Detecting Cyberbullying Tweets Using Machine Learning And Deep Learning With Python Gui
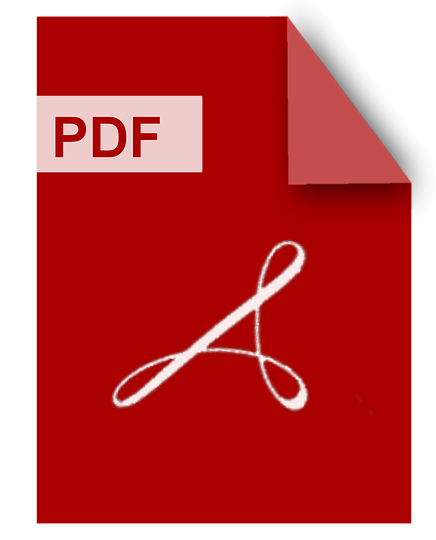
DOWNLOAD
Download Detecting Cyberbullying Tweets Using Machine Learning And Deep Learning With Python Gui PDF/ePub or read online books in Mobi eBooks. Click Download or Read Online button to get Detecting Cyberbullying Tweets Using Machine Learning And Deep Learning With Python Gui book now. This website allows unlimited access to, at the time of writing, more than 1.5 million titles, including hundreds of thousands of titles in various foreign languages. If the content not found or just blank you must refresh this page
Detecting Cyberbullying Tweets Using Machine Learning And Deep Learning With Python Gui
DOWNLOAD
Author : Vivian Siahaan
language : en
Publisher: BALIGE PUBLISHING
Release Date : 2023-08-05
Detecting Cyberbullying Tweets Using Machine Learning And Deep Learning With Python Gui written by Vivian Siahaan and has been published by BALIGE PUBLISHING this book supported file pdf, txt, epub, kindle and other format this book has been release on 2023-08-05 with Computers categories.
This project focuses on detecting cyberbullying tweets using both Machine Learning and Deep Learning techniques with a Python GUI implemented using PyQt. The first step involves data exploration, where the dataset is loaded and analyzed to gain insights into its structure and contents. Visualizations are created to understand the distribution of cyberbullying types and other features in the data. After data exploration, preprocessing is performed to clean and prepare the tweets for analysis. Text cleaning techniques, such as removing emojis, punctuation, links, and stop words, are applied to the tweet text. The data is then categorized based on the length of the tweets to facilitate further analysis. Next, the data is split into input and output variables, where the tweet text becomes the input feature (X) and the cyberbullying type becomes the output (y). The cyberbullying types are converted into numerical labels for ML models' compatibility. Machine Learning models are trained and evaluated using TF-IDF, Count Vectorizer, and Hashing Vectorizer as feature extraction techniques. SMOTE is applied to handle class imbalance, and the data is split into training and testing sets. Grid Search is utilized to find the best hyperparameters for ML models, optimizing their performance. Machine Learning models used are Logistic Regression, Support Vector Machines, K-Nearest Neighbors, Decision Trees, Random Forests, Gradient Boosting, Extreme Gradient Boosting, Light Gradient Boosting. Moving to Deep Learning, LSTM (Long Short-Term Memory) and 1D CNN (Convolutional Neural Network) models are constructed to detect cyberbullying types. The tweet text is embedded, and various layers are added to the models to extract meaningful features. The models are then compiled with appropriate loss functions and optimizers. The evaluation process is carried out using the test set for both Machine Learning and Deep Learning models. Metrics like accuracy, precision, recall, and F1-score are used to assess the models' performance in detecting cyberbullying types. To enable easy access to the functionalities, a Graphical User Interface (GUI) is developed using PyQt. The GUI allows users to interact with the models and dataset easily. Users can select the feature extraction technique, choose the classifier, and initiate the model training process using the GUI's buttons and dropdown menus. For better data visualization, the GUI includes various plots, such as bar plots and pie charts, to show the distribution of cyberbullying types and other features. These visualizations help users understand the data and model performance intuitively. The final stage involves testing the GUI with different inputs and exploring model predictions on user-provided text. The GUI provides predictions for the given tweet text, indicating the likelihood of each cyberbullying type based on the trained models. Overall, this project combines data exploration, preprocessing, feature extraction using Machine Learning and Deep Learning models, GUI development, and data visualization to detect cyberbullying tweets effectively and provide an accessible interface for users to interact with the models. The end product allows users to analyze tweets for potential cyberbullying and contributes to promoting a safer and more respectful online environment.
