some knowledge of machine learning and R, and want to further enhance your skills to build recommendation systems, then this book is for you. What You Will Learn Get to grips with the most important branches of recommendation Understand various data processing and data mining techniques Evaluate and optimize the recommendation algorithms Prepare and structure the data before building models Discover different recommender systems along with their implementation in R Explore various evaluation techniques used in recommender systems Get to know about recommenderlab, an R package, and understand how to optimize it to build efficient recommendation systems In Detail A recommendation system performs extensive data analysis in order to generate suggestions to its users about what might interest them. R has recently become one of the most popular programming languages for the data analysis. Its structure allows you to interactively explore the data and its modules contain the most cutting-edge techniques thanks to its wide international community. This distinctive feature of the R language makes it a preferred choice for developers who are looking to build recommendation systems. The book will help you understand how to build recommender systems using R. It starts off by explaining the basics of data mining and machine learning. Next, you will be familiarized with how to build and optimize recommender models using R. Following that, you will be given an overview of the most popular recommendation techniques. Finally, you will learn to implement all the concepts you have learned throughout the book to build a recommender system. Style and approach This is a step-by-step guide that will take you through a series of core tasks. Every task is explained in detail with the help of practical examples.
Building Recommender Systems With Machine Learning And Ai Help People Discover New Products And Content With Deep Learning Neural Networks And Mach
DOWNLOAD
Author : Frank Kane
language : en
Publisher:
Release Date : 2018-08-11
Building Recommender Systems With Machine Learning And Ai Help People Discover New Products And Content With Deep Learning Neural Networks And Mach written by Frank Kane and has been published by this book supported file pdf, txt, epub, kindle and other format this book has been release on 2018-08-11 with Computers categories.
Learn how to build recommender systems from one of Amazon's pioneers in the field. Frank Kane spent over nine years at Amazon, where he managed and led the development of many of Amazon's personalized product recommendation technologies.You've seen automated recommendations everywhere - on Netflix's home page, on YouTube, and on Amazon as these machine learning algorithms learn about your unique interests, and show the best products or content for you as an individual. These technologies have become central to the largest, most prestigious tech employers out there, and by understanding how they work, you'll become very valuable to them.This book is adapted from Frank's popular online course published by Sundog Education, so you can expect lots of visual aids from its slides and a conversational, accessible tone throughout the book. The graphics and scripts from over 300 slides are included, and you'll have access to all of the source code associated with it as well.We'll cover tried and true recommendation algorithms based on neighborhood-based collaborative filtering, and work our way up to more modern techniques including matrix factorization and even deep learning with artificial neural networks. Along the way, you'll learn from Frank's extensive industry experience to understand the real-world challenges you'll encounter when applying these algorithms at large scale and with real-world data.This book is very hands-on; you'll develop your own framework for evaluating and combining many different recommendation algorithms together, and you'll even build your own neural networks using Tensorflow to generate recommendations from real-world movie ratings from real people. We'll cover: -Building a recommendation engine-Evaluating recommender systems-Content-based filtering using item attributes-Neighborhood-based collaborative filtering with user-based, item-based, and KNN CF-Model-based methods including matrix factorization and SVD-Applying deep learning, AI, and artificial neural networks to recommendations-Session-based recommendations with recursive neural networks-Scaling to massive data sets with Apache Spark machine learning, Amazon DSSTNE deep learning, and AWS SageMaker with factorization machines-Real-world challenges and solutions with recommender systems-Case studies from YouTube and Netflix-Building hybrid, ensemble recommendersThis comprehensive book takes you all the way from the early days of collaborative filtering, to bleeding-edge applications of deep neural networks and modern machine learning techniques for recommending the best items to every individual user.The coding exercises for this book use the Python programming language. We include an intro to Python if you're new to it, but you'll need some prior programming experience in order to use this book successfully. We also include a short introduction to deep learning, Tensorfow, and Keras if you are new to the field of artificial intelligence, but you'll need to be able to understand new computer algorithms.Dive in, and learn about one of the most interesting and lucrative applications of machine learning and deep learning there is!
Building Recommendation Systems In Python And Jax
DOWNLOAD
Author : Bryan Bischof Ph.D
language : en
Publisher: "O'Reilly Media, Inc."
Release Date : 2023-12-04
Building Recommendation Systems In Python And Jax written by Bryan Bischof Ph.D and has been published by "O'Reilly Media, Inc." this book supported file pdf, txt, epub, kindle and other format this book has been release on 2023-12-04 with Computers categories.
