Deep Neural Networks And Image Analysis For Quantitative Microscopy
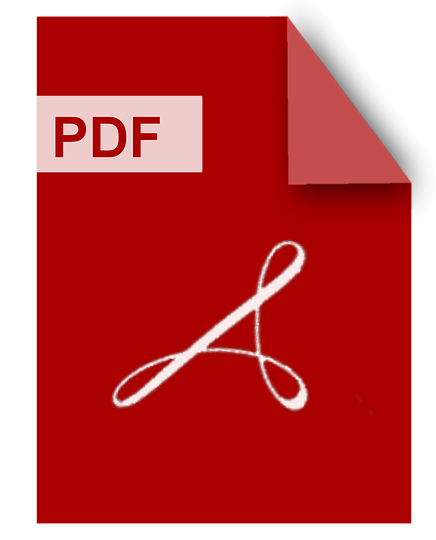
DOWNLOAD
Download Deep Neural Networks And Image Analysis For Quantitative Microscopy PDF/ePub or read online books in Mobi eBooks. Click Download or Read Online button to get Deep Neural Networks And Image Analysis For Quantitative Microscopy book now. This website allows unlimited access to, at the time of writing, more than 1.5 million titles, including hundreds of thousands of titles in various foreign languages. If the content not found or just blank you must refresh this page
Deep Neural Networks And Image Analysis For Quantitative Microscopy
DOWNLOAD
Author : Sajith Kecheril Sadanandan
language : en
Publisher:
Release Date : 2017
Deep Neural Networks And Image Analysis For Quantitative Microscopy written by Sajith Kecheril Sadanandan and has been published by this book supported file pdf, txt, epub, kindle and other format this book has been release on 2017 with categories.
Microscope Image Processing
DOWNLOAD
Author : Fatima Merchant
language : en
Publisher: Academic Press
Release Date : 2022-08-26
Microscope Image Processing written by Fatima Merchant and has been published by Academic Press this book supported file pdf, txt, epub, kindle and other format this book has been release on 2022-08-26 with Computers categories.
Microscope Image Processing, Second Edition, introduces the basic fundamentals of image formation in microscopy including the importance of image digitization and display, which are key to quality visualization. Image processing and analysis are discussed in detail to provide readers with the tools necessary to improve the visual quality of images, and to extract quantitative information. Basic techniques such as image enhancement, filtering, segmentation, object measurement, and pattern recognition cover concepts integral to image processing. In addition, chapters on specific modern microscopy techniques such as fluorescence imaging, multispectral imaging, three-dimensional imaging and time-lapse imaging, introduce these key areas with emphasis on the differences among the various techniques. The new edition discusses recent developments in microscopy such as light sheet microscopy, digital microscopy, whole slide imaging, and the use of deep learning techniques for image segmentation and analysis with big data image informatics and management. Microscope Image Processing, Second Edition, is suitable for engineers, scientists, clinicians, post-graduate fellows and graduate students working in bioengineering, biomedical engineering, biology, medicine, chemistry, pharmacology and related fields, who use microscopes in their work and would like to understand the methodologies and capabilities of the latest digital image processing techniques or desire to develop their own image processing algorithms and software for specific applications. Presents a unique practical perspective of state-of-the-art microscope image processing and the development of specialized algorithms Each chapter includes in-depth analysis of methods coupled with the results of specific real-world experiments Co-edited by Kenneth R. Castleman, world-renowned pioneer in digital image processing and author of two seminal textbooks on the subject
Deep Learning Optics For Computational Microscopy And Diffractive Computing
DOWNLOAD
Author : Bijie Bai
language : en
Publisher:
Release Date : 2023
Deep Learning Optics For Computational Microscopy And Diffractive Computing written by Bijie Bai and has been published by this book supported file pdf, txt, epub, kindle and other format this book has been release on 2023 with categories.
