Generalized Low Rank Models
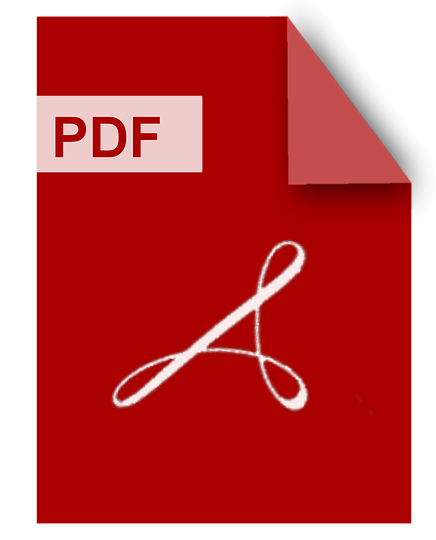
DOWNLOAD eBooks
Download Generalized Low Rank Models PDF/ePub or read online books in Mobi eBooks. Click Download or Read Online button to get Generalized Low Rank Models book now. This website allows unlimited access to, at the time of writing, more than 1.5 million titles, including hundreds of thousands of titles in various foreign languages. If the content not found or just blank you must refresh this page
Generalized Low Rank Models
DOWNLOAD eBooks
Author : Madeleine Udell
language : en
Publisher:
Release Date : 2016-05-03
Generalized Low Rank Models written by Madeleine Udell and has been published by this book supported file pdf, txt, epub, kindle and other format this book has been release on 2016-05-03 with categories.
Principal components analysis (PCA) is a well-known technique for approximating a tabular data set by a low rank matrix. Here, we extend the idea of PCA to handle arbitrary data sets consisting of numerical, Boolean, categorical, ordinal, and other data types. This framework encompasses many well-known techniques in data analysis, such as nonnegative matrix factorization, matrix completion, sparse and robust PCA, k-means, k-SVD, and maximum margin matrix factorization. The method handles heterogeneous data sets, and leads to coherent schemes for compressing, denoising, and imputing missing entries across all data types simultaneously. It also admits a number of interesting interpretations of the low rank factors, which allow clustering of examples or of features. We propose several parallel algorithms for fitting generalized low rank models, and describe implementations and numerical results.
Generalized Low Rank Models
DOWNLOAD eBooks
Author : Madeleine Udell
language : en
Publisher:
Release Date : 2016
Generalized Low Rank Models written by Madeleine Udell and has been published by this book supported file pdf, txt, epub, kindle and other format this book has been release on 2016 with Principal components analysis categories.
Principal components analysis (PCA) is a well-known technique for approximating a tabular data set by a low rank matrix. Here, we extend the idea of PCA to handle arbitrary data sets consisting of numerical, Boolean, categorical, ordinal, and other data types. This framework encompasses many well-known techniques in data analysis, such as nonnegative matrix factorization, matrix completion, sparse and robust PCA, k-means, k-SVD, and maximum margin matrix factorization. The method handles heterogeneous data sets, and leads to coherent schemes for compressing, denoising, and imputing missing entries across all data types simultaneously. It also admits a number of interesting interpretations of the low rank factors, which allow clustering of examples or of features. We propose several parallel algorithms for fitting generalized low rank models, and describe implementations and numerical results.
Generalized Low Rank Models
DOWNLOAD eBooks
Author : Madeleine Udell
language : en
Publisher:
Release Date : 2015
Generalized Low Rank Models written by Madeleine Udell and has been published by this book supported file pdf, txt, epub, kindle and other format this book has been release on 2015 with categories.
Principal components analysis (PCA) is a well-known technique for approximating a tabular data set by a low rank matrix. This dissertation extends the idea of PCA to handle arbitrary data sets consisting of numerical, Boolean, categorical, ordinal, and other data types. This framework encompasses many well known techniques in data analysis, such as nonnegative matrix factorization, matrix completion, sparse and robust PCA, k-means, k-SVD, and maximum margin matrix factorization. The method handles heterogeneous data sets, and leads to coherent schemes for compressing, denoising, and imputing missing entries across all data types simultaneously. It also admits a number of interesting interpretations of the low rank factors, which allow clustering of examples or of features. We propose several parallel algorithms for fitting generalized low rank models, and describe implementations and numerical results.
Ultra Dense Networks
DOWNLOAD eBooks
Author : Haijun Zhang
language : en
Publisher: Cambridge University Press
Release Date : 2020-11-26
Ultra Dense Networks written by Haijun Zhang and has been published by Cambridge University Press this book supported file pdf, txt, epub, kindle and other format this book has been release on 2020-11-26 with Computers categories.
