Explainable And Interpretable Models In Computer Vision And Machine Learning
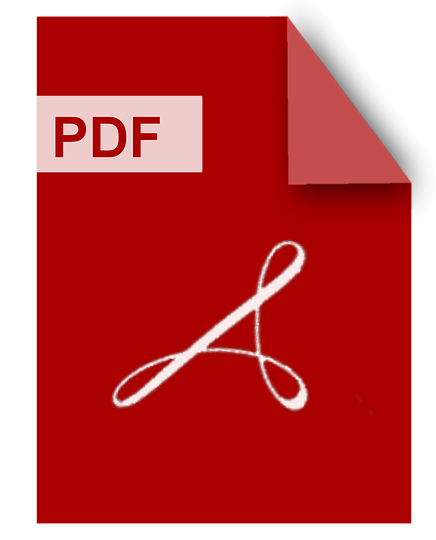
DOWNLOAD
Download Explainable And Interpretable Models In Computer Vision And Machine Learning PDF/ePub or read online books in Mobi eBooks. Click Download or Read Online button to get Explainable And Interpretable Models In Computer Vision And Machine Learning book now. This website allows unlimited access to, at the time of writing, more than 1.5 million titles, including hundreds of thousands of titles in various foreign languages. If the content not found or just blank you must refresh this page
Explainable And Interpretable Models In Computer Vision And Machine Learning
DOWNLOAD
Author : Hugo Jair Escalante
language : en
Publisher: Springer
Release Date : 2018-11-29
Explainable And Interpretable Models In Computer Vision And Machine Learning written by Hugo Jair Escalante and has been published by Springer this book supported file pdf, txt, epub, kindle and other format this book has been release on 2018-11-29 with Computers categories.
This book compiles leading research on the development of explainable and interpretable machine learning methods in the context of computer vision and machine learning. Research progress in computer vision and pattern recognition has led to a variety of modeling techniques with almost human-like performance. Although these models have obtained astounding results, they are limited in their explainability and interpretability: what is the rationale behind the decision made? what in the model structure explains its functioning? Hence, while good performance is a critical required characteristic for learning machines, explainability and interpretability capabilities are needed to take learning machines to the next step to include them in decision support systems involving human supervision. This book, written by leading international researchers, addresses key topics of explainability and interpretability, including the following: · Evaluation and Generalization in Interpretable Machine Learning · Explanation Methods in Deep Learning · Learning Functional Causal Models with Generative Neural Networks · Learning Interpreatable Rules for Multi-Label Classification · Structuring Neural Networks for More Explainable Predictions · Generating Post Hoc Rationales of Deep Visual Classification Decisions · Ensembling Visual Explanations · Explainable Deep Driving by Visualizing Causal Attention · Interdisciplinary Perspective on Algorithmic Job Candidate Search · Multimodal Personality Trait Analysis for Explainable Modeling of Job Interview Decisions · Inherent Explainability Pattern Theory-based Video Event Interpretations
Interpretable Machine Learning
DOWNLOAD
Author : Christoph Molnar
language : en
Publisher: Lulu.com
Release Date : 2020
Interpretable Machine Learning written by Christoph Molnar and has been published by Lulu.com this book supported file pdf, txt, epub, kindle and other format this book has been release on 2020 with Computers categories.
This book is about making machine learning models and their decisions interpretable. After exploring the concepts of interpretability, you will learn about simple, interpretable models such as decision trees, decision rules and linear regression. Later chapters focus on general model-agnostic methods for interpreting black box models like feature importance and accumulated local effects and explaining individual predictions with Shapley values and LIME. All interpretation methods are explained in depth and discussed critically. How do they work under the hood? What are their strengths and weaknesses? How can their outputs be interpreted? This book will enable you to select and correctly apply the interpretation method that is most suitable for your machine learning project.
