Flexible Semi And Non Parametric Modelling And Prognosis For Discrete Outcomes
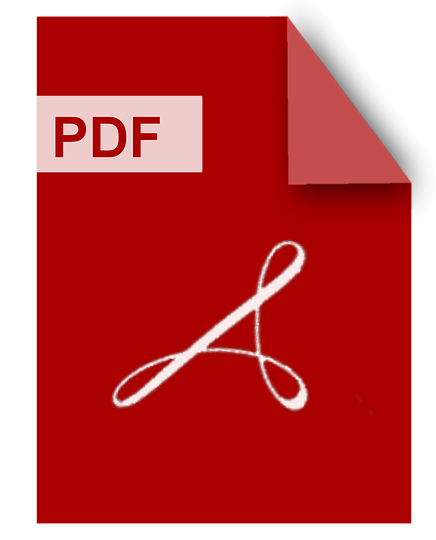
DOWNLOAD
Download Flexible Semi And Non Parametric Modelling And Prognosis For Discrete Outcomes PDF/ePub or read online books in Mobi eBooks. Click Download or Read Online button to get Flexible Semi And Non Parametric Modelling And Prognosis For Discrete Outcomes book now. This website allows unlimited access to, at the time of writing, more than 1.5 million titles, including hundreds of thousands of titles in various foreign languages. If the content not found or just blank you must refresh this page
Flexible Semi And Non Parametric Modelling And Prognosis For Discrete Outcomes
DOWNLOAD
Author : Harald Binder
language : en
Publisher:
Release Date : 2006
Flexible Semi And Non Parametric Modelling And Prognosis For Discrete Outcomes written by Harald Binder and has been published by this book supported file pdf, txt, epub, kindle and other format this book has been release on 2006 with categories.
This thesis presents new models and estimation techniques based on the generalized linear model framework. In Chapter 1 the GAMBoost procedure for estimation of generalized additive models is developed. Based on boosting and gradient descent in function space it generalizes the notion of repeated fitting of residuals to exponential family responses. By using a flexible number of updates for each covariate the selection of smoothing parameters is reduced to the selection of the number of boosting steps. The latter is chosen based on approximate effective degrees of freedom. The resulting procedure shows good performance for a wide variety of examples with binary and Poisson response data. A considerable advantage compared to other procedures is found for a large number of covariates and a low level of information. An application to real data is presented. In Chapter 2 a flexible model for discrete time survival data is developed that allows for non-linear covariate effects that vary over time. For estimation an iterative two-step procedure based on Fisher scoring is given. A simulation study with various levels of complexity underlying the data compares the performance of adequate models to the performance of models that are too restrictive, too flexible or that provide the wrong kind of flexibility. It is shown that effective degrees of freedom work well as a basis for selection of smoothing parameters as well as for model selection. An example with real data is given. In Chapter 3 a technique for classification with binary response data is developed based on logistic regression. It uses local models with local selection of predictors for reduction of complexity and penalized estimation for numerical stability. Standard simulated data examples are used to evaluate components of the algorithm such as the kernel for local weight calculation, and to compare the performance to other procedures. It is found that the procedure is competitive for a wide range of examples and that selection of predictors is crucial for local quadratic models while being regulated rather well for local linear models. Good performance can also be seen for real data examples.
Structural Equation Modeling For Health And Medicine
DOWNLOAD
Author : Douglas D. Gunzler
language : en
Publisher: CRC Press
Release Date : 2021-03-29
Structural Equation Modeling For Health And Medicine written by Douglas D. Gunzler and has been published by CRC Press this book supported file pdf, txt, epub, kindle and other format this book has been release on 2021-03-29 with Mathematics categories.
