Computation In Constrained Stochastic Model Predictive Control Of Linear Systems
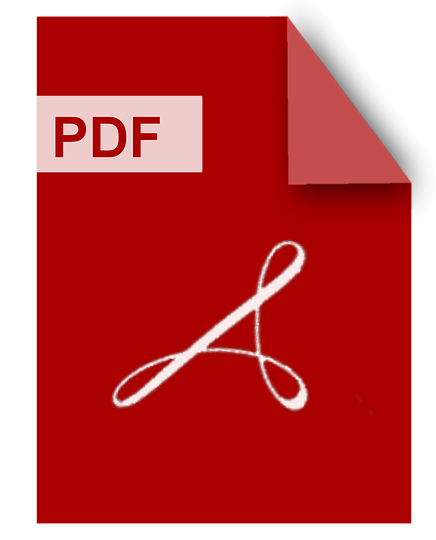
DOWNLOAD
Download Computation In Constrained Stochastic Model Predictive Control Of Linear Systems PDF/ePub or read online books in Mobi eBooks. Click Download or Read Online button to get Computation In Constrained Stochastic Model Predictive Control Of Linear Systems book now. This website allows unlimited access to, at the time of writing, more than 1.5 million titles, including hundreds of thousands of titles in various foreign languages. If the content not found or just blank you must refresh this page
Computation In Constrained Stochastic Model Predictive Control Of Linear Systems
DOWNLOAD
Author : Minyong Shin
language : en
Publisher: Stanford University
Release Date : 2011
Computation In Constrained Stochastic Model Predictive Control Of Linear Systems written by Minyong Shin and has been published by Stanford University this book supported file pdf, txt, epub, kindle and other format this book has been release on 2011 with categories.
Despite its sub-optimality, Model Predictive Control (MPC) has received much attention over the recent decades due to its ability to handle constraints. In particular, stochastic MPC, which includes uncertainty in the system dynamics, is one of the most active recent research topics in MPC. In this dissertation, we focus on (1) increasing computation speed of constrained stochastic MPC of linear systems with additive noise and, (2) improving the accuracy of an approximate solution involving systems with additive and multiplicative noise. Constrained MPC for linear systems with additive noise has been successfully formulated as a semidefinite programming problem (SDP) using the Youla parameterization or innovation feedback and linear matrix inequalities. Unfortunately, this method can be prohibitively slow even for problems with moderate size state. Thus, in this thesis we develop an interior point algorithm which can more efficiently solve the problem. This algorithm converts the stochastic problem into a deterministic one using the mean and the covariance matrix as the system state and using affine feedback. A line search interior point method is then directly applied to the nonlinear deterministic optimization problem. In the process, we take advantage of a recursive structure that appears when a control problem is solved via the line search interior point method in order to decrease the algorithmic complexity of the solution. We compare the computation time and complexity of our algorithm against an SDP solver. The second part of the dissertation deals with systems with additive and multiplicative noise under probabilistic constraints. This class of systems differs from the additive noise case in that the probability distribution of a state is neither Gaussian nor known in closed form. This causes a problem when the probability constraints are dealt with. In previous studies, this problem has been tackled by approximating the state as a Gaussian random variable or by approximating the probability bound as an ellipsoid. In this dissertation, we use the Cornish-Fisher expansion to approximate the probability bounds of the constraints. Since the Cornish-Fisher expansion utilizes quantile values with the first several moments, the probabilistic constraints have the same form as those in the additive noise case when the constraints are converted to deterministic ones. This makes the procedure smooth when we apply the developed algorithm to a linear system with multiplicative noise. Moreover, we can easily extend the application of the algorithm to a linear system with additive plus multiplicative noise.
Computation In Constrained Stochastic Model Predictive Control Of Linear Systems
DOWNLOAD
Author : Minyong Shin
language : en
Publisher:
Release Date : 2011
Computation In Constrained Stochastic Model Predictive Control Of Linear Systems written by Minyong Shin and has been published by this book supported file pdf, txt, epub, kindle and other format this book has been release on 2011 with categories.
