Data Mining Frameworks For Energy Consumption Reduction Of Existing Buildings
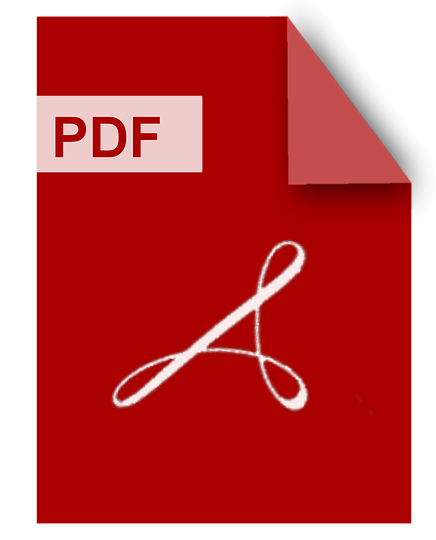
DOWNLOAD
Download Data Mining Frameworks For Energy Consumption Reduction Of Existing Buildings PDF/ePub or read online books in Mobi eBooks. Click Download or Read Online button to get Data Mining Frameworks For Energy Consumption Reduction Of Existing Buildings book now. This website allows unlimited access to, at the time of writing, more than 1.5 million titles, including hundreds of thousands of titles in various foreign languages. If the content not found or just blank you must refresh this page
Data Mining Frameworks For Energy Consumption Reduction Of Existing Buildings
DOWNLOAD
Author : Milad Ashouri Sanjani
language : en
Publisher:
Release Date : 2021
Data Mining Frameworks For Energy Consumption Reduction Of Existing Buildings written by Milad Ashouri Sanjani and has been published by this book supported file pdf, txt, epub, kindle and other format this book has been release on 2021 with categories.
Many technical solutions have been developed to reduce buildings' energy consumption, but limited efforts have been made to adequately address the role or action of building occupants in this process. On the other side, Building Management System (BMS) monitors the performance of buildings by recording the data to improve the building operation, control systems and maintenance. Usually, BMS produces a large volume of data throughout the year including information with regard to patterns of energy use, occupant behavior, etc. The availability of this huge data has created an opportunity to extract information to improve the building energy performance through leveraging powerful data analytic tools. The objectives defined in this thesis lie in developing methodologies to find energy saving opportunities by analyzing data coming from occupants' energy consumption. Three tasks are defined in this thesis. The first task is to provide a recommender system to alert the occupants to take certain measures in order to reduce their energy consumption through end-use loads. Therefore, the quantification of potential savings is provided upon following recommendations. The proposed methodology is also capable to detect the energy saving measures performed by occupants. The second task focuses on a systematic comparison procedure between the buildings to make the occupants aware of their rank among other buildings and hence give them clues on how to improve their performance. The third task focuses on developing a framework to create a reference building acting as a reference for a given building. Therefore, the given building can be compared against its reference building. Potential savings are given to the given building along with directions how to achieve them. The results show successfulness of developed methodologies in finding energy saving opportunities through modifying occupant behavior.
Data Mining And Machine Learning In Building Energy Analysis
DOWNLOAD
Author : Frédéric Magoules
language : en
Publisher: John Wiley & Sons
Release Date : 2016-01-05
Data Mining And Machine Learning In Building Energy Analysis written by Frédéric Magoules and has been published by John Wiley & Sons this book supported file pdf, txt, epub, kindle and other format this book has been release on 2016-01-05 with Computers categories.
The energy consumption of a building has, in recent years, become a determining factor during its design and construction. With carbon footprints being a growing issue, it is important that buildings be optimized for energy conservation and CO2 reduction. This book therefore presents AI models and optimization techniques related to this application. The authors start with a review of recent models for the prediction of building energy consumption: engineering methods, statistical methods, artificial intelligence methods, ANNs and SVMs in particular. The book then focuses on SVMs, by first applying them to building energy consumption, then presenting the principles and various extensions, and SVR. The authors then move on to RDP, which they use to determine building energy faults through simulation experiments before presenting SVR model reduction methods and the benefits of parallel computing. The book then closes by presenting some of the current research and advancements in the field.
Optimizing Energy Performance Of Building Renovation Using Traditional And Machine Learning Approaches
DOWNLOAD
Author : Seyed Amirhosain Sharif Arani
language : en
Publisher:
Release Date : 2020
Optimizing Energy Performance Of Building Renovation Using Traditional And Machine Learning Approaches written by Seyed Amirhosain Sharif Arani and has been published by this book supported file pdf, txt, epub, kindle and other format this book has been release on 2020 with categories.