Explainable Ai With Python
DOWNLOAD
Author : Leonida Gianfagna
language : en
Publisher: Springer Nature
Release Date : 2021-04-28
Explainable Ai With Python written by Leonida Gianfagna and has been published by Springer Nature this book supported file pdf, txt, epub, kindle and other format this book has been release on 2021-04-28 with Computers categories.
This book provides a full presentation of the current concepts and available techniques to make “machine learning” systems more explainable. The approaches presented can be applied to almost all the current “machine learning” models: linear and logistic regression, deep learning neural networks, natural language processing and image recognition, among the others. Progress in Machine Learning is increasing the use of artificial agents to perform critical tasks previously handled by humans (healthcare, legal and finance, among others). While the principles that guide the design of these agents are understood, most of the current deep-learning models are "opaque" to human understanding. Explainable AI with Python fills the current gap in literature on this emerging topic by taking both a theoretical and a practical perspective, making the reader quickly capable of working with tools and code for Explainable AI. Beginning with examples of what Explainable AI (XAI) is and why it is needed in the field, the book details different approaches to XAI depending on specific context and need. Hands-on work on interpretable models with specific examples leveraging Python are then presented, showing how intrinsic interpretable models can be interpreted and how to produce “human understandable” explanations. Model-agnostic methods for XAI are shown to produce explanations without relying on ML models internals that are “opaque.” Using examples from Computer Vision, the authors then look at explainable models for Deep Learning and prospective methods for the future. Taking a practical perspective, the authors demonstrate how to effectively use ML and XAI in science. The final chapter explains Adversarial Machine Learning and how to do XAI with adversarial examples.
Practical Explainable Ai Using Python
DOWNLOAD
Author : Pradeepta Mishra
language : en
Publisher: Apress
Release Date : 2021-12-15
Practical Explainable Ai Using Python written by Pradeepta Mishra and has been published by Apress this book supported file pdf, txt, epub, kindle and other format this book has been release on 2021-12-15 with Computers categories.
Learn the ins and outs of decisions, biases, and reliability of AI algorithms and how to make sense of these predictions. This book explores the so-called black-box models to boost the adaptability, interpretability, and explainability of the decisions made by AI algorithms using frameworks such as Python XAI libraries, TensorFlow 2.0+, Keras, and custom frameworks using Python wrappers. You'll begin with an introduction to model explainability and interpretability basics, ethical consideration, and biases in predictions generated by AI models. Next, you'll look at methods and systems to interpret linear, non-linear, and time-series models used in AI. The book will also cover topics ranging from interpreting to understanding how an AI algorithm makes a decision Further, you will learn the most complex ensemble models, explainability, and interpretability using frameworks such as Lime, SHAP, Skater, ELI5, etc. Moving forward, you will be introduced to model explainability for unstructured data, classification problems, and natural language processing–related tasks. Additionally, the book looks at counterfactual explanations for AI models. Practical Explainable AI Using Python shines the light on deep learning models, rule-based expert systems, and computer vision tasks using various XAI frameworks. What You'll Learn Review the different ways of making an AI model interpretable and explainable Examine the biasness and good ethical practices of AI models Quantify, visualize, and estimate reliability of AI models Design frameworks to unbox the black-box models Assess the fairness of AI models Understand the building blocks of trust in AI models Increase the level of AI adoption Who This Book Is For AI engineers, data scientists, and software developers involved in driving AI projects/ AI products.
Interpretable Machine Learning With Python
DOWNLOAD
Author : Serg Masís
language : en
Publisher:
Release Date : 2021-03-26
Interpretable Machine Learning With Python written by Serg Masís and has been published by this book supported file pdf, txt, epub, kindle and other format this book has been release on 2021-03-26 with categories.
