Discrepancy Based Model Selection Criteria Using Cross Validation
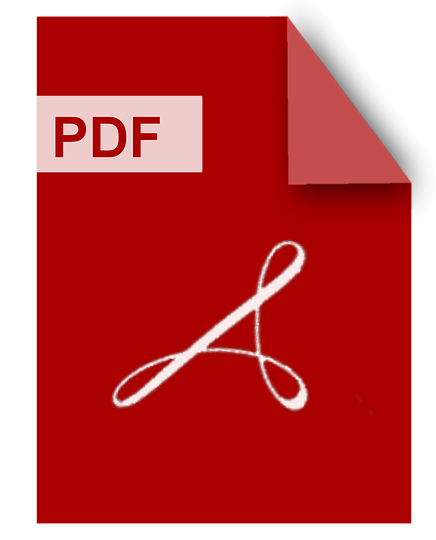
DOWNLOAD
Download Discrepancy Based Model Selection Criteria Using Cross Validation PDF/ePub or read online books in Mobi eBooks. Click Download or Read Online button to get Discrepancy Based Model Selection Criteria Using Cross Validation book now. This website allows unlimited access to, at the time of writing, more than 1.5 million titles, including hundreds of thousands of titles in various foreign languages. If the content not found or just blank you must refresh this page
Discrepancy Based Model Selection Criteria Using Cross Validation
DOWNLOAD
Author : Simon Lee Davies
language : en
Publisher:
Release Date : 2002
Discrepancy Based Model Selection Criteria Using Cross Validation written by Simon Lee Davies and has been published by this book supported file pdf, txt, epub, kindle and other format this book has been release on 2002 with Linear models (Statistics) categories.
An important component of any linear modeling problem consists of determining an appropriate size and form of the design matrix. Improper specification may substantially impact both estimators of the model parameters and predictors of the response variable: underspecification may lead to results which are severely biased, whereas overspecification may lead to results with unnecessarily high variability. Model selection criteria provide a powerful and useful tool for choosing a suitable design matrix. Once a setting has been proposed for an experiment, data can be collected, leading to a set of competing candidate models. One may then attempt to select an appropriate model from this set using a model selection criterion. In this thesis we establish four frameworks which initialize with previously proposed model selection criteria targeting well-known traditional discrepancies, namely the Kullback-Leibler discrepancy, the Gauss discrepancy, the transformed Gauss discrepancy, and the Kullback symmetric discrepancy. These criteria are developed using the bias adjustment approach. Prior work has focused on finding approximately or exactly unbiased estimators of these discrepancies. We expand on this work to additionally show that the criteria which are exactly unbiased serve as the minimum variance unbiased estimators. In many situations, the predictive ability of a candidate model is its most important attribute. In light of our interest in this property, we also concentrate on model selection techniques based on cross validation. New cross validation model selection criteria that serve as counterparts to the standard bias adjusted forms are introduced, together with descriptions of the target discrepancies upon which they are based. We then develop model selection criteria which are minimum variance unbiased estimators of the cross validation discrepancies. Furthermore, we argue that these criteria serve as approximate minimum variance unbiased estimators of the corresponding traditional discrepancies. We propose a general framework to unify and elucidate part of our cross validation criterion development. We show that for the cross validation analogue of a traditional discrepancy, we can always find a "natural" criterion which serves as an exactly unbiased estimator. We study how the cross validation criteria compare to the standard bias adjusted criteria as selection rules in the linear regression framework. This is done by concluding our development of each of the four frameworks with simulation results which illustrate how frequently each criterion identifies the correctly specified model among a sequence of nested fitted candidate models. Our results indicate that the cross validation criteria tend to outperform their bias adjusted counterparts. We close by evaluating the performance of all the model selection criteria considered throughout our work by investigating the results of a simulation study compiled using a sample of data from the Missouri Trauma Registry.