International Energy Agency (IEA) studies show that buildings are responsible for more than 30% of the total energy consumption and an equally large amount of related greenhouse gas emissions. Improving the energy performance of buildings is a critical element of building energy conservation. Furthermore, renovating existing buildings envelopes and systems offers significant opportunities for reducing Life-Cycle cost (LCC) and minimizing negative environmental impacts. This approach can be considered as one of the key strategies for achieving sustainable development goals at a relatively low cost, especially when compared with the demolition and reconstruction of new buildings. One of the main methodological and technical issues of this approach is selecting a desirable renovation strategy among a wide range of available options. The main motivation behind this research relies on trying to bridge the gap between building simulation, optimization algorithms, and Artificial Intelligence (AI) techniques, to take full advantage of the value of their couplings. Furthermore, for a whole building simulation and optimization, current simulation-based optimization models, often need thousands of simulation evaluations. Therefore, the optimization becomes unfeasible because of the computation time and complexity of the dependent parameters. To this end, one feasible technique to solve this problem is to implement surrogate models to computationally imitate expensive real building simulation models. The aim of this research is three-fold: (1) to propose a Simulation-Based Multi-Objective Optimization (SBMO) model for optimizing the selection of renovation scenarios for existing buildings by minimizing Total Energy Consumption (TEC), LCC and negative environmental impacts considering Life-Cycle Assessment (LCA); (2) to develop surrogate Artificial Neural Networks (ANNs) for selecting near-optimal building energy renovation methods; and (3) to develop generative deep Machine Learning Models (MLMs) to generate renovation scenarios considering TEC and LCC. This study considers three main areas of building renovation, which are the building envelope, Heating, Ventilation and Air-Conditioning (HVAC) system, and lighting system; each of which has a significant impact on building energy performance. On this premise, this research initially develops a framework for data collection and preparation to define the renovation strategies and proposes a comprehensive database including different renovation methods. Using this database, different renovation scenarios can be compared to find the near-optimal scenario based on the renovation strategy. Each scenario is created from the combination of several methods within the applicable strategy. The SBMO model simulates the process of renovating buildings by using the renovation data in energy analysis software to analyze TEC, LCC, and LCA and identifies the near-optimal renovation scenarios based on the selected renovation methods. Furthermore, an LCA tool is used to evaluate the environmental sustainability of the final decision. It is found that, although the proposed SBMO is accurate, the process of simulation is time consuming. To this end, the second objective focuses on developing robust MLMs to explore vast and complex data generated from the SBMO model and develop a surrogate building energy model to predict TEC, LCC, and LCA for all building renovation scenarios. The main advantage of these MLMs is improving the computing time while achieving acceptable accuracy. More specifically, the second developed model integrates the optimization power of SBMO with the modeling capability of ANNs. While, the proposed ANNs are found to provide satisfactory approximation to the SBMO model in a very short period of time, they do not have the capability to generate renovation scenarios. Finally, the third objective focuses on developing a generative deep learning building energy model using Variational Autoencoders (VAEs). The proposed semi-supervised VAEs extract deep features from a whole building renovation dataset and generate renovation scenarios considering TEC and LCC of existing institutional buildings. The proposed model also has the generalization ability due to its potential to reuse the dataset from a specific case in similar situations. The proposed models will potentially offer new venues in two directions: (1) to predict TEC, LCC, and LCA for different renovation scenarios, and select the near-optimal scenario, and (2) to generate renovation scenarios considering TEC and LCC. Architects and engineers can see the effects of different materials, HVAC systems, etc., on the energy consumption, and make necessary changes to increase the energy performance of the building. The proposed models encourage the implementation of sustainable materials and components to decrease negative environmental impacts. The ultimate impact of the practical implementation of this research is significant savings in buildings' energy consumption and having more environmentally friendly buildings within the predefined renovation budget.
Recognizing And Visualizing Energy Consumption Patterns Of Buildings Using Data Mining
DOWNLOAD
Author :
language : en
Publisher:
Release Date : 2015
Recognizing And Visualizing Energy Consumption Patterns Of Buildings Using Data Mining written by and has been published by this book supported file pdf, txt, epub, kindle and other format this book has been release on 2015 with categories.
Season Based Occupancy Prediction In Residential Buildings Using Data Mining Techniques
DOWNLOAD
Author : Bowen Yang
language : en
Publisher:
Release Date : 2021
Season Based Occupancy Prediction In Residential Buildings Using Data Mining Techniques written by Bowen Yang and has been published by this book supported file pdf, txt, epub, kindle and other format this book has been release on 2021 with categories.
