Three Essays On The Application Of Machine Learning Methods In Economics
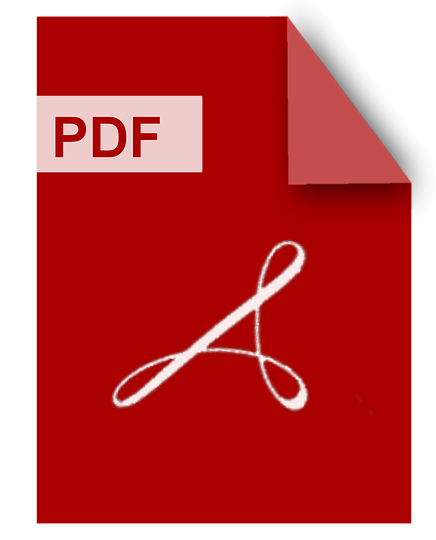
DOWNLOAD
Download Three Essays On The Application Of Machine Learning Methods In Economics PDF/ePub or read online books in Mobi eBooks. Click Download or Read Online button to get Three Essays On The Application Of Machine Learning Methods In Economics book now. This website allows unlimited access to, at the time of writing, more than 1.5 million titles, including hundreds of thousands of titles in various foreign languages. If the content not found or just blank you must refresh this page
Three Essays On The Application Of Machine Learning Methods In Economics
DOWNLOAD
Author : Abdelaziz Lawani
language : en
Publisher:
Release Date : 2018
Three Essays On The Application Of Machine Learning Methods In Economics written by Abdelaziz Lawani and has been published by this book supported file pdf, txt, epub, kindle and other format this book has been release on 2018 with categories.
Essays In The Application Of Machine Learning In Development Economics
DOWNLOAD
Author : Dweepobotee Brahma
language : en
Publisher:
Release Date : 2019
Essays In The Application Of Machine Learning In Development Economics written by Dweepobotee Brahma and has been published by this book supported file pdf, txt, epub, kindle and other format this book has been release on 2019 with Child health insurance categories.
This dissertation comprises four essays which apply Machine Learning (ML) techniques to examine India's progress towards meeting several child-health targets under United Nation's "Sustainable Development Goals (SDG) 2030". The application of novel ML techniques unmasks certain detailed empirical aspects of the road to meeting specific indicators of SDG - child mortality, malnutrition, immunization coverage and health-expenses. The first essay employs multiple parametric and non-parametric Machine Learning (ML) techniques (LASSO, Classification Random Forest, Boosted Logistic Regression, Boosted Classification Trees) to build predictive models for the incidences of neonatal and infant mortality. A large national level household survey dataset is used. All the ML techniques display higher prediction accuracy compared to a standard logistic regression. The consensus from the ML techniques is used to identify a 'high-mortality risk' group of mothers and infants who can be the potential beneficiaries of 'targeted' public health policies in future. The second essay investigates multifaceted nature of infant malnutrition in India. A large comprehensive set of covariates from a survey are considered leading to a near high-dimensional setting (with the number of regressors coming closer to the sample size) which necessitates the use of a sparsity-based ML technique. LASSO - a variable selection technique is used to select predictors with strong association with malnutrition and subsequently a post-selection inference (PoSI) technique is applied to conduct hypothesis testing on the selected predictors. The results indicate that while safe drinking water is important in curbing infant malnutrition, many existing government policies are ineffective. Using state-level data the third essay compares performances of the Indian states in achieving coverage of five essential child vaccines (BCG, DPT, Measles, Polio, Tetanus) under the 'Universal Immunization Program (UIP)'. The roles of the two policy pillars - (a) funds disbursed by the Central Government to the State Governments under UIP, and (b) the required health infrastructure in each state, are evaluated through the lens of both inference and prediction. Traditional panel regression techniques identify a complementarity between funds and infrastructure. While digging deeper into the questions of complementarity in the aforementioned covariates in various states as well as identifying under-performing states, a comprehensive set of interactions are considered, leading to a near high-dimensional setting. Sparsity-based ML technique (LASSO) is therefore used for variable selection. The results identify certain under-performing states where the policy pillars need to be strengthened. The fourth essay focuses on three leading causes of morbidity in infants, namely prematurity, jaundice and cardio-respiratory ailments. The role of government sponsored health insurance schemes in mitigating out-of-pocket and overall medical expenses are evaluated using a nationwide survey. A newly developed ML method (Double/de-biased LASSO) is used, which enables us to (a) select important predictors of health expenditure from a vast set of covariates and (b) estimate the 'treatment effect' of health insurance on health expenses. While health insurance schemes are found to be effective in mitigating health expenses due to premature birth, no such evidence is found for jaundice and cardio-respiratory diseases in infants.
