Deep Learning For Tomographic Reconstruction
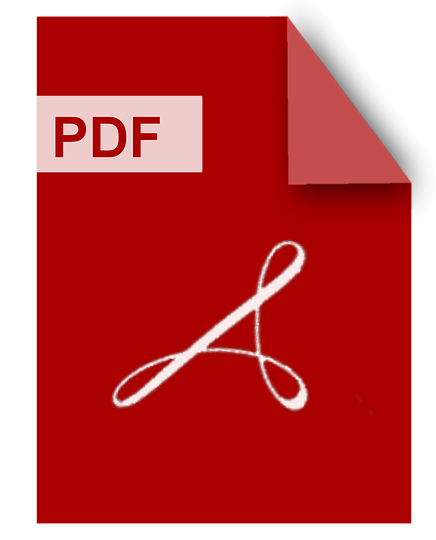
DOWNLOAD
Download Deep Learning For Tomographic Reconstruction PDF/ePub or read online books in Mobi eBooks. Click Download or Read Online button to get Deep Learning For Tomographic Reconstruction book now. This website allows unlimited access to, at the time of writing, more than 1.5 million titles, including hundreds of thousands of titles in various foreign languages. If the content not found or just blank you must refresh this page
Machine Learning For Tomographic Imaging
DOWNLOAD
Author : Ge Wang
language : en
Publisher: Programme: Iop Expanding Physi
Release Date : 2019-12-30
Machine Learning For Tomographic Imaging written by Ge Wang and has been published by Programme: Iop Expanding Physi this book supported file pdf, txt, epub, kindle and other format this book has been release on 2019-12-30 with Technology & Engineering categories.
Machine learning represents a paradigm shift in tomographic imaging, and image reconstruction is a new frontier of machine learning. This book will meet the needs of those who want to catch the wave of smart imaging. The book targets graduate students and researchers in the imaging community. Open network software, working datasets, and multimedia will be included. The first of its kind in the emerging field of deep reconstruction and deep imaging, Machine Learning for Tomographic Imaging presents the most essential elements, latest progresses and an in-depth perspective on this important topic.
Deep Learning For Tomographic Reconstruction
DOWNLOAD
Author : Théo Leuliet
language : en
Publisher:
Release Date : 2022
Deep Learning For Tomographic Reconstruction written by Théo Leuliet and has been published by this book supported file pdf, txt, epub, kindle and other format this book has been release on 2022 with categories.
The purpose of tomography is to reconstruct a volume from its projections. In Computed Tomography (CT), X-rays are transmitted to a patient and attenuated by their tissues: the projections are obtained from the measured attenuation. For Positron Emission Tomography (PET), a radionuclide injected inside a patient emits a positron that generates two gamma photons in opposite directions. The projections correspond to the set of lines of response between each pair of simultaneously detected photons. Tomographic reconstruction for PET or CT amounts to solving an inverse problem. Analytical methods are fast but their efficiency is limited when data are under-sampled or noisy. Iterative methods are efficient for noise and artefacts removal, but the computation time represents a major drawback for practical use. Deep learning based methods have the potential to overcome those limits. The first objective of this thesis is to study the impact of the training loss on medical diagnosis-oriented evaluation metrics. We perform this study on bone microarchitecture CT imaging and show that in this case L1 loss should be used regarding all the considered metrics. Networks trained with perceptual losses show better transcription of structural features, at the cost of a deteriorated resolution. Adversarial losses improve the accuracy of the reconstruction in terms of density distribution. We then focus on Time of Flight (TOF) PET data for intraoperative surgical applications; our aim is to design a reconstruction method to improve the detectability of small tumors in the context of breast cancer. We propose a neural network called PAVENET that simultaneously retrieves the image and the image-dependent point-spread function (PSF) from a poor-quality initial reconstruction. We present in this thesis the proof of concept for PAVENET with experiments on Monte-Carlo simulations reproducing acquisitions from an innovative detector studied in the Radiation Physics Instrumentation Laboratory (RPIL) in Boston.
