Finding Groups In Data
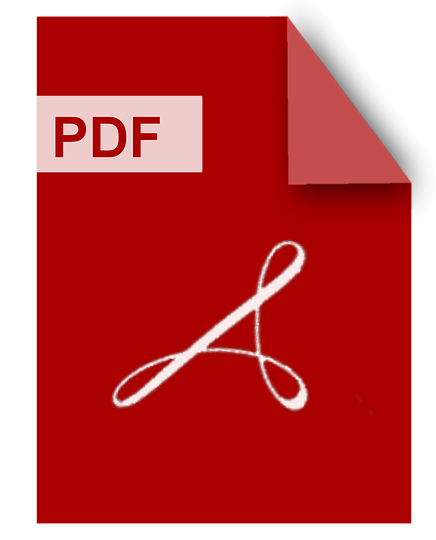
DOWNLOAD
Download Finding Groups In Data PDF/ePub or read online books in Mobi eBooks. Click Download or Read Online button to get Finding Groups In Data book now. This website allows unlimited access to, at the time of writing, more than 1.5 million titles, including hundreds of thousands of titles in various foreign languages. If the content not found or just blank you must refresh this page
Finding Groups In Data
DOWNLOAD
Author : Leonard Kaufman
language : en
Publisher:
Release Date : 1990
Finding Groups In Data written by Leonard Kaufman and has been published by this book supported file pdf, txt, epub, kindle and other format this book has been release on 1990 with categories.
Finding Groups In Data
DOWNLOAD
Author : Leonard Kaufman
language : en
Publisher: John Wiley & Sons
Release Date : 2009-09-25
Finding Groups In Data written by Leonard Kaufman and has been published by John Wiley & Sons this book supported file pdf, txt, epub, kindle and other format this book has been release on 2009-09-25 with Mathematics categories.
The Wiley-Interscience Paperback Series consists of selected books that have been made more accessible to consumers in an effort to increase global appeal and general circulation. With these new unabridged softcover volumes, Wiley hopes to extend the lives of these works by making them available to future generations of statisticians, mathematicians, and scientists. "Cluster analysis is the increasingly important and practical subject of finding groupings in data. The authors set out to write a book for the user who does not necessarily have an extensive background in mathematics. They succeed very well." —Mathematical Reviews "Finding Groups in Data [is] a clear, readable, and interesting presentation of a small number of clustering methods. In addition, the book introduced some interesting innovations of applied value to clustering literature." —Journal of Classification "This is a very good, easy-to-read, and practical book. It has many nice features and is highly recommended for students and practitioners in various fields of study." —Technometrics An introduction to the practical application of cluster analysis, this text presents a selection of methods that together can deal with most applications. These methods are chosen for their robustness, consistency, and general applicability. This book discusses various types of data, including interval-scaled and binary variables as well as similarity data, and explains how these can be transformed prior to clustering.
Finding Groups In Data
DOWNLOAD
Author : Leonard Kaufman
language : en
Publisher: Wiley-Interscience
Release Date : 1990-03-22
Finding Groups In Data written by Leonard Kaufman and has been published by Wiley-Interscience this book supported file pdf, txt, epub, kindle and other format this book has been release on 1990-03-22 with Mathematics categories.
Partitioning around medoids (Program PAM). Clustering large applications (Program CLARA). Fuzzy analysis (Program FANNY). Agglomerative Nesting (Program AGNES). Divisive analysis (Program DIANA). Monothetic analysis (Program MONA). Appendix.
Data Mining With Ontologies Implementations Findings And Frameworks
DOWNLOAD
Author : Nigro, Hector Oscar
language : en
Publisher: IGI Global
Release Date : 2007-07-31
Data Mining With Ontologies Implementations Findings And Frameworks written by Nigro, Hector Oscar and has been published by IGI Global this book supported file pdf, txt, epub, kindle and other format this book has been release on 2007-07-31 with Computers categories.
"Prior knowledge in data mining is helpful for selecting suitable data and mining techniques, pruning the space of hypothesis, representing the output in a comprehensible way, and improving the overall method. This book examines methodologies and research for the development of ontological foundations for data mining to enhance the ability of ontology utilization and design"--Provided by publisher.
Model Based Clustering And Classification For Data Science
DOWNLOAD
Author : Charles Bouveyron
language : en
Publisher: Cambridge University Press
Release Date : 2019-07-25
Model Based Clustering And Classification For Data Science written by Charles Bouveyron and has been published by Cambridge University Press this book supported file pdf, txt, epub, kindle and other format this book has been release on 2019-07-25 with Business & Economics categories.
Colorful example-rich introduction to the state-of-the-art for students in data science, as well as researchers and practitioners.
How To Find Inter Groups Differences Using Spss Excel Web Tools In Common Experimental Designs
DOWNLOAD
Author : P.Y. Cheng
language : en
Publisher: Xlibris Corporation
Release Date : 2014-04-08
How To Find Inter Groups Differences Using Spss Excel Web Tools In Common Experimental Designs written by P.Y. Cheng and has been published by Xlibris Corporation this book supported file pdf, txt, epub, kindle and other format this book has been release on 2014-04-08 with Computers categories.
