Data Driven Clinical Decision Making Using Deep Learning In Imaging
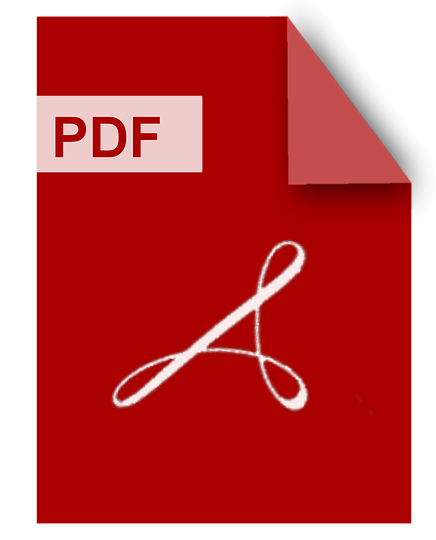
DOWNLOAD
Download Data Driven Clinical Decision Making Using Deep Learning In Imaging PDF/ePub or read online books in Mobi eBooks. Click Download or Read Online button to get Data Driven Clinical Decision Making Using Deep Learning In Imaging book now. This website allows unlimited access to, at the time of writing, more than 1.5 million titles, including hundreds of thousands of titles in various foreign languages. If the content not found or just blank you must refresh this page
Data Driven Clinical Decision Making Using Deep Learning In Imaging
DOWNLOAD
Author : M. F. Mridha
language : en
Publisher: Springer Nature
Release Date : 2024-08-13
Data Driven Clinical Decision Making Using Deep Learning In Imaging written by M. F. Mridha and has been published by Springer Nature this book supported file pdf, txt, epub, kindle and other format this book has been release on 2024-08-13 with Computers categories.
This book explores cutting-edge medical imaging advancements and their applications in clinical decision-making. The book contains various topics, methodologies, and applications, providing readers with a comprehensive understanding of the field's current state and prospects. It begins with exploring domain adaptation in medical imaging and evaluating the effectiveness of transfer learning to overcome challenges associated with limited labeled data. The subsequent chapters delve into specific applications, such as improving kidney lesion classification in CT scans, elevating breast cancer research through attention-based U-Net architecture for segmentation and classifying brain MRI images for neurological disorders. Furthermore, the book addresses the development of multimodal machine learning models for brain tumor prognosis, the identification of unique dermatological signatures using deep transfer learning, and the utilization of generative adversarial networks to enhance breast cancer detection systems by augmenting mammogram images. Additionally, the authors present a privacy-preserving approach for breast cancer risk prediction using federated learning, ensuring the confidentiality and security of sensitive patient data. This book brings together a global network of experts from various corners of the world, reflecting the truly international nature of its research.
Artificial Intelligence In Medical Imaging
DOWNLOAD
Author : Erik R. Ranschaert
language : en
Publisher: Springer
Release Date : 2019-01-29
Artificial Intelligence In Medical Imaging written by Erik R. Ranschaert and has been published by Springer this book supported file pdf, txt, epub, kindle and other format this book has been release on 2019-01-29 with Medical categories.
This book provides a thorough overview of the ongoing evolution in the application of artificial intelligence (AI) within healthcare and radiology, enabling readers to gain a deeper insight into the technological background of AI and the impacts of new and emerging technologies on medical imaging. After an introduction on game changers in radiology, such as deep learning technology, the technological evolution of AI in computing science and medical image computing is described, with explanation of basic principles and the types and subtypes of AI. Subsequent sections address the use of imaging biomarkers, the development and validation of AI applications, and various aspects and issues relating to the growing role of big data in radiology. Diverse real-life clinical applications of AI are then outlined for different body parts, demonstrating their ability to add value to daily radiology practices. The concluding section focuses on the impact of AI on radiology and the implicationsfor radiologists, for example with respect to training. Written by radiologists and IT professionals, the book will be of high value for radiologists, medical/clinical physicists, IT specialists, and imaging informatics professionals.
The Combination Of Data Driven Machine Learning Approaches And Prior Knowledge For Robust Medical Image Processing And Analysis
DOWNLOAD
Author : Jinming Duan
language : en
Publisher: Frontiers Media SA
Release Date : 2024-06-11
The Combination Of Data Driven Machine Learning Approaches And Prior Knowledge For Robust Medical Image Processing And Analysis written by Jinming Duan and has been published by Frontiers Media SA this book supported file pdf, txt, epub, kindle and other format this book has been release on 2024-06-11 with Medical categories.