Despite its sub-optimality, Model Predictive Control (MPC) has received much attention over the recent decades due to its ability to handle constraints. In particular, stochastic MPC, which includes uncertainty in the system dynamics, is one of the most active recent research topics in MPC. In this dissertation, we focus on (1) increasing computation speed of constrained stochastic MPC of linear systems with additive noise and, (2) improving the accuracy of an approximate solution involving systems with additive and multiplicative noise. Constrained MPC for linear systems with additive noise has been successfully formulated as a semidefinite programming problem (SDP) using the Youla parameterization or innovation feedback and linear matrix inequalities. Unfortunately, this method can be prohibitively slow even for problems with moderate size state. Thus, in this thesis we develop an interior point algorithm which can more efficiently solve the problem. This algorithm converts the stochastic problem into a deterministic one using the mean and the covariance matrix as the system state and using affine feedback. A line search interior point method is then directly applied to the nonlinear deterministic optimization problem. In the process, we take advantage of a recursive structure that appears when a control problem is solved via the line search interior point method in order to decrease the algorithmic complexity of the solution. We compare the computation time and complexity of our algorithm against an SDP solver. The second part of the dissertation deals with systems with additive and multiplicative noise under probabilistic constraints. This class of systems differs from the additive noise case in that the probability distribution of a state is neither Gaussian nor known in closed form. This causes a problem when the probability constraints are dealt with. In previous studies, this problem has been tackled by approximating the state as a Gaussian random variable or by approximating the probability bound as an ellipsoid. In this dissertation, we use the Cornish-Fisher expansion to approximate the probability bounds of the constraints. Since the Cornish-Fisher expansion utilizes quantile values with the first several moments, the probabilistic constraints have the same form as those in the additive noise case when the constraints are converted to deterministic ones. This makes the procedure smooth when we apply the developed algorithm to a linear system with multiplicative noise. Moreover, we can easily extend the application of the algorithm to a linear system with additive plus multiplicative noise.
Handbook Of Model Predictive Control
DOWNLOAD
Author : Saša V. Raković
language : en
Publisher: Springer
Release Date : 2018-09-01
Handbook Of Model Predictive Control written by Saša V. Raković and has been published by Springer this book supported file pdf, txt, epub, kindle and other format this book has been release on 2018-09-01 with Science categories.
Recent developments in model-predictive control promise remarkable opportunities for designing multi-input, multi-output control systems and improving the control of single-input, single-output systems. This volume provides a definitive survey of the latest model-predictive control methods available to engineers and scientists today. The initial set of chapters present various methods for managing uncertainty in systems, including stochastic model-predictive control. With the advent of affordable and fast computation, control engineers now need to think about using “computationally intensive controls,” so the second part of this book addresses the solution of optimization problems in “real” time for model-predictive control. The theory and applications of control theory often influence each other, so the last section of Handbook of Model Predictive Control rounds out the book with representative applications to automobiles, healthcare, robotics, and finance. The chapters in this volume will be useful to working engineers, scientists, and mathematicians, as well as students and faculty interested in the progression of control theory. Future developments in MPC will no doubt build from concepts demonstrated in this book and anyone with an interest in MPC will find fruitful information and suggestions for additional reading.
Robust Stochastic Model Predictive Control
DOWNLOAD
Author : Qifeng Cheng
language : en
Publisher:
Release Date : 2012
Robust Stochastic Model Predictive Control written by Qifeng Cheng and has been published by this book supported file pdf, txt, epub, kindle and other format this book has been release on 2012 with categories.
In the thesis, two different model predictive control (MPC) strategies are investigated for linear systems with uncertainty in the presence of constraints: namely robust MPC and stochastic MPC. Firstly, a Youla Parameter is integrated into an efficient robust MPC algorithm. It is demonstrated that even in the constrained cases, the use of the Youla Parameter can desensitize the costs to the effect of uncertainty while not affecting the nominal performance, and hence it strengthens the robustness of the MPC strategy. Since the controller u = KLQX + c can offer many advantages and is used across the thesis, the work provides two solutions to the problem when the unconstrained nominal LQ-optimal feedback KLQ cannot stabilise the whole class of system models. The work develops two stochastic tube approaches to account for probabilistic constraints. By using a semi closed-loop paradigm, the nominal and the error dynamics are analyzed separately, and this makes it possible to compute the tube scalings offline. First, ellipsoidal tubes are considered. The evolution for the tube scalings is simplified to be affine and using Markov Chain model, the probabilistic tube scalings can be calculated to tighten the constraints on the nominal. The on- line algorithm can be formulated into a quadratic programming (QP) problem and the MPC strategy is closed-loop stable. Following that, a direct way to compute the tube scalings is studied. It makes use of the information on the distribution of the uncertainty explicitly. The tubes do not take a particular shape but are defined implicitly by tightened constraints. This stochastic MPC strategy leads to a non-conservative performance in the sense that the probability of constraint violation can be as large as is allowed. It also ensures the recursive feasibility and closed-loop stability, and is extended to the output feedback case.
