Deep Learning Models And Its Application An Overview With The Help Of R Software Second In Series Machine Learning
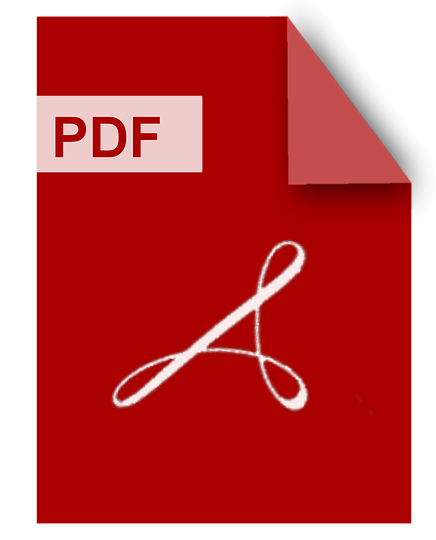
DOWNLOAD
Download Deep Learning Models And Its Application An Overview With The Help Of R Software Second In Series Machine Learning PDF/ePub or read online books in Mobi eBooks. Click Download or Read Online button to get Deep Learning Models And Its Application An Overview With The Help Of R Software Second In Series Machine Learning book now. This website allows unlimited access to, at the time of writing, more than 1.5 million titles, including hundreds of thousands of titles in various foreign languages. If the content not found or just blank you must refresh this page
Deep Learning Models And Its Application An Overview With The Help Of R Software Second In Series Machine Learning
DOWNLOAD
Author : Editor IJSMI
language : en
Publisher: International Journal of Statistics and Medical Informatics
Release Date : 2019-02-09
Deep Learning Models And Its Application An Overview With The Help Of R Software Second In Series Machine Learning written by Editor IJSMI and has been published by International Journal of Statistics and Medical Informatics this book supported file pdf, txt, epub, kindle and other format this book has been release on 2019-02-09 with Computers categories.
Deep Learning Models and its application: An overview with the help of R softwarePrefaceDeep learning models are widely used in different fields due to its capability to handle large and complex datasets and produce the desired results with more accuracy at a greater speed. In Deep learning models, features are selected automatically through the iterative process wherein the model learns the features by going deep into the dataset and selects the features to be modeled. In the traditional models the features of the dataset needs to be specified in advance. The Deep Learning algorithms are derived from Artificial Neural Network concepts and it is a part of broader Machine Learning Models. This book intends to provide an overview of Deep Learning models, its application in the areas of image recognition & classification, sentiment analysis, natural language processing, stock market prediction using R statistical software package, an open source software package. The book also includes an introduction to python software package which is also open source software for the benefit of the users.This books is a second book in series after the author’s first book- Machine Learning: An Overview with the Help of R Software https://www.amazon.com/dp/B07KQSN447EditorInternational Journal of Statistics and Medical Informaticswww.ijsmi.com/book.php
Deep Learning Models Explored With Help Of Python Programming
DOWNLOAD
Author : Editor IJSMI
language : en
Publisher: International Journal of Statistics and Medical Informatics
Release Date : 2020-11-04
Deep Learning Models Explored With Help Of Python Programming written by Editor IJSMI and has been published by International Journal of Statistics and Medical Informatics this book supported file pdf, txt, epub, kindle and other format this book has been release on 2020-11-04 with Young Adult Nonfiction categories.
This is the second book in the Deep Learning models series by the author. Deep learning models are widely used in different fields due to its capability to handle large and complex datasets and produce the desired results with more accuracy at a greater speed. In Deep learning models, features are selected automatically through the iterative process wherein the model learns the features by going deep into the dataset and selects the features to be modeled. In the traditional models the features of the dataset needs to be specified in advance. The Deep Learning algorithms are derived from Artificial Neural Network concepts and it is a part of broader Machine Learning Models. The book starts with the Introduction part which is adopted from Author’s Deep Learning Models and its application: An overview with the help of R software book and move on to the Python’s important data processing packages such Numpy, and Pandas. Book then explores the Deep Learning models with the help of packages such as Pytorch, Tensor Flow and Keras and their applications in image processing, stock market prediction, recommender systems and natural language processing. Editor International Journal of Statistics and Medical Informatics www.ijsmi.com/book.php ISBN: 9798558877953 E-Books: https://www.amazon.com/dp/B08MQTM1ZP Paperbacks: https://www.amazon.com/dp/B08MSQ3R8R
Introduction To Deep Learning Using R
DOWNLOAD
Author : Taweh Beysolow II
language : en
Publisher: Apress
Release Date : 2017-07-19
Introduction To Deep Learning Using R written by Taweh Beysolow II and has been published by Apress this book supported file pdf, txt, epub, kindle and other format this book has been release on 2017-07-19 with Business & Economics categories.