5 Five Data Science Projects For Analysis Classification Prediction And Sentiment Analysis With Python Gui
DOWNLOAD
Author : Vivian Siahaan
language : en
Publisher: BALIGE PUBLISHING
Release Date : 2022-04-29
5 Five Data Science Projects For Analysis Classification Prediction And Sentiment Analysis With Python Gui written by Vivian Siahaan and has been published by BALIGE PUBLISHING this book supported file pdf, txt, epub, kindle and other format this book has been release on 2022-04-29 with Computers categories.
PROJECT 1: SUPERMARKET SALES ANALYSIS AND PREDICTION USING MACHINE LEARNING WITH PYTHON GUI The dataset used in this project consists of the growth of supermarkets with high market competitions in most populated cities. The dataset is one of the historical sales of supermarket company which has recorded in 3 different branches for 3 months data. Predictive data analytics methods are easy to apply with this dataset. Attribute information in the dataset are as follows: Invoice id: Computer generated sales slip invoice identification number; Branch: Branch of supercenter (3 branches are available identified by A, B and C); City: Location of supercenters; Customer type: Type of customers, recorded by Members for customers using member card and Normal for without member card; Gender: Gender type of customer; Product line: General item categorization groups - Electronic accessories, Fashion accessories, Food and beverages, Health and beauty, Home and lifestyle, Sports and travel; Unit price: Price of each product in $; Quantity: Number of products purchased by customer; Tax: 5% tax fee for customer buying; Total: Total price including tax; Date: Date of purchase (Record available from January 2019 to March 2019); Time: Purchase time (10am to 9pm); Payment: Payment used by customer for purchase (3 methods are available – Cash, Credit card and Ewallet); COGS: Cost of goods sold; Gross margin percentage: Gross margin percentage; Gross income: Gross income; and Rating: Customer stratification rating on their overall shopping experience (On a scale of 1 to 10). In this project, you will perform predicting rating using machine learning. The machine learning models used in this project to predict clusters as target variable are K-Nearest Neighbor, Random Forest, Naive Bayes, Logistic Regression, Decision Tree, Support Vector Machine, LGBM, Gradient Boosting, XGB, and MLP. Finally, you will plot boundary decision, distribution of features, feature importance, cross validation score, and predicted values versus true values, confusion matrix, learning curve, performance of the model, scalability of the model, training loss, and training accuracy. PROJECT 2: DETECTING CYBERBULLYING TWEETS USING MACHINE LEARNING AND DEEP LEARNING WITH PYTHON GUI As social media usage becomes increasingly prevalent in every age group, a vast majority of citizens rely on this essential medium for day-to-day communication. Social media’s ubiquity means that cyberbullying can effectively impact anyone at any time or anywhere, and the relative anonymity of the internet makes such personal attacks more difficult to stop than traditional bullying. On April 15th, 2020, UNICEF issued a warning in response to the increased risk of cyberbullying during the COVID-19 pandemic due to widespread school closures, increased screen time, and decreased face-to-face social interaction. The statistics of cyberbullying are outright alarming: 36.5% of middle and high school students have felt cyberbullied and 87% have observed cyberbullying, with effects ranging from decreased academic performance to depression to suicidal thoughts. In light of all of this, this dataset contains more than 47000 tweets labelled according to the class of cyberbullying: Age; Ethnicity; Gender; Religion; Other type of cyberbullying; and Not cyberbullying. The data has been