Structural equation modeling (SEM) is a very general and flexible multivariate technique that allows relationships among variables to be examined. The roots of SEM are in the social sciences. In writing this textbook, the authors look to make SEM accessible to a wider audience of researchers across many disciplines, addressing issues unique to health and medicine. SEM is often used in practice to model and test hypothesized causal relationships among observed and latent (unobserved) variables, including in analysis across time and groups. It can be viewed as the merging of a conceptual model, path diagram, confirmatory factor analysis, and path analysis. In this textbook the authors also discuss techniques, such as mixture modeling, that expand the capacity of SEM using a combination of both continuous and categorical latent variables. Features: Basic, intermediate, and advanced SEM topics Detailed applications, particularly relevant for health and medical scientists Topics and examples that are pertinent to both new and experienced SEM researchers Substantive issues in health and medicine in the context of SEM Both methodological and applied examples Numerous figures and diagrams to illustrate the examples As SEM experts situated among clinicians and multidisciplinary researchers in medical settings, the authors provide a broad, current, on the ground understanding of the issues faced by clinical and health services researchers and decision scientists. This book gives health and medical researchers the tools to apply SEM approaches to study complex relationships between clinical measurements, individual and community-level characteristics, and patient-reported scales.
Semi Parametric Estimation In Network Data And Tools For Conducting Complex Simulation Studies In Causal Inference
DOWNLOAD
Author : Oleg Sofrygin
language : en
Publisher:
Release Date : 2016
Semi Parametric Estimation In Network Data And Tools For Conducting Complex Simulation Studies In Causal Inference written by Oleg Sofrygin and has been published by this book supported file pdf, txt, epub, kindle and other format this book has been release on 2016 with categories.
This dissertation is concerned with application of robust semi-parametric methods to problems of estimation in network-dependent data and the conduct of large-scale simulation studies for causal inference research in epidemiological and medical data. Specifically, Chapter 1 presents a modern semi-parametric approach to estimation of causal effects in a population connected by a single social network. The connectivity of the population units will typically imply that the observed data on these units is no longer independent and identically distributed. Moreover, such social settings typically result in highly dimensional data. This chapter contributes to current statistical methodology by presenting an approach that allows valid estimation and inference and addresses the statistical issues specific to such networked population datasets. The framework of semi-parametric estimation, called the targeted maximum likelihood estimation (TMLE), is presented. This framework improves upon the existing methods by offering robustness, weakened sensitivity to near positivity violations, as well as the ability to deal with high-dimensionality issues of social network data. In particular, this approach relies on the accurate reflection of the background knowledge available for a given scientific problem, allowing estimation and inference without having to make unrealistic assumptions about the structure of the data. In addition, this chapter generalizes previous work describing estimation of complex causal parameters, such as the direct treatment effects under interference and the causal effects of interventions on social network structure. Although the past decade has produced many contributions towards estimation of causal effects in social network settings, there has been considerably less research on the topic of variance estimation for such highly-dependent data. This chapter presents an approach to constructing valid inference, providing a variance estimator that is scalable to very large datasets with highly-connected observations. The efficient open-source software implementation of these methods also accompanies this chapter. Chapter 2 presents open-source software tools for conduct of reproducible simulation studies for complex parameters that emerge from application of causal inference methods in epidemiological and medical research. This simulation software is build on the framework of non-parametric structural equation modeling. This chapter also studies simulation-based testing of statistical methods in causal inference for longitudinal data with time-varying exposure and confounding. It contributes to existing literature by presenting a unified syntax for non-parametrically defining complex causal parameters, which can be used as the model-free and agnostic gold standard for comparison of different statistical methods for causal inference. For instance, this chapter provides various examples of specification and evaluation of causal parameters that arise naturally in longitudinal causal effect analyses when using marginal structural models (MSMs). The application of these newly developed software tools to replication of several previously published simulation studies in causal inference are also described. Chapter 3 builds on the work described in Chapter 2 and addresses the issue of dependent data simulation for causal inference research in social network data. In particular, it provides a model-free approach to test the validity of various estimation procedures in simulated network-settings. This chapter first outlines a non-parametric causal model for units connected by a network and provides various applied examples of simulations with social network data. This chapter also showcases a possible application of the highly scalable open-source software implementation of the semi-parametric estimation methods described in Chapter 1. In particular, a large scale social network simulation study is described, and the performance of three dependent-data estimators