Deep Learning Architecture To Improve Edge Accuracy Of Auto Contouring For Head And Neck Radiotherapy
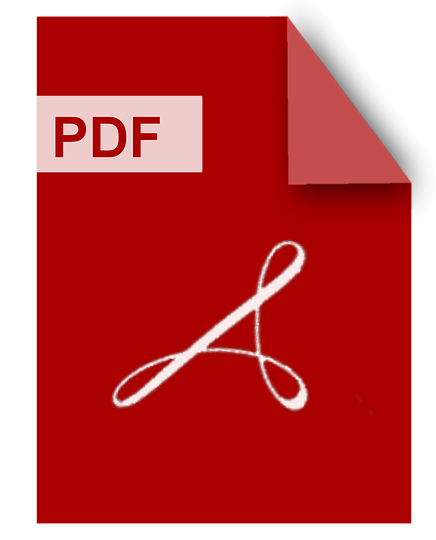
DOWNLOAD
Download Deep Learning Architecture To Improve Edge Accuracy Of Auto Contouring For Head And Neck Radiotherapy PDF/ePub or read online books in Mobi eBooks. Click Download or Read Online button to get Deep Learning Architecture To Improve Edge Accuracy Of Auto Contouring For Head And Neck Radiotherapy book now. This website allows unlimited access to, at the time of writing, more than 1.5 million titles, including hundreds of thousands of titles in various foreign languages. If the content not found or just blank you must refresh this page
Deep Learning Architecture To Improve Edge Accuracy Of Auto Contouring For Head And Neck Radiotherapy
DOWNLOAD
Author : Ryan C. Gifford
language : en
Publisher:
Release Date : 2022
Deep Learning Architecture To Improve Edge Accuracy Of Auto Contouring For Head And Neck Radiotherapy written by Ryan C. Gifford and has been published by this book supported file pdf, txt, epub, kindle and other format this book has been release on 2022 with Cancer categories.
The manual delineation of the gross tumor volume (GTV) for Head and Neck Cancer (HNC) patients is an essential step in the radiotherapy treatment process. Methods to automate this process have the potential to decrease the amount of time it takes for a clinician to complete a plan, while also decreasing the inter-observer variability between clinicians. Deep learning (DL) methods have shown great promise in auto-segmentation problems. For HNC, we show that DL methods systematically fail at the axial edges of GTV where the segmentation is dependent on both information from the center of the tumor and nearby slices. These failures may decrease trust and usage of proposed Auto-Contouring Systems if not accounted for. In this paper we propose a modified version of the U-Net, a fully convolutional network for image segmentation, which can more accurately process dependence between slices to create a more robust GTV contour. We also show that it can outperform the current proposed methods that capture slice dependencies by leveraging 3D convolutions. Our method uses Convolutional Recurrent Neural Networks throughout the decoder section of the U-Net to capture both spatial and adjacent-slice information when considering a contour. To account for shifts in anatomical structures through adjacent CT slices, we allow an affine transformation to the adjacent feature space using Spatial Transformer Networks. Our proposed model increases accuracy at the edges by 12% inferiorly and 26% superiorly over a baseline 2D U-Net, which has no inherent way to capture information between adjacent slices.
Auto Segmentation For Radiation Oncology
DOWNLOAD
Author : Jinzhong Yang
language : en
Publisher: CRC Press
Release Date : 2021-04-18
Auto Segmentation For Radiation Oncology written by Jinzhong Yang and has been published by CRC Press this book supported file pdf, txt, epub, kindle and other format this book has been release on 2021-04-18 with Science categories.
This book provides a comprehensive introduction to current state-of-the-art auto-segmentation approaches used in radiation oncology for auto-delineation of organs-of-risk for thoracic radiation treatment planning. Containing the latest, cutting edge technologies and treatments, it explores deep-learning methods, multi-atlas-based methods, and model-based methods that are currently being developed for clinical radiation oncology applications. Each chapter focuses on a specific aspect of algorithm choices and discusses the impact of the different algorithm modules to the algorithm performance as well as the implementation issues for clinical use (including data curation challenges and auto-contour evaluations). This book is an ideal guide for radiation oncology centers looking to learn more about potential auto-segmentation tools for their clinic in addition to medical physicists commissioning auto-segmentation for clinical use. Features: Up-to-date with the latest technologies in the field Edited by leading authorities in the area, with chapter contributions from subject area specialists All approaches presented in this book are validated using a standard benchmark dataset established by the Thoracic Auto-segmentation Challenge held as an event of the 2017 Annual Meeting of American Association of Physicists in Medicine
Deep Learning Based Organ At Risk Segmentation In Head And Neck Radiotherapy
DOWNLOAD
Author :
language : en
Publisher:
Release Date : 2023
Deep Learning Based Organ At Risk Segmentation In Head And Neck Radiotherapy written by and has been published by this book supported file pdf, txt, epub, kindle and other format this book has been release on 2023 with categories.