Understand the theory, key technologies and applications of UDNs with this authoritative survey.
Multivariate Reduced Rank Regression
DOWNLOAD eBooks
Author : Gregory C. Reinsel
language : en
Publisher: Springer Nature
Release Date : 2022-11-30
Multivariate Reduced Rank Regression written by Gregory C. Reinsel and has been published by Springer Nature this book supported file pdf, txt, epub, kindle and other format this book has been release on 2022-11-30 with Mathematics categories.
This book provides an account of multivariate reduced-rank regression, a tool of multivariate analysis that enjoys a broad array of applications. In addition to a historical review of the topic, its connection to other widely used statistical methods, such as multivariate analysis of variance (MANOVA), discriminant analysis, principal components, canonical correlation analysis, and errors-in-variables models, is also discussed. This new edition incorporates Big Data methodology and its applications, as well as high-dimensional reduced-rank regression, generalized reduced-rank regression with complex data, and sparse and low-rank regression methods. Each chapter contains developments of basic theoretical results, as well as details on computational procedures, illustrated with numerical examples drawn from disciplines such as biochemistry, genetics, marketing, and finance. This book is designed for advanced students, practitioners, and researchers, who may deal with moderate and high-dimensional multivariate data. Because regression is one of the most popular statistical methods, the multivariate regression analysis tools described should provide a natural way of looking at large (both cross-sectional and chronological) data sets. This book can be assigned in seminar-type courses taken by advanced graduate students in statistics, machine learning, econometrics, business, and engineering.
Low Rank Models In Visual Analysis
DOWNLOAD eBooks
Author : Zhouchen Lin
language : en
Publisher: Academic Press
Release Date : 2017-06-06
Low Rank Models In Visual Analysis written by Zhouchen Lin and has been published by Academic Press this book supported file pdf, txt, epub, kindle and other format this book has been release on 2017-06-06 with Computers categories.
Low-Rank Models in Visual Analysis: Theories, Algorithms, and Applications presents the state-of-the-art on low-rank models and their application to visual analysis. It provides insight into the ideas behind the models and their algorithms, giving details of their formulation and deduction. The main applications included are video denoising, background modeling, image alignment and rectification, motion segmentation, image segmentation and image saliency detection. Readers will learn which Low-rank models are highly useful in practice (both linear and nonlinear models), how to solve low-rank models efficiently, and how to apply low-rank models to real problems. Presents a self-contained, up-to-date introduction that covers underlying theory, algorithms and the state-of-the-art in current applications Provides a full and clear explanation of the theory behind the models Includes detailed proofs in the appendices
Biocomputing 2016
DOWNLOAD eBooks
Author : Russ B Altman
language : en
Publisher: World Scientific Publishing Company
Release Date : 2015-11-19
Biocomputing 2016 written by Russ B Altman and has been published by World Scientific Publishing Company this book supported file pdf, txt, epub, kindle and other format this book has been release on 2015-11-19 with Science categories.
The Pacific Symposium on Biocomputing (PSB) 2016 is an international, multidisciplinary conference for the presentation and discussion of current research in the theory and application of computational methods in problems of biological significance. Presentations are rigorously peer reviewed and are published in an archival proceedings volume. PSB 2016 will be held on January 4 – 8, 2016 in Kohala Coast, Hawaii. Tutorials and workshops will be offered prior to the start of the conference. PSB 2016 will bring together top researchers from the US, the Asian Pacific nations, and around the world to exchange research results and address open issues in all aspects of computational biology. It is a forum for the presentation of work in databases, algorithms, interfaces, visualization, modeling, and other computational methods, as applied to biological problems, with emphasis on applications in data-rich areas of molecular biology. The PSB has been designed to be responsive to the need for critical mass in sub-disciplines within biocomputing. For that reason, it is the only meeting whose sessions are defined dynamically each year in response to specific proposals. PSB sessions are organized by leaders of research in biocomputing's "hot topics." In this way, the meeting provides an early forum for serious examination of emerging methods and approaches in this rapidly changing field.
Biocomputing 2016 Proceedings Of The Pacific Symposium
DOWNLOAD eBooks
Author : Russ B Altman
language : en
Publisher: World Scientific
Release Date : 2015-11-19
Biocomputing 2016 Proceedings Of The Pacific Symposium written by Russ B Altman and has been published by World Scientific this book supported file pdf, txt, epub, kindle and other format this book has been release on 2015-11-19 with Science categories.