Considering the continuous increase of global energy consumption and the fact that buildings account for a large part of electricity use, it is essential to reduce energy consumption in buildings to mitigate greenhouse gas emissions and costs for both building owners and tenants. A reliable occupancy prediction model plays a critical role in improving the performance of energy simulation and occupant-centric building operations. In general, occupancy and occupant activities differ by season, and it is important to account for the dynamic nature of occupancy in simulations and to propose energy-efficient strategies. The present work aims to develop a data mining-based framework, including feature selection and the establishment of seasonal-customized occupancy prediction (SCOP) models to predict the occupancy in buildings considering different seasons. In the proposed framework, the recursive feature elimination with cross-validation (RFECV) feature selection was first implemented to select the optimal variables concerning the highest prediction accuracy. Later, six machine learning (ML) algorithms were considered to establish four SCOP models to predict occupancy presence, and their prediction performances were compared in terms of prediction accuracy and computational cost. To evaluate the effectiveness of the developed data mining framework, it was applied to an apartment in Lyon, France. The results show that the RFECV process reduced the computational time while improving the ML models' prediction performances. Additionally, the SCOP models could achieve higher prediction accuracy than the conventional prediction model measured by performance evaluation metrics of F-1 score and area under the curve. Among the considered ML models, the gradient-boosting decision tree, random forest, and artificial neural network showed better performances, achieving more than 85% accuracy in Summer, Fall, and Winter, and over 80% in Spring. The essence of the framework is valuable for developing strategies for building energy consumption estimation and higher-resolution occupancy level prediction, which are easily influenced by seasons.
Data Mining For Accurately Estimating Residential Natural Gas Energy Consumption And Savings Using A Random Forest Approach
DOWNLOAD
Author : Adel Ali Naji
language : en
Publisher:
Release Date : 2019
Data Mining For Accurately Estimating Residential Natural Gas Energy Consumption And Savings Using A Random Forest Approach written by Adel Ali Naji and has been published by this book supported file pdf, txt, epub, kindle and other format this book has been release on 2019 with Architecture and energy conservation categories.
Cost effective energy efficiency improvements in residential buildings could yield annual electricity savings of approximately 30 percent within this sector for the United States. Furthermore, such investment can create millions of direct and indirect jobs throughout the economy. Unfortunately, realizing these savings is difficult. One of the impediments for realization is the means by which savings can be estimated. The prevalent approach is to use energy models to estimate. However, actual energy savings are more often than not over-predicted by energy models, leading to wariness on the part of potential investors which include the residents themselves. A driver for this research is 500 residential buildings with known geometrical and historical energy data owned by the University of Dayton. Further, the energy characteristics of these buildings are knowable. This housing stock offers significant diversity in size (ranging from a floor area of 715 to 2800 square feet), age (from the early 1900s to new construction) and energy effectiveness, the latter occurring as a result of gradual improvements made to residences over the past 15 years. In the summer of 2015 energy and building data audits were conducted on a subset of 139 homes. The audit documented the areas of the walls and attic, the amount and type of insulation in the walls and attic, areas and types of windows, floor heights, maximum occupancy, appliance (refrigerator, range, oven) specifications, heating ventilation air-conditioning system specifications domestic hot water equipment specifications, interior attic penetration area, and the presence of a basement. A data mining approach was used for developing the Random Forest (RF) model to predict energy consumption in a group of single family houses based upon knowledge of residential energy characteristics, historical energy consumption, occupancy and building geometrical data, as well as inferred energy characteristics from energy consumption data. The model was used to estimate savings and develop a cost implementation model from discrete measures for each residence. Thus, the cost effectiveness of each possible measure could be assessed. From these, prioritized energy reduction measures among all possible measures for all residences could be identified based upon a "worst-to-first" strategy in order to achieve community-scale energy (and carbon) savings most cost effectively. The results when extrapolated 45,000 single family houses in Dayton, Ohio show that a preliminary investment in energy efficiency of $26 million can achieve annual energy cost savings of $2.21M per year. As or more importantly, an Economic Input-Output analysis reveals a total sequential economic impact of $41.2M from the investment. Thus, this approach offers significant and indisputable local impact.
Mining Hidden Knowledge From Measured Data For Improving Building Energy Performance
DOWNLOAD
Author : Zhun Yu
language : en
Publisher:
Release Date : 2012
Mining Hidden Knowledge From Measured Data For Improving Building Energy Performance written by Zhun Yu and has been published by this book supported file pdf, txt, epub, kindle and other format this book has been release on 2012 with categories.
