Data Assimilation And Precision Annealing Monte Carlo Method In Nonlinear Dynamical Systems
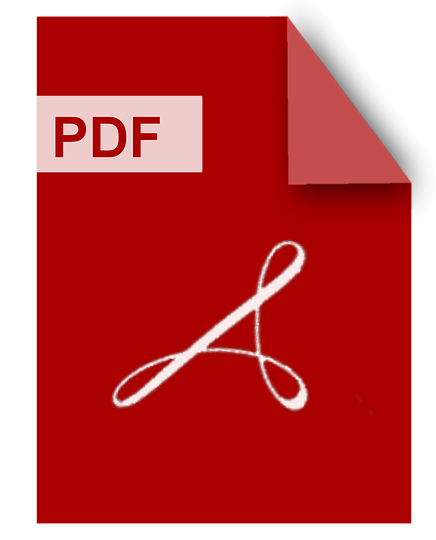
DOWNLOAD
Download Data Assimilation And Precision Annealing Monte Carlo Method In Nonlinear Dynamical Systems PDF/ePub or read online books in Mobi eBooks. Click Download or Read Online button to get Data Assimilation And Precision Annealing Monte Carlo Method In Nonlinear Dynamical Systems book now. This website allows unlimited access to, at the time of writing, more than 1.5 million titles, including hundreds of thousands of titles in various foreign languages. If the content not found or just blank you must refresh this page
Data Assimilation And Precision Annealing Monte Carlo Method In Nonlinear Dynamical Systems
DOWNLOAD
Author : Kangbo Hao
language : en
Publisher:
Release Date : 2020
Data Assimilation And Precision Annealing Monte Carlo Method In Nonlinear Dynamical Systems written by Kangbo Hao and has been published by this book supported file pdf, txt, epub, kindle and other format this book has been release on 2020 with categories.
In the study of data assimilation, people focus on estimating state variables and parameters of dynamical models, and make predictions forward in time, using given observations. It is a method that has been applied to many different fields, such as numerical weather prediction and neurobiology. To make successful estimations and predictions using data assimilation methods, there are a few difficulties that are often encountered. First is the quantity and quality of the data. In some of the typical problems in data assimilation, the number of observations are usually a few order of magnitude smaller than the number of total variables. Considering this and the fact that almost all the data gathered are noisy, how to estimate the observed and unobserved state variables and make good predictions using the noisy and incomplete data is one of the key challenge in data assimilation. Another issue arises from the dynamical model. Most of the interesting models are non-linear, and usually chaotic, which means that a small error in the estimation will grow exponentially over time. This property of the chaotic system addresses the necessity of accurate estimations of variables. In this thesis, I will start with an overview of data assimilation, by formulating the problem that data assimilation tries to solve, and introducing several widely used methods. Then I will explain the Precision Annealing Monte Carlo method that has been developed in the group, as well as its variation using Hamiltonian Monte Carlo. Finally I will demonstrate a few example problems that can be solved using data assimilation methods, varying from a simple but instructional 20-dimension Lorenz 96 model, to a complicated ocean model named Regional Ocean Modeling System.
Strategic Monte Carlo And Variational Methods In Statistical Data Assimilation For Nonlinear Dynamical Systems
DOWNLOAD
Author : Aleksandra Shirman
language : en
Publisher:
Release Date : 2018
Strategic Monte Carlo And Variational Methods In Statistical Data Assimilation For Nonlinear Dynamical Systems written by Aleksandra Shirman and has been published by this book supported file pdf, txt, epub, kindle and other format this book has been release on 2018 with categories.
Data Assimilation (DA) is a method through which information is extracted from measured quantities and with the help of a mathematical model is transferred through a probability distribution to unknown or unmeasured states and parameters characterizing the system of study. With an estimate of the model paramters, quantitative predictions may be made and compared to subsequent data. Many recent DA efforts rely on an probability distribution optimization that locates the most probable state and parameter values given a set of data. The procedure developed and demonstrated here extends the optimization by appending a biased random walk around the states and parameters of high probability to generate an estimate of the structure in state space of the probability density function (PDF). The estimate of the structure of the PDF will facilitate more accurate estimates of expectation values of means, standard deviations and higher moments of states and parameters that characterize the behavior of the system of study. The ability to calculate these expectation values will allow for an error bar or tolerance interval to be attached to each estimated state or parameter, in turn giving significance to any results generated. The estimation method's merits will be demonstrated on a simulated well known chaotic system, the Lorenz 96 system, and on a toy model of a neuron. In both situations the model system provides unique challenges for estimation: In chaotic systems any small error in estimation generates extremely large prediction errors while in neurons only one of the (at minimum) four dynamical variables can be measured leading to a small amount of data with which to work. This thesis will conclude with an exploration of the equivalence of machine learning and the formulation of statistical DA. The application of previous DA methods are demonstrated on the classic machine learning problem: the characterization of handwritten images from the MNIST data set. The results of this work are used to validate common assumptions in machine learning work such as the dependence of the quality of results on the amount of data presented and the size of the network used. Finally DA is proposed as a method through which to discern an 'ideal' network size for a set of given data which optimizes predictive capabilities while minimizing computational costs.