from Chapter 1 is examined. This simulation study also examines the problem of inference for network-dependent data, specifically, by comparing the performance of the dependent-data TMLE variance estimator from Chapter 1 to the true TMLE variance derived from simulations. Finally, Chapter 3 concludes with a simulation study of an HIV epidemic described in terms of a longitudinal process which evolves over a static network in discrete time-steps among several highly inter-connected communities. The abstracts of the three works which make up this dissertation are reproduced below. Chapter 1: This chapter describes the robust semi-parametric approach towards estimation and inference for the sample average treatment-specific mean in observational settings where data are collected on a single network of connected units (e.g., in the presence of interference or spillover). Despite recent advances, many of the currently used statistical methods rely on assumption of a specific parametric model for the outcome, even though some of the most important statistical assumptions required by these models are most likely violated in the observational network data settings, resulting in invalid and anti-conservative statistical inference. In this chapter, we rely on the recent methodological advances for the targeted maximum likelihood estimation (TMLE) for data collected on a single population of causally connected units, to describe an estimation approach that permits for more realistic classes of data-generative models and provides valid statistical inference in the context of such network-dependent data. The approach is applied to an observational setting with a single time point stochastic intervention. We start by assuming that the true observed data-generating distribution belongs to a large class of semi-parametric statistical models. We then impose some restrictions on the possible set of the data-generative distributions that may belong to our statistical model. For example, we assume that the dependence among units can be fully described by the known network, and that the dependence on other units can be summarized via some known (but otherwise arbitrary) summary measures. We show that under our modeling assumptions, our estimand is equivalent to an estimand in a hypothetical IID data distribution, where the latter distribution is a function of the observed network data-generating distribution. With this key insight in mind, we show that the TMLE for our estimand in dependent network data can be described as a certain IID data TMLE algorithm, also resulting in a new simplified approach to conducting statistical inference. We demonstrate the validity of our approach in a network simulation study. We also extend prior work on dependent-data TMLE towards estimation of novel causal parameters, e.g., the unit-specific direct treatment effects under interference and the effects of interventions that modify the initial network structure. Chapter 2: This chapter introduces the \pkg{simcausal} \proglang{R} package - an open-source software tool for specification and simulation of complex longitudinal data structures that are based on non-parametric structural equation models. The package aims to provide a flexible tool for simplifying the conduct of transparent and reproducible simulation studies, with a particular emphasis on the types of data and interventions frequently encountered in real-world causal inference problems, such as, observational data with time-dependent confounding, selection bias, and random monitoring processes. The package interface allows for concise expression of complex functional dependencies between a large number of nodes, where each node may represent a measurement at a specific time point. The package allows for specification and simulation of counterfactual data under various user-specified interventions (e.g., static, dynamic, deterministic, or stochastic). In particular, the interventions may represent exposures to treatment regimens, the occurrence or non-occurrence of right-censoring events, or of clinical monitoring events. Finally, the package enables the computation of a selected set of user-specified features of the distribution of the counterfactual data that represent common causal quantities of interest, such as, treatment-specific means, the average treatment effects and coefficients from working marginal structural models. The applicability of \pkg{simcausal} is demonstrated by replicating the results of two published simulation studies. Chapter 3: The past decade has seen an increasing body of literature devoted to the estimation of causal effects in network-dependent data. However, the validity of many classical statistical methods in such data is often questioned. There is an emerging need for objective and practical ways to assess which causal methodologies might be applicable and valid in such novel network-based datasets. In this chapter we describe a set of tools implemented as part of the \pkg{simcausal} \proglang{R} package that allow simulating data based on the non-parametric structural equation model for connected units. We also provide examples of how these simulations may be applied to evaluation of different statistical methods for estimation of causal effects in such data. In particular, these simulation tools are targeted to the types of data and interventions frequently encountered in real-world causal inference research in social networks, such as, observational studies with spill-over or interference. We developed a novel \proglang{R} language interface which simplifies the specification of network-based functional relationships between connected units. Moreover, this network-based syntax can be combined with.
Complex Systems In Finance And Econometrics
DOWNLOAD
Author : Robert A. Meyers
language : en
Publisher: Springer Science & Business Media
Release Date : 2010-11-03
Complex Systems In Finance And Econometrics written by Robert A. Meyers and has been published by Springer Science & Business Media this book supported file pdf, txt, epub, kindle and other format this book has been release on 2010-11-03 with Business & Economics categories.
