Essays In The Application Of Machine Learning In Development Economics
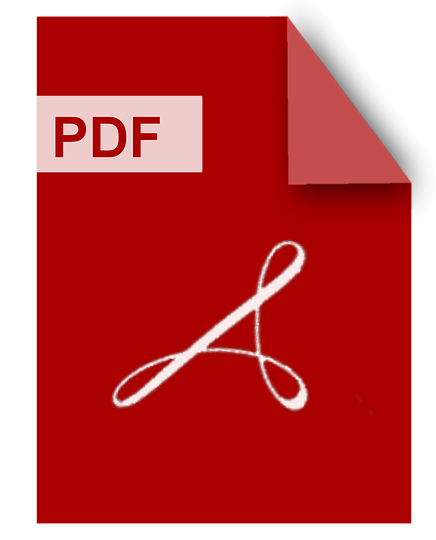
DOWNLOAD
Download Essays In The Application Of Machine Learning In Development Economics PDF/ePub or read online books in Mobi eBooks. Click Download or Read Online button to get Essays In The Application Of Machine Learning In Development Economics book now. This website allows unlimited access to, at the time of writing, more than 1.5 million titles, including hundreds of thousands of titles in various foreign languages. If the content not found or just blank you must refresh this page
Essays In The Application Of Machine Learning In Development Economics
DOWNLOAD
Author : Dweepobotee Brahma
language : en
Publisher:
Release Date : 2019
Essays In The Application Of Machine Learning In Development Economics written by Dweepobotee Brahma and has been published by this book supported file pdf, txt, epub, kindle and other format this book has been release on 2019 with Child health insurance categories.
This dissertation comprises four essays which apply Machine Learning (ML) techniques to examine India's progress towards meeting several child-health targets under United Nation's "Sustainable Development Goals (SDG) 2030". The application of novel ML techniques unmasks certain detailed empirical aspects of the road to meeting specific indicators of SDG - child mortality, malnutrition, immunization coverage and health-expenses. The first essay employs multiple parametric and non-parametric Machine Learning (ML) techniques (LASSO, Classification Random Forest, Boosted Logistic Regression, Boosted Classification Trees) to build predictive models for the incidences of neonatal and infant mortality. A large national level household survey dataset is used. All the ML techniques display higher prediction accuracy compared to a standard logistic regression. The consensus from the ML techniques is used to identify a 'high-mortality risk' group of mothers and infants who can be the potential beneficiaries of 'targeted' public health policies in future. The second essay investigates multifaceted nature of infant malnutrition in India. A large comprehensive set of covariates from a survey are considered leading to a near high-dimensional setting (with the number of regressors coming closer to the sample size) which necessitates the use of a sparsity-based ML technique. LASSO - a variable selection technique is used to select predictors with strong association with malnutrition and subsequently a post-selection inference (PoSI) technique is applied to conduct hypothesis testing on the selected predictors. The results indicate that while safe drinking water is important in curbing infant malnutrition, many existing government policies are ineffective. Using state-level data the third essay compares performances of the Indian states in achieving coverage of five essential child vaccines (BCG, DPT, Measles, Polio, Tetanus) under the 'Universal Immunization Program (UIP)'. The roles of the two policy pillars - (a) funds disbursed by the Central Government to the State Governments under UIP, and (b) the required health infrastructure in each state, are evaluated through the lens of both inference and prediction. Traditional panel regression techniques identify a complementarity between funds and infrastructure. While digging deeper into the questions of complementarity in the aforementioned covariates in various states as well as identifying under-performing states, a comprehensive set of interactions are considered, leading to a near high-dimensional setting. Sparsity-based ML technique (LASSO) is therefore used for variable selection. The results identify certain under-performing states where the policy pillars need to be strengthened. The fourth essay focuses on three leading causes of morbidity in infants, namely prematurity, jaundice and cardio-respiratory ailments. The role of government sponsored health insurance schemes in mitigating out-of-pocket and overall medical expenses are evaluated using a nationwide survey. A newly developed ML method (Double/de-biased LASSO) is used, which enables us to (a) select important predictors of health expenditure from a vast set of covariates and (b) estimate the 'treatment effect' of health insurance on health expenses. While health insurance schemes are found to be effective in mitigating health expenses due to premature birth, no such evidence is found for jaundice and cardio-respiratory diseases in infants.
Three Essays On The Application Of Machine Learning Methods In Economics
DOWNLOAD
Author : Abdelaziz Lawani
language : en
Publisher:
Release Date : 2018
Three Essays On The Application Of Machine Learning Methods In Economics written by Abdelaziz Lawani and has been published by this book supported file pdf, txt, epub, kindle and other format this book has been release on 2018 with categories.