The Statistical Physics Of Data Assimilation And Machine Learning
DOWNLOAD
Author : Henry D. I. Abarbanel
language : en
Publisher: Cambridge University Press
Release Date : 2022-02-17
The Statistical Physics Of Data Assimilation And Machine Learning written by Henry D. I. Abarbanel and has been published by Cambridge University Press this book supported file pdf, txt, epub, kindle and other format this book has been release on 2022-02-17 with Computers categories.
The theory of data assimilation and machine learning is introduced in an accessible manner for undergraduate and graduate students.
Methods For Inference And Prediction In Nonlinear Dynamical Systems
DOWNLOAD
Author : Zheng Fang
language : en
Publisher:
Release Date : 2021
Methods For Inference And Prediction In Nonlinear Dynamical Systems written by Zheng Fang and has been published by this book supported file pdf, txt, epub, kindle and other format this book has been release on 2021 with categories.
Transferring information from data to models is crucial to many scientific disciplines. Typically, the data collected are noisy, and the total number of degrees of freedom of the model far exceeds that of the data. For data assimilation in which a physical dynamical system is of interest, one could usually observe only a subset of the vector state of the system at any given time. For an artificial neural network that may be formulated as a dynamical model, observations are limited to only the input and output layers; the network topology of the hidden layers remains flexible. As a result, to train such dynamical models, it is necessary to simultaneously estimate both the observed and unobserved degrees of freedom in the models, along with all the time-independent parameters. These requirements bring significant challenges to the task. This dissertation develops methods for systematically transferring information from noisy, partial data into nonlinear dynamical models. A theoretical basis for all these methods is first formulated. Specifically, a high-dimensional probability distribution containing the structure of the dynamics and the data is derived. The task can then be formally cast as the evaluation of an expected-value integral under that probability distribution. A well-studied sampling procedure called Hamiltonian Monte Carlo is then introduced as a functioning part to be combined with Precision Annealing, a framework for gradually enforcing the model constraints into the probability distribution. Numerical applications are then demonstrated on two physical dynamical systems. In each case, inferences are made for both the model states within the observation window and the time-independent parameters.-Once complete, the predictive power of the model is then validated by additional data. Following these is a discussion of the role of the state-space representation. The dissertation concludes with an exploration of new methods for training artificial neural networks without using the well-known backpropagation procedure. Given the equivalence between the structure of an artificial neural network and that of a dynamical system, the aforementioned theoretical basis is applicable in this arena. The computational results presented indicate promising potentials of the proposed methods.
The Statistical Physics Of Data Assimilation And Machine Learning
DOWNLOAD
Author : Henry D. I. Abarbanel
language : en
Publisher: Cambridge University Press
Release Date : 2022-02-17
The Statistical Physics Of Data Assimilation And Machine Learning written by Henry D. I. Abarbanel and has been published by Cambridge University Press this book supported file pdf, txt, epub, kindle and other format this book has been release on 2022-02-17 with Science categories.
Data assimilation is a hugely important mathematical technique, relevant in fields as diverse as geophysics, data science, and neuroscience. This modern book provides an authoritative treatment of the field as it relates to several scientific disciplines, with a particular emphasis on recent developments from machine learning and its role in the optimisation of data assimilation. Underlying theory from statistical physics, such as path integrals and Monte Carlo methods, are developed in the text as a basis for data assimilation, and the author then explores examples from current multidisciplinary research such as the modelling of shallow water systems, ocean dynamics, and neuronal dynamics in the avian brain. The theory of data assimilation and machine learning is introduced in an accessible and unified manner, and the book is suitable for undergraduate and graduate students from science and engineering without specialized experience of statistical physics.
Data Assimilation And Control Theory And Applications In Life Sciences
DOWNLOAD
Author : Axel Hutt
language : en
Publisher: Frontiers Media SA
Release Date : 2019-08-16
Data Assimilation And Control Theory And Applications In Life Sciences written by Axel Hutt and has been published by Frontiers Media SA this book supported file pdf, txt, epub, kindle and other format this book has been release on 2019-08-16 with categories.
The understanding of complex systems is a key element to predict and control the system’s dynamics. To gain deeper insights into the underlying actions of complex systems today, more and more data of diverse types are analyzed that mirror the systems dynamics, whereas system models are still hard to derive. Data assimilation merges both data and model to an optimal description of complex systems’ dynamics. The present eBook brings together both recent theoretical work in data assimilation and control and demonstrates applications in diverse research fields.
Dynamic Data Assimilation
DOWNLOAD
Author : John M. Lewis
language : en
Publisher: Cambridge University Press
Release Date : 2006-08-03
Dynamic Data Assimilation written by John M. Lewis and has been published by Cambridge University Press this book supported file pdf, txt, epub, kindle and other format this book has been release on 2006-08-03 with Mathematics categories.
