Complex Valued Neural Networks Learning Algorithms And Applications
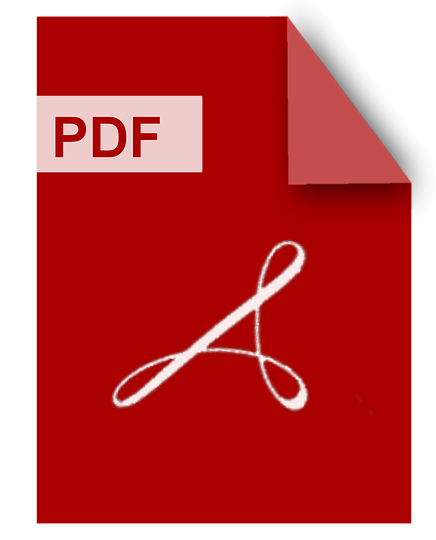
DOWNLOAD
Download Complex Valued Neural Networks Learning Algorithms And Applications PDF/ePub or read online books in Mobi eBooks. Click Download or Read Online button to get Complex Valued Neural Networks Learning Algorithms And Applications book now. This website allows unlimited access to, at the time of writing, more than 1.5 million titles, including hundreds of thousands of titles in various foreign languages. If the content not found or just blank you must refresh this page
Complex Valued Neural Networks Learning Algorithms And Applications
DOWNLOAD
Author : Md Faijul Amin
language : en
Publisher:
Release Date : 2018-07-03
Complex Valued Neural Networks Learning Algorithms And Applications written by Md Faijul Amin and has been published by this book supported file pdf, txt, epub, kindle and other format this book has been release on 2018-07-03 with categories.
Complex Valued Neural Networks
DOWNLOAD
Author : Akira Hirose
language : en
Publisher: Wiley-IEEE Press
Release Date : 2013-04-29
Complex Valued Neural Networks written by Akira Hirose and has been published by Wiley-IEEE Press this book supported file pdf, txt, epub, kindle and other format this book has been release on 2013-04-29 with Computers categories.
Presents the latest advances in complex-valued neural networks by demonstrating the theory in a wide range of applications Complex-valued neural networks is a rapidly developing neural network framework that utilizes complex arithmetic, exhibiting specific characteristics in its learning, self-organizing, and processing dynamics. They are highly suitable for processing complex amplitude, composed of amplitude and phase, which is one of the core concepts in physical systems to deal with electromagnetic, light, sonic/ultrasonic waves as well as quantum waves, namely, electron and superconducting waves. This fact is a critical advantage in practical applications in diverse fields of engineering, where signals are routinely analyzed and processed in time/space, frequency, and phase domains. Complex-Valued Neural Networks: Advances and Applications covers cutting-edge topics and applications surrounding this timely subject. Demonstrating advanced theories with a wide range of applications, including communication systems, image processing systems, and brain-computer interfaces, this text offers comprehensive coverage of: Conventional complex-valued neural networks Quaternionic neural networks Clifford-algebraic neural networks Presented by international experts in the field, Complex-Valued Neural Networks: Advances and Applications is ideal for advanced-level computational intelligence theorists, electromagnetic theorists, and mathematicians interested in computational intelligence, artificial intelligence, machine learning theories, and algorithms.
Supervised Learning With Complex Valued Neural Networks
DOWNLOAD
Author : Sundaram Suresh
language : en
Publisher: Springer
Release Date : 2012-07-28
Supervised Learning With Complex Valued Neural Networks written by Sundaram Suresh and has been published by Springer this book supported file pdf, txt, epub, kindle and other format this book has been release on 2012-07-28 with Computers categories.