Essays On Applied Economics With Machine Learning Approach
DOWNLOAD
Author : Tzai-Shuen Chen
language : en
Publisher:
Release Date : 2018
Essays On Applied Economics With Machine Learning Approach written by Tzai-Shuen Chen and has been published by this book supported file pdf, txt, epub, kindle and other format this book has been release on 2018 with Electronic dissertations categories.
This dissertation concentrates on applying machine learning methods to economic policy analysis. When talking about using machine learning or other non-behavioral model to conduct policy analysis, the first question raised by economists is the Lucas critique. A policy intervention would affect the incentive that people face and thus changes the underlying decision-making problem. A predictive model without the component of optimizing behavior might not capture people's reactions to the policy intervention to give a reliable prediction. Even if the quantitative effect of the Lucas critique is not significant, the machine learning method might have no advantage over a well-performed standard econometric model in terms of prediction or time efficiency. The first chapter presents an out-of-sample prediction comparison between major machine learning models and the structural econometric model. To evaluate the benefits of this approach, I use the most common machine learning algorithms, CART, C4.5, LASSO, random forest, and adaboost, to construct prediction models for a cash transfer experiment conducted by the Progresa program in Mexico, and I compare the prediction results with those of a previous structural econometric study. Two prediction tasks are performed in this paper: the out-of-sample forecast and the long-term within-sample simulation. For the out-of-sample forecast, both the mean absolute error and the root mean square error of the school attendance rates found by all machine learning models are smaller than those found by the structural model. Random forest and adaboost have the highest accuracy for the individual outcomes of all subgroups. For the long-term within-sample simulation, the structural model has better performance than do all of the machine learning models. The poor within-sample fitness of the machine learning model results from the inaccuracy of the income and pregnancy prediction models. The result shows that the machine learning model performs better than does the structural model when there are many data to learn; however, when the data are limited, the structural model offers a more sensible prediction. In addition to prediction outcome, machine learning models are more time-efficient than the structural model. The most complicated model, random forest, takes less than half an hour to build and less than one minute to predict. The findings show promise for adopting machine learning in economic policy analyses in the era of big data. The second chapter exploits the predictive power of machine learning algorithms to conduct covariate adjustment for estimating average treatment effects and the log-odds ratio. Previous semi-parametric approaches have proven that baseline covariate adjustment can increase the estimator efficiency and statistical power, compared to an unadjusted estimator. I use random forest model to select predictive covariates and conduct a Monte Carlo simulation to compare the efficiency and statistical power of unadjusted, OLS-based, and random-forest-based approaches in different parameter settings. The simulation result indicates that the random-forest-based estimator is more efficient and has higher statistical power than the other two methods. In addition, I apply this approach to the Zomba Cash Transfer Experiment in Malawi to study the difference in policy effect between conditional and unconditional cash transfers. The third chapter investigates the possibility of using machine learning models to conduct the counterfactual analysis for conditional policies. Conditional Cash Transfer has become a popular tool to alleviate intergenerational poverty in many developing countries due to the success of the Progresa program in Mexico. There are some experiments focused on the implementation details to explore the efficient practice of the policy implementation. The policy analysis, however, still heavily relies on the counterfactual prediction because of the budget and time constraints. Recently, machine learning has been proved successful in many prediction applications. Adopting machine learning model into economic policy analysis might help to increase the prediction performance and hence offer another approach of counterfactual analysis. While it is straightforward to apply machine learning algorithms to conduct counterfactual prediction for the unconditional policy, there is no direct prediction for the conditional policy due to the lack of behavioral description. This chapter uses the Zomba Cash Transfer Experiment in Malawi to examine the error of using an unconditional machine learning approach to prediction the outcome of the conditional policy. The result shows that the error from the conditional-unconditional difference is a minor source of prediction errors, which provides support of exploiting the predictive power of machine learning algorithms to offer policy suggestions for the conditional policy.
Essays In Quantitative Macroeconomics
DOWNLOAD
Author : Hanno Kase
language : en
Publisher:
Release Date : 2021
Essays In Quantitative Macroeconomics written by Hanno Kase and has been published by this book supported file pdf, txt, epub, kindle and other format this book has been release on 2021 with Consumer credit categories.