Book 2: How to find Inter-Groups Differences Using SPSS/Excel/Web Tools In Common Experimental Designs Book 3: Analysis of Experimental Data Using Excel as if using SPSS A Break Through English Version Book 4: ???????????????? Excel ???? SPSS
How To Find Inter Groups Differences Using Spss Excel Web Tools In Common Experimental Designs Traditional Chinese
DOWNLOAD
Author : PY Cheng
language : en
Publisher: Xlibris Corporation
Release Date : 2014-06-28
How To Find Inter Groups Differences Using Spss Excel Web Tools In Common Experimental Designs Traditional Chinese written by PY Cheng and has been published by Xlibris Corporation this book supported file pdf, txt, epub, kindle and other format this book has been release on 2014-06-28 with Computers categories.
The first characteristic of this book is the skipping of difficult theories, starting directly with typical examples, and the working out of the answers clearly by computer software. The aim is to help readers to be able to solve basic statistical problems in various Common Experimental Designs as soon as possible, and to be confident to run the tests and interpret the computer output, without being hesitated by the starting with large amount of difficult theories behind. This book has been reviewed by some experts as ‘...very practical, illustrative and directive, and is very useful for both practitioners and those who perform statistical analysis in experimental designs...’ On the other hand, there is a general belief that after getting a significant Anova result, using the built-in ‘Analysis TookPak’ Excel Add-In, nothing can be done further to find where do the differences exist, and we must use large packages such as SPSS to complete the job! However, we find that this might not be absolutely true. We can often find similar results as using SPSS by combining the overall Anova results with a few, simple, manual steps introduced in this book!
Storytelling With Data
DOWNLOAD
Author : Cole Nussbaumer Knaflic
language : en
Publisher: John Wiley & Sons
Release Date : 2015-10-09
Storytelling With Data written by Cole Nussbaumer Knaflic and has been published by John Wiley & Sons this book supported file pdf, txt, epub, kindle and other format this book has been release on 2015-10-09 with Mathematics categories.
Don't simply show your data—tell a story with it! Storytelling with Data teaches you the fundamentals of data visualization and how to communicate effectively with data. You'll discover the power of storytelling and the way to make data a pivotal point in your story. The lessons in this illuminative text are grounded in theory, but made accessible through numerous real-world examples—ready for immediate application to your next graph or presentation. Storytelling is not an inherent skill, especially when it comes to data visualization, and the tools at our disposal don't make it any easier. This book demonstrates how to go beyond conventional tools to reach the root of your data, and how to use your data to create an engaging, informative, compelling story. Specifically, you'll learn how to: Understand the importance of context and audience Determine the appropriate type of graph for your situation Recognize and eliminate the clutter clouding your information Direct your audience's attention to the most important parts of your data Think like a designer and utilize concepts of design in data visualization Leverage the power of storytelling to help your message resonate with your audience Together, the lessons in this book will help you turn your data into high impact visual stories that stick with your audience. Rid your world of ineffective graphs, one exploding 3D pie chart at a time. There is a story in your data—Storytelling with Data will give you the skills and power to tell it!
DOWNLOAD
Author :
language : en
Publisher: EduGorilla Community Pvt. Ltd.
Release Date :
written by and has been published by EduGorilla Community Pvt. Ltd. this book supported file pdf, txt, epub, kindle and other format this book has been release on with categories.
Data Forecasting And Segmentation Using Microsoft Excel
DOWNLOAD
Author : Fernando Roque
language : en
Publisher: Packt Publishing Ltd
Release Date : 2022-05-27
Data Forecasting And Segmentation Using Microsoft Excel written by Fernando Roque and has been published by Packt Publishing Ltd this book supported file pdf, txt, epub, kindle and other format this book has been release on 2022-05-27 with Computers categories.
Perform time series forecasts, linear prediction, and data segmentation with no-code Excel machine learning Key Features • Segment data, regression predictions, and time series forecasts without writing any code • Group multiple variables with K-means using Excel plugin without programming • Build, validate, and predict with a multiple linear regression model and time series forecasts Book Description Data Forecasting and Segmentation Using Microsoft Excel guides you through basic statistics to test whether your data can be used to perform regression predictions and time series forecasts. The exercises covered in this book use real-life data from Kaggle, such as demand for seasonal air tickets and credit card fraud detection. You'll learn how to apply the grouping K-means algorithm, which helps you find segments of your data that are impossible to see with other analyses, such as business intelligence (BI) and pivot analysis. By analyzing groups returned by K-means, you'll be able to detect outliers that could indicate possible fraud or a bad function in network packets. By the end of this Microsoft Excel book, you'll be able to use the classification algorithm to group data with different variables. You'll also be able to train linear and time series models to perform predictions and forecasts based on past data. What you will learn • Understand why machine learning is important for classifying data segmentation • Focus on basic statistics tests for regression variable dependency • Test time series autocorrelation to build a useful forecast • Use Excel add-ins to run K-means without programming • Analyze segment outliers for possible data anomalies and fraud • Build, train, and validate multiple regression models and time series forecasts Who this book is for This book is for data and business analysts as well as data science professionals. MIS, finance, and auditing professionals working with MS Excel will also find this book beneficial.