Model Predictive Control
DOWNLOAD
Author : Basil Kouvaritakis
language : en
Publisher: Springer
Release Date : 2015-12-01
Model Predictive Control written by Basil Kouvaritakis and has been published by Springer this book supported file pdf, txt, epub, kindle and other format this book has been release on 2015-12-01 with Technology & Engineering categories.
For the first time, a textbook that brings together classical predictive control with treatment of up-to-date robust and stochastic techniques. Model Predictive Control describes the development of tractable algorithms for uncertain, stochastic, constrained systems. The starting point is classical predictive control and the appropriate formulation of performance objectives and constraints to provide guarantees of closed-loop stability and performance. Moving on to robust predictive control, the text explains how similar guarantees may be obtained for cases in which the model describing the system dynamics is subject to additive disturbances and parametric uncertainties. Open- and closed-loop optimization are considered and the state of the art in computationally tractable methods based on uncertainty tubes presented for systems with additive model uncertainty. Finally, the tube framework is also applied to model predictive control problems involving hard or probabilistic constraints for the cases of multiplicative and stochastic model uncertainty. The book provides: extensive use of illustrative examples; sample problems; and discussion of novel control applications such as resource allocation for sustainable development and turbine-blade control for maximized power capture with simultaneously reduced risk of turbulence-induced damage. Graduate students pursuing courses in model predictive control or more generally in advanced or process control and senior undergraduates in need of a specialized treatment will find Model Predictive Control an invaluable guide to the state of the art in this important subject. For the instructor it provides an authoritative resource for the construction of courses.
Constrained Model Predictive Control State Estimation And Coordination
DOWNLOAD
Author :
language : en
Publisher:
Release Date : 2006
Constrained Model Predictive Control State Estimation And Coordination written by and has been published by this book supported file pdf, txt, epub, kindle and other format this book has been release on 2006 with categories.
In this dissertation, we study the interaction between the control performance and the quality of the state estimation in a constrained Model Predictive Control (MPC) framework for systems with stochastic disturbances. This consists of three parts: (i) the development of a constrained MPC formulation that adapts to the quality of the state estimation via constraints; (ii) the application of such a control law in a multi-vehicle formation coordinated control problem in which each vehicle operates subject to a no-collision constraint posed by others' imperfect prediction computed from finite bit-rate, communicated data; (iii) the design of the predictors and the communication resource assignment problem that satisfy the performance requirement from Part (ii). Model Predictive Control (MPC) is of interest because it is one of the few control design methods which preserves standard design variables and yet handles constraints. MPC is normally posed as a full-state feedback control and is implemented in a certainty-equivalence fashion with best estimates of the states being used in place of the exact state. However, if the state constraints were handled in the same certainty-equivalence fashion, the resulting control law could drive the real state to violate the constraints frequently. Part (i) focuses on exploring the inclusion of state estimates into the constraints. It does this by applying constrained MPC to a system with stochastic disturbances. The stochastic nature of the problem requires re-posing the constraints in a probabilistic form. Using a gaussian assumption, the original problem is approximated by a standard deterministic constrained MPC problem or the conditional mean process of the state (the prediction). The state estimates' conditional covariances appear in tightening the constraints as measuring the necessary standoff from the bound on the real state. `Closed-loop covariance' is introduced to reduce the infeasibility and the conservativeness caused by using long-horizon, open-loop prediction covariances. The resulting control law is applied to a telecommunications network traffic control problem as an example. The idea of posing and transforming a probabilistic MPC problem works well, but not limited to, linear systems. In Part (ii), we consider applying constrained MPC as a local control law in a coordinated control problem of a group of distributed autonomous systems. Interactions between the systems are captured via constraints. First, we inspect the application of constrained MPC to a completely deterministic case. Formation stability theorems are derived for the subsystems and conditions on the local constraint set are derived in order to guarantee local stability or convergence to a target state. If these conditions are met for all subsystems, then this stability is inherited by the overall system. For the case when each subsystem suffers from disturbances in the dynamics, own self-measurement noises, and quantization errors on neighbors' information due to the finite-bit-rate channels, the constrained MPC strategy developed in Part (i) is appropriate to apply. Disturbance attenuation, or ``string stability", is studied in this framework and it is shown that inactivity of the MPC constraints implies stability. This then provides a connection between control objective, communications resource assignment and performance. A one-dimensional vehicle example is computed to crystallize