Deep Learning For Tomographic Reconstruction With Limited Data
DOWNLOAD
Author :
language : en
Publisher:
Release Date : 2022
Deep Learning For Tomographic Reconstruction With Limited Data written by and has been published by this book supported file pdf, txt, epub, kindle and other format this book has been release on 2022 with categories.
Deep Learning For Biomedical Image Reconstruction
DOWNLOAD
Author : Jong Chul Ye
language : en
Publisher: Cambridge University Press
Release Date : 2023-09-30
Deep Learning For Biomedical Image Reconstruction written by Jong Chul Ye and has been published by Cambridge University Press this book supported file pdf, txt, epub, kindle and other format this book has been release on 2023-09-30 with Technology & Engineering categories.
Discover the power of deep neural networks for image reconstruction with this state-of-the-art review of modern theories and applications. The background theory of deep learning is introduced step-by-step, and by incorporating modeling fundamentals this book explains how to implement deep learning in a variety of modalities, including X-ray, CT, MRI and others. Real-world examples demonstrate an interdisciplinary approach to medical image reconstruction processes, featuring numerous imaging applications. Recent clinical studies and innovative research activity in generative models and mathematical theory will inspire the reader towards new frontiers. This book is ideal for graduate students in Electrical or Biomedical Engineering or Medical Physics.
Deep Learning In Medical Image Analysis
DOWNLOAD
Author : R. Indrakumari
language : en
Publisher: CRC Press
Release Date : 2024-07-10
Deep Learning In Medical Image Analysis written by R. Indrakumari and has been published by CRC Press this book supported file pdf, txt, epub, kindle and other format this book has been release on 2024-07-10 with Computers categories.
This book is designed as a reference text and provides a comprehensive overview of conceptual and practical knowledge about deep learning in medical image processing techniques. The post-pandemic situation teaches us the importance of doctors, medical analysis, and diagnosis of diseases in a rapid manner. This book provides a snapshot of the state of current research between deep learning, medical image processing, and health care with special emphasis on saving human life. The chapters cover a range of advanced technologies related to patient health monitoring, predicting diseases from genomic data, detecting artefactual events in vital signs monitoring data, and managing chronic diseases. This book Delivers an ideal introduction to image processing in medicine, emphasizing the clinical relevance and special requirements of the field Presents key principles by implementing algorithms from scratch and using simple MATLAB®/Octave scripts with image data Provides an overview of the physics of medical image processing alongside discussing image formats and data storage, intensity transforms, filtering of images and applications of the Fourier transform, three-dimensional spatial transforms, volume rendering, image registration, and tomographic reconstruction Highlights the new potential applications of machine learning techniques to the solution of important problems in biomedical image applications This book is for students, scholars, and professionals of biomedical technology and healthcare data analytics.
Machine Learning For Medical Image Reconstruction
DOWNLOAD
Author : Florian Knoll
language : en
Publisher: Springer
Release Date : 2018-09-11
Machine Learning For Medical Image Reconstruction written by Florian Knoll and has been published by Springer this book supported file pdf, txt, epub, kindle and other format this book has been release on 2018-09-11 with Computers categories.
This book constitutes the refereed proceedings of the First International Workshop on Machine Learning for Medical Reconstruction, MLMIR 2018, held in conjunction with MICCAI 2018, in Granada, Spain, in September 2018. The 17 full papers presented were carefully reviewed and selected from 21 submissions. The papers are organized in the following topical sections: deep learning for magnetic resonance imaging; deep learning for computed tomography, and deep learning for general image reconstruction.
Machine Learning For Medical Image Reconstruction
DOWNLOAD
Author : Florian Knoll
language : en
Publisher: Springer Nature
Release Date : 2019-10-24
Machine Learning For Medical Image Reconstruction written by Florian Knoll and has been published by Springer Nature this book supported file pdf, txt, epub, kindle and other format this book has been release on 2019-10-24 with Computers categories.
