Deep Learning For Alternative Splicing
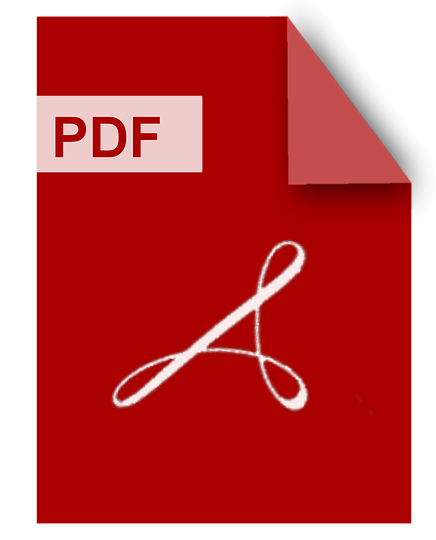
DOWNLOAD
Download Deep Learning For Alternative Splicing PDF/ePub or read online books in Mobi eBooks. Click Download or Read Online button to get Deep Learning For Alternative Splicing book now. This website allows unlimited access to, at the time of writing, more than 1.5 million titles, including hundreds of thousands of titles in various foreign languages. If the content not found or just blank you must refresh this page
Deep Learning For Alternative Splicing
DOWNLOAD
Author : Richard Brown
language : en
Publisher:
Release Date : 2019
Deep Learning For Alternative Splicing written by Richard Brown and has been published by this book supported file pdf, txt, epub, kindle and other format this book has been release on 2019 with categories.
Machine Learning Strategies For Alternative Splicing
DOWNLOAD
Author : Zhicheng Pan
language : en
Publisher:
Release Date : 2021
Machine Learning Strategies For Alternative Splicing written by Zhicheng Pan and has been published by this book supported file pdf, txt, epub, kindle and other format this book has been release on 2021 with categories.
Alternative splicing (AS) is a fundamental biological process that diversifies the transcriptomes and proteomes. Aberrant splicing is the main cause of rare diseases and cancers. Our understanding of AS is far from complete, resulting in a limited comprehension of phenotypic effects of splicing dysregulation. Recent advances in next-generation sequencing (NGS) technologies have revolutionized the discoveries of AS. There are considerable efforts put into generating a large compendium of RNA-seq datasets. These datasets offer an opportunity to study the regulation of AS in tissues, cell stages, and perturbation of biological conditions at unprecedented resolutions and scales. However, utilizing the large number of datasets to make biological discoveries remains a challenge. In this dissertation, we developed machine-learning-based strategies to integrate various types of RNA-seq datasets and transform them into biological knowledge, thereby enabling discoveries towards regulatory mechanisms and functional consequences of AS. In the first part of the dissertation, we report a deep-learning-based computational framework, Deep-learning Augmented RNA-seq analysis of Transcript Splicing (DARTS), that utilizes the Bayesian integration of deep-learning-based predictions with empirical RNA datasets to make inference of differential alternative splicing between biological samples. RNA sequencing (RNA-seq) analysis of alternative splicing is largely limited by depending on high sequencing coverage. DARTS transforms large amounts of publicly available RNA-seq datasets into biological knowledge of how splicing is regulated through deep learning, thus enabling researchers to better characterize alternative splicing inaccessible from RNA-seq datasets with modest coverage. In the second part of the dissertation, we present a computational tool, Systematic Investigation of Retained Introns (SIRI), to quantify unspliced introns and describe a deep-learning-based computational framework to investigate the sequence preferences of different intron groups across subcellular locations. Steps of mRNA maturation occur in distinct cellular locations, while subcellular distribution of processed and unprocessed transcripts often miss in transcriptomic analyses. We employed SIRI to measure intron levels in subcellular locations across cell development and identified four intron groups that have disparate patterns of RNA enrichment across subcellular locations. Through the deep-learning based framework, we identified a set of triplet motifs and sequence conservation patterns that are predictive of intron behavior among biological conditions. In the third part of the dissertation, we exhibit a deep-learning-based tissue-specific framework, individualized Deep-learning Analysis of RNA Transcript Splicing (iDARTS), for predicting splicing levels. The rapid accumulation of RNA-seq datasets matched with whole exome or genome sequencing yields enormous variants underlying diseases, traits, and cancer. Interpreting the functional consequences of these variants remains a challenge in disease diagnostics and precision medicine. iDARTS leverages the publicly available RNA-seq datasets to model the cis RNA sequence features and trans RNA binding protein levels determinants of AS, allowing precise predictions of genetic splice-altering variants. We demonstrated that predicted splice-altering variants are functionally relevant and related to cancer development when analysing ~10 million intronic and exonic variants with iDARTS. Applying iDARTS to interpret functional consequences of variants of uncertain significance in clinical studies, we found that predicted splice-altering variants are ten times enriched in pathogenic categories over benign categories. Our results indicate that iDARTS will benefit large-scale screening disease-implicated variants, thus improving disease diagnosis and enabling discoveries for precision medicine. In the fourth part of the dissertation, we study the underlying mechanisms of N6-methyladenosine (m6A) RNA modification by investigating the biological consequences of arginine methylation of METTL14 through transcriptome-wide profiling of m6A. Arginine methylation of METTL14 controls m6A deposition in mammalian cells. Mouse embryonic stem cells (mESCs) expressing arginine methylation-deficient METTL14 exhibit significantly reduced global m6A levels. These arginine methylation-dependent m6A sites identified from transcriptome-wide analysis are associated with enhanced translation of genes essential for the repair of DNA interstrand crosslinks. Collectively, these findings reveal important aspects of m6A regulation and new functions of arginine methylation in RNA metabolism.