A Framework For Benchmarking Energy Retrofit Systems Through Building Information Modeling Bim
DOWNLOAD
Author : Ahmad Hussein Elmani
language : en
Publisher:
Release Date : 2015
A Framework For Benchmarking Energy Retrofit Systems Through Building Information Modeling Bim written by Ahmad Hussein Elmani and has been published by this book supported file pdf, txt, epub, kindle and other format this book has been release on 2015 with Architecture and energy conservation categories.
"The rapid increase in worldwide energy consumption has raised a global concern about the future of energy use and its impact on the surrounding environment. Such impact is causing resource degradation, increased carbon emissions, depletion of the ozone layer, and global warming. As the building sector is considered one of the main energy-consuming sectors, the trend of sustainable designs, green projects, energy optimization and reduction policies are many countries’ recent focus. This focus is typically on new buildings, and it constantly overlooks the vast impact of existing buildings. Efficient energy retrofitting of existing buildings, therefore, is highly needed to mitigate the impact on the surrounding environment, due to the excess use of energy. This research presents a framework for benchmarking energy retrofit systems of office buildings in climate zone 1 (very hot humid regions), by integrating building information modeling (BIM) and laser scanning. The reason for considering existing office buildings is related to the great benefits of retrofitting and the ability to efficiently analyze the main energy consuming systems, which are office equipment, lighting, and heating ventilation and air conditioning (HVAC) systems. The proposed non-destructive methodology will assist in providing an estimate of the amount of possible energy use reduction, and the likelihood of achieving standard and sustainable conditions. The analysis of separate and overall energy consumptions for each of the systems will be investigated through a developed benchmarking tool. The tool will analyze each of the systems’ existing performance and benchmark this performance to an enhanced condition. The integration of laser scanning and BIM will provide an accurate measure of the building’s existing condition and will provide the required data input for analysis in the developed tool. In addition, the benchmarking tool analysis for an office building energy system in the UAE will illustrate the proposed procedure and analytical process. The results of the investigative framework show that the examined office floor can reduce its total energy consumption according to standard and sustainable conditions by almost 14% and 29%, respectively. Also, the energy end use breakdown was found to be 55% for the HVAC system, 23% for lighting, and 17% for office equipment."--Abstract.
Proceedings Of The 24th International Symposium On Advancement Of Construction Management And Real Estate
DOWNLOAD
Author : Gui Ye
language : en
Publisher: Springer Nature
Release Date : 2021-06-07
Proceedings Of The 24th International Symposium On Advancement Of Construction Management And Real Estate written by Gui Ye and has been published by Springer Nature this book supported file pdf, txt, epub, kindle and other format this book has been release on 2021-06-07 with Business & Economics categories.
This book covers various current and emerging topics in construction management and real estate. Papers selected in this book cover a wide variety of topics such as new-type urbanization, planning and construction of smart city and eco-city, urban–rural infrastructure development, land use and development, housing market and housing policy, new theory and practice of construction project management, big data application, smart construction and BIM, international construction (i.e., belt and road project), green building, off-site prefabrication, rural rejuvenation and eco-civilization and other topics related to construction management and real estate. These papers provide useful references to both scholars and practitioners. This book is the documentation of “The 24th International Symposium on Advancement of Construction Management and Real Estate,” which was held in Chongqing, China.
Transition To Sustainable Buildings
DOWNLOAD
Author : Organisation for Economic Co-operation and Development
language : en
Publisher: Organization for Economic Co-Operation & Development
Release Date : 2013
Transition To Sustainable Buildings written by Organisation for Economic Co-operation and Development and has been published by Organization for Economic Co-Operation & Development this book supported file pdf, txt, epub, kindle and other format this book has been release on 2013 with Architecture categories.
Buildings are the largest energy consuming sector in the world, and account for over one-third of total final energy consumption and an equally important source of carbon dioxide (CO2) emissions. Achieving significant energy and emissions reduction in the buildings sector is a challenging but achievable policy goal. Transition to Sustainable Buildings presents detailed scenarios and strategies to 2050, and demonstrates how to reach deep energy and emissions reduction through a combination of best available technologies and intelligent public policy. This IEA study is an indispensible guide for decision makers, providing informative insights on: cost-effective options, key technologies and opportunities in the buildings sector; solutions for reducing electricity demand growth and flattening peak demand; effective energy efficiency policies and lessons learned from different countries; future trends and priorities for ASEAN, Brazil, China, the European Union, India, Mexico, Russia, South Africa and the United States; implementing a systems approach using innovative products in a cost effective manner; and pursuing whole-building (e.g. zero energy buildings) and advanced-component policies to initiate a fundamental shift in the way energy is consumed.