Evolution Of Artificial Neural Development
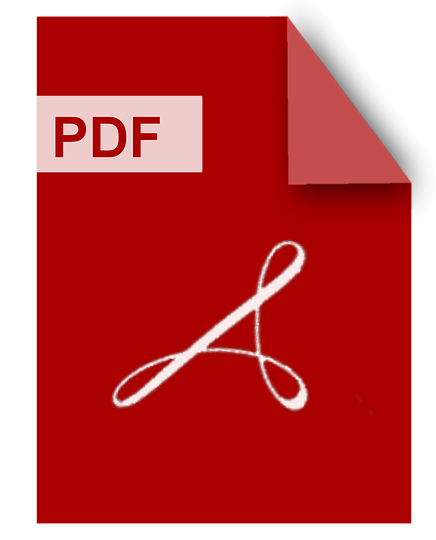
DOWNLOAD
Download Evolution Of Artificial Neural Development PDF/ePub or read online books in Mobi eBooks. Click Download or Read Online button to get Evolution Of Artificial Neural Development book now. This website allows unlimited access to, at the time of writing, more than 1.5 million titles, including hundreds of thousands of titles in various foreign languages. If the content not found or just blank you must refresh this page
Evolution Of Artificial Neural Development
DOWNLOAD
Author : Gul Muhammad Khan
language : en
Publisher: Springer
Release Date : 2017-10-27
Evolution Of Artificial Neural Development written by Gul Muhammad Khan and has been published by Springer this book supported file pdf, txt, epub, kindle and other format this book has been release on 2017-10-27 with Technology & Engineering categories.
This book presents recent research on the evolution of artificial neural development, and searches for learning genes. It is fascinating to see how all biological cells share virtually the same traits, but humans have a decided edge over other species when it comes to intelligence. Although DNA decides the form each particular species takes, does it also account for intelligent behaviour in living beings? The authors explore the factors that are perceived as intelligent behaviour in living beings and the incorporation of these factors in machines using genetic programming, which ultimately provides a platform for exploring the possibility of machines that can learn by themselves, i.e. that can “learn how to learn”. The book will be of interest not only to the specialized scientific community pursuing machine intelligence, but also general readers who would like to know more about the incorporation of intelligent behaviour in machines, inspired by the human brain.
Growing Adaptive Machines
DOWNLOAD
Author : Taras Kowaliw
language : en
Publisher: Springer
Release Date : 2014-06-04
Growing Adaptive Machines written by Taras Kowaliw and has been published by Springer this book supported file pdf, txt, epub, kindle and other format this book has been release on 2014-06-04 with Technology & Engineering categories.
The pursuit of artificial intelligence has been a highly active domain of research for decades, yielding exciting scientific insights and productive new technologies. In terms of generating intelligence, however, this pursuit has yielded only limited success. This book explores the hypothesis that adaptive growth is a means of moving forward. By emulating the biological process of development, we can incorporate desirable characteristics of natural neural systems into engineered designs and thus move closer towards the creation of brain-like systems. The particular focus is on how to design artificial neural networks for engineering tasks. The book consists of contributions from 18 researchers, ranging from detailed reviews of recent domains by senior scientists, to exciting new contributions representing the state of the art in machine learning research. The book begins with broad overviews of artificial neurogenesis and bio-inspired machine learning, suitable both as an introduction to the domains and as a reference for experts. Several contributions provide perspectives and future hypotheses on recent highly successful trains of research, including deep learning, the Hyper NEAT model of developmental neural network design, and a simulation of the visual cortex. Other contributions cover recent advances in the design of bio-inspired artificial neural networks, including the creation of machines for classification, the behavioural control of virtual agents, the desi gn of virtual multi-component robots and morphologies and the creation of flexible intelligence. Throughout, the contributors share their vast expertise on the means and benefits of creating brain-like machines. This book is appropriate for advanced students and practitioners of artificial intelligence and machine learning.
Computational Evolution Of Neural And Morphological Development
DOWNLOAD
Author : Yaochu Jin
language : en
Publisher: Springer Nature
Release Date : 2023-07-14
Computational Evolution Of Neural And Morphological Development written by Yaochu Jin and has been published by Springer Nature this book supported file pdf, txt, epub, kindle and other format this book has been release on 2023-07-14 with Computers categories.