Statistical Models And Methods For Biomedical And Technical Systems
DOWNLOAD
Author : Filia Vonta
language : en
Publisher: Springer Science & Business Media
Release Date : 2008-03-05
Statistical Models And Methods For Biomedical And Technical Systems written by Filia Vonta and has been published by Springer Science & Business Media this book supported file pdf, txt, epub, kindle and other format this book has been release on 2008-03-05 with Medical categories.
This book deals with the mathematical aspects of survival analysis and reliability as well as other topics, reflecting recent developments in the following areas: applications in epidemiology; probabilistic and statistical models and methods in reliability; models and methods in survival analysis, longevity, aging, and degradation; accelerated life models; quality of life; new statistical challenges in genomics. The work will be useful to a broad interdisciplinary readership of researchers and practitioners in applied probability and statistics, industrial statistics, biomedicine, biostatistics, and engineering.
Model Selection
DOWNLOAD
Author : Parhasarathi Lahiri
language : en
Publisher: IMS
Release Date : 2001
Model Selection written by Parhasarathi Lahiri and has been published by IMS this book supported file pdf, txt, epub, kindle and other format this book has been release on 2001 with Mathematics categories.
Handbook Of Structural Equation Modeling
DOWNLOAD
Author : Rick H. Hoyle
language : en
Publisher: Guilford Publications
Release Date : 2023-02-17
Handbook Of Structural Equation Modeling written by Rick H. Hoyle and has been published by Guilford Publications this book supported file pdf, txt, epub, kindle and other format this book has been release on 2023-02-17 with Business & Economics categories.
"This accessible volume presents both the mechanics of structural equation modeling (SEM) and specific SEM strategies and applications. The editor, along with an international group of contributors, and editorial advisory board are leading methodologists who have organized the book to move from simpler material to more statistically complex modeling approaches. Sections cover the foundations of SEM; statistical underpinnings, from assumptions to model modifications; steps in implementation, from data preparation through writing the SEM report; and basic and advanced applications, including new and emerging topics in SEM. Each chapter provides conceptually oriented descriptions, fully explicated analyses, and engaging examples that reveal modeling possibilities for use with readers' data. Many of the chapters also include access to data and syntax files at the companion website, allowing readers to try their hands at reproducing the authors' results"--
Generalized Latent Variable Modeling
DOWNLOAD
Author : Anders Skrondal
language : en
Publisher: CRC Press
Release Date : 2004-05-11
Generalized Latent Variable Modeling written by Anders Skrondal and has been published by CRC Press this book supported file pdf, txt, epub, kindle and other format this book has been release on 2004-05-11 with Mathematics categories.
This book unifies and extends latent variable models, including multilevel or generalized linear mixed models, longitudinal or panel models, item response or factor models, latent class or finite mixture models, and structural equation models. Following a gentle introduction to latent variable modeling, the authors clearly explain and contrast a wide range of estimation and prediction methods from biostatistics, psychometrics, econometrics, and statistics. They present exciting and realistic applications that demonstrate how researchers can use latent variable modeling to solve concrete problems in areas as diverse as medicine, economics, and psychology. The examples considered include many nonstandard response types, such as ordinal, nominal, count, and survival data. Joint modeling of mixed responses, such as survival and longitudinal data, is also illustrated. Numerous displays, figures, and graphs make the text vivid and easy to read. About the authors: Anders Skrondal is Professor and Chair in Social Statistics, Department of Statistics, London School of Economics, UK Sophia Rabe-Hesketh is a Professor of Educational Statistics at the Graduate School of Education and Graduate Group in Biostatistics, University of California, Berkeley, USA.
Model Selection Criteria Based On Discrepancy Functions
DOWNLOAD
Author : Julie A. Kleine
language : en
Publisher:
Release Date : 1998
Model Selection Criteria Based On Discrepancy Functions written by Julie A. Kleine and has been published by this book supported file pdf, txt, epub, kindle and other format this book has been release on 1998 with Linear models (Statistics) categories.