balanced in order to contain ~8000 of each class. The models used in this project are K-Nearest Neighbor, Random Forest, Naive Bayes, Logistic Regression, Decision Tree, Support Vector Machine, Adaboost, LGBM classifier, Gradient Boosting, XGB classifier, LSTM, and CNN. Three feature scaling used in machine learning are raw, minmax scaler, and standard scaler. Finally, you will develop a GUI using PyQt5 to plot cross validation score, predicted values versus true values, confusion matrix, learning curve, decision boundaries, performance of the model, scalability of the model, training loss, and training accuracy. PROJECT 3: HIGHER EDUCATION STUDENT ACADEMIC PERFORMANCE ANALYSIS AND PREDICTION USING MACHINE LEARNING WITH PYTHON GUI The dataset used in this project was collected from the Faculty of Engineering and Faculty of Educational Sciences students in 2019. The purpose is to predict students' end-of-term performances using ML techniques. Attribute information in the dataset are as follows: Student ID; Student Age (1: 18-21, 2: 22-25, 3: above 26); Sex (1: female, 2: male); Graduated high-school type: (1: private, 2: state, 3: other); Scholarship type: (1: None, 2: 25%, 3: 50%, 4: 75%, 5: Full); Additional work: (1: Yes, 2: No); Regular artistic or sports activity: (1: Yes, 2: No); Do you have a partner: (1: Yes, 2: No); Total salary if available (1: USD 135-200, 2: USD 201-270, 3: USD 271-340, 4: USD 341-410, 5: above 410); Transportation to the university: (1: Bus, 2: Private car/taxi, 3: bicycle, 4: Other); Accommodation type in Cyprus: (1: rental, 2: dormitory, 3: with family, 4: Other); Mother's education: (1: primary school, 2: secondary school, 3: high school, 4: university, 5: MSc., 6: Ph.D.); Father's education: (1: primary school, 2: secondary school, 3: high school, 4: university, 5: MSc., 6: Ph.D.); Number of sisters/brothers (if available): (1: 1, 2:, 2, 3: 3, 4: 4, 5: 5 or above); Parental status: (1: married, 2: divorced, 3: died - one of them or both); Mother's occupation: (1: retired, 2: housewife, 3: government officer, 4: private sector employee, 5: self-employment, 6: other); Father's occupation: (1: retired, 2: government officer, 3: private sector employee, 4: self-employment, 5: other); Weekly study hours: (1: None, 2: <5 hours, 3: 6-10 hours, 4: 11-20 hours, 5: more than 20 hours); Reading frequency (non-scientific books/journals): (1: None, 2: Sometimes, 3: Often); Reading frequency (scientific books/journals): (1: None, 2: Sometimes, 3: Often); Attendance to the seminars/conferences related to the department: (1: Yes, 2: No); Impact of your projects/activities on your success: (1: positive, 2: negative, 3: neutral); Attendance to classes (1: always, 2: sometimes, 3: never); Preparation to midterm exams 1: (1: alone, 2: with friends, 3: not applicable); Preparation to midterm exams 2: (1: closest date to the exam, 2: regularly during the semester, 3: never); Taking notes in classes: (1: never, 2: sometimes, 3: always); Listening in classes: (1: never, 2: sometimes, 3: always); Discussion improves my interest and success in the course: (1: never, 2: sometimes, 3: always); Flip-classroom: (1: not useful, 2: useful, 3: not applicable); Cumulative grade point average in the last semester (/4.00): (1: <2.00, 2: 2.00-2.49, 3: 2.50-2.99, 4: 3.00-3.49, 5: above 3.49); Expected Cumulative grade point average in the graduation (/4.00): (1: <2.00, 2: 2.00-2.49, 3: 2.50-2.99, 4: 3.00-3.49, 5: above 3.49); Course ID; and OUTPUT: Grade (0: Fail, 1: DD, 2: DC, 3: CC, 4: CB, 5: BB, 6: BA, 7: AA). The models used in this project are K-Nearest Neighbor, Random Forest, Naive Bayes, Logistic Regression, Decision Tree, Support Vector Machine, Adaboost, LGBM classifier, Gradient Boosting, and XGB classifier. Three feature scaling used in machine learning are raw, minmax scaler, and standard scaler. Finally, you