Alternative Splice Site Prediction With Deep Learning
DOWNLOAD
Author : Hannes Bretschneider
language : en
Publisher:
Release Date : 2019
Alternative Splice Site Prediction With Deep Learning written by Hannes Bretschneider and has been published by this book supported file pdf, txt, epub, kindle and other format this book has been release on 2019 with categories.
Alternative splicing of mRNA is tightly regulated in different tissues and developmental stages and its disruption is one of the leading mechanisms that cause genetic disease in humans. Gene splicing depends on the precise interaction of hundreds of cis-motifs that control the action of trans-acting splicing factors. These regulatory factors have been investigated in great detail, but it remains challenging to predict the splicing pattern of a transcript from its sequence, which led to the development of computational "splicing codes" using machine learning methods. I present multiple models that learn regulatory sequence features ab initio using convolutional neural networks instead of requiring a hand-engineered feature library. The first model is trained to distinguish true splice sites from decoys and improves on the performance of a previous support vector machine model. Another model is trained to predict the splicing pattern of pairs of alternative 3' splice sites, achieving 95% auROC on this task. When this model was applied to the DBASS3 database of genomic variants that activate cryptic splice sites, it was able to identify 63% of cryptic splice sites correctly. Next, I developed the competitive splice site model (COSSMO), which, for the first time, can predict complex, non-binary splicing patterns. COSSMO can analyze a variable number of alternative acceptor or donor splice sites, uses convolutional and recurrent neural networks to learn from directly from sequence, and was trained on over 15M sites. COSSMO achieves 77% accuracy on the task of identifying the dominant splice sites from, on average, 90 candidate sites and matches the true splicing pattern with an R2 of 71.3%. On a dataset of deep intronic variants, COSSMO can identify cryptic splice sites that are located hundreds of kilobases within the intron. An ensemble model of MaxEntScan and COSSMO achieves an auROC of 92.6% at discriminating between cryptic splice site-activating variants and common variants nearby. Applying COSSMO to the HGMD dataset of disease-causing variants showed that thousands of missense or nonsense variants also disrupt splicing and that intronic variants up to a distance of 1000 nt affect splicing.
Alternative Splicing Site Prediction Using Machine Learning Methods
DOWNLOAD
Author : Yu Lu
language : en
Publisher:
Release Date : 2007
Alternative Splicing Site Prediction Using Machine Learning Methods written by Yu Lu and has been published by this book supported file pdf, txt, epub, kindle and other format this book has been release on 2007 with Genetic engineering categories.
Bioinformatics Analyses Of Alternative Splicing Est Based And Machine Learning Based Prediction
DOWNLOAD
Author : Jing Xia
language : en
Publisher:
Release Date : 2008
Bioinformatics Analyses Of Alternative Splicing Est Based And Machine Learning Based Prediction written by Jing Xia and has been published by this book supported file pdf, txt, epub, kindle and other format this book has been release on 2008 with categories.
Alternative splicing is a mechanism for generating different gene transcripts (called iso- forms) from the same genomic sequence. Finding alternative splicing events experimentally is both expensive and time consuming. Computational methods in general, and EST analy- sis and machine learning algorithms in particular, can be used to complement experimental methods in the process of identifying alternative splicing events. In this thesis, I first iden- tify alternative splicing exons by analyzing EST-genome alignment. Next, I explore the predictive power of a rich set of features that have been experimentally shown to affect al- ternative splicing. I use these features to build support vector machine (SVM) classifiers for distinguishing between alternatively spliced exons and constitutive exons. My results show that simple, linear SVM classifiers built from a rich set of features give results comparable to those of more sophisticated SVM classifiers that use more basic sequence features. Finally, I use feature selection methods to identify computationally the most informative features for the prediction problem considered.