Essays On Applied Economics With Machine Learning Approach
DOWNLOAD
Author : Tzai-Shuen Chen
language : en
Publisher:
Release Date : 2018
Essays On Applied Economics With Machine Learning Approach written by Tzai-Shuen Chen and has been published by this book supported file pdf, txt, epub, kindle and other format this book has been release on 2018 with Electronic dissertations categories.
This dissertation concentrates on applying machine learning methods to economic policy analysis. When talking about using machine learning or other non-behavioral model to conduct policy analysis, the first question raised by economists is the Lucas critique. A policy intervention would affect the incentive that people face and thus changes the underlying decision-making problem. A predictive model without the component of optimizing behavior might not capture people's reactions to the policy intervention to give a reliable prediction. Even if the quantitative effect of the Lucas critique is not significant, the machine learning method might have no advantage over a well-performed standard econometric model in terms of prediction or time efficiency. The first chapter presents an out-of-sample prediction comparison between major machine learning models and the structural econometric model. To evaluate the benefits of this approach, I use the most common machine learning algorithms, CART, C4.5, LASSO, random forest, and adaboost, to construct prediction models for a cash transfer experiment conducted by the Progresa program in Mexico, and I compare the prediction results with those of a previous structural econometric study. Two prediction tasks are performed in this paper: the out-of-sample forecast and the long-term within-sample simulation. For the out-of-sample forecast, both the mean absolute error and the root mean square error of the school attendance rates found by all machine learning models are smaller than those found by the structural model. Random forest and adaboost have the highest accuracy for the individual outcomes of all subgroups. For the long-term within-sample simulation, the structural model has better performance than do all of the machine learning models. The poor within-sample fitness of the machine learning model results from the inaccuracy of the income and pregnancy prediction models. The result shows that the machine learning model performs better than does the structural model when there are many data to learn; however, when the data are limited, the structural model offers a more sensible prediction. In addition to prediction outcome, machine learning models are more time-efficient than the structural model. The most complicated model, random forest, takes less than half an hour to build and less than one minute to predict. The findings show promise for adopting machine learning in economic policy analyses in the era of big data. The second chapter exploits the predictive power of machine learning algorithms to conduct covariate adjustment for estimating average treatment effects and the log-odds ratio. Previous semi-parametric approaches have proven that baseline covariate adjustment can increase the estimator efficiency and statistical power, compared to an unadjusted estimator. I use random forest model to select predictive covariates and conduct a Monte Carlo simulation to compare the efficiency and statistical power of unadjusted, OLS-based, and random-forest-based approaches in different parameter settings. The simulation result indicates that the random-forest-based estimator is more efficient and has higher statistical power than the other two methods. In addition, I apply this approach to the Zomba Cash Transfer Experiment in Malawi to study the difference in policy effect between conditional and unconditional cash transfers. The third chapter investigates the possibility of using machine learning models to conduct the counterfactual analysis for conditional policies. Conditional Cash Transfer has become a popular tool to alleviate intergenerational poverty in many developing countries due to the success of the Progresa program in Mexico. There are some experiments focused on the implementation details to explore the efficient practice of the policy implementation. The policy analysis, however, still heavily relies on the counterfactual prediction because of the budget and time constraints. Recently, machine learning has been proved successful in many prediction applications. Adopting machine learning model into economic policy analysis might help to increase the prediction performance and hence offer another approach of counterfactual analysis. While it is straightforward to apply machine learning algorithms to conduct counterfactual prediction for the unconditional policy, there is no direct prediction for the conditional policy due to the lack of behavioral description. This chapter uses the Zomba Cash Transfer Experiment in Malawi to examine the error of using an unconditional machine learning approach to prediction the outcome of the conditional policy. The result shows that the error from the conditional-unconditional difference is a minor source of prediction errors, which provides support of exploiting the predictive power of machine learning algorithms to offer policy suggestions for the conditional policy.
Essays On Information Technology Intangible Capital And The Economics Of Artificial Intelligence
DOWNLOAD
Author : Daniel Ian Rock
language : en
Publisher:
Release Date : 2019
Essays On Information Technology Intangible Capital And The Economics Of Artificial Intelligence written by Daniel Ian Rock and has been published by this book supported file pdf, txt, epub, kindle and other format this book has been release on 2019 with categories.