This thesis consists of three essays in quantitative macroeconomics. In Chapter 1, joint with Leonardo Melosi and Matthias Rottner, we leverage recent developments in machine learning to develop methods to solve and estimate large and complex nonlinear macroeconomic models, e.g. HANK models. Our method relies on neural networks because of their appealing feature that even models with hundreds of state variables can be solved. While likelihood estimation requires the repeated solving of the model, something that is infeasible for highly complex models, we overcome this problem by exploiting the scalability of neural networks. Including the parameters of the model as quasi state variables in the neural network, we solve this extended neural network and apply it directly in the estimation. To show the potential of our approach, we estimate a quantitative HANK model that features nonlinearities on an individual (borrowing limit) and aggregate level (zero lower bound) using simulated data. The model also shows that there is an important economic interaction between the impact of the zero lower bound and the degree of household heterogeneity. Chapter 2 studies the impact of macroprudential limits on mortgage lending in a heterogeneous agent life-cycle model with incomplete markets, long-term mortgage, and default. The model is calibrated to German economy using Household Finance and Consumption Survey data. I consider the effects of four policy instruments: loan-to-value limit, debt-toincome limit, payment-to-income limit, and maximum maturity. I find that their effect on homeownership rate is fairly modest. Only the loan-to-value limit significantly reduces the homeownership rate among young households. At the same time, it has the largest positive welfare effect. Chapter 3 explores applications of the backpropagation algorithm on heterogeneous agent models. In addition, I clarify the connection between deep learning and dynamic structural models by showing how a standard value function iteration algorithm can be viewed as a recurrent convolutional neural network. As a result, many advances in the field of machine learning can carry over to economics. This in turn makes the solution and estimation of more complex models feasible.
Three Essays On The Application Of Machine Learning For Risk Governance In Financial Institutions
DOWNLOAD
Author : Abena Fosua Owusu
language : en
Publisher:
Release Date : 2020
Three Essays On The Application Of Machine Learning For Risk Governance In Financial Institutions written by Abena Fosua Owusu and has been published by this book supported file pdf, txt, epub, kindle and other format this book has been release on 2020 with categories.
Machine Learning Applications For Agricultural Economics
DOWNLOAD
Author : Kennedy Odongo
language : en
Publisher:
Release Date : 2023
Machine Learning Applications For Agricultural Economics written by Kennedy Odongo and has been published by this book supported file pdf, txt, epub, kindle and other format this book has been release on 2023 with Agriculture categories.
This dissertation utilizes machine learning to answer questions in agricultural economics in three related but independent essays. Machine learning and data science are increasingly being adopted in interdisciplinary work providing complimentary analytical methods and data tools for economics research. I use machine learning to investigate how COVID-19 and the resulting media coverage affected specialty crop markets dynamics and to develop insights into how attributes of a new apple variety can be utilized in an advertising campaign to derive demand.The first paper of my dissertation investigates how COVID-19 and related social and traditional media coverage affected shipping point prices of specialty crops. I use Twitter data to estimate how the prevalence COVID-19 topics affect crop demand. The results show that crops that are usually consumed as food away from home (FAFH) were the most affected by COVID-19 relative to crops usually consumed as food at home (FAH). The impact of the pandemic was heterogenous across specialty crops with crops whose usage is concentrated in FAFH settings experiencing a decrease in demand compared to crops used mostly in FAH settings.The second compares the performance of two time series forecasting techniques in the context of event studies. The event in this paper is the economy-wide COVID-19 shutdown. The results show that the prices in strawberry and apples markets were higher during the pandemic than they should have been. In comparing the two forecasting methods, the neural network outperforms ARIMA on error metrics such as the Mean Absolute Error.The third paper evaluates how attributes for newly developed WA 38 apple sold under the Cosmic Crisp brand can be used to accelerate demand. I identify the market segments where marketing is effective and identify the attributes of the brand that most appeal to consumers. The results show that sentiment on Cosmic Crisp brand is positive with an overall compound score of 0.2263. The online conversation on the brand revolves around the history and novelty of the variety, farm tours to drive demand, the taste and appearance of the apple and its affiliation to the university where it was developed.
Three Essays On Machine Learning In Empirical Finance
DOWNLOAD
Author : Jinhua Wang
language : en
Publisher:
Release Date : 2022
Three Essays On Machine Learning In Empirical Finance written by Jinhua Wang and has been published by this book supported file pdf, txt, epub, kindle and other format this book has been release on 2022 with categories.