Machine Learning In Computational Biology
DOWNLOAD
Author : Ofer Shai
language : en
Publisher:
Release Date : 2009
Machine Learning In Computational Biology written by Ofer Shai and has been published by this book supported file pdf, txt, epub, kindle and other format this book has been release on 2009 with categories.
Alternative splicing, the process by which a single gene may code for similar but different proteins, is an important process in biology, linked to development, cellular differentiation, genetic diseases, and more. Genome-wide analysis of alternative splicing patterns and regulation has been recently made possible due to new high throughput techniques for monitoring gene expression and genomic sequencing. This thesis introduces two algorithms for alternative splicing analysis based on large microarray and genomic sequence data. The algorithms, based on generative probabilistic models that capture structure and patterns in the data, are used to study global properties of alternative splicing. GenASAP, the first method to provide quantitative predictions of alternative splicing patterns on large scale data sets, is shown to generate useful and precise predictions based on independent RT-PCR validation (a slow but more accurate approach to measuring cellular expression patterns). In the second part of the thesis, the results obtained by GenASAP are analysed to reveal jointly regulated genes. The sequences of the genes are examined for potential regulatory factors binding sites using a new motif finding algorithm designed for this purpose. The motif finding algorithm, called GenBITES (generative model for binding sites) uses a fully Bayesian generative model for sequences, and the MCMC approach used for inference in the model includes moves that can efficiently create or delete motifs, and extend or contract the width of existing motifs. GenBITES has been applied to several synthetic and real data sets, and is shown to be highly competitive at a task for which many algorithms already exist. Although developed to analyze alternative splicing data, GenBITES outperforms most reported results on a benchmark data set based on transcription data. In the first part of the thesis, a microarray platform for monitoring alternative splicing is introduced. A spatial noise removal algorithm that removes artifacts and improves data fidelity is presented. The GenASAP algorithm (generative model for alternative splicing array platform) models the non-linear process in which targeted molecules bind to a microarray's probes and is used to predict patterns of alternative splicing. Two versions of GenASAP have been developed. The first uses variational approximation to infer the relative amounts of the targeted molecules, while the second incorporates a more accurate noise and generative model and utilizes Markov chain Monte Carlo (MCMC) sampling.
Inference Of Computational Models Of Alternative Polyadenylation And Splicing
DOWNLOAD
Author : Michael Ka Kit Leung
language : en
Publisher:
Release Date : 2018
Inference Of Computational Models Of Alternative Polyadenylation And Splicing written by Michael Ka Kit Leung and has been published by this book supported file pdf, txt, epub, kindle and other format this book has been release on 2018 with categories.
Instructions from the genome are first copied to make messenger RNAs, which are then translated to make proteins. To expand the repertoire of these instructions, cells can modify the messenger RNAs in different ways. Two such modifications are alternative polyadenylation and alternative splicing. Using RNA-Seq data and deep learning, we trained computational models that can be applied to sequences in the genome to predict tissue-specific polyadenylation and splicing patterns. Presented with multiple alternative polyadenylation sites, the polyadenylation model can predict the probability each site would be selected for cleavage and polyadenylation. Similarly, given alternative exons, the splicing model can predict which exon would more likely be included. The performance of these models in predicting polyadenylation and splicing patterns for genomic regions not observed during training is evaluated, and an analysis of what the models have learned reveals sequence elements that are known to influence these cellular processes. Importantly, these computational models are trained on genome-wide patterns based on the reference genome but can generalize to individual variations. Each model can thus be viewed as a simulator, where the genotype of an individual can be fed in as an input, and the output describes how the individual's mutations affect the mechanisms of polyadenylation and splicing in different tissue types. The relevance of these models for problems in genomic medicine is described, and proof-of-concept applications are demonstrated.
Semi Supervised And Transductive Learning Algorithms For Predicting Alternative Splicing Events In Genes
DOWNLOAD
Author : Karthik Tangirala
language : en
Publisher:
Release Date : 2011
Semi Supervised And Transductive Learning Algorithms For Predicting Alternative Splicing Events In Genes written by Karthik Tangirala and has been published by this book supported file pdf, txt, epub, kindle and other format this book has been release on 2011 with categories.