Understand the key aspects and challenges of machine learning interpretability, learn how to overcome them with interpretation methods, and leverage them to build fairer, safer, and more reliable models Key Features: Learn how to extract easy-to-understand insights from any machine learning model Become well-versed with interpretability techniques to build fairer, safer, and more reliable models Mitigate risks in AI systems before they have broader implications by learning how to debug black-box models Book Description: Do you want to understand your models and mitigate risks associated with poor predictions using machine learning (ML) interpretation? Interpretable Machine Learning with Python can help you work effectively with ML models. The first section of the book is a beginner's guide to interpretability, covering its relevance in business and exploring its key aspects and challenges. You'll focus on how white-box models work, compare them to black-box and glass-box models, and examine their trade-off. The second section will get you up to speed with a vast array of interpretation methods, also known as Explainable AI (XAI) methods, and how to apply them to different use cases, be it for classification or regression, for tabular, time-series, image or text. In addition to the step-by-step code, the book also helps the reader to interpret model outcomes using examples. In the third section, you'll get hands-on with tuning models and training data for interpretability by reducing complexity, mitigating bias, placing guardrails, and enhancing reliability. The methods you'll explore here range from state-of-the-art feature selection and dataset debiasing methods to monotonic constraints and adversarial retraining. By the end of this book, you'll be able to understand ML models better and enhance them through interpretability tuning. What You Will Learn: Recognize the importance of interpretability in business Study models that are intrinsically interpretable such as linear models, decision trees, and Naïve Bayes Become well-versed in interpreting models with model-agnostic methods Visualize how an image classifier works and what it learns Understand how to mitigate the influence of bias in datasets Discover how to make models more reliable with adversarial robustness Use monotonic constraints to make fairer and safer models Who this book is for: This book is for data scientists, machine learning developers, and data stewards who have an increasingly critical responsibility to explain how the AI systems they develop work, their impact on decision making, and how they identify and manage bias. Working knowledge of machine learning and the Python programming language is expected.
Escaping A Life Sentence
DOWNLOAD
Author : Cornell W Bunting
language : en
Publisher:
Release Date : 2020-06-17
Escaping A Life Sentence written by Cornell W Bunting and has been published by this book supported file pdf, txt, epub, kindle and other format this book has been release on 2020-06-17 with categories.
After escaping a prison sentence, Cornell Bunting finds himself in a complicated time, as he died for sixteen minutes. After death knocked at his door, Cornell finds himself in between worlds, trying to figure out what's real and what's not. The chaos brought him closer to God and his purpose. Thinking back on the situation, Cornell wondered what got him into his life-changing situations. After spending ten months in jail, facing 32 years to a life sentence, getting out of jail, and stressing on his role as a father of three boys, what caused him to die? How will he escape his life sentence of the mind after escaping a life sentence in prison? The drama unfolds as Cornell's memory goes on a quest to resolve his mental life sentence. God has given him a second chance at life to get on the path to his true destiny, understanding everything in this world is temporary. Escaping a life sentence of the mind starts with letting go of self and the temptations of the world.
Deep Learning In Gaming And Animations
DOWNLOAD
Author : Vikas Chaudhary
language : en
Publisher: CRC Press
Release Date : 2021-12-07
Deep Learning In Gaming And Animations written by Vikas Chaudhary and has been published by CRC Press this book supported file pdf, txt, epub, kindle and other format this book has been release on 2021-12-07 with Computers categories.