Finance, Econometrics and System Dynamics presents an overview of the concepts and tools for analyzing complex systems in a wide range of fields. The text integrates complexity with deterministic equations and concepts from real world examples, and appeals to a broad audience.
Identification In Nonparametric Models For Dynamic Treatment Effects
DOWNLOAD
Author : Sukjin Han
language : en
Publisher:
Release Date : 2018
Identification In Nonparametric Models For Dynamic Treatment Effects written by Sukjin Han and has been published by this book supported file pdf, txt, epub, kindle and other format this book has been release on 2018 with categories.
This paper develops a nonparametric model that represents how sequences of outcomes and treatment choices influence one another in a dynamic manner. In this setting, we are interested in identifying the average outcome for individuals in each period, had a particular treatment sequence been assigned. The identification of this quantity allows us to identify the average treatment effects (ATE's) and the ATE's on transitions, as well as the optimal treatment regimes, namely, the regimes that maximize the (weighted) sum of the average potential outcomes, possibly less the cost of the treatments. The main contribution of this paper is to relax the sequential randomization assumption widely used in the biostatistics literature by introducing a flexible choice-theoretic framework for a sequence of endogenous treatments. We show that the parameters of interest are identified under each period's two-way exclusion restriction, i.e., with instruments excluded from the outcome-determining process and other exogenous variables excluded from the treatment-selection process. We also consider partial identification in the case where the latter variables are not available. Lastly, we extend our results to a setting where treatments do not appear in every period.
Advances In Contemporary Statistics And Econometrics
DOWNLOAD
Author : Abdelaati Daouia
language : en
Publisher: Springer Nature
Release Date : 2021-06-14
Advances In Contemporary Statistics And Econometrics written by Abdelaati Daouia and has been published by Springer Nature this book supported file pdf, txt, epub, kindle and other format this book has been release on 2021-06-14 with Mathematics categories.
This book presents a unique collection of contributions on modern topics in statistics and econometrics, written by leading experts in the respective disciplines and their intersections. It addresses nonparametric statistics and econometrics, quantiles and expectiles, and advanced methods for complex data, including spatial and compositional data, as well as tools for empirical studies in economics and the social sciences. The book was written in honor of Christine Thomas-Agnan on the occasion of her 65th birthday. Given its scope, it will appeal to researchers and PhD students in statistics and econometrics alike who are interested in the latest developments in their field.
The Oxford Handbook Of Bayesian Econometrics
DOWNLOAD
Author : John Geweke
language : en
Publisher: Oxford University Press
Release Date : 2011-09-29
The Oxford Handbook Of Bayesian Econometrics written by John Geweke and has been published by Oxford University Press this book supported file pdf, txt, epub, kindle and other format this book has been release on 2011-09-29 with Business & Economics categories.
Bayesian econometric methods have enjoyed an increase in popularity in recent years. Econometricians, empirical economists, and policymakers are increasingly making use of Bayesian methods. This handbook is a single source for researchers and policymakers wanting to learn about Bayesian methods in specialized fields, and for graduate students seeking to make the final step from textbook learning to the research frontier. It contains contributions by leading Bayesians on the latest developments in their specific fields of expertise. The volume provides broad coverage of the application of Bayesian econometrics in the major fields of economics and related disciplines, including macroeconomics, microeconomics, finance, and marketing. It reviews the state of the art in Bayesian econometric methodology, with chapters on posterior simulation and Markov chain Monte Carlo methods, Bayesian nonparametric techniques, and the specialized tools used by Bayesian time series econometricians such as state space models and particle filtering. It also includes chapters on Bayesian principles and methodology.
Cure Models
DOWNLOAD
Author : Yingwei Peng
language : en
Publisher: CRC Press
Release Date : 2021-03-22
Cure Models written by Yingwei Peng and has been published by CRC Press this book supported file pdf, txt, epub, kindle and other format this book has been release on 2021-03-22 with Mathematics categories.