This doctoral thesis is the product of scientific research conducted from early 2018 to early 2021. It covers work investigating the potential of deep learning-based segmentation for organ-at-risk segmentation in head and neck radiotherapy. Deep learning-based segmentation of organs-at-risk in head and neck radiotherapy can be used to generate treatment plans that are as safe as treatment plans based on manual segmentation. However, in our study, deep learning-based segmentation did not yet perform well enough to be very specific during quality assurance of manual segmentation. There are several strategies that can be applied to improve the performance and reliability of deep learning-based segmentation, but there seems to be an upper limit on the similarity coefficient that can be achieved. This may be caused by the sub-optimal quality of the manual segmentations used to train deep learning models. Contrarily, sub-optimal quality of the imaging data can make the model more robust. The imperfect performance of deep learning-based segmentation may be one of the reasons that it is not yet standard practice in radiotherapy clinics around the world. Probability maps may be a way to increase user-confidence and facilitate adoption of these methods in the clinic.
Investigation Of Adaptive Radiation Therapy Including Deformable Image Registration Treatment Planning Modification Strategies Machine Learning Deep Learning
DOWNLOAD
Author : Pawel Siciarz
language : en
Publisher:
Release Date : 2021
Investigation Of Adaptive Radiation Therapy Including Deformable Image Registration Treatment Planning Modification Strategies Machine Learning Deep Learning written by Pawel Siciarz and has been published by this book supported file pdf, txt, epub, kindle and other format this book has been release on 2021 with categories.
The goal of this research was to propose and evaluate solutions to four important aspects of adaptive radiation therapy in order to make it more reliable, accurate, and efficient in clinical environment. The first study focused on the evaluation of several deformable image registration algorithms. Results demonstrated that the Dense Anatomical Block Matching registration outperformed the other methods making it a very promising alternative to the existing registration methods for challenging CT-to-CBCT registration and its applications for radiation dose calculation, dose mapping and contour propagation in adaptive radiation therapy (ART) of the pelvic region. The second study focused on the quantitative evaluation of eight proposed adaptive radiation therapy approaches for prostate cancer patients treated with hypofractionated VMAT. The ART strategies included online and offline methods. The comprehensive analysis showed that daily on-line adaptation approaches were the most impactful. The findings of this study provided applicable insights into the selection of the optimal ART strategy, improving the quality of the decision-making process based on the quantitatively evaluated dosimetric benefits. The third study aimed to utilize a deep learning network to automatically contour critical organs on the computed tomography (CT) scans of head and neck cancer patients. Proposed model achieved expert level accuracy and was able to segment 25 critical organs on unseen CT images in approximately 7 seconds per patient. High accuracy and short contouring time allow for the implementation of the model within a clinical ART workflow, which would lead to a significant decrease in the time required to create a new adapted treatment plan. The objective of the fourth study was to use artificial intelligence methods to build a decision making support system that would classify previously delivered plans of brain tumor patients into those that met treatment planning objectives and those for which objectives were not met due to the priority given to one or more organs-at-risk. Among evaluated machine learning algorithms, the Logistic Regression model achieved the highest accuracy and can be used by radiation oncologists to support their decision-making process in terms of treatment plan adaptations and plan approvals in a data-driven quality assurance program.
Machine Learning Based Adaptive Radiotherapy Treatments From Bench Top To Bedside
DOWNLOAD
Author : Jiahan Zhang
language : en
Publisher: Frontiers Media SA
Release Date : 2023-05-12
Machine Learning Based Adaptive Radiotherapy Treatments From Bench Top To Bedside written by Jiahan Zhang and has been published by Frontiers Media SA this book supported file pdf, txt, epub, kindle and other format this book has been release on 2023-05-12 with Medical categories.
Automated Segmentation Of Head And Neck Cancer Using Texture Analysis With Co Registered Pet Ct Images
DOWNLOAD
Author : Huan Yu
language : en
Publisher:
Release Date : 2010
Automated Segmentation Of Head And Neck Cancer Using Texture Analysis With Co Registered Pet Ct Images written by Huan Yu and has been published by this book supported file pdf, txt, epub, kindle and other format this book has been release on 2010 with categories.