Understand deep learning, the nuances of its different models, and where these models can be applied. The abundance of data and demand for superior products/services have driven the development of advanced computer science techniques, among them image and speech recognition. Introduction to Deep Learning Using R provides a theoretical and practical understanding of the models that perform these tasks by building upon the fundamentals of data science through machine learning and deep learning. This step-by-step guide will help you understand the disciplines so that you can apply the methodology in a variety of contexts. All examples are taught in the R statistical language, allowing students and professionals to implement these techniques using open source tools. What You'll Learn Understand the intuition and mathematics that power deep learning models Utilize various algorithms using the R programming language and its packages Use best practices for experimental design and variable selection Practice the methodology to approach and effectively solve problems as a data scientist Evaluate the effectiveness of algorithmic solutions and enhance their predictive power Who This Book Is For Students, researchers, and data scientists who are familiar with programming using R. This book also is also of use for those who wish to learn how to appropriately deploy these algorithms in applications where they would be most useful.
Advanced Deep Learning With R
DOWNLOAD
Author : Bharatendra Rai
language : en
Publisher: Packt Publishing Ltd
Release Date : 2019-12-17
Advanced Deep Learning With R written by Bharatendra Rai and has been published by Packt Publishing Ltd this book supported file pdf, txt, epub, kindle and other format this book has been release on 2019-12-17 with Computers categories.
Discover best practices for choosing, building, training, and improving deep learning models using Keras-R, and TensorFlow-R libraries Key FeaturesImplement deep learning algorithms to build AI models with the help of tips and tricksUnderstand how deep learning models operate using expert techniquesApply reinforcement learning, computer vision, GANs, and NLP using a range of datasetsBook Description Deep learning is a branch of machine learning based on a set of algorithms that attempt to model high-level abstractions in data. Advanced Deep Learning with R will help you understand popular deep learning architectures and their variants in R, along with providing real-life examples for them. This deep learning book starts by covering the essential deep learning techniques and concepts for prediction and classification. You will learn about neural networks, deep learning architectures, and the fundamentals for implementing deep learning with R. The book will also take you through using important deep learning libraries such as Keras-R and TensorFlow-R to implement deep learning algorithms within applications. You will get up to speed with artificial neural networks, recurrent neural networks, convolutional neural networks, long short-term memory networks, and more using advanced examples. Later, you'll discover how to apply generative adversarial networks (GANs) to generate new images; autoencoder neural networks for image dimension reduction, image de-noising and image correction and transfer learning to prepare, define, train, and model a deep neural network. By the end of this book, you will be ready to implement your knowledge and newly acquired skills for applying deep learning algorithms in R through real-world examples. What you will learnLearn how to create binary and multi-class deep neural network modelsImplement GANs for generating new imagesCreate autoencoder neural networks for image dimension reduction, image de-noising and image correctionImplement deep neural networks for performing efficient text classificationLearn to define a recurrent convolutional network model for classification in KerasExplore best practices and tips for performance optimization of various deep learning modelsWho this book is for This book is for data scientists, machine learning practitioners, deep learning researchers and AI enthusiasts who want to develop their skills and knowledge to implement deep learning techniques and algorithms using the power of R. A solid understanding of machine learning and working knowledge of the R programming language are required.
Deep Learning With R For Beginners
DOWNLOAD
Author : Mark Hodnett
language : en
Publisher: Packt Publishing Ltd
Release Date : 2019-05-20
Deep Learning With R For Beginners written by Mark Hodnett and has been published by Packt Publishing Ltd this book supported file pdf, txt, epub, kindle and other format this book has been release on 2019-05-20 with Computers categories.