ideas. The application of this part is not restricted to linear systems. In Part (iii), we discuss the local predictor design and bandwidth assignment problem in a coordinated vehicle formation context. The MPC controller used in Part (ii) relates the formation control performance and the information quality in the way that large standoff implies conservative performance. If the communication channels used to exchange local information are noiseless, but have only finite bit-rate, the bits assigned to each variable in the information package will change the prediction error covariance, and hence the control performance, via the quantization errors which can be regarded as measurement noises. In this part, we aim at deriving the minimal communication resource and the corresponding bit-rate assignment strategy the corresponding stable state predictors that is used to formulate the MPC constraints. We first develop an LMI (Linear Matrix Inequality) formulation for cross-estimator design in a simple two-vehicle scenario with non-standard information: one vehicle does not have access to the other's exact control value applied at each sampling time, but to its known, pre-computed, coupling linear feedback control law. Then a similar LMI problem is formulated for the bandwidth assignment problem that minimizes the total number of bits by adjusting the prediction gain matrices and the number of bits assigned to each variable. This LMI formulation takes care of the constraint on steady state prediction error covariance imposed by the formation performance requirement, the constraint on the limited total bandwidth, and the constraint on the predictors being stable. Some linear approximation is used to include the bandwidth assignment variables in the LMI formulation. The solution of the resulting LMIs guarantees the feasibility of the bandwidth assignment scheme and stable predictors, but not optimality. An example of a three-vehicle formation is also provided. The LMI formulation here is restricted to linear systems.
Aperiodically Sampled Stochastic Model Predictive Control
DOWNLOAD
Author : Jicheng Chen
language : en
Publisher:
Release Date : 2021
Aperiodically Sampled Stochastic Model Predictive Control written by Jicheng Chen and has been published by this book supported file pdf, txt, epub, kindle and other format this book has been release on 2021 with categories.
Stochastic model predictive control (MPC) is a fascinating field for research and of increasing practical importance since optimal control techniques have been intensively investigated in modern control system design. With the development of computer technologies and communication networks, networked control systems (NCSs) or cyber-physical systems (CPSs) have become an interest of research due to the comprehensive integration of physical systems, such as sensors, actuators and plants, with intricate cyber components, possessing information communication and computation. In CPSs, advantages of low installation cost, high reliability, flexible modularity, improved efficiency, and greater autonomy can be obtained by the tight coordination of physical and cyber components. Several sectors, including robotics, transportation, health care, smart buildings, and smart grid, have witnessed the successful application of CPSs design. The integration of extensive cyber capability and physical plants with ubiquitous uncertainties also introduces concerns over communication efficiency, robustness and stability of the CPSs. Thus, to achieve satisfactory performance metrics of efficiency, robustness and stability, a detailed investigation into control synthesis of CPSs under the stochastic model predictive control framework is of importance. The stochastic model predictive control synthesis plays a vital role in CPSs design since the multivariable stochastic system subject to probabilistic constraints can be controlled in an optimized way. On the other hand, aperiodically sampled, or event-based, model predictive control has also been applied to CPSs extensively to improve communication efficiency. In this thesis, the control synthesis and analysis of aperiodically sampled stochastic model predictive control for CPSs is considered. Chapter 1 provides an introductory literature review of the current development of stochastic MPC, distributed stochastic MPC and event-based MPC. Chapter 2 presents a stochastic self-triggered model predictive control scheme for linear systems with additive uncertainty and with the states and inputs being subject to chance constraints. In the proposed control scheme, the succeeding sampling time instant and current control inputs are computed online by solving a formulated optimization problem. Chapter 3 discusses a stochastic self-triggered model predictive control algorithm with an adaptive prediction horizon. The communication cost is explicitly considered by adding a damping factor in the cost function. Sufficient conditions are provided to guarantee closed-loop chance constraints satisfactions. Furthermore, the recursive feasibility of the algorithm is analyzed, and the closed-loop system is shown to be stable. Chapter 4 proposes a distributed self-triggered stochastic MPC control scheme for CPSs under coupled chance constraints and additive disturbances. Based on the assumptions on stochastic disturbances, both local and coupled probabilistic constraints are transformed into the deterministic form using the tube-based method, and improved terminal constraints are constructed to guarantee the recursive feasibility of the control scheme. Theoretical analysis has shown that the overall closed-loop CPSs are quadratically stable. Numerical examples illustrate the efficacy of the proposed control method in terms of data transmission reductions. Chapter 5 concludes the thesis and suggests some promising directions for future research.