Over the last decade, progress in deep learning has had a profound and transformational effect on many complex problems, including speech recognition, machine translation, natural language understanding, and computer vision. As a result, computers can now achieve human-competitive performance in a wide range of perception and recognition tasks. Many of these systems are now available to the programmer via a range of so-called cognitive services. More recently, deep reinforcement learning has achieved ground-breaking success in several complex challenges. This book makes an enormous contribution to this beautiful, vibrant area of study: an area that is developing rapidly both in breadth and depth. Deep learning can cope with a broader range of tasks (and perform those tasks to increasing levels of excellence). This book lays a good foundation for the core concepts and principles of deep learning in gaming and animation, walking you through the fundamental ideas with expert ease. This book progresses in a step-by-step manner. It reinforces theory with a full-fledged pedagogy designed to enhance students' understanding and offer them a practical insight into its applications. Also, some chapters introduce and cover novel ideas about how artificial intelligence (AI), deep learning, and machine learning have changed the world in gaming and animation. It gives us the idea that AI can also be applied in gaming, and there are limited textbooks in this area. This book comprehensively addresses all the aspects of AI and deep learning in gaming. Also, each chapter follows a similar structure so that students, teachers, and industry experts can orientate themselves within the text. There are few books in the field of gaming using AI. Deep Learning in Gaming and Animations teaches you how to apply the power of deep learning to build complex reasoning tasks. After being exposed to the foundations of machine and deep learning, you will use Python to build a bot and then teach it the game's rules. This book also focuses on how different technologies have revolutionized gaming and animation with various illustrations.
Deep Learning For Nlp And Speech Recognition
DOWNLOAD
Author : Uday Kamath
language : en
Publisher: Springer
Release Date : 2019-06-10
Deep Learning For Nlp And Speech Recognition written by Uday Kamath and has been published by Springer this book supported file pdf, txt, epub, kindle and other format this book has been release on 2019-06-10 with Computers categories.
This textbook explains Deep Learning Architecture, with applications to various NLP Tasks, including Document Classification, Machine Translation, Language Modeling, and Speech Recognition. With the widespread adoption of deep learning, natural language processing (NLP),and speech applications in many areas (including Finance, Healthcare, and Government) there is a growing need for one comprehensive resource that maps deep learning techniques to NLP and speech and provides insights into using the tools and libraries for real-world applications. Deep Learning for NLP and Speech Recognition explains recent deep learning methods applicable to NLP and speech, provides state-of-the-art approaches, and offers real-world case studies with code to provide hands-on experience. Many books focus on deep learning theory or deep learning for NLP-specific tasks while others are cookbooks for tools and libraries, but the constant flux of new algorithms, tools, frameworks, and libraries in a rapidly evolving landscape means that there are few available texts that offer the material in this book. The book is organized into three parts, aligning to different groups of readers and their expertise. The three parts are: Machine Learning, NLP, and Speech Introduction The first part has three chapters that introduce readers to the fields of NLP, speech recognition, deep learning and machine learning with basic theory and hands-on case studies using Python-based tools and libraries. Deep Learning Basics The five chapters in the second part introduce deep learning and various topics that are crucial for speech and text processing, including word embeddings, convolutional neural networks, recurrent neural networks and speech recognition basics. Theory, practical tips, state-of-the-art methods, experimentations and analysis in using the methods discussed in theory on real-world tasks. Advanced Deep Learning Techniques for Text and Speech The third part has five chapters that discuss the latest and cutting-edge research in the areas of deep learning that intersect with NLP and speech. Topics including attention mechanisms, memory augmented networks, transfer learning, multi-task learning, domain adaptation, reinforcement learning, and end-to-end deep learning for speech recognition are covered using case studies.
Computer Vision Eccv 2014
DOWNLOAD
Author : David Fleet
language : en
Publisher: Springer
Release Date : 2014-08-13
Computer Vision Eccv 2014 written by David Fleet and has been published by Springer this book supported file pdf, txt, epub, kindle and other format this book has been release on 2014-08-13 with Computers categories.
The seven-volume set comprising LNCS volumes 8689-8695 constitutes the refereed proceedings of the 13th European Conference on Computer Vision, ECCV 2014, held in Zurich, Switzerland, in September 2014. The 363 revised papers presented were carefully reviewed and selected from 1444 submissions. The papers are organized in topical sections on tracking and activity recognition; recognition; learning and inference; structure from motion and feature matching; computational photography and low-level vision; vision; segmentation and saliency; context and 3D scenes; motion and 3D scene analysis; and poster sessions.