will develop a GUI using PyQt5 to plot cross validation score, predicted values versus true values, confusion matrix, learning curve, decision boundaries, performance of the model, scalability of the model, training loss, and training accuracy. PROJECT 4: COMPANY BANKRUPTCY ANALYSIS AND PREDICTION USING MACHINE LEARNING WITH PYTHON GUI The dataset was collected from the Taiwan Economic Journal for the years 1999 to 2009. Company bankruptcy was defined based on the business regulations of the Taiwan Stock Exchange. Attribute information in the dataset are as follows: Y - Bankrupt?: Class label; X1 - ROA(C) before interest and depreciation before interest: Return On Total Assets(C); X2 - ROA(A) before interest and % after tax: Return On Total Assets(A); X3 - ROA(B) before interest and depreciation after tax: Return On Total Assets(B); X4 - Operating Gross Margin: Gross Profit/Net Sales; X5 - Realized Sales Gross Margin: Realized Gross Profit/Net Sales; X6 - Operating Profit Rate: Operating Income/Net Sales; X7 - Pre-tax net Interest Rate: Pre-Tax Income/Net Sales; X8 - After-tax net Interest Rate: Net Income/Net Sales; X9 - Non-industry income and expenditure/revenue: Net Non-operating Income Ratio; X10 - Continuous interest rate (after tax): Net Income-Exclude Disposal Gain or Loss/Net Sales; X11 - Operating Expense Rate: Operating Expenses/Net Sales; X12 - Research and development expense rate: (Research and Development Expenses)/Net Sales X13 - Cash flow rate: Cash Flow from Operating/Current Liabilities; X14 - Interest-bearing debt interest rate: Interest-bearing Debt/Equity; X15 - Tax rate (A): Effective Tax Rate; X16 - Net Value Per Share (B): Book Value Per Share(B); X17 - Net Value Per Share (A): Book Value Per Share(A); X18 - Net Value Per Share (C): Book Value Per Share(C); X19 - Persistent EPS in the Last Four Seasons: EPS-Net Income; X20 - Cash Flow Per Share; X21 - Revenue Per Share (Yuan ¥): Sales Per Share; X22 - Operating Profit Per Share (Yuan ¥): Operating Income Per Share; X23 - Per Share Net profit before tax (Yuan ¥): Pretax Income Per Share; X24 - Realized Sales Gross Profit Growth Rate; X25 - Operating Profit Growth Rate: Operating Income Growth; X26 - After-tax Net Profit Growth Rate: Net Income Growth; X27 - Regular Net Profit Growth Rate: Continuing Operating Income after Tax Growth; X28 - Continuous Net Profit Growth Rate: Net Income-Excluding Disposal Gain or Loss Growth; X29 - Total Asset Growth Rate: Total Asset Growth; X30 - Net Value Growth Rate: Total Equity Growth; X31 - Total Asset Return Growth Rate Ratio: Return on Total Asset Growth; X32 - Cash Reinvestment %: Cash Reinvestment Ratio X33 - Current Ratio; X34 - Quick Ratio: Acid Test; X35 - Interest Expense Ratio: Interest Expenses/Total Revenue; X36 - Total debt/Total net worth: Total Liability/Equity Ratio; X37 - Debt ratio %: Liability/Total Assets; X38 - Net worth/Assets: Equity/Total Assets; X39 - Long-term fund suitability ratio (A): (Long-term Liability+Equity)/Fixed Assets; X40 - Borrowing dependency: Cost of Interest-bearing Debt; X41 - Contingent liabilities/Net worth: Contingent Liability/Equity; X42 - Operating profit/Paid-in capital: Operating Income/Capital; X43 - Net profit before tax/Paid-in capital: Pretax Income/Capital; X44 - Inventory and accounts receivable/Net value: (Inventory+Accounts Receivables)/Equity; X45 - Total Asset Turnover; X46 - Accounts Receivable Turnover; X47 - Average Collection Days: Days Receivable Outstanding; X48 - Inventory Turnover Rate (times); X49 - Fixed Assets Turnover Frequency; X50 - Net Worth Turnover Rate (times): Equity Turnover; X51 - Revenue per person: Sales Per Employee; X52 - Operating profit per person: Operation Income Per Employee; X53 - Allocation rate per person: Fixed Assets Per Employee; X54 - Working Capital to Total Assets; X55 - Quick Assets/Total