The rapid development of machine learning has transformed conventional optical imaging processes, setting new benchmarks in computational imaging tasks. In this dissertation, we delve into the transformative impact of recent advancements in machine learning on optical imaging processes, focusing on how these technologies revolutionize computational imaging tasks. Specifically, this dissertation centers on two major topics: deep learning-enabled computational microscopy and the all-optical diffractive networks. Optical microscopy has long served as the benchmark technique for diagnosing various diseases over centuries. However, its reliance on high-end optical components and accessories, necessary to adapt to various imaging samples and conditions, often limits its applicability and throughput. Recent advancements in computational imaging techniques utilizing deep learning methods have transformed conventional microscopic imaging modalities, delivering both enhanced speed and superior image quality without introducing extra complexity of the optical systems. In the first topic of this dissertation, we demonstrate that deep learning-enabled image translation approach can significantly benefit a wide range of applications for microscopic imaging. We start with introducing a customized system for single-shot quantitative polarization imaging, capable of reconstructing comprehensive birefringent maps from a single image capture, which offers enhanced sensitivity and specificity in diagnosing crystal-induced diseases. Utilizing these quantitative birefringent maps as a baseline, we employ deep learning tools to convert phase-recovered holograms into quantitative birefringence maps, thereby improving the throughput of crystal detection with simplified system complexity. Extending this concept of deep learning-enabled image translation, we also explore its applications in histopathology. Our technique, termed as "virtual histological staining", transforms unstained biological samples into visually rich, stained-like images without the need for chemical agents. This innovation minimizes costs, labor, and diagnostic delays as well as opens up new possibilities in histopathology workflow. The evolution of deep learning tools not only facilities the optical image analysis and processing, but also provides guidance in design and enhancement of optical systems. The second topic of this dissertation is the development and application of diffractive deep neural networks (D2NN). Developed with deep learning, D2NNs execute given computational tasks by manipulating light diffraction through a series of engineered surfaces, which is completed at the speed of light propagation with negligible power consumption. Based on this framework, a lot of novel computational tasks can be executed in an all-optical way, which is beyond the capabilities of the traditional optics design approaches. We introduce several all-optical computational imaging applications based on D2NN, including class-specific imaging, class-specific image encryption, and unidirectional image magnification and demagnification, demonstrating the versatility of this promising framework.
Handbook Of Research On Deep Learning Based Image Analysis Under Constrained And Unconstrained Environments
DOWNLOAD
Author : Raj, Alex Noel Joseph
language : en
Publisher: IGI Global
Release Date : 2020-12-25
Handbook Of Research On Deep Learning Based Image Analysis Under Constrained And Unconstrained Environments written by Raj, Alex Noel Joseph and has been published by IGI Global this book supported file pdf, txt, epub, kindle and other format this book has been release on 2020-12-25 with Computers categories.
Recent advancements in imaging techniques and image analysis has broadened the horizons for their applications in various domains. Image analysis has become an influential technique in medical image analysis, optical character recognition, geology, remote sensing, and more. However, analysis of images under constrained and unconstrained environments require efficient representation of the data and complex models for accurate interpretation and classification of data. Deep learning methods, with their hierarchical/multilayered architecture, allow the systems to learn complex mathematical models to provide improved performance in the required task. The Handbook of Research on Deep Learning-Based Image Analysis Under Constrained and Unconstrained Environments provides a critical examination of the latest advancements, developments, methods, systems, futuristic approaches, and algorithms for image analysis and addresses its challenges. Highlighting concepts, methods, and tools including convolutional neural networks, edge enhancement, image segmentation, machine learning, and image processing, the book is an essential and comprehensive reference work for engineers, academicians, researchers, and students.
Handbook Of Deep Learning In Biomedical Engineering
DOWNLOAD
Author : Valentina Emilia Balas
language : en
Publisher: Academic Press
Release Date : 2020-11-12
Handbook Of Deep Learning In Biomedical Engineering written by Valentina Emilia Balas and has been published by Academic Press this book supported file pdf, txt, epub, kindle and other format this book has been release on 2020-11-12 with Science categories.