With the availability of big image datasets and state-of-the-art computing hardware, data-driven machine learning approaches, particularly deep learning, have been used in numerous medical image (CT-scans, MRI, PET, SPECT, etc..) computing tasks, ranging from image reconstruction, super-resolution, segmentation, registration all the way to disease classification and survival prediction. However, training such high-precision approaches often require large amounts of data to be collected and labelled and high-capacity graphics processing units (GPUs) installed, which are resource intensive and hence not always practical. Other hurdles such as the generalization ability to unseen new data and difficulty to interpret and explain can prevent their deployment to those clinical applications which deem such abilities imperative.
Data Driven Decision Making Using Analytics
DOWNLOAD
Author : Parul Gandhi
language : en
Publisher: CRC Press
Release Date : 2021-12-21
Data Driven Decision Making Using Analytics written by Parul Gandhi and has been published by CRC Press this book supported file pdf, txt, epub, kindle and other format this book has been release on 2021-12-21 with Computers categories.
This book aims to explain Data Analytics towards decision making in terms of models and algorithms, theoretical concepts, applications, experiments in relevant domains or focused on specific issues. It explores the concepts of database technology, machine learning, knowledge-based system, high performance computing, information retrieval, finding patterns hidden in large datasets and data visualization. Also, it presents various paradigms including pattern mining, clustering, classification, and data analysis. Overall aim is to provide technical solutions in the field of data analytics and data mining. Features: Covers descriptive statistics with respect to predictive analytics and business analytics. Discusses different data analytics platforms for real-time applications. Explain SMART business models. Includes algorithms in data sciences alongwith automated methods and models. Explores varied challenges encountered by researchers and businesses in the realm of real-time analytics. This book aims at researchers and graduate students in data analytics, data sciences, data mining, and signal processing.
Radiomics And Radiogenomics
DOWNLOAD
Author : Ruijiang Li
language : en
Publisher: CRC Press
Release Date : 2019-07-09
Radiomics And Radiogenomics written by Ruijiang Li and has been published by CRC Press this book supported file pdf, txt, epub, kindle and other format this book has been release on 2019-07-09 with Science categories.
Radiomics and Radiogenomics: Technical Basis and Clinical Applications provides a first summary of the overlapping fields of radiomics and radiogenomics, showcasing how they are being used to evaluate disease characteristics and correlate with treatment response and patient prognosis. It explains the fundamental principles, technical bases, and clinical applications with a focus on oncology. The book’s expert authors present computational approaches for extracting imaging features that help to detect and characterize disease tissues for improving diagnosis, prognosis, and evaluation of therapy response. This book is intended for audiences including imaging scientists, medical physicists, as well as medical professionals and specialists such as diagnostic radiologists, radiation oncologists, and medical oncologists. Features Provides a first complete overview of the technical underpinnings and clinical applications of radiomics and radiogenomics Shows how they are improving diagnostic and prognostic decisions with greater efficacy Discusses the image informatics, quantitative imaging, feature extraction, predictive modeling, software tools, and other key areas Covers applications in oncology and beyond, covering all major disease sites in separate chapters Includes an introduction to basic principles and discussion of emerging research directions with a roadmap to clinical translation
Diverse Perspectives And State Of The Art Approaches To The Utilization Of Data Driven Clinical Decision Support Systems
DOWNLOAD
Author : Connolly, Thomas M.
language : en
Publisher: IGI Global
Release Date : 2022-11-11
Diverse Perspectives And State Of The Art Approaches To The Utilization Of Data Driven Clinical Decision Support Systems written by Connolly, Thomas M. and has been published by IGI Global this book supported file pdf, txt, epub, kindle and other format this book has been release on 2022-11-11 with Business & Economics categories.
The medical domain is home to many critical challenges that stand to be overcome with the use of data-driven clinical decision support systems (CDSS), and there is a growing set of examples of automated diagnosis, prognosis, drug design, and testing. However, the current state of AI in medicine has been summarized as “high on promise and relatively low on data and proof.” If such problems can be addressed, a data-driven approach will be very important to the future of CDSSs as it simplifies the knowledge acquisition and maintenance process, a process that is time-consuming and requires considerable human effort. Diverse Perspectives and State-of-the-Art Approaches to the Utilization of Data-Driven Clinical Decision Support Systems critically reflects on the challenges that data-driven CDSSs must address to become mainstream healthcare systems rather than a small set of exemplars of what might be possible. It further identifies evidence-based, successful data-driven CDSSs. Covering topics such as automated planning, diagnostic systems, and explainable artificial intelligence, this premier reference source is an excellent resource for medical professionals, healthcare administrators, IT managers, pharmacists, students and faculty of higher education, librarians, researchers, and academicians.
Ai And Iot Based Technologies For Precision Medicine
DOWNLOAD
Author : Khang, Alex
language : en
Publisher: IGI Global
Release Date : 2023-10-18
Ai And Iot Based Technologies For Precision Medicine written by Khang, Alex and has been published by IGI Global this book supported file pdf, txt, epub, kindle and other format this book has been release on 2023-10-18 with Medical categories.