Assets; X56 - Current Assets/Total Assets; X57 - Cash/Total Assets; X58 - Quick Assets/Current Liability; X59 - Cash/Current Liability; X60 - Current Liability to Assets; X61 - Operating Funds to Liability; X62 - Inventory/Working Capital; X63 - Inventory/Current Liability X64 - Current Liabilities/Liability; X65 - Working Capital/Equity; X66 - Current Liabilities/Equity; X67 - Long-term Liability to Current Assets; X68 - Retained Earnings to Total Assets; X69 - Total income/Total expense; X70 - Total expense/Assets; X71 - Current Asset Turnover Rate: Current Assets to Sales; X72 - Quick Asset Turnover Rate: Quick Assets to Sales; X73 - Working capitcal Turnover Rate: Working Capital to Sales; X74 - Cash Turnover Rate: Cash to Sales; X75 - Cash Flow to Sales; X76 - Fixed Assets to Assets; X77 - Current Liability to Liability; X78 - Current Liability to Equity; X79 - Equity to Long-term Liability; X80 - Cash Flow to Total Assets; X81 - Cash Flow to Liability; X82 - CFO to Assets; X83 - Cash Flow to Equity; X84 - Current Liability to Current Assets; X85 - Liability-Assets Flag: 1 if Total Liability exceeds Total Assets, 0 otherwise; X86 - Net Income to Total Assets; X87 - Total assets to GNP price; X88 - No-credit Interval; X89 - Gross Profit to Sales; X90 - Net Income to Stockholder's Equity; X91 - Liability to Equity; X92 - Degree of Financial Leverage (DFL); X93 - Interest Coverage Ratio (Interest expense to EBIT); X94 - Net Income Flag: 1 if Net Income is Negative for the last two years, 0 otherwise; and X95 - Equity to Liabilitys. The models used in this project are K-Nearest Neighbor, Random Forest, Naive Bayes, Logistic Regression, Decision Tree, Support Vector Machine, Adaboost, LGBM classifier, Gradient Boosting, and XGB classifier. Three feature scaling used in machine learning are raw, minmax scaler, and standard scaler. Finally, you will develop a GUI using PyQt5 to plot cross validation score, predicted values versus true values, confusion matrix, learning curve, decision boundaries, performance of the model, scalability of the model, training loss, and training accuracy. PROJECT 5: DATA SCIENCE FOR RAIN CLASSIFICATION AND PREDICTION WITH PYTHON GUI This dataset contains about 10 years of daily weather observations from many locations across Australia. RainTomorrow is the target variable to predict. You will determine rain or not in the next day. This column is Yes if the rain for that day was 1mm or more. Observations were drawn from numerous weather stations. The daily observations are available from http://www.bom.gov.au/climate/data. The dataset contains 23 attributes. Some of them are as follows: About some of them are: DATE - The date of observation; LOCATION - The common name of the location of the weather station; MINTEMP - The minimum temperature in degrees celsius; MAXTEMP - The maximum temperature in degrees celsius; RAINFALL - The amount of rainfall recorded for the day in mm; EVAPORATION - The so-called Class A pan evaporation (mm) in the 24 hours to 9am; SUNSHINE - The number of hours of bright sunshine in the day; WINDGUESTDIR - The direction of the strongest wind gust in the 24 hours to midnight; WINDGUESTSPEED- The speed (km/h) of the strongest wind gust in the 24 hours to midnight; and WINDDIR9AM - Direction of the wind at 9am. The models used in this project are K-Nearest Neighbor, Random Forest, Naive Bayes, Logistic Regression, Decision Tree, Support Vector Machine, Adaboost, LGBM classifier, Gradient Boosting, and XGB classifier. Three feature scaling used in machine learning are raw, minmax scaler, and standard scaler. Finally, you will develop a GUI using PyQt5 to plot cross validation score, predicted values versus true values, confusion matrix, learning curve, decision boundaries, performance of the model, scalability of the model, training loss, and training accuracy.