The Pacific Symposium on Biocomputing (PSB) 2016 is an international, multidisciplinary conference for the presentation and discussion of current research in the theory and application of computational methods in problems of biological significance. Presentations are rigorously peer reviewed and are published in an archival proceedings volume. PSB 2016 will be held on January 4 - 8, 2016 in Kohala Coast, Hawaii. Tutorials and workshops will be offered prior to the start of the conference.PSB 2016 will bring together top researchers from the US, the Asian Pacific nations, and around the world to exchange research results and address open issues in all aspects of computational biology. It is a forum for the presentation of work in databases, algorithms, interfaces, visualization, modeling, and other computational methods, as applied to biological problems, with emphasis on applications in data-rich areas of molecular biology.The PSB has been designed to be responsive to the need for critical mass in sub-disciplines within biocomputing. For that reason, it is the only meeting whose sessions are defined dynamically each year in response to specific proposals. PSB sessions are organized by leaders of research in biocomputing's 'hot topics.' In this way, the meeting provides an early forum for serious examination of emerging methods and approaches in this rapidly changing field.
Nordic Artificial Intelligence Research And Development
DOWNLOAD eBooks
Author : Evi Zouganeli
language : en
Publisher: Springer Nature
Release Date : 2023-02-01
Nordic Artificial Intelligence Research And Development written by Evi Zouganeli and has been published by Springer Nature this book supported file pdf, txt, epub, kindle and other format this book has been release on 2023-02-01 with Computers categories.
This book constitutes the refereed proceedings of the 4th Symposium of the Norwegian AI Society, NAIS 2022, held in Oslo, Norway, during May 31–June 1, 2022. The 11 full papers included in this book were carefully reviewed and selected from 17 submissions. They were organized in topical sections as follows: robotics and intelligent systems; ai in cyber and digital sphere; ai in biological applications and medicine; and towards new ai methods. This is an open access book.
Big Data In Omics And Imaging
DOWNLOAD eBooks
Author : Momiao Xiong
language : en
Publisher: CRC Press
Release Date : 2018-06-14
Big Data In Omics And Imaging written by Momiao Xiong and has been published by CRC Press this book supported file pdf, txt, epub, kindle and other format this book has been release on 2018-06-14 with Mathematics categories.
Big Data in Omics and Imaging: Integrated Analysis and Causal Inference addresses the recent development of integrated genomic, epigenomic and imaging data analysis and causal inference in big data era. Despite significant progress in dissecting the genetic architecture of complex diseases by genome-wide association studies (GWAS), genome-wide expression studies (GWES), and epigenome-wide association studies (EWAS), the overall contribution of the new identified genetic variants is small and a large fraction of genetic variants is still hidden. Understanding the etiology and causal chain of mechanism underlying complex diseases remains elusive. It is time to bring big data, machine learning and causal revolution to developing a new generation of genetic analysis for shifting the current paradigm of genetic analysis from shallow association analysis to deep causal inference and from genetic analysis alone to integrated omics and imaging data analysis for unraveling the mechanism of complex diseases. FEATURES Provides a natural extension and companion volume to Big Data in Omic and Imaging: Association Analysis, but can be read independently. Introduce causal inference theory to genomic, epigenomic and imaging data analysis Develop novel statistics for genome-wide causation studies and epigenome-wide causation studies. Bridge the gap between the traditional association analysis and modern causation analysis Use combinatorial optimization methods and various causal models as a general framework for inferring multilevel omic and image causal networks Present statistical methods and computational algorithms for searching causal paths from genetic variant to disease Develop causal machine learning methods integrating causal inference and machine learning Develop statistics for testing significant difference in directed edge, path, and graphs, and for assessing causal relationships between two networks The book is designed for graduate students and researchers in genomics, epigenomics, medical image, bioinformatics, and data science. Topics covered are: mathematical formulation of causal inference, information geometry for causal inference, topology group and Haar measure, additive noise models, distance correlation, multivariate causal inference and causal networks, dynamic causal networks, multivariate and functional structural equation models, mixed structural equation models, causal inference with confounders, integer programming, deep learning and differential equations for wearable computing, genetic analysis of function-valued traits, RNA-seq data analysis, causal networks for genetic methylation analysis, gene expression and methylation deconvolution, cell –specific causal networks, deep learning for image segmentation and image analysis, imaging and genomic data analysis, integrated multilevel causal genomic, epigenomic and imaging data analysis.