Regression And Time Series Model Selection
DOWNLOAD
Author : Allan D. R. McQuarrie
language : en
Publisher: World Scientific
Release Date : 1998
Regression And Time Series Model Selection written by Allan D. R. McQuarrie and has been published by World Scientific this book supported file pdf, txt, epub, kindle and other format this book has been release on 1998 with Mathematics categories.
This important book describes procedures for selecting a model from a large set of competing statistical models. It includes model selection techniques for univariate and multivariate regression models, univariate and multivariate autoregressive models, nonparametric (including wavelets) and semiparametric regression models, and quasi-likelihood and robust regression models. Information-based model selection criteria are discussed, and small sample and asymptotic properties are presented. The book also provides examples and large scale simulation studies comparing the performances of information-based model selection criteria, bootstrapping, and cross-validation selection methods over a wide range of models.
Discrepancy Based Algorithms For Best Subset Model Selection
DOWNLOAD
Author : Tao Zhang
language : en
Publisher:
Release Date : 2013
Discrepancy Based Algorithms For Best Subset Model Selection written by Tao Zhang and has been published by this book supported file pdf, txt, epub, kindle and other format this book has been release on 2013 with Akaike Information Criterion categories.
The selection of a best-subset regression model from a candidate family is a common problem that arises in many analyses. In best-subset model selection, we consider all possible subsets of regressor variables; thus, numerous candidate models may need to be fit and compared. One of the main challenges of best-subset selection arises from the size of the candidate model family: specifically, the probability of selecting an inappropriate model generally increases as the size of the family increases. For this reason, it is usually difficult to select an optimal model when best-subset selection is attempted based on a moderate to large number of regressor variables. Model selection criteria are often constructed to estimate discrepancy measures used to assess the disparity between each fitted candidate model and the generating model. The Akaike information criterion (AIC) and the corrected AIC (AICc) are designed to estimate the expected Kullback-Leibler (K-L) discrepancy. For best-subset selection, both AIC and AICc are negatively biased, and the use of either criterion will lead to overfitted models. To correct for this bias, we introduce a criterion AICi, which has a penalty term evaluated from Monte Carlo simulation. A multistage model selection procedure AICaps, which utilizes AICi, is proposed for best-subset selection. In the framework of linear regression models, the Gauss discrepancy is another frequently applied measure of proximity between a fitted candidate model and the generating model. Mallows' conceptual predictive statistic (Cp) and the modified Cp (MCp) are designed to estimate the expected Gauss discrepancy. For best-subset selection, Cp and MCp exhibit negative estimation bias. To correct for this bias, we propose a criterion CPSi that again employs a penalty term evaluated from Monte Carlo simulation. We further devise a multistage procedure, CPSaps, which selectively utilizes CPSi. In this thesis, we consider best-subset selection in two different modeling frameworks: linear models and generalized linear models. Extensive simulation studies are compiled to compare the selection behavior of our methods and other traditional model selection criteria. We also apply our methods to a model selection problem in a study of bipolar disorder.
Technometrics
DOWNLOAD
Author :
language : en
Publisher:
Release Date : 2000
Technometrics written by and has been published by this book supported file pdf, txt, epub, kindle and other format this book has been release on 2000 with Experimental design categories.
Handbook Of Financial Time Series
DOWNLOAD
Author : Torben Gustav Andersen
language : en
Publisher: Springer Science & Business Media
Release Date : 2009-04-21
Handbook Of Financial Time Series written by Torben Gustav Andersen and has been published by Springer Science & Business Media this book supported file pdf, txt, epub, kindle and other format this book has been release on 2009-04-21 with Business & Economics categories.
The Handbook of Financial Time Series gives an up-to-date overview of the field and covers all relevant topics both from a statistical and an econometrical point of view. There are many fine contributions, and a preamble by Nobel Prize winner Robert F. Engle.