Hate Speech Detection And Sentiment Analysis Using Machine Learning And Deep Learning With Python Gui
DOWNLOAD
Author : Vivian Siahaan
language : en
Publisher: BALIGE PUBLISHING
Release Date : 2023-08-04
Hate Speech Detection And Sentiment Analysis Using Machine Learning And Deep Learning With Python Gui written by Vivian Siahaan and has been published by BALIGE PUBLISHING this book supported file pdf, txt, epub, kindle and other format this book has been release on 2023-08-04 with Computers categories.
The purpose of this project is to develop a comprehensive Hate Speech Detection and Sentiment Analysis system using both Machine Learning and Deep Learning techniques. The project aims to create a robust and accurate system that can automatically identify hate speech in text data and perform sentiment analysis to determine the emotions and opinions expressed in the text. The project is designed to address the growing concern over the spread of hate speech and offensive content online. By implementing an automated detection system, it can help social media platforms, content moderators, and online communities to proactively identify and remove harmful content, fostering a safer and more inclusive online environment. Additionally, sentiment analysis plays a crucial role in understanding public opinions, customer feedback, and social media trends. By accurately predicting sentiment, businesses can make data-driven decisions, improve customer satisfaction, and gain valuable insights into consumer preferences. This project focuses on Hate Speech Detection and Sentiment Analysis using both Machine Learning and Deep Learning techniques. It begins with exploring the dataset, analyzing feature distributions, and predicting sentiment using Machine Learning models like Logistic Regression, Support Vector Machines, K-Nearest Neighbors, Decision Trees, Random Forests, Gradient Boosting, Extreme Gradient Boosting, Light Gradient Boosting, and AdaBoost, while optimizing their performance through Grid Search for hyperparameter tuning. Subsequently, Deep Learning LSTM and 1D CNN models are implemented for sentiment analysis to capture long-term dependencies and local patterns in the text data. The project starts with exploring the dataset, understanding its structure, and analyzing the distribution of classes for hate speech and sentiment labels. This initial step allows us to gain insights into the dataset and potential challenges. After exploring the data, the distribution of text features, such as word frequency and sentiment scores, is analyzed to identify any patterns or biases that could impact the model's performance. The dataset is then divided into training, validation, and testing sets to evaluate the models' generalization capabilities. Early stopping techniques are utilized during training to prevent overfitting and enhance model generalization. Performance evaluation involves calculating metrics like accuracy, precision, recall, and F1-score to gauge the models' effectiveness. Confusion matrices and visualizations provide further insights into model predictions and potential areas for improvement. A graphical user interface (GUI) is developed using PyQt to facilitate user interaction with the Hate Speech Detection and Sentiment Analysis system. Before training the Deep Learning models, the text data is tokenized and padded for uniform input sequences. The dataset is split into training and validation sets for model evaluation, and early stopping is used to prevent overfitting during training. The final system combines predictions from both Machine Learning and Deep Learning models to provide robust sentiment analysis results. The PyQt GUI allows users to input text and receive real-time sentiment analysis predictions. The LSTM and 1D CNN models, along with their optimized hyperparameters, are saved and deployed for future sentiment analysis tasks. Users can interact with the GUI, analyze sentiment in different texts, and provide feedback for continuous improvement of the Hate Speech Detection and Sentiment Analysis system.
Automatic Cyberbullying Detection Emerging Research And Opportunities
DOWNLOAD
Author : Ptaszynski, Michal E.
language : en
Publisher: IGI Global
Release Date : 2018-11-02
Automatic Cyberbullying Detection Emerging Research And Opportunities written by Ptaszynski, Michal E. and has been published by IGI Global this book supported file pdf, txt, epub, kindle and other format this book has been release on 2018-11-02 with Family & Relationships categories.