As genomes are sequenced, a major challenge is their annotation -- the identification of genes and regulatory elements, their locations and their functions. For years, it was believed that one gene corresponds to one protein, but the discovery of alternative splicing provided a mechanism for generating different gene transcripts (isoforms) from the same genomic sequence. In the recent years, it has become obvious that a large fraction of genes undergoes alternative splicing. Thus, understanding alternative splicing is a problem of great interest to biologists. Supervised machine learning approaches can be used to predict alternative splicing events at genome level. However, supervised approaches require large amounts of labeled data to produce accurate classifiers. While large amounts of genomic data are produced by the new sequencing technologies, labeling these data can be costly and time consuming. Therefore, semi-supervised learning approaches that can make use of large amounts of unlabeled data, in addition to small amounts of labeled data are highly desirable. In this work, we study the usefulness of a semi-supervised learning approach, co-training, for classifying exons as alternatively spliced or constitutive. The co-training algorithm makes use of two views of the data to iteratively learn two classifiers that can inform each other, at each step, with their best predictions on the unlabeled data. We consider three sets of features for constructing views for the problem of predicting alternatively spliced exons: lengths of the exon of interest and its flanking introns, exonic splicing enhancers (a.k.a., ESE motifs) and intronic regulatory sequences (a.k.a., IRS motifs). Naive Bayes and Support Vector Machine (SVM) algorithms are used as based classifiers in our study. Experimental results show that the usage of the unlabeled data can result in better classifiers as compared to those obtained from the small amount of labeled data alone. In addition to semi-supervised approaches, we also also study the usefulness of graph based transductive learning approaches for predicting alternatively spliced exons. Similar to the semi-supervised learning algorithms, transductive learning algorithms can make use of unlabeled data, together with labeled data, to produce labels for the unlabeled data. However, a classification model that could be used to classify new unlabeled data is not learned in this case. Experimental results show that graph based transductive approaches can make effective use of the unlabeled data.
Bioinformatics Analyses Of Alternative Splicing
DOWNLOAD
Author : Rileen Sinha
language : en
Publisher:
Release Date : 2009
Bioinformatics Analyses Of Alternative Splicing written by Rileen Sinha and has been published by this book supported file pdf, txt, epub, kindle and other format this book has been release on 2009 with categories.
Inference And Analysis Of The Human Splicing Code
DOWNLOAD
Author : Hui Yuan Xiong
language : en
Publisher:
Release Date : 2016
Inference And Analysis Of The Human Splicing Code written by Hui Yuan Xiong and has been published by this book supported file pdf, txt, epub, kindle and other format this book has been release on 2016 with categories.
We construct and analyse a computational model that predicts the outcome of alternative splicing by recognizing features in RNA sequences. The computational model can be viewed as a ``splicing simulator'' for a range of healthy human tissues. It takes as input a pre-mRNA sequence surrounding a possibly alternatively spliced exon and estimates the inclusion level of that exon in mature RNA, after splicing occurs. The model is trained using a supervised machine learning framework where the training examples are the alternatively spliced exons, the feature vectors are derived RNA sequences near these exons, and the targets are their corresponding splicing outcomes in healthy individuals. The model is inferred from over 15 million DNA elements derived from the human reference genome and encoded as 1300 numerical RNA features, 10689 alternative exons mined from RefSeq and EST databases and RNA-Seq data from 16 healthy human tissues. A Bayesian ensemble of neural networks capable of accounting for combinatorial effects of RNA features is used to learn the relationship between the RNA features and the splicing outcomes. By identifying combinations of functionally important DNA elements, the model accounts for 65\% of the variance in the inclusion level of out-of-sample test exons. By learning genome-wide patterns that relate RNA sequences to splicing on the reference genome, we found the model is capable of generalizing to new genetic contexts and predicting splicing outcome for novel sequences. We applied the model to analyze the effects of more than 650,000 intronic and exonic variants on splicing. We observed that disease-associated mutations disrupt splicing much more often than common mutations, revealing previously unknown potential diseases mechanisms. Surprisingly, these splicing-disrupting mutations are not limited to mutations at splice sites. Many deep intronic mutations are also predicted to disrupt splicing. In focused studies on mutations related to spinal muscular atrophy and Lynch syndrome, we found our computational predictions have good agreement with previously identified effects of splicing-disrupting mutations that were found in independent biological experiments. In a focused study on autism spectrum disorder, we found that mutations with large effects on splicing are significantly more concentrated in brain related genes in autism patients compared to control subjects. This thesis is a step towards using artificial intelligence and large amounts of genomic data to automatically model the complex cellular mechanisms that read and process DNA. In our opinion, computational models constructed using this approach will bring significant value to genomic medicine, because they can model biological mechanisms and can be used for a wide range of sequences. As a result, the cellular effects of mutations can be predicted even if the mutation has not been observed before. This ability can be used for genetic diagnostics, studying the effects of complex diseases, and searching for treatments. In addition, it is anticipated that these computational models will improve with the growing size of genomic data data available for training.