This book provides a basic yet unified overview of theory and methodologies for evolutionary developmental systems. Based on the author’s extensive research into the synergies between various approaches to artificial intelligence including evolutionary computation, artificial neural networks, and systems biology, it also examines the inherent links between biological intelligence and artificial intelligence. The book begins with an introduction to computational algorithms used to understand and simulate biological evolution and development, including evolutionary algorithms, gene regulatory network models, multi-cellular models for neural and morphological development, and computational models of neural plasticity. Chap. 2 discusses important properties of biological gene regulatory systems, including network motifs, network connectivity, robustness and evolvability. Going a step further, Chap. 3 presents methods for synthesizing regulatory motifs from scratch and creating more complex regulatory dynamics by combining basic regulatory motifs using evolutionary algorithms. Multi-cellular growth models, which can be used to simulate either neural or morphological development, are presented in Chapters 4 and 5. Chap. 6 examines the synergies and coupling between neural and morphological evolution and development. In turn, Chap. 7 provides preliminary yet promising examples of how evolutionary developmental systems can help in self-organized pattern generation, referred to as morphogenetic self-organization, highlighting the great potentials of evolutionary developmental systems. Finally, Chap. 8 rounds out the book, stressing the importance and promise of the evolutionary developmental approach to artificial intelligence. Featuring a wealth of diagrams, graphs and charts to aid in comprehension, this book offers a valuable asset for graduate students, researchers and practitioners who are interested in pursuing a different approach to artificial intelligence.
Evolving Artificial Neural Networks With Generative Encodings Inspired By Developmental Biology
DOWNLOAD
Author : Jeff Clune
language : en
Publisher:
Release Date : 2010
Evolving Artificial Neural Networks With Generative Encodings Inspired By Developmental Biology written by Jeff Clune and has been published by this book supported file pdf, txt, epub, kindle and other format this book has been release on 2010 with Evolutionary computation categories.
The Evolution Of Modular Artificial Neural Networks
DOWNLOAD
Author :
language : en
Publisher:
Release Date : 2001
The Evolution Of Modular Artificial Neural Networks written by and has been published by this book supported file pdf, txt, epub, kindle and other format this book has been release on 2001 with categories.
This thesis describes a novel approach to the evolution of Modular Artificial Neural Networks. Standard Evolutionary Algorithms, used in this application include: Genetic Algorithms, Evolutionary Strategies, Evolutionary Programming and Genetic Programming; however, these often fail in the evolution of complex systems, particularly when such systems involve multi-domain sensory information which interacts in complex ways with system outputs. The aim in this work is to produce an evolutionary method that allows the structure of the network to evolve from simple to complex as it interacts with a dynamic environment. This new algorithm is therefore based on Incremental Evolution. A simulated model of a legged robot was used as a test-bed for the approach. The algorithm starts with a simple robotic body plan. This then grows incrementally in complexity along with its controlling neural network and the environment it reacts with. The network grows by adding modules to its structure - so the technique may also be termed a Growth Algorithm. Experiments are presented showing the successful evolution of multi-legged gaits and a simple vision system. These are then integrated together to form a complete robotic system. The possibility of the evolution of complex systems is one advantage of the algorithm and it is argued that it represents a possible path towards more advanced artificial intelligence. Applications in Electronics, Computer Science, Mechanical Engineering and Aerospace are also discussed.
The Synthesis Of Artificial Neural Networks Using Single String Evolutionary Techniques
DOWNLOAD
Author :
language : en
Publisher:
Release Date : 2009
The Synthesis Of Artificial Neural Networks Using Single String Evolutionary Techniques written by and has been published by this book supported file pdf, txt, epub, kindle and other format this book has been release on 2009 with categories.