This book constitutes the refereed proceedings of the Second International Workshop on Machine Learning for Medical Reconstruction, MLMIR 2019, held in conjunction with MICCAI 2019, in Shenzhen, China, in October 2019. The 24 full papers presented were carefully reviewed and selected from 32 submissions. The papers are organized in the following topical sections: deep learning for magnetic resonance imaging; deep learning for computed tomography; and deep learning for general image reconstruction.
Deep Learning Based Ct Image Reconstruction
DOWNLOAD
Author : Ruiwen Xing
language : en
Publisher:
Release Date : 2020
Deep Learning Based Ct Image Reconstruction written by Ruiwen Xing and has been published by this book supported file pdf, txt, epub, kindle and other format this book has been release on 2020 with Image reconstruction categories.
As a common medical imaging method, Computed Tomography (CT) can create tomographic images using X-ray data acquired from around the human body. However, high quality and adequately sampled X-ray measurement data are not always available. In this scenario, the tomographic image created by conventional reconstruction algorithms will be noisy, or contain artifacts. The goal of our study is to reconstruct high-quality tomographic images from noisy or incomplete scan data, including low-dose, sparse-view, and limited-angle scenarios, by utilizing novel deep learning techniques. In this project, we trained a Generative Adversarial Network (GAN) and used it as a signal prior in the Simultaneous Algebraic Reconstruction Technique (SART) iterative reconstruction algorithm. The GAN we trained includes a self-attention block to model long-range dependencies in the scan data. Compared with the state-of-the-art denoising cycle GAN, CIRCLE GAN, and a conventional mathematical reconstruction algorithm incorporating total variation minimization, our Self-Attention GAN for CT image reconstruction produces competitive results on solving limited-angle data reconstruction problems. On sparse view and low-dose scenarios, our method is not always the best among compared methods, but produces competitive results in some situations.
Deep Learning For Image Reconstruction
DOWNLOAD
Author : Markus Haltmeier
language : en
Publisher:
Release Date : 2020-01-14
Deep Learning For Image Reconstruction written by Markus Haltmeier and has been published by this book supported file pdf, txt, epub, kindle and other format this book has been release on 2020-01-14 with categories.
Many problems in science, engineering and medicine follow an inverse approach to problem by observations the output data to calculate or predict the inputs should be to generated the responses: for example, calculating an image in X-ray computed tomography, source reconstruction in acoustics, or calculating the density of the Earth from measurements of its gravity field. Recent advance in deep learning-based algorithms has emerged as a novel paradigm for image processing.This book compile the state-of-the-art approaches for solving inverse problems by deep learning; from basic concetps to deep learning. algorithms in image processing. It serves as an introduction to researchers working in image processing, and pattern recognition, as well as, students undertaken research in signal processing and AI.The book will include the following:
Medical Image Reconstruction
DOWNLOAD
Author : Gengsheng Lawrence Zeng
language : en
Publisher: Walter de Gruyter GmbH & Co KG
Release Date : 2023-07-04
Medical Image Reconstruction written by Gengsheng Lawrence Zeng and has been published by Walter de Gruyter GmbH & Co KG this book supported file pdf, txt, epub, kindle and other format this book has been release on 2023-07-04 with Science categories.
This textbook introduces the essential concepts of tomography in the field of medical imaging. The medical imaging modalities include x-ray CT (computed tomography), PET (positron emission tomography), SPECT (single photon emission tomography) and MRI. In these modalities, the measurements are not in the image domain and the conversion from the measurements to the images is referred to as the image reconstruction. The work covers various image reconstruction methods, ranging from the classic analytical inversion methods to the optimization-based iterative image reconstruction methods. As machine learning methods have lately exhibited astonishing potentials in various areas including medical imaging the author devotes one chapter to applications of machine learning in image reconstruction. Based on college level in mathematics, physics, and engineering the textbook supports students in understanding the concepts. It is an essential reference for graduate students and engineers with electrical engineering and biomedical background due to its didactical structure and the balanced combination of methodologies and applications,