Recent advancements in the field of telecommunications, medical imaging and signal processing deal with signals that are inherently time varying, nonlinear and complex-valued. The time varying, nonlinear characteristics of these signals can be effectively analyzed using artificial neural networks. Furthermore, to efficiently preserve the physical characteristics of these complex-valued signals, it is important to develop complex-valued neural networks and derive their learning algorithms to represent these signals at every step of the learning process. This monograph comprises a collection of new supervised learning algorithms along with novel architectures for complex-valued neural networks. The concepts of meta-cognition equipped with a self-regulated learning have been known to be the best human learning strategy. In this monograph, the principles of meta-cognition have been introduced for complex-valued neural networks in both the batch and sequential learning modes. For applications where the computation time of the training process is critical, a fast learning complex-valued neural network called as a fully complex-valued relaxation network along with its learning algorithm has been presented. The presence of orthogonal decision boundaries helps complex-valued neural networks to outperform real-valued networks in performing classification tasks. This aspect has been highlighted. The performances of various complex-valued neural networks are evaluated on a set of benchmark and real-world function approximation and real-valued classification problems.
Complex Valued Neural Networks With Multi Valued Neurons
DOWNLOAD
Author : Igor Aizenberg
language : en
Publisher: Springer
Release Date : 2011-06-24
Complex Valued Neural Networks With Multi Valued Neurons written by Igor Aizenberg and has been published by Springer this book supported file pdf, txt, epub, kindle and other format this book has been release on 2011-06-24 with Technology & Engineering categories.
Complex-Valued Neural Networks have higher functionality, learn faster and generalize better than their real-valued counterparts. This book is devoted to the Multi-Valued Neuron (MVN) and MVN-based neural networks. It contains a comprehensive observation of MVN theory, its learning, and applications. MVN is a complex-valued neuron whose inputs and output are located on the unit circle. Its activation function is a function only of argument (phase) of the weighted sum. MVN derivative-free learning is based on the error-correction rule. A single MVN can learn those input/output mappings that are non-linearly separable in the real domain. Such classical non-linearly separable problems as XOR and Parity n are the simplest that can be learned by a single MVN. Another important advantage of MVN is a proper treatment of the phase information. These properties of MVN become even more remarkable when this neuron is used as a basic one in neural networks. The Multilayer Neural Network based on Multi-Valued Neurons (MLMVN) is an MVN-based feedforward neural network. Its backpropagation learning algorithm is derivative-free and based on the error-correction rule. It does not suffer from the local minima phenomenon. MLMVN outperforms many other machine learning techniques in terms of learning speed, network complexity and generalization capability when solving both benchmark and real-world classification and prediction problems. Another interesting application of MVN is its use as a basic neuron in multi-state associative memories. The book is addressed to those readers who develop theoretical fundamentals of neural networks and use neural networks for solving various real-world problems. It should also be very suitable for Ph.D. and graduate students pursuing their degrees in computational intelligence.
Complex Valued Neural Networks Utilizing High Dimensional Parameters
DOWNLOAD
Author : Nitta, Tohru
language : en
Publisher: IGI Global
Release Date : 2009-02-28
Complex Valued Neural Networks Utilizing High Dimensional Parameters written by Nitta, Tohru and has been published by IGI Global this book supported file pdf, txt, epub, kindle and other format this book has been release on 2009-02-28 with Computers categories.
"This book covers the current state-of-the-art theories and applications of neural networks with high-dimensional parameters"--Provided by publisher.
Neural Networks And Computing
DOWNLOAD
Author : Tommy W. S. Chow
language : en
Publisher: Imperial College Press
Release Date : 2007
Neural Networks And Computing written by Tommy W. S. Chow and has been published by Imperial College Press this book supported file pdf, txt, epub, kindle and other format this book has been release on 2007 with Computers categories.
This book covers neural networks with special emphasis on advanced learning methodologies and applications. It includes practical issues of weight initializations, stalling of learning, and escape from a local minima, which have not been covered by many existing books in this area. Additionally, the book highlights the important feature selection problem, which baffles many neural networks practitioners because of the difficulties handling large datasets. It also contains several interesting IT, engineering and bioinformatics applications.
Deep Learning
DOWNLOAD
Author : Siddhartha Bhattacharyya
language : en
Publisher: Walter de Gruyter GmbH & Co KG
Release Date : 2020-06-22
Deep Learning written by Siddhartha Bhattacharyya and has been published by Walter de Gruyter GmbH & Co KG this book supported file pdf, txt, epub, kindle and other format this book has been release on 2020-06-22 with Computers categories.