The research presented in this thesis is concerned with optimising the structure of Artificial Neural Networks. These techniques are based on computer modelling of biological evolution or foetal development. They are known as Evolutionary, Genetic or Embryological methods. Specifically, Embryological techniques are used to grow Artificial Neural Network topologies. The Embryological Algorithm is an alternative to the popular Genetic Algorithm, which is widely used to achieve similar results. The algorithm grows in the sense that the network structure is added to incrementally and thus changes from a simple form to a more complex form. This is unlike the Genetic Algorithm, which causes the structure of the network to evolve in an unstructured or random way. The thesis outlines the following original work: The operation of the Embryological Algorithm is described and compared with the Genetic Algorithm. The results of an exhaustive literature search in the subject area are reported. The growth strategies which may be used to evolve Artificial Neural Network structure are listed. These growth strategies are integrated into an algorithm for network growth. Experimental results obtained from using such a system are described and there is a discussion of the applications of the approach. Consideration is given of the advantages and disadvantages of this technique and suggestions are made for future work in the area. A new learning algorithm based on Taguchi methods is also described. The report concludes that the method of incremental growth is a useful and powerful technique for defining neural network structures and is more efficient than its alternatives. Recommendations are also made with regard to the types of network to which this approach is best suited. Finally, the report contains a discussion of two important aspects of Genetic or Evolutionary techniques related to the above. These are Modular networks (and their synthesis) and the functionality of the network i.
The Self Assembling Brain
DOWNLOAD
Author : Peter Robin Hiesinger
language : en
Publisher: Princeton University Press
Release Date : 2022-12-13
The Self Assembling Brain written by Peter Robin Hiesinger and has been published by Princeton University Press this book supported file pdf, txt, epub, kindle and other format this book has been release on 2022-12-13 with Computers categories.
"In this book, Peter Robin Hiesinger explores historical and contemporary attempts to understand the information needed to make biological and artificial neural networks. Developmental neurobiologists and computer scientists with an interest in artificial intelligence - driven by the promise and resources of biomedical research on the one hand, and by the promise and advances of computer technology on the other - are trying to understand the fundamental principles that guide the generation of an intelligent system. Yet, though researchers in these disciplines share a common interest, their perspectives and approaches are often quite different. The book makes the case that "the information problem" underlies both fields, driving the questions that are driving forward the frontiers, and aims to encourage cross-disciplinary communication and understanding, to help both fields make progress. The questions that challenge researchers in these fields include the following. How does genetic information unfold during the years-long process of human brain development, and can this be a short-cut to create human-level artificial intelligence? Is the biological brain just messy hardware that can be improved upon by running learning algorithms in computers? Can artificial intelligence bypass evolutionary programming of "grown" networks? These questions are tightly linked, and answering them requires an understanding of how information unfolds algorithmically to generate functional neural networks. Via a series of closely linked "discussions" (fictional dialogues between researchers in different disciplines) and pedagogical "seminars," the author explores the different challenges facing researchers working on neural networks, their different perspectives and approaches, as well as the common ground and understanding to be found amongst those sharing an interest in the development of biological brains and artificial intelligent systems"--
Hands On Neuroevolution With Python
DOWNLOAD
Author : Iaroslav Omelianenko
language : en
Publisher: Packt Publishing Ltd
Release Date : 2019-12-24
Hands On Neuroevolution With Python written by Iaroslav Omelianenko and has been published by Packt Publishing Ltd this book supported file pdf, txt, epub, kindle and other format this book has been release on 2019-12-24 with Computers categories.