In the post-COVID-19 healthcare landscape, the demand for smart healthcare solutions and precision medicine systems has grown significantly. To address these challenges, the book AI and IoT-Based Technologies for Precision Medicine provides a comprehensive resource for doctors, researchers, engineers, and students. By leveraging AI and IoT technologies, the book equips healthcare professionals with advanced tools and methodologies for predictive disease analysis, informed decision-making, and other aspects of precision medicine. This resource bridges the gap between theory and practice, exploring concepts like machine learning, deep learning, computer vision, AI-integrated applications, IoT-based technologies, healthcare data analytics, and biotechnology applications. Through this, the book empowers healthcare practitioners to pioneer innovative solutions that enhance efficiency, accuracy, and security in medical practices. AI and IoT-Based Technologies for Precision Medicine not only offer insights into the potential of AI-powered applications and IoT-equipped techniques in smart healthcare but also foster collaboration among healthcare scholars and professionals. This authoritative guide encourages knowledge sharing and collaboration to harness the transformative potential of AI and IoT, leading to revolutionary advancements in medical practices and healthcare services. With this book as a guide, readers can navigate the evolving landscape of high-tech medicine, taking confident steps toward a cutting-edge and precise medical ecosystem.
Artificial Intelligence In Healthcare
DOWNLOAD
Author : Adam Bohr
language : en
Publisher: Academic Press
Release Date : 2020-06-21
Artificial Intelligence In Healthcare written by Adam Bohr and has been published by Academic Press this book supported file pdf, txt, epub, kindle and other format this book has been release on 2020-06-21 with Computers categories.
Artificial Intelligence (AI) in Healthcare is more than a comprehensive introduction to artificial intelligence as a tool in the generation and analysis of healthcare data. The book is split into two sections where the first section describes the current healthcare challenges and the rise of AI in this arena. The ten following chapters are written by specialists in each area, covering the whole healthcare ecosystem. First, the AI applications in drug design and drug development are presented followed by its applications in the field of cancer diagnostics, treatment and medical imaging. Subsequently, the application of AI in medical devices and surgery are covered as well as remote patient monitoring. Finally, the book dives into the topics of security, privacy, information sharing, health insurances and legal aspects of AI in healthcare. - Highlights different data techniques in healthcare data analysis, including machine learning and data mining - Illustrates different applications and challenges across the design, implementation and management of intelligent systems and healthcare data networks - Includes applications and case studies across all areas of AI in healthcare data
Advances In Data Driven Computing And Intelligent Systems
DOWNLOAD
Author : Swagatam Das
language : en
Publisher: Springer Nature
Release Date : 2024-04-10
Advances In Data Driven Computing And Intelligent Systems written by Swagatam Das and has been published by Springer Nature this book supported file pdf, txt, epub, kindle and other format this book has been release on 2024-04-10 with Technology & Engineering categories.
The volume is a collection of best selected research papers presented at International Conference on Advances in Data-driven Computing and Intelligent Systems (ADCIS 2023) held at BITS Pilani, K K Birla Goa Campus, Goa, India during 21 – 23 September 2023. It includes state-of-the art research work in the cutting-edge technologies in the field of data science and intelligent systems. The book presents data-driven computing; it is a new field of computational analysis which uses provided data to directly produce predictive outcomes. The book will be useful for academicians, research scholars, and industry persons.
Ai Insights On Nuclear Medicine
DOWNLOAD
Author : Satishkumar, D.
language : en
Publisher: IGI Global
Release Date : 2025-04-24
Ai Insights On Nuclear Medicine written by Satishkumar, D. and has been published by IGI Global this book supported file pdf, txt, epub, kindle and other format this book has been release on 2025-04-24 with Medical categories.
The integration of artificial intelligence into nuclear medicine is transforming the field by enhancing diagnostic accuracy, optimizing treatment plans, and expanding patient access to high-quality care. As AI-driven technologies continue to evolve, they offer new opportunities for improving efficiency, reducing human error, and personalizing medical interventions. However, these advancements also come with challenges, requiring careful oversight to ensure ethical implementation, patient safety, and adherence to professional standards. The active involvement of the medical community is essential in shaping the responsible use of AI to maximize its benefits while safeguarding both patients and society. AI Insights on Nuclear Medicine explores the transformative role of artificial intelligence in nuclear medicine, focusing on its applications in diagnostic imaging, treatment planning, and predictive analytics. By leveraging machine learning and automation, AI enhances accuracy, efficiency, and personalized care, ultimately improving patient outcomes and streamlining clinical workflows. Covering topics such as hybrid imaging, precision therapeutics, and decentralized infrastructure, this book is an excellent resource for physicists, computational imaging scientists, physicians, statisticians, industry and regulatory agency representatives, professionals, researchers, scholars, academicians, and more.