Explore the world of neural networks by building powerful deep learning models using the R ecosystem Key FeaturesGet to grips with the fundamentals of deep learning and neural networksUse R 3.5 and its libraries and APIs to build deep learning models for computer vision and text processingImplement effective deep learning systems in R with the help of end-to-end projectsBook Description Deep learning finds practical applications in several domains, while R is the preferred language for designing and deploying deep learning models. This Learning Path introduces you to the basics of deep learning and even teaches you to build a neural network model from scratch. As you make your way through the chapters, you’ll explore deep learning libraries and understand how to create deep learning models for a variety of challenges, right from anomaly detection to recommendation systems. The book will then help you cover advanced topics, such as generative adversarial networks (GANs), transfer learning, and large-scale deep learning in the cloud, in addition to model optimization, overfitting, and data augmentation. Through real-world projects, you’ll also get up to speed with training convolutional neural networks (CNNs), recurrent neural networks (RNNs), and long short-term memory networks (LSTMs) in R. By the end of this Learning Path, you’ll be well versed with deep learning and have the skills you need to implement a number of deep learning concepts in your research work or projects. This Learning Path includes content from the following Packt products: R Deep Learning Essentials - Second Edition by Joshua F. Wiley and Mark HodnettR Deep Learning Projects by Yuxi (Hayden) Liu and Pablo MaldonadoWhat you will learnImplement credit card fraud detection with autoencodersTrain neural networks to perform handwritten digit recognition using MXNetReconstruct images using variational autoencodersExplore the applications of autoencoder neural networks in clustering and dimensionality reductionCreate natural language processing (NLP) models using Keras and TensorFlow in RPrevent models from overfitting the data to improve generalizabilityBuild shallow neural network prediction modelsWho this book is for This Learning Path is for aspiring data scientists, data analysts, machine learning developers, and deep learning enthusiasts who are well versed in machine learning concepts and are looking to explore the deep learning paradigm using R. A fundamental understanding of R programming and familiarity with the basic concepts of deep learning are necessary to get the most out of this Learning Path.
Hands On Deep Learning With R
DOWNLOAD
Author : Michael Pawlus
language : en
Publisher: Packt Publishing Ltd
Release Date : 2020-04-24
Hands On Deep Learning With R written by Michael Pawlus and has been published by Packt Publishing Ltd this book supported file pdf, txt, epub, kindle and other format this book has been release on 2020-04-24 with Computers categories.
Explore and implement deep learning to solve various real-world problems using modern R libraries such as TensorFlow, MXNet, H2O, and Deepnet Key FeaturesUnderstand deep learning algorithms and architectures using R and determine which algorithm is best suited for a specific problemImprove models using parameter tuning, feature engineering, and ensemblingApply advanced neural network models such as deep autoencoders and generative adversarial networks (GANs) across different domainsBook Description Deep learning enables efficient and accurate learning from a massive amount of data. This book will help you overcome a number of challenges using various deep learning algorithms and architectures with R programming. This book starts with a brief overview of machine learning and deep learning and how to build your first neural network. You’ll understand the architecture of various deep learning algorithms and their applicable fields, learn how to build deep learning models, optimize hyperparameters, and evaluate model performance. Various deep learning applications in image processing, natural language processing (NLP), recommendation systems, and predictive analytics will also be covered. Later chapters will show you how to tackle recognition problems such as image recognition and signal detection, programmatically summarize documents, conduct topic modeling, and forecast stock market prices. Toward the end of the book, you will learn the common applications of GANs and how to build a face generation model using them. Finally, you’ll get to grips with using reinforcement learning and deep reinforcement learning to solve various real-world problems. By the end of this deep learning book, you will be able to build and deploy your own deep learning applications using appropriate frameworks and algorithms. What you will learnDesign a feedforward neural network to see how the activation function computes an outputCreate an image recognition model using convolutional neural networks (CNNs)Prepare data, decide hidden layers and neurons and train your model with the backpropagation algorithmApply text cleaning techniques to remove uninformative text using NLPBuild, train, and evaluate a GAN model for face generationUnderstand the concept and implementation of reinforcement learning in RWho this book is for This book is for data scientists, machine learning engineers, and deep learning developers who are familiar with machine learning and are looking to enhance their knowledge of deep learning using practical examples. Anyone interested in increasing the efficiency of their machine learning applications and exploring various options in R will also find this book useful. Basic knowledge of machine learning techniques and working knowledge of the R programming language is expected.
R Deep Learning Essentials
DOWNLOAD
Author : Mark Hodnett
language : en
Publisher: Packt Publishing Ltd
Release Date : 2018-08-24
R Deep Learning Essentials written by Mark Hodnett and has been published by Packt Publishing Ltd this book supported file pdf, txt, epub, kindle and other format this book has been release on 2018-08-24 with Computers categories.