Essays In Applied Microeconomics
DOWNLOAD
Author : Arman Khachiyan
language : en
Publisher:
Release Date : 2022
Essays In Applied Microeconomics written by Arman Khachiyan and has been published by this book supported file pdf, txt, epub, kindle and other format this book has been release on 2022 with categories.
This dissertation contains three essays studying topics in applied microeconomics. The first chapter is a co-authored paper in which we use daytime satellite imagery and convolutional neural networks to model economic growth at the neighborhood level. In the second chapter, I use this model to examine the spatial distribution of residential impacts from fracking. The third chapter investigates methods of measuring skill distance between occupations and proposes a new method which matches patterns of observed occupational transition. Each chapter uses unconventional data sources and machine learning techniques to contribute to central questions in labor economics research and policy. In the first chapter we apply deep learning to daytime satellite imagery to predict changes in income and population at high spatial resolution in US data. Our model predictions achieve R2 values of and 0.32 to 0.46 in decadal changes, which have no counterpart in the literature and are 3-4 times larger than for commonly used nighttime lights. Our network has wide application for analyzing localized economic shocks. One such application is my second chapter, which studies changes in total neighborhood income and population in areas near fracking extraction and shale reserves. My microspatial approach identifies that fracking exposure as far as 20 miles away leads to a 2 percent decline in neighborhood income. The spatial gradient and associated mechanisms of this effect indicate that it is driven by local industrialization rather than direct environmental externalities. Examination reveals margins of policy and labor conditions which attenuate the observed impacts. In the third chapter I show that a regression framework generates a novel, empirical occupational skill distance norm which is disciplined by observed occupation switching patterns. This approach relieves key limitations of existing measures such as linearity and symmetry. It also allows for an analysis of which skill dimensions relate to the portability of human capital, and which do not. Implications for existing results on skill portability are discussed, along with immediate policy applications on employee adjustment costs.
Essays On The Application Of Machine Learning Techniques In The Empirical Asset Pricing Research
DOWNLOAD
Author : Tizian Otto
language : en
Publisher:
Release Date : 2022
Essays On The Application Of Machine Learning Techniques In The Empirical Asset Pricing Research written by Tizian Otto and has been published by this book supported file pdf, txt, epub, kindle and other format this book has been release on 2022 with categories.
Essays On Information Technology Intangible Capital And The Economics Of Artificial Intelligence
DOWNLOAD
Author : Daniel Ian Rock
language : en
Publisher:
Release Date : 2019
Essays On Information Technology Intangible Capital And The Economics Of Artificial Intelligence written by Daniel Ian Rock and has been published by this book supported file pdf, txt, epub, kindle and other format this book has been release on 2019 with categories.
This dissertation contains four essays concerning the economics of information technology, intangible capital, and artificial intelligence. In the first essay, "Engineering Value: The Returns to Technological Talent and Investments in Artificial Intelligence" I describe how firms can appropriate some of the value of their employees' human capital by assigning firm-specific tasks. I then use a database of employment records to document dynamics in the valuation of publicly traded firms as they relate to different types of employment, focusing especially on AI skills. The second essay, "The Productivity J-Curve: How Intangibles Complement General Purpose Technologies" (coauthored with Erik Brynjolfsson and Chad Syverson) addresses the concern that new technologies with wide applicability throughout the economy can cause both underestimation and overestimation of total factor productivity. As capital is accumulated, intangible investment output, and therefore productivity growth, will be underestimated only to later generate a yield (at which point productivity growth will be overestimated). Presenting a theoretical description of how to use corporate valuations to recover hidden investment value, we discuss how productivity growth and levels can be adjusted to accommodate these changes. Implications for research and development, computer hardware, and computer software investments are considered. The third essay, "Machine Learning and Occupational Change" (coauthored with Erik Brynjolfsson and Tom Mitchell), develops and implements a method to measure the labor market impact potential of machine learning technologies. Tasks are evaluated for their Suitability for Machine Learning (SML). We find that few occupations can be fully automated with machine learning, but many occupations will potentially be redesigned. The final essay, "Do Labor Demand Shifts Occur Within Firms or Across Them? Non-Routine-Biased Technological Change 2000-2016" (coauthored with Seth Benzell and Guillermo Lagarda) decomposes labor share shifts of occupational groups into changes between firms, within firms, and due to entry and exit. We find that within-firm compositional shifts are an important component of changes in the overall labor market. We also find that the rate of within-firm shifts has declined in the period from 2000 to 2016. Together, these essays offer insights into how artificial intelligence technologies, particularly machine learning, will impact the U.S. economy.