Due to the prevalence of social network service and social media, the problem of cyberbullying has risen to the forefront as a major social issue over the last decade. Internet hate, harassment, cyberstalking, cyberbullying—these terms, which were almost unknown 10 years ago—are in the everyday lexicon of all internet users. Unfortunately, it is becoming increasingly difficult to undertake continuous surveillance of websites as new ones are appearing daily. Methods for automatic detection and mitigation for online bullying have become necessary in order to protect the online user experience. Automatic Cyberbullying Detection: Emerging Research and Opportunities provides innovative insights into online bullying and methods of early identification, mitigation, and prevention of harassing speech and activity. Explanations and reasoning for each of these applied methods are provided as well as their pros and cons when applied to the language of online bullying. Also included are some generalizations of cyberbullying as a phenomenon and how to approach the problem from a practical technology-backed point of view. The content within this publication represents the work of deep learning, language modeling, and web mining. It is designed for academicians, social media moderators, IT consultants, programmers, education administrators, researchers, and professionals and covers topics centered on identification methods and mitigation of internet hate and online harassment.
Combatting Cyberbullying In Digital Media With Artificial Intelligence
DOWNLOAD
Author : Mohamed Lahby
language : en
Publisher: CRC Press
Release Date : 2023-12-13
Combatting Cyberbullying In Digital Media With Artificial Intelligence written by Mohamed Lahby and has been published by CRC Press this book supported file pdf, txt, epub, kindle and other format this book has been release on 2023-12-13 with Computers categories.
Rapid advancements in mobile computing and communication technology and recent technological progress have opened up a plethora of opportunities. These advancements have expanded knowledge, facilitated global business, enhanced collaboration, and connected people through various digital media platforms. While these virtual platforms have provided new avenues for communication and self-expression, they also pose significant threats to our privacy. As a result, we must remain vigilant against the propagation of electronic violence through social networks. Cyberbullying has emerged as a particularly concerning form of online harassment and bullying, with instances of racism, terrorism, and various types of trolling becoming increasingly prevalent worldwide. Addressing the issue of cyberbullying to find effective solutions is a challenge for the web mining community, particularly within the realm of social media. In this context, artificial intelligence (AI) can serve as a valuable tool in combating the diverse manifestations of cyberbullying on the Internet and social networks. This book presents the latest cutting-edge research, theoretical methods, and novel applications in AI techniques to combat cyberbullying. Discussing new models, practical solutions, and technological advances related to detecting and analyzing cyberbullying is based on AI models and other related techniques. Furthermore, the book helps readers understand AI techniques to combat cyberbullying systematically and forthrightly, as well as future insights and the societal and technical aspects of natural language processing (NLP)-based cyberbullying research efforts. Key Features: Proposes new models, practical solutions and technological advances related to machine intelligence techniques for detecting cyberbullying across multiple social media platforms. Combines both theory and practice so that readers (beginners or experts) of this book can find both a description of the concepts and context related to the machine intelligence. Includes many case studies and applications of machine intelligence for combating cyberbullying.
Kids Cybersecurity Using Computational Intelligence Techniques
DOWNLOAD
Author : Wael M. S. Yafooz
language : en
Publisher: Springer Nature
Release Date : 2023-02-20
Kids Cybersecurity Using Computational Intelligence Techniques written by Wael M. S. Yafooz and has been published by Springer Nature this book supported file pdf, txt, epub, kindle and other format this book has been release on 2023-02-20 with Technology & Engineering categories.
This book introduces and presents the newest up-to-date methods, approaches and technologies on how to detect child cyberbullying on social media as well as monitor kids E-learning, monitor games designed and social media activities for kids. On a daily basis, children are exposed to harmful content online. There have been many attempts to resolve this issue by conducting methods based on rating and ranking as well as reviewing comments to show the relevancy of these videos to children; unfortunately, there still remains a lack of supervision on videos dedicated to kids. This book also introduces a new algorithm for content analysis against harmful information for kids. Furthermore, it establishes the goal to track useful information of kids and institutes detection of kid’s textual aggression through methods of machine and deep learning and natural language processing for a safer space for children on social media and online and to combat problems, such as lack of supervision, cyberbullying, kid’s exposure to harmful content. This book is beneficial to postgraduate students and researchers' concerns on recent methods and approaches to kids' cybersecurity.
Malware Analysis Using Artificial Intelligence And Deep Learning
DOWNLOAD
Author : Mark Stamp
language : en
Publisher: Springer Nature
Release Date : 2020-12-20
Malware Analysis Using Artificial Intelligence And Deep Learning written by Mark Stamp and has been published by Springer Nature this book supported file pdf, txt, epub, kindle and other format this book has been release on 2020-12-20 with Computers categories.