Deep Learning (DL) is a method of machine learning, running over Artificial Neural Networks, that uses multiple layers to extract high-level features from large amounts of raw data. Deep Learning methods apply levels of learning to transform input data into more abstract and composite information. Handbook for Deep Learning in Biomedical Engineering: Techniques and Applications gives readers a complete overview of the essential concepts of Deep Learning and its applications in the field of Biomedical Engineering. Deep learning has been rapidly developed in recent years, in terms of both methodological constructs and practical applications. Deep Learning provides computational models of multiple processing layers to learn and represent data with higher levels of abstraction. It is able to implicitly capture intricate structures of large-scale data and is ideally suited to many of the hardware architectures that are currently available. The ever-expanding amount of data that can be gathered through biomedical and clinical information sensing devices necessitates the development of machine learning and AI techniques such as Deep Learning and Convolutional Neural Networks to process and evaluate the data. Some examples of biomedical and clinical sensing devices that use Deep Learning include: Computed Tomography (CT), Magnetic Resonance Imaging (MRI), Ultrasound, Single Photon Emission Computed Tomography (SPECT), Positron Emission Tomography (PET), Magnetic Particle Imaging, EE/MEG, Optical Microscopy and Tomography, Photoacoustic Tomography, Electron Tomography, and Atomic Force Microscopy. Handbook for Deep Learning in Biomedical Engineering: Techniques and Applications provides the most complete coverage of Deep Learning applications in biomedical engineering available, including detailed real-world applications in areas such as computational neuroscience, neuroimaging, data fusion, medical image processing, neurological disorder diagnosis for diseases such as Alzheimer’s, ADHD, and ASD, tumor prediction, as well as translational multimodal imaging analysis. Presents a comprehensive handbook of the biomedical engineering applications of DL, including computational neuroscience, neuroimaging, time series data such as MRI, functional MRI, CT, EEG, MEG, and data fusion of biomedical imaging data from disparate sources, such as X-Ray/CT Helps readers understand key concepts in DL applications for biomedical engineering and health care, including manifold learning, classification, clustering, and regression in neuroimaging data analysis Provides readers with key DL development techniques such as creation of algorithms and application of DL through artificial neural networks and convolutional neural networks Includes coverage of key application areas of DL such as early diagnosis of specific diseases such as Alzheimer’s, ADHD, and ASD, and tumor prediction through MRI and translational multimodality imaging and biomedical applications such as detection, diagnostic analysis, quantitative measurements, and image guidance of ultrasonography
Deep Learning For Medical Image Analysis
DOWNLOAD
Author : S. Kevin Zhou
language : en
Publisher: Academic Press
Release Date : 2023-12-01
Deep Learning For Medical Image Analysis written by S. Kevin Zhou and has been published by Academic Press this book supported file pdf, txt, epub, kindle and other format this book has been release on 2023-12-01 with Computers categories.
Deep Learning for Medical Image Analysis, Second Edition is a great learning resource for academic and industry researchers and graduate students taking courses on machine learning and deep learning for computer vision and medical image computing and analysis. Deep learning provides exciting solutions for medical image analysis problems and is a key method for future applications. This book gives a clear understanding of the principles and methods of neural network and deep learning concepts, showing how the algorithms that integrate deep learning as a core component are applied to medical image detection, segmentation, registration, and computer-aided analysis. · Covers common research problems in medical image analysis and their challenges · Describes the latest deep learning methods and the theories behind approaches for medical image analysis · Teaches how algorithms are applied to a broad range of application areas including cardiac, neural and functional, colonoscopy, OCTA applications and model assessment · Includes a Foreword written by Nicholas Ayache
Deep Learning For Biomedical Data Analysis
DOWNLOAD
Author : Mourad Elloumi
language : en
Publisher: Springer Nature
Release Date : 2021-07-13
Deep Learning For Biomedical Data Analysis written by Mourad Elloumi and has been published by Springer Nature this book supported file pdf, txt, epub, kindle and other format this book has been release on 2021-07-13 with Medical categories.
This book is the first overview on Deep Learning (DL) for biomedical data analysis. It surveys the most recent techniques and approaches in this field, with both a broad coverage and enough depth to be of practical use to working professionals. This book offers enough fundamental and technical information on these techniques, approaches and the related problems without overcrowding the reader's head. It presents the results of the latest investigations in the field of DL for biomedical data analysis. The techniques and approaches presented in this book deal with the most important and/or the newest topics encountered in this field. They combine fundamental theory of Artificial Intelligence (AI), Machine Learning (ML) and DL with practical applications in Biology and Medicine. Certainly, the list of topics covered in this book is not exhaustive but these topics will shed light on the implications of the presented techniques and approaches on other topics in biomedical data analysis. The book finds a balance between theoretical and practical coverage of a wide range of issues in the field of biomedical data analysis, thanks to DL. The few published books on DL for biomedical data analysis either focus on specific topics or lack technical depth. The chapters presented in this book were selected for quality and relevance. The book also presents experiments that provide qualitative and quantitative overviews in the field of biomedical data analysis. The reader will require some familiarity with AI, ML and DL and will learn about techniques and approaches that deal with the most important and/or the newest topics encountered in the field of DL for biomedical data analysis. He/she will discover both the fundamentals behind DL techniques and approaches, and their applications on biomedical data. This book can also serve as a reference book for graduate courses in Bioinformatics, AI, ML and DL. The book aims not only at professional researchers and practitioners but also graduate students, senior undergraduate students and young researchers. This book will certainly show the way to new techniques and approaches to make new discoveries.
Deep Learning Methods For Fibrotic Tissue Microenvironment Analysis
DOWNLOAD
Author : Bin Li
language : en
Publisher:
Release Date : 2023
Deep Learning Methods For Fibrotic Tissue Microenvironment Analysis written by Bin Li and has been published by this book supported file pdf, txt, epub, kindle and other format this book has been release on 2023 with categories.