Implement neural network models in R 3.5 using TensorFlow, Keras, and MXNet Key Features Use R 3.5 for building deep learning models for computer vision and text Apply deep learning techniques in cloud for large-scale processing Build, train, and optimize neural network models on a range of datasets Book Description Deep learning is a powerful subset of machine learning that is very successful in domains such as computer vision and natural language processing (NLP). This second edition of R Deep Learning Essentials will open the gates for you to enter the world of neural networks by building powerful deep learning models using the R ecosystem. This book will introduce you to the basic principles of deep learning and teach you to build a neural network model from scratch. As you make your way through the book, you will explore deep learning libraries, such as Keras, MXNet, and TensorFlow, and create interesting deep learning models for a variety of tasks and problems, including structured data, computer vision, text data, anomaly detection, and recommendation systems. You’ll cover advanced topics, such as generative adversarial networks (GANs), transfer learning, and large-scale deep learning in the cloud. In the concluding chapters, you will learn about the theoretical concepts of deep learning projects, such as model optimization, overfitting, and data augmentation, together with other advanced topics. By the end of this book, you will be fully prepared and able to implement deep learning concepts in your research work or projects. What you will learn Build shallow neural network prediction models Prevent models from overfitting the data to improve generalizability Explore techniques for finding the best hyperparameters for deep learning models Create NLP models using Keras and TensorFlow in R Use deep learning for computer vision tasks Implement deep learning tasks, such as NLP, recommendation systems, and autoencoders Who this book is for This second edition of R Deep Learning Essentials is for aspiring data scientists, data analysts, machine learning developers, and deep learning enthusiasts who are well versed in machine learning concepts and are looking to explore the deep learning paradigm using R. Fundamental understanding of the R language is necessary to get the most out of this book.
Deep Learning With R Second Edition
DOWNLOAD
Author : Francois Chollet
language : en
Publisher: Simon and Schuster
Release Date : 2022-09-13
Deep Learning With R Second Edition written by Francois Chollet and has been published by Simon and Schuster this book supported file pdf, txt, epub, kindle and other format this book has been release on 2022-09-13 with Computers categories.
Deep learning from the ground up using R and the powerful Keras library! In Deep Learning with R, Second Edition you will learn: Deep learning from first principles Image classification and image segmentation Time series forecasting Text classification and machine translation Text generation, neural style transfer, and image generation Deep Learning with R, Second Edition shows you how to put deep learning into action. It’s based on the revised new edition of François Chollet’s bestselling Deep Learning with Python. All code and examples have been expertly translated to the R language by Tomasz Kalinowski, who maintains the Keras and Tensorflow R packages at RStudio. Novices and experienced ML practitioners will love the expert insights, practical techniques, and important theory for building neural networks. About the technology Deep learning has become essential knowledge for data scientists, researchers, and software developers. The R language APIs for Keras and TensorFlow put deep learning within reach for all R users, even if they have no experience with advanced machine learning or neural networks. This book shows you how to get started on core DL tasks like computer vision, natural language processing, and more using R. About the book Deep Learning with R, Second Edition is a hands-on guide to deep learning using the R language. As you move through this book, you’ll quickly lock in the foundational ideas of deep learning. The intuitive explanations, crisp illustrations, and clear examples guide you through core DL skills like image processing and text manipulation, and even advanced features like transformers. This revised and expanded new edition is adapted from Deep Learning with Python, Second Edition by François Chollet, the creator of the Keras library. What's inside Image classification and image segmentation Time series forecasting Text classification and machine translation Text generation, neural style transfer, and image generation About the reader For readers with intermediate R skills. No previous experience with Keras, TensorFlow, or deep learning is required. About the author François Chollet is a software engineer at Google and creator of Keras. Tomasz Kalinowski is a software engineer at RStudio and maintainer of the Keras and Tensorflow R packages. J.J. Allaire is the founder of RStudio, and the author of the first edition of this book. Table of Contents 1 What is deep learning? 2 The mathematical building blocks of neural networks 3 Introduction to Keras and TensorFlow 4 Getting started with neural networks: Classification and regression 5 Fundamentals of machine learning 6 The universal workflow of machine learning 7 Working with Keras: A deep dive 8 Introduction to deep learning for computer vision 9 Advanced deep learning for computer vision 10 Deep learning for time series 11 Deep learning for text 12 Generative deep learning 13 Best practices for the real world 14 Conclusions
R Deep Learning Projects
DOWNLOAD
Author : Yuxi (Hayden) Liu
language : en
Publisher: Packt Publishing Ltd
Release Date : 2018-02-22
R Deep Learning Projects written by Yuxi (Hayden) Liu and has been published by Packt Publishing Ltd this book supported file pdf, txt, epub, kindle and other format this book has been release on 2018-02-22 with Mathematics categories.