Radiation therapy is often offered as the primary treatment for head and neck cancer(HNC). Accurate target delineation is essential for the success of radiation therapy. The current target definition technique - manual delineation using Computed Tomography(CT) - is subject to high observer variability. Functional imaging modalities such as 2-[18F]-fluoro-2-deoxy-D-glucose Positron Emission Tomography(FDG-PET) can greatly improve the visualization of tumor. FDG-PET co-registered with CT has shown potential to improve the accuracy of target localization and reduce observer variability. Unfortunately, due to the limitation of PET, the degree of improvement obtained by qualitative and simple quantitative (e.g. thresholding) use of FDG-PET is not ideal. However, both PET and CT images contain a wealth of texture information that could be used to improve the accuracy of target definition. This thesis has investigated using texture analysis techniques to automatically delineate radiation targets. Firstly, PET and CT texture features with high discrimination ability were identified and a texture analysis technique- a decision tree based K Nearest Neighbour(DTKNN) classifier - was developed. DTKNN could accurately classify head and neck tissue with an area under curve(AUC) of a Receiver Operator Characteristic(ROC) of 0.95. Subsequently, an automated target delineation technique - CO-registered Multi-modality Pattern Analysis Segmentation System(COMPASS) - was developed that can delineate tumor on a voxel-by-voxel basis. COMPASS was found to accurately delineate HNC with 84% sensitivity and 95% specificity on a voxel basis per patient. To accurately evaluate the utility of the COMPASS in radiation targeting, a validation method which can combine biased observers' contours to generate a probabilistic reference for validation was developed. The method was based on maximum likelihood analysis using a simulated annealing(SA) algorithm. The results from this thesis show that texture features of both PET and CT images can enhance the discrimination between HNC and normal tissue, and an automated delineation method of HNC using texture analysis of PET and CT images can accurately and consistently define radiation targets in head and neck. This suggests that automated segmentation of radiation targets based on texture analysis techniques may significantly reduce observer variability and improve the accuracy of radiation targeting.
Machine Learning In Radiation Oncology
DOWNLOAD
Author : Issam El Naqa
language : en
Publisher: Springer
Release Date : 2015-06-19
Machine Learning In Radiation Oncology written by Issam El Naqa and has been published by Springer this book supported file pdf, txt, epub, kindle and other format this book has been release on 2015-06-19 with Medical categories.
This book provides a complete overview of the role of machine learning in radiation oncology and medical physics, covering basic theory, methods, and a variety of applications in medical physics and radiotherapy. An introductory section explains machine learning, reviews supervised and unsupervised learning methods, discusses performance evaluation, and summarizes potential applications in radiation oncology. Detailed individual sections are then devoted to the use of machine learning in quality assurance; computer-aided detection, including treatment planning and contouring; image-guided radiotherapy; respiratory motion management; and treatment response modeling and outcome prediction. The book will be invaluable for students and residents in medical physics and radiation oncology and will also appeal to more experienced practitioners and researchers and members of applied machine learning communities.
Deep Learning In Medical Image Analysis
DOWNLOAD
Author : Gobert Lee
language : en
Publisher: Springer Nature
Release Date : 2020-02-06
Deep Learning In Medical Image Analysis written by Gobert Lee and has been published by Springer Nature this book supported file pdf, txt, epub, kindle and other format this book has been release on 2020-02-06 with Medical categories.
This book presents cutting-edge research and applications of deep learning in a broad range of medical imaging scenarios, such as computer-aided diagnosis, image segmentation, tissue recognition and classification, and other areas of medical and healthcare problems. Each of its chapters covers a topic in depth, ranging from medical image synthesis and techniques for muskuloskeletal analysis to diagnostic tools for breast lesions on digital mammograms and glaucoma on retinal fundus images. It also provides an overview of deep learning in medical image analysis and highlights issues and challenges encountered by researchers and clinicians, surveying and discussing practical approaches in general and in the context of specific problems. Academics, clinical and industry researchers, as well as young researchers and graduate students in medical imaging, computer-aided-diagnosis, biomedical engineering and computer vision will find this book a great reference and very useful learning resource.
Application Of Deep Learning Intelligence In Segmentation Of Neck Tumor And Accuracy Evaluation
DOWNLOAD
Author : 許文樂
language : en
Publisher:
Release Date : 2020
Application Of Deep Learning Intelligence In Segmentation Of Neck Tumor And Accuracy Evaluation written by 許文樂 and has been published by this book supported file pdf, txt, epub, kindle and other format this book has been release on 2020 with categories.
Contouring In Head And Neck Cancer
DOWNLOAD
Author : Peter C. Levendag
language : en
Publisher: Elsevier,Urban&FischerVerlag
Release Date : 2009
Contouring In Head And Neck Cancer written by Peter C. Levendag and has been published by Elsevier,Urban&FischerVerlag this book supported file pdf, txt, epub, kindle and other format this book has been release on 2009 with categories.