Publisher description
Nonlinear Data Assimilation
DOWNLOAD
Author : Peter Jan Van Leeuwen
language : en
Publisher:
Release Date : 2015
Nonlinear Data Assimilation written by Peter Jan Van Leeuwen and has been published by this book supported file pdf, txt, epub, kindle and other format this book has been release on 2015 with categories.
This book contains two review articles on nonlinear data assimilation that deal with closely related topics but were written and can be read independently. Both contributions focus on so-called particle filters. The first contribution by Jan van Leeuwen focuses on the potential of proposal densities. It discusses the issues with present-day particle filters and explorers new ideas for proposal densities to solve them, converging to particle filters that work well in systems of any dimension, closing the contribution with a high-dimensional example. The second contribution by Cheng and Reich discusses a unified framework for ensemble-transform particle filters. This allows one to bridge successful ensemble Kalman filters with fully nonlinear particle filters, and allows a proper introduction of localization in particle filters, which has been lacking up to now.
Systematic Annealing Approach For Statistical Data Assimilation
DOWNLOAD
Author : Jingxin Ye
language : en
Publisher:
Release Date : 2016
Systematic Annealing Approach For Statistical Data Assimilation written by Jingxin Ye and has been published by this book supported file pdf, txt, epub, kindle and other format this book has been release on 2016 with categories.
Data assimilation transfers information from observations of a complex system to physically-based system models. Typically, the observations are noisy, the model has errors, and the initial state of the model is uncertain, so the data assimilation is statistical. In statistical data assimilation one evaluates the conditional expected values, conditioned on measurements, of interesting quantities on the path of a model through observation and prediction windows. This often requires working with very high dimensional integrals in discrete time descriptions of the observations and model dynamics, which become function integrals in the continuous time limit. Two familiar methods for performing these integrals include (1) Monte Carlo calculations and (2) variational approximations using the method of Laplace plus perturbative corrections to the dominant contributions. We attend here to aspects of the Laplace approximation and develop an annealing method for locating the variational path satisfying the Euler- Lagrange equations that comprises the major contribution to the integrals. This begins with the identification of the minimum action path starting with a situation where the model dynamics is totally unresolved in state space, and the consistent minimum of the variational problem is known. We then proceed to slowly increase the model resolution, seeking to remain in the basin of the minimum action path, until a path that gives the dominant contribution to the integral is identified. After a discussion of some general issues, we give examples of the assimilation process for some simple, instructive models from the geophysical literature. Then we explore a slightly richer model of the same type with two distinct time scales. This is followed by a model characterizing the biophysics of individual neurons.
Data Assimilation With Gaussian Mixture Models Using The Dynamically Orthogonal Field Equations
DOWNLOAD
Author : Thomas Sondergaard (S.M.)
language : en
Publisher:
Release Date : 2011
Data Assimilation With Gaussian Mixture Models Using The Dynamically Orthogonal Field Equations written by Thomas Sondergaard (S.M.) and has been published by this book supported file pdf, txt, epub, kindle and other format this book has been release on 2011 with categories.
Data assimilation, as presented in this thesis, is the statistical merging of sparse observational data with computational models so as to optimally improve the probabilistic description of the field of interest, thereby reducing uncertainties. The centerpiece of this thesis is the introduction of a novel such scheme that overcomes prior shortcomings observed within the community. Adopting techniques prevalent in Machine Learning and Pattern Recognition, and building on the foundations of classical assimilation schemes, we introduce the GMM-DO filter: Data Assimilation with Gaussian mixture models using the Dynamically Orthogonal field equations. We combine the use of Gaussian mixture models, the EM algorithm and the Bayesian Information Criterion to accurately approximate distributions based on Monte Carlo data in a framework that allows for efficient Bayesian inference. We give detailed descriptions of each of these techniques, supporting their application by recent literature. One novelty of the GMM-DO filter lies in coupling these concepts with an efficient representation of the evolving probabilistic description of the uncertain dynamical field: the Dynamically Orthogonal field equations. By limiting our attention to a dominant evolving stochastic subspace of the total state space, we bridge an important gap previously identified in the literature caused by the dimensionality of the state space. We successfully apply the GMM-DO filter to two test cases: (1) the Double Well Diffusion Experiment and (2) the Sudden Expansion fluid flow. With the former, we prove the validity of utilizing Gaussian mixture models, the EM algorithm and the Bayesian Information Criterion in a dynamical systems setting. With the application of the GMM-DO filter to the two-dimensional Sudden Expansion fluid flow, we further show its applicability to realistic test cases of non-trivial dimensionality. The GMMDO filter is shown to consistently capture and retain the far-from-Gaussian statistics that arise, both prior and posterior to the assimilation of data, resulting in its superior performance over contemporary filters. We present the GMM-DO filter as an efficient, data-driven assimilation scheme, focused on a dominant evolving stochastic subspace of the total state space, that respects nonlinear dynamics and captures non-Gaussian statistics, obviating the use of heuristic arguments.