Whole slide imaging and multimodal optical microscopy methods developed in the last several decades are currently transforming the conventional practices of histopathology. These imaging methods including associated computational tools are introducing quantitative and comprehensive views of tissue microenvironment to pathologists and researchers for a better understanding of disease development and biological processes. Many of these computational analysis pipelines are powered by machine learning and artificial intelligence (AI), where models are trained on example data and perform predictions on new samples. The performance of computational workflows is further increased attributed to the recent advances of deep learning and the ability to train large deep neural networks (DNNs) on graphics processing units (GPUs).Meanwhile, the development of modern quantitative optical microscopy has greatly increased the contrasts available for characterizing tissue microenvironment for histopathological assessment. The rapid progress of digital histopathology also gave rise to many challenges and opportunities that appeal to more robust and efficient computational workflows, such as whole slide screening, tumor detection, and survival analysis. On the other hand, the clinical use of multimodal microscopy in histopathology still remains limited due to the high cost and complexity of these optical imaging modalities. In this thesis, we present the designs of deep learning-based methods targeting two topics in digital histopathology, namely histological image classification and image generation in tissue microscopy. The first topic involves building classification workflows following the idea of weakly supervised learning, where models are trained with slide-level labels or coarsely annotated region-level labels. The workflows can be further improved regarding computation efficiency and image feature quality, utilizing compression domain processing and graph neural networks, respectively. The second topic focuses on pixel-wise prediction problems where synthetic images are generated by DNNs. We developed such a model capable of synthesizing SHG images from standard clinical modality such that collagen topography can be directly visualized without operating costly and complex SHG imaging instruments. A similar idea can be applied to improve the resolution of WSIs collected using low-cost devices with less expensive optics and lower storage costs. We also show that deep generative models can be used to generate synthetic image data for training a collagen fiber centerline extraction network. The network produces high-quality fiber centerline extraction results for analyzing collagen fiber alignment, length, and density that are previously shown to correlate with disease diagnosis and prognosis. Lastly, we present an open-source automatic multimodal whole slide histopathology imaging system empowered by an open hardware controlling platform and run-time image processing functionality, where deep learning-based selective acquisition and image restoration can be integrated into the acquisition workflow.
Artificial Intelligence And Deep Learning In Pathology
DOWNLOAD
Author : Stanley Cohen
language : en
Publisher: Elsevier Health Sciences
Release Date : 2020-06-02
Artificial Intelligence And Deep Learning In Pathology written by Stanley Cohen and has been published by Elsevier Health Sciences this book supported file pdf, txt, epub, kindle and other format this book has been release on 2020-06-02 with Medical categories.
Recent advances in computational algorithms, along with the advent of whole slide imaging as a platform for embedding artificial intelligence (AI), are transforming pattern recognition and image interpretation for diagnosis and prognosis. Yet most pathologists have just a passing knowledge of data mining, machine learning, and AI, and little exposure to the vast potential of these powerful new tools for medicine in general and pathology in particular. In Artificial Intelligence and Deep Learning in Pathology, Dr. Stanley Cohen covers the nuts and bolts of all aspects of machine learning, up to and including AI, bringing familiarity and understanding to pathologists at all levels of experience. Focuses heavily on applications in medicine, especially pathology, making unfamiliar material accessible and avoiding complex mathematics whenever possible. Covers digital pathology as a platform for primary diagnosis and augmentation via deep learning, whole slide imaging for 2D and 3D analysis, and general principles of image analysis and deep learning. Discusses and explains recent accomplishments such as algorithms used to diagnose skin cancer from photographs, AI-based platforms developed to identify lesions of the retina, using computer vision to interpret electrocardiograms, identifying mitoses in cancer using learning algorithms vs. signal processing algorithms, and many more.
Images As Data For Social Science Research
DOWNLOAD
Author : Nora Webb Williams
language : en
Publisher: Cambridge University Press
Release Date : 2020-08-13
Images As Data For Social Science Research written by Nora Webb Williams and has been published by Cambridge University Press this book supported file pdf, txt, epub, kindle and other format this book has been release on 2020-08-13 with Political Science categories.
Images play a crucial role in shaping and reflecting political life. Digitization has vastly increased the presence of such images in daily life, creating valuable new research opportunities for social scientists. We show how recent innovations in computer vision methods can substantially lower the costs of using images as data. We introduce readers to the deep learning algorithms commonly used for object recognition, facial recognition, and visual sentiment analysis. We then provide guidance and specific instructions for scholars interested in using these methods in their own research.