5 real-world projects to help you master deep learning concepts Key Features Master the different deep learning paradigms and build real-world projects related to text generation, sentiment analysis, fraud detection, and more Get to grips with R's impressive range of Deep Learning libraries and frameworks such as deepnet, MXNetR, Tensorflow, H2O, Keras, and text2vec Practical projects that show you how to implement different neural networks with helpful tips, tricks, and best practices Book Description R is a popular programming language used by statisticians and mathematicians for statistical analysis, and is popularly used for deep learning. Deep Learning, as we all know, is one of the trending topics today, and is finding practical applications in a lot of domains. This book demonstrates end-to-end implementations of five real-world projects on popular topics in deep learning such as handwritten digit recognition, traffic light detection, fraud detection, text generation, and sentiment analysis. You'll learn how to train effective neural networks in R—including convolutional neural networks, recurrent neural networks, and LSTMs—and apply them in practical scenarios. The book also highlights how neural networks can be trained using GPU capabilities. You will use popular R libraries and packages—such as MXNetR, H2O, deepnet, and more—to implement the projects. By the end of this book, you will have a better understanding of deep learning concepts and techniques and how to use them in a practical setting. What you will learn Instrument Deep Learning models with packages such as deepnet, MXNetR, Tensorflow, H2O, Keras, and text2vec Apply neural networks to perform handwritten digit recognition using MXNet Get the knack of CNN models, Neural Network API, Keras, and TensorFlow for traffic sign classification -Implement credit card fraud detection with Autoencoders Master reconstructing images using variational autoencoders Wade through sentiment analysis from movie reviews Run from past to future and vice versa with bidirectional Long Short-Term Memory (LSTM) networks Understand the applications of Autoencoder Neural Networks in clustering and dimensionality reduction Who this book is for Machine learning professionals and data scientists looking to master deep learning by implementing practical projects in R will find this book a useful resource. A knowledge of R programming and the basic concepts of deep learning is required to get the best out of this book.
Applied Supervised Learning With R
DOWNLOAD
Author : Karthik Ramasubramanian
language : en
Publisher: Packt Publishing Ltd
Release Date : 2019-05-31
Applied Supervised Learning With R written by Karthik Ramasubramanian and has been published by Packt Publishing Ltd this book supported file pdf, txt, epub, kindle and other format this book has been release on 2019-05-31 with Computers categories.
Learn the ropes of supervised machine learning with R by studying popular real-world use-cases, and understand how it drives object detection in driver less cars, customer churn, and loan default prediction. Key FeaturesStudy supervised learning algorithms by using real-world datasets Fine tune optimal parameters with hyperparameter optimizationSelect the best algorithm using the model evaluation frameworkBook Description R provides excellent visualization features that are essential for exploring data before using it in automated learning. Applied Supervised Learning with R helps you cover the complete process of employing R to develop applications using supervised machine learning algorithms for your business needs. The book starts by helping you develop your analytical thinking to create a problem statement using business inputs and domain research. You will then learn different evaluation metrics that compare various algorithms, and later progress to using these metrics to select the best algorithm for your problem. After finalizing the algorithm you want to use, you will study the hyperparameter optimization technique to fine-tune your set of optimal parameters. To prevent you from overfitting your model, a dedicated section will even demonstrate how you can add various regularization terms. By the end of this book, you will have the advanced skills you need for modeling a supervised machine learning algorithm that precisely fulfills your business needs. What you will learnDevelop analytical thinking to precisely identify a business problemWrangle data with dplyr, tidyr, and reshape2Visualize data with ggplot2Validate your supervised machine learning model using k-fold Optimize hyperparameters with grid and random search, and Bayesian optimizationDeploy your model on Amazon Web Services (AWS) Lambda with plumberImprove your model’s performance with feature selection and dimensionality reductionWho this book is for This book is specially designed for novice and intermediate-level data analysts, data scientists, and data engineers who want to explore different methods of supervised machine learning and its various use cases. Some background in statistics, probability, calculus, linear algebra, and programming will help you thoroughly understand and follow the content of this book.