Increase the performance of various neural network architectures using NEAT, HyperNEAT, ES-HyperNEAT, Novelty Search, SAFE, and deep neuroevolution Key FeaturesImplement neuroevolution algorithms to improve the performance of neural network architecturesUnderstand evolutionary algorithms and neuroevolution methods with real-world examplesLearn essential neuroevolution concepts and how they are used in domains including games, robotics, and simulationsBook Description Neuroevolution is a form of artificial intelligence learning that uses evolutionary algorithms to simplify the process of solving complex tasks in domains such as games, robotics, and the simulation of natural processes. This book will give you comprehensive insights into essential neuroevolution concepts and equip you with the skills you need to apply neuroevolution-based algorithms to solve practical, real-world problems. You'll start with learning the key neuroevolution concepts and methods by writing code with Python. You'll also get hands-on experience with popular Python libraries and cover examples of classical reinforcement learning, path planning for autonomous agents, and developing agents to autonomously play Atari games. Next, you'll learn to solve common and not-so-common challenges in natural computing using neuroevolution-based algorithms. Later, you'll understand how to apply neuroevolution strategies to existing neural network designs to improve training and inference performance. Finally, you'll gain clear insights into the topology of neural networks and how neuroevolution allows you to develop complex networks, starting with simple ones. By the end of this book, you will not only have explored existing neuroevolution-based algorithms, but also have the skills you need to apply them in your research and work assignments. What you will learnDiscover the most popular neuroevolution algorithms – NEAT, HyperNEAT, and ES-HyperNEATExplore how to implement neuroevolution-based algorithms in PythonGet up to speed with advanced visualization tools to examine evolved neural network graphsUnderstand how to examine the results of experiments and analyze algorithm performanceDelve into neuroevolution techniques to improve the performance of existing methodsApply deep neuroevolution to develop agents for playing Atari gamesWho this book is for This book is for machine learning practitioners, deep learning researchers, and AI enthusiasts who are looking to implement neuroevolution algorithms from scratch. Working knowledge of the Python programming language and basic knowledge of deep learning and neural networks are mandatory.
Nature Vs Nurture Effects Of Learning On Evolution
DOWNLOAD
Author : Nagina Nagrani
language : en
Publisher:
Release Date : 2010
Nature Vs Nurture Effects Of Learning On Evolution written by Nagina Nagrani and has been published by this book supported file pdf, txt, epub, kindle and other format this book has been release on 2010 with categories.
In the field of Evolutionary Robotics, the design, development and application of artificial neural networks as controllers have derived their inspiration from biology. Biologists and artificial intelligence researchers are trying to understand the effects of neural network learning during the lifetime of the individuals on evolution of these individuals by qualitative and quantitative analyses. The conclusion of these analyses can help develop optimized artificial neural networks to perform any given task. The purpose of this thesis is to study the effects of learning on evolution. This has been done by applying Temporal Difference Reinforcement Learning methods to the evolution of Artificial Neural Tissue controller. The controller has been assigned the task to collect resources in a designated area in a simulated environment. The performance of the individuals is measured by the amount of resources collected. A comparison has been made between the results obtained by incorporating learning in evolution and evolution alone. The effects of learning parameters: learning rate, training period, discount rate, and policy on evolution have also been studied. It was observed that learning delays the performance of the evolving individuals over the generations. However, the non zero learning rate throughout the evolution process signifies natural selection preferring individuals possessing plasticity.
Artificial Neural Nets And Genetic Algorithms
DOWNLOAD
Author : George D. Smith
language : en
Publisher: Springer Science & Business Media
Release Date : 2012-12-06
Artificial Neural Nets And Genetic Algorithms written by George D. Smith and has been published by Springer Science & Business Media this book supported file pdf, txt, epub, kindle and other format this book has been release on 2012-12-06 with Computers categories.
This is the third in a series of conferences devoted primarily to the theory and applications of artificial neural networks and genetic algorithms. The first such event was held in Innsbruck, Austria, in April 1993, the second in Ales, France, in April 1995. We are pleased to host the 1997 event in the mediaeval city of Norwich, England, and to carryon the fine tradition set by its predecessors of providing a relaxed and stimulating environment for both established and emerging researchers working in these and other, related fields. This series of conferences is unique in recognising the relation between the two main themes of artificial neural networks and genetic algorithms, each having its origin in a natural process fundamental to life on earth, and each now well established as a paradigm fundamental to continuing technological development through the solution of complex, industrial, commercial and financial problems. This is well illustrated in this volume by the numerous applications of both paradigms to new and challenging problems. The third key theme of the series, therefore, is the integration of both technologies, either through the use of the genetic algorithm to construct the most effective network architecture for the problem in hand, or, more recently, the use of neural networks as approximate fitness functions for a genetic algorithm searching for good solutions in an 'incomplete' solution space, i.e. one for which the fitness is not easily established for every possible solution instance.