Implementing and designing systems that make suggestions to users are among the most popular and essential machine learning applications available. Whether you want customers to find the most appealing items at your online store, videos to enrich and entertain them, or news they need to know, recommendation systems (RecSys) provide the way. In this practical book, authors Bryan Bischof and Hector Yee illustrate the core concepts and examples to help you create a RecSys for any industry or scale. You'll learn the math, ideas, and implementation details you need to succeed. This book includes the RecSys platform components, relevant MLOps tools in your stack, plus code examples and helpful suggestions in PySpark, SparkSQL, FastAPI, and Weights & Biases. You'll learn: The data essential for building a RecSys How to frame your data and business as a RecSys problem Ways to evaluate models appropriate for your system Methods to implement, train, test, and deploy the model you choose Metrics you need to track to ensure your system is working as planned How to improve your system as you learn more about your users, products, and business case
Building Recommendation Systems With Python
DOWNLOAD
Author : Eric Rodríguez
language : en
Publisher:
Release Date : 2019
Building Recommendation Systems With Python written by Eric Rodríguez and has been published by this book supported file pdf, txt, epub, kindle and other format this book has been release on 2019 with categories.
Build real-world recommendation systems using collaborative, content-based, and hybrid filtering techniques in Python About This Video Understand how to work with real data using a recommendation in Python Graphical representation of categories or classes to visualize your data Comparison of different recommendation systems and learning to help you choose the right one In Detail Recommendation Engines have become an integral part of any application. For accurate recommendations, you require user information. The more data you feed to your engine, the more output it can generate - for example, a movie recommendation based on its rating, a YouTube video recommendation to a viewer, or recommending a product to a shopper online. In this practical course, you will be building three powerful real-world recommendation engines using three different filtering techniques. You'll start by creating usable data from your data source and implementing the best data filtering techniques for recommendations. Then you will use machine learning techniques to create your own algorithm, which will predict and recommend accurate data. By the end of the course, you'll be able to build effective online recommendation engines with machine learning and Python - on your own. Downloading the example code for this course: You can download the example code files for this course on GitHub at the following link: https://github.com/PacktPublishing/Building-Recommendation-Systems-with-Python . If you require support please email: [email protected].
Applied Recommender Systems With Python
DOWNLOAD
Author : Akshay Kulkarni
language : en
Publisher: Apress
Release Date : 2022-12-08
Applied Recommender Systems With Python written by Akshay Kulkarni and has been published by Apress this book supported file pdf, txt, epub, kindle and other format this book has been release on 2022-12-08 with Computers categories.
This book will teach you how to build recommender systems with machine learning algorithms using Python. Recommender systems have become an essential part of every internet-based business today. You'll start by learning basic concepts of recommender systems, with an overview of different types of recommender engines and how they function. Next, you will see how to build recommender systems with traditional algorithms such as market basket analysis and content- and knowledge-based recommender systems with NLP. The authors then demonstrate techniques such as collaborative filtering using matrix factorization and hybrid recommender systems that incorporate both content-based and collaborative filtering techniques. This is followed by a tutorial on building machine learning-based recommender systems using clustering and classification algorithms like K-means and random forest. The last chapters cover NLP, deep learning, and graph-based techniques to build a recommender engine. Each chapter includes data preparation, multiple ways to evaluate and optimize the recommender systems, supporting examples, and illustrations. By the end of this book, you will understand and be able to build recommender systems with various tools and techniques with machine learning, deep learning, and graph-based algorithms. What You Will Learn Understand and implement different recommender systems techniques with Python Employ popular methods like content- and knowledge-based, collaborative filtering, market basket analysis, and matrix factorization Build hybrid recommender systems that incorporate both content-based and collaborative filtering Leverage machine learning, NLP, and deep learning for building recommender systems Who This Book Is ForData scientists, machine learning engineers, and Python programmers interested in building and implementing recommender systems to solve problems.
Recommender Systems Handbook
DOWNLOAD
Author : Francesco Ricci
language : en
Publisher: Springer Nature
Release Date : 2022-04-21
Recommender Systems Handbook written by Francesco Ricci and has been published by Springer Nature this book supported file pdf, txt, epub, kindle and other format this book has been release on 2022-04-21 with Computers categories.
This third edition handbook describes in detail the classical methods as well as extensions and novel approaches that were more recently introduced within this field. It consists of five parts: general recommendation techniques, special recommendation techniques, value and impact of recommender systems, human computer interaction, and applications. The first part presents the most popular and fundamental techniques currently used for building recommender systems, such as collaborative filtering, semantic-based methods, recommender systems based on implicit feedback, neural networks and context-aware methods. The second part of this handbook introduces more advanced recommendation techniques, such as session-based recommender systems, adversarial machine learning for recommender systems, group recommendation techniques, reciprocal recommenders systems, natural language techniques for recommender systems and cross-domain approaches to recommender systems. The third part covers a wide perspective to the evaluation of recommender systems with papers on methods for evaluating recommender systems, their value and impact, the multi-stakeholder perspective of recommender systems, the analysis of the fairness, novelty and diversity in recommender systems. The fourth part contains a few chapters on the human computer dimension of recommender systems, with research on the role of explanation, the user personality and how to effectively support individual and group decision with recommender systems. The last part focusses on application in several important areas, such as, food, music, fashion and multimedia recommendation. This informative third edition handbook provides a comprehensive, yet concise and convenient reference source to recommender systems for researchers and advanced-level students focused on computer science and data science. Professionals working in data analytics that are using recommendation and personalization techniques will also find this handbook a useful tool.