This book focuses on the fundamentals of deep learning along with reporting on the current state-of-art research on deep learning. In addition, it provides an insight of deep neural networks in action with illustrative coding examples. Deep learning is a new area of machine learning research which has been introduced with the objective of moving ML closer to one of its original goals, i.e. artificial intelligence. Deep learning was developed as an ML approach to deal with complex input-output mappings. While traditional methods successfully solve problems where final value is a simple function of input data, deep learning techniques are able to capture composite relations between non-immediately related fields, for example between air pressure recordings and English words, millions of pixels and textual description, brand-related news and future stock prices and almost all real world problems. Deep learning is a class of nature inspired machine learning algorithms that uses a cascade of multiple layers of nonlinear processing units for feature extraction and transformation. Each successive layer uses the output from the previous layer as input. The learning may be supervised (e.g. classification) and/or unsupervised (e.g. pattern analysis) manners. These algorithms learn multiple levels of representations that correspond to different levels of abstraction by resorting to some form of gradient descent for training via backpropagation. Layers that have been used in deep learning include hidden layers of an artificial neural network and sets of propositional formulas. They may also include latent variables organized layer-wise in deep generative models such as the nodes in deep belief networks and deep boltzmann machines. Deep learning is part of state-of-the-art systems in various disciplines, particularly computer vision, automatic speech recognition (ASR) and human action recognition.
Advances In Neural Networks Isnn 2018
DOWNLOAD
Author : Tingwen Huang
language : en
Publisher: Springer
Release Date : 2018-05-25
Advances In Neural Networks Isnn 2018 written by Tingwen Huang and has been published by Springer this book supported file pdf, txt, epub, kindle and other format this book has been release on 2018-05-25 with Computers categories.
This book constitutes the refereed proceedings of the 15th International Symposium on Neural Networks, ISNN 2018, held in Minsk, Belarus in June 2018.The 98 revised regular papers presented in this volume were carefully reviewed and selected from 214 submissions. The papers cover many topics of neural network-related research including intelligent control, neurodynamic analysis, bio-signal, bioinformatics and biomedical engineering, clustering, classification, forecasting, models, algorithms, cognitive computation, machine learning, and optimization.
Complex Valued Neural Networks Theories And Applications
DOWNLOAD
Author : Akira Hirose
language : en
Publisher: World Scientific
Release Date : 2003-11-18
Complex Valued Neural Networks Theories And Applications written by Akira Hirose and has been published by World Scientific this book supported file pdf, txt, epub, kindle and other format this book has been release on 2003-11-18 with Computers categories.
In recent years, complex-valued neural networks have widened the scope of application in optoelectronics, imaging, remote sensing, quantum neural devices and systems, spatiotemporal analysis of physiological neural systems, and artificial neural information processing. In this first-ever book on complex-valued neural networks, the most active scientists at the forefront of the field describe theories and applications from various points of view to provide academic and industrial researchers with a comprehensive understanding of the fundamentals, features and prospects of the powerful complex-valued networks.
Artificial Neural Networks And Neural Information Processing Icann Iconip 2003
DOWNLOAD
Author : Okyay Kaynak
language : en
Publisher: Springer
Release Date : 2003-08-03
Artificial Neural Networks And Neural Information Processing Icann Iconip 2003 written by Okyay Kaynak and has been published by Springer this book supported file pdf, txt, epub, kindle and other format this book has been release on 2003-08-03 with Computers categories.
The refereed proceedings of the Joint International Conference on Artificial Neural Networks and International Conference on Neural Information Processing, ICANN/ICONIP 2003, held in Istanbul, Turkey, in June 2003. The 138 revised full papers were carefully reviewed and selected from 346 submissions. The papers are organized in topical sections on learning algorithms, support vector machine and kernel methods, statistical data analysis, pattern recognition, vision, speech recognition, robotics and control, signal processing, time-series prediction, intelligent systems, neural network hardware, cognitive science, computational neuroscience, context aware systems, complex-valued neural networks, emotion recognition, and applications in bioinformatics.