This dissertation contains four essays concerning the economics of information technology, intangible capital, and artificial intelligence. In the first essay, "Engineering Value: The Returns to Technological Talent and Investments in Artificial Intelligence" I describe how firms can appropriate some of the value of their employees' human capital by assigning firm-specific tasks. I then use a database of employment records to document dynamics in the valuation of publicly traded firms as they relate to different types of employment, focusing especially on AI skills. The second essay, "The Productivity J-Curve: How Intangibles Complement General Purpose Technologies" (coauthored with Erik Brynjolfsson and Chad Syverson) addresses the concern that new technologies with wide applicability throughout the economy can cause both underestimation and overestimation of total factor productivity. As capital is accumulated, intangible investment output, and therefore productivity growth, will be underestimated only to later generate a yield (at which point productivity growth will be overestimated). Presenting a theoretical description of how to use corporate valuations to recover hidden investment value, we discuss how productivity growth and levels can be adjusted to accommodate these changes. Implications for research and development, computer hardware, and computer software investments are considered. The third essay, "Machine Learning and Occupational Change" (coauthored with Erik Brynjolfsson and Tom Mitchell), develops and implements a method to measure the labor market impact potential of machine learning technologies. Tasks are evaluated for their Suitability for Machine Learning (SML). We find that few occupations can be fully automated with machine learning, but many occupations will potentially be redesigned. The final essay, "Do Labor Demand Shifts Occur Within Firms or Across Them? Non-Routine-Biased Technological Change 2000-2016" (coauthored with Seth Benzell and Guillermo Lagarda) decomposes labor share shifts of occupational groups into changes between firms, within firms, and due to entry and exit. We find that within-firm compositional shifts are an important component of changes in the overall labor market. We also find that the rate of within-firm shifts has declined in the period from 2000 to 2016. Together, these essays offer insights into how artificial intelligence technologies, particularly machine learning, will impact the U.S. economy.
Essays In Development Economics
DOWNLOAD
Author : Martin Wiegand
language : en
Publisher:
Release Date : 2021
Essays In Development Economics written by Martin Wiegand and has been published by this book supported file pdf, txt, epub, kindle and other format this book has been release on 2021 with categories.
Essays In Applied Machine Learning And Economics
DOWNLOAD
Author : Garima Singal
language : en
Publisher:
Release Date : 2022
Essays In Applied Machine Learning And Economics written by Garima Singal and has been published by this book supported file pdf, txt, epub, kindle and other format this book has been release on 2022 with categories.
In this dissertation, we contribute to two strands of economic literature- applied economics and game theory. The contribution to applied economics is developing a prediction model for PM2.5, a key indicator of air pollution. The PM2.5 predictions from this model enable an analysis of economic and environmental policies that were previously infeasible due to a lack of PM2.5 measurements, especially in developing regions. We also demonstrate the unsuitability, for Delhi specifically, of the predominant benchmark estimates for PM2.5, in the applied economics literature supplied by van Donkelaar et al. Additionally, we were able to introduce and demonstrably improve upon a frontier technique from the deep learning literature to the applied economics literature. The contribution to the game theory literature takes the form of assessing the optimality of contests as a mechanism in the context of a standard Myersonian mechanism design environment, a previously unexplored setting. We find that despite extensive usage of contests as a mechanism in the real world, it is not without loss in revenue to use optimal contests. This dissertation's primary contribution is developing a modeling pipeline with lower data requirements and better predictive performance than the existing state-of-the-art estimates in the applied economics literature.
Essays In Economics And Machine Learning
DOWNLOAD
Author : Friedrich Christian Geiecke
language : en
Publisher:
Release Date : 2019
Essays In Economics And Machine Learning written by Friedrich Christian Geiecke and has been published by this book supported file pdf, txt, epub, kindle and other format this book has been release on 2019 with categories.
Essays On Probabilistic Machine Learning For Economics
DOWNLOAD
Author : Nikolas Kuhlen
language : en
Publisher:
Release Date : 2021
Essays On Probabilistic Machine Learning For Economics written by Nikolas Kuhlen and has been published by this book supported file pdf, txt, epub, kindle and other format this book has been release on 2021 with categories.
Essays In Machine Learning Applications For Asset Pricing
DOWNLOAD
Author : Yavor Kovachev
language : en
Publisher:
Release Date : 2021
Essays In Machine Learning Applications For Asset Pricing written by Yavor Kovachev and has been published by this book supported file pdf, txt, epub, kindle and other format this book has been release on 2021 with categories.
Machine Learning And Causality The Impact Of Financial Crises On Growth
DOWNLOAD
Author : Mr.Andrew J Tiffin
language : en
Publisher: International Monetary Fund
Release Date : 2019-11-01
Machine Learning And Causality The Impact Of Financial Crises On Growth written by Mr.Andrew J Tiffin and has been published by International Monetary Fund this book supported file pdf, txt, epub, kindle and other format this book has been release on 2019-11-01 with Computers categories.
Machine learning tools are well known for their success in prediction. But prediction is not causation, and causal discovery is at the core of most questions concerning economic policy. Recently, however, the literature has focused more on issues of causality. This paper gently introduces some leading work in this area, using a concrete example—assessing the impact of a hypothetical banking crisis on a country’s growth. By enabling consideration of a rich set of potential nonlinearities, and by allowing individually-tailored policy assessments, machine learning can provide an invaluable complement to the skill set of economists within the Fund and beyond.