Accelerating The Calculation Of Model Predictive Control Laws For Constrained Linear Systems
DOWNLOAD
Author : Michael Jost
language : en
Publisher:
Release Date : 2015
Accelerating The Calculation Of Model Predictive Control Laws For Constrained Linear Systems written by Michael Jost and has been published by this book supported file pdf, txt, epub, kindle and other format this book has been release on 2015 with categories.
Predictive Control Under Uncertainty
DOWNLOAD
Author : Matthias Lorenzen
language : de
Publisher:
Release Date : 2019-09-18
Predictive Control Under Uncertainty written by Matthias Lorenzen and has been published by this book supported file pdf, txt, epub, kindle and other format this book has been release on 2019-09-18 with categories.
This thesis addresses constrained control of systems under stochastic disturbances and model uncertainty. In particular, stochastic and adaptive model predictive control (MPC) algorithms that solve such problems are presented and studied.Given a stochastic disturbance model, the focus is put on chance constraints, tractable approximations, and their implication on feasibility of the online optimization in a receding horizon framework. For systems with model uncertainty, online parameter identification to reduce conservatism and improve closed-loop performance is addressed.More specifically, building upon the analysis of conceptual aspects, we develop computational approaches for linear systems with additive and multiplicative disturbances. The analysis of a non-conservative, computationally tractable relaxation of chance constraints leads to an important separation of sufficient conditions for feasibility and stability. The latter is of particular interest for rigorously applying approximations, such as finite sample approximations, to solve the online stochastic optimal control problem. We discuss the differences between online and offline sampling approximations and, for systems that are linear in the state and input variables, provide explicit bounds on the sample complexity to guarantee satisfaction of chance constraints with a user chosen confidence. The proposed algorithms provide rigorous guarantees for relevant properties like feasibility of the online optimization, constraint satisfaction, and convergence of the closed-loop system.
Performance And Constraint Satisfaction In Robust Economic Model Predictive Control
DOWNLOAD
Author : Florian A. Bayer
language : en
Publisher: Logos Verlag Berlin GmbH
Release Date : 2017
Performance And Constraint Satisfaction In Robust Economic Model Predictive Control written by Florian A. Bayer and has been published by Logos Verlag Berlin GmbH this book supported file pdf, txt, epub, kindle and other format this book has been release on 2017 with Technology & Engineering categories.
In this thesis, we develop a novel framework for model predictive control (MPC) which combines the concepts of robust MPC and economic MPC. The goal of this thesis is to develop and analyze MPC schemes for nonlinear discrete-time systems which explicitly consider the influence of disturbances on arbitrary performance criteria. Instead of regarding the two aspects separately, we propose robust economic MPC approaches that integrate information which is available about the disturbance directly into the economic framework. In more detail, we develop three concepts which differ in which information about the disturbance is used and how this information is taken into account. Furthermore, we provide a thorough theoretical analysis for each of the three approaches. To this end, we present results on the asymptotic average performance as well as on optimal operating regimes. Optimal operating regimes are closely related to the notion of dissipativity, which is therefore analyzed for the presented concepts. Under suitable assumptions, results on necessity and sufficiency of dissipativity for optimal steady-state operation are established for all three robust economic MPC concepts. A detailed discussion is provided which compares the different performance statements derived for the approaches as well as the respective notions of dissipativity.