This book is focused on the use of deep learning (DL) and artificial intelligence (AI) as tools to advance the fields of malware detection and analysis. The individual chapters of the book deal with a wide variety of state-of-the-art AI and DL techniques, which are applied to a number of challenging malware-related problems. DL and AI based approaches to malware detection and analysis are largely data driven and hence minimal expert domain knowledge of malware is needed. This book fills a gap between the emerging fields of DL/AI and malware analysis. It covers a broad range of modern and practical DL and AI techniques, including frameworks and development tools enabling the audience to innovate with cutting-edge research advancements in a multitude of malware (and closely related) use cases.
Neural Network Methods For Natural Language Processing
DOWNLOAD
Author : Yoav Goldberg
language : en
Publisher: Springer Nature
Release Date : 2022-06-01
Neural Network Methods For Natural Language Processing written by Yoav Goldberg and has been published by Springer Nature this book supported file pdf, txt, epub, kindle and other format this book has been release on 2022-06-01 with Computers categories.
Neural networks are a family of powerful machine learning models. This book focuses on the application of neural network models to natural language data. The first half of the book (Parts I and II) covers the basics of supervised machine learning and feed-forward neural networks, the basics of working with machine learning over language data, and the use of vector-based rather than symbolic representations for words. It also covers the computation-graph abstraction, which allows to easily define and train arbitrary neural networks, and is the basis behind the design of contemporary neural network software libraries. The second part of the book (Parts III and IV) introduces more specialized neural network architectures, including 1D convolutional neural networks, recurrent neural networks, conditioned-generation models, and attention-based models. These architectures and techniques are the driving force behind state-of-the-art algorithms for machine translation, syntactic parsing, and many other applications. Finally, we also discuss tree-shaped networks, structured prediction, and the prospects of multi-task learning.
2018 International Conference On Computational Science And Computational Intelligence Csci
DOWNLOAD
Author : IEEE Staff
language : en
Publisher:
Release Date : 2018-12-12
2018 International Conference On Computational Science And Computational Intelligence Csci written by IEEE Staff and has been published by this book supported file pdf, txt, epub, kindle and other format this book has been release on 2018-12-12 with categories.
Based on IEEE taxonomy (FOI list), CSCI is directly related to many of IEEE Computer Society s fields of interest (BUT note that in this conference we DO NOT plan to consider topics that are theoretical in nature such as automatic proof based systems, solutions to open problems in mathematics, ) Using IEEE classifications taxonomy, please find below a representative list of fields of interest for the conference (which maps nicely with IEEE FOI) (Separated with semi columns) Essentially, we are interested in all aspects of computational science and computational intelligence and applications products B Hardware (B 2 Arithmetic and Logic Structures, B 2 1 b Parallel, B 2 4 a Algorithms, B 4 1 Data Communications Devices, B 4 3 h Wireless systems, B 7 1 f Microprocessors and microcomputers, B 7 1 g Network connectivity chips) C Computer Systems Organization (C 1 2 g Parallel processors, C 1 4 Parallel Architectures, C 1 4 a Distributed architectures, C 1 4 b Mobile processors
Cognitive Informatics And Soft Computing
DOWNLOAD
Author : Pradeep Kumar Mallick
language : en
Publisher: Springer Nature
Release Date : 2020-01-14
Cognitive Informatics And Soft Computing written by Pradeep Kumar Mallick and has been published by Springer Nature this book supported file pdf, txt, epub, kindle and other format this book has been release on 2020-01-14 with Technology & Engineering categories.
The book presents new approaches and methods for solving real-world problems. It highlights, in particular, innovative research in the fields of Cognitive Informatics, Cognitive Computing, Computational Intelligence, Advanced Computing, and Hybrid Intelligent Models and Applications. New algorithms and methods in a variety of fields are presented, together with solution-based approaches. The topics addressed include various theoretical aspects and applications of Computer Science, Artificial Intelligence, Cybernetics, Automation Control Theory, and Software Engineering.