Cure Models: Methods, Applications and Implementation is the first book in the last 25 years that provides a comprehensive and systematic introduction to the basics of modern cure models, including estimation, inference, and software. This book is useful for statistical researchers and graduate students, and practitioners in other disciplines to have a thorough review of modern cure model methodology and to seek appropriate cure models in applications. The prerequisites of this book include some basic knowledge of statistical modeling, survival models, and R and SAS for data analysis. The book features real-world examples from clinical trials and population-based studies and a detailed introduction to R packages, SAS macros, and WinBUGS programs to fit some cure models. The main topics covered include the foundation of statistical estimation and inference of cure models for independent and right-censored survival data, cure modeling for multivariate, recurrent-event, and competing-risks survival data, and joint modeling with longitudinal data, statistical testing for the existence and difference of cure rates and sufficient follow-up, new developments in Bayesian cure models, applications of cure models in public health research and clinical trials.
Discrete Choice Methods With Simulation
DOWNLOAD
Author : Kenneth Train
language : en
Publisher: Cambridge University Press
Release Date : 2009-07-06
Discrete Choice Methods With Simulation written by Kenneth Train and has been published by Cambridge University Press this book supported file pdf, txt, epub, kindle and other format this book has been release on 2009-07-06 with Business & Economics categories.
This book describes the new generation of discrete choice methods, focusing on the many advances that are made possible by simulation. Researchers use these statistical methods to examine the choices that consumers, households, firms, and other agents make. Each of the major models is covered: logit, generalized extreme value, or GEV (including nested and cross-nested logits), probit, and mixed logit, plus a variety of specifications that build on these basics. Simulation-assisted estimation procedures are investigated and compared, including maximum stimulated likelihood, method of simulated moments, and method of simulated scores. Procedures for drawing from densities are described, including variance reduction techniques such as anithetics and Halton draws. Recent advances in Bayesian procedures are explored, including the use of the Metropolis-Hastings algorithm and its variant Gibbs sampling. The second edition adds chapters on endogeneity and expectation-maximization (EM) algorithms. No other book incorporates all these fields, which have arisen in the past 25 years. The procedures are applicable in many fields, including energy, transportation, environmental studies, health, labor, and marketing.
Analysis Of Clinical Trials Using Sas
DOWNLOAD
Author : Alex Dmitrienko
language : en
Publisher: SAS Institute
Release Date : 2017-07-17
Analysis Of Clinical Trials Using Sas written by Alex Dmitrienko and has been published by SAS Institute this book supported file pdf, txt, epub, kindle and other format this book has been release on 2017-07-17 with Computers categories.
Analysis of Clinical Trials Using SAS®: A Practical Guide, Second Edition bridges the gap between modern statistical methodology and real-world clinical trial applications. Tutorial material and step-by-step instructions illustrated with examples from actual trials serve to define relevant statistical approaches, describe their clinical trial applications, and implement the approaches rapidly and efficiently using the power of SAS. Topics reflect the International Conference on Harmonization (ICH) guidelines for the pharmaceutical industry and address important statistical problems encountered in clinical trials. Commonly used methods are covered, including dose-escalation and dose-finding methods that are applied in Phase I and Phase II clinical trials, as well as important trial designs and analysis strategies that are employed in Phase II and Phase III clinical trials, such as multiplicity adjustment, data monitoring, and methods for handling incomplete data. This book also features recommendations from clinical trial experts and a discussion of relevant regulatory guidelines. This new edition includes more examples and case studies, new approaches for addressing statistical problems, and the following new technological updates: SAS procedures used in group sequential trials (PROC SEQDESIGN and PROC SEQTEST) SAS procedures used in repeated measures analysis (PROC GLIMMIX and PROC GEE) macros for implementing a broad range of randomization-based methods in clinical trials, performing complex multiplicity adjustments, and investigating the design and analysis of early phase trials (Phase I dose-escalation trials and Phase II dose-finding trials) Clinical statisticians, research scientists, and graduate students in biostatistics will greatly benefit from the decades of clinical research experience and the ready-to-use SAS macros compiled in this book.