Hessian Based Response Surface Approximations For Uncertainty Quantification In Large Scale Statistical Inverse Problems With Applications To Groundwater Flow
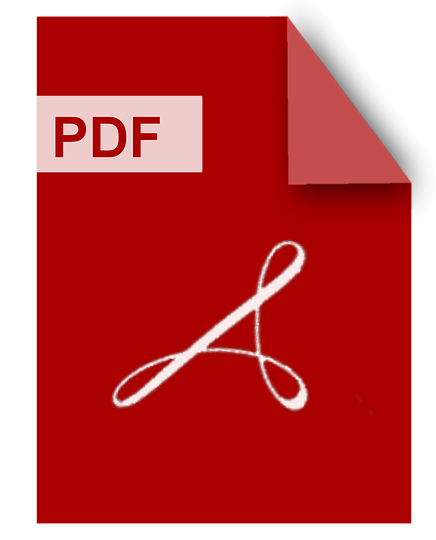
DOWNLOAD
Download Hessian Based Response Surface Approximations For Uncertainty Quantification In Large Scale Statistical Inverse Problems With Applications To Groundwater Flow PDF/ePub or read online books in Mobi eBooks. Click Download or Read Online button to get Hessian Based Response Surface Approximations For Uncertainty Quantification In Large Scale Statistical Inverse Problems With Applications To Groundwater Flow book now. This website allows unlimited access to, at the time of writing, more than 1.5 million titles, including hundreds of thousands of titles in various foreign languages. If the content not found or just blank you must refresh this page
Hessian Based Response Surface Approximations For Uncertainty Quantification In Large Scale Statistical Inverse Problems With Applications To Groundwater Flow
DOWNLOAD
Author : Hannah Pearl Flath
language : en
Publisher:
Release Date : 2013
Hessian Based Response Surface Approximations For Uncertainty Quantification In Large Scale Statistical Inverse Problems With Applications To Groundwater Flow written by Hannah Pearl Flath and has been published by this book supported file pdf, txt, epub, kindle and other format this book has been release on 2013 with categories.
Subsurface flow phenomena characterize many important societal issues in energy and the environment. A key feature of these problems is that subsurface properties are uncertain, due to the sparsity of direct observations of the subsurface. The Bayesian formulation of this inverse problem provides a systematic framework for inferring uncertainty in the properties given uncertainties in the data, the forward model, and prior knowledge of the properties. We address the problem: given noisy measurements of the head, the pdf describing the noise, prior information in the form of a pdf of the hydraulic conductivity, and a groundwater flow model relating the head to the hydraulic conductivity, find the posterior probability density function (pdf) of the parameters describing the hydraulic conductivity field. Unfortunately, conventional sampling of this pdf to compute statistical moments is intractable for problems governed by large-scale forward models and high-dimensional parameter spaces. We construct a Gaussian process surrogate of the posterior pdf based on Bayesian interpolation between a set of "training" points. We employ a greedy algorithm to find the training points by solving a sequence of optimization problems where each new training point is placed at the maximizer of the error in the approximation. Scalable Newton optimization methods solve this "optimal" training point problem. We tailor the Gaussian process surrogate to the curvature of the underlying posterior pdf according to the Hessian of the log posterior at a subset of training points, made computationally tractable by a low-rank approximation of the data misfit Hessian. A Gaussian mixture approximation of the posterior is extracted from the Gaussian process surrogate, and used as a proposal in a Markov chain Monte Carlo method for sampling both the surrogate as well as the true posterior. The Gaussian process surrogate is used as a first stage approximation in a two-stage delayed acceptance MCMC method. We provide evidence for the viability of the low-rank approximation of the Hessian through numerical experiments on a large scale atmospheric contaminant transport problem and analysis of an infinite dimensional model problem. We provide similar results for our groundwater problem. We then present results from the proposed MCMC algorithms.
Data Driven Uncertainty Quantification For Predictive Subsurface Flow And Transport Modeling
DOWNLOAD
Author : Jiachuan He
language : en
Publisher:
Release Date : 2018
Data Driven Uncertainty Quantification For Predictive Subsurface Flow And Transport Modeling written by Jiachuan He and has been published by this book supported file pdf, txt, epub, kindle and other format this book has been release on 2018 with categories.
Specification of hydraulic conductivity as a model parameter in groundwater flow and transport equations is an essential step in predictive simulations. It is often infeasible in practice to characterize this model parameter at all points in space due to complex hydrogeological environments leading to significant parameter uncertainties. Quantifying these uncertainties requires the formulation and solution of an inverse problem using data corresponding to observable model responses. Several types of inverse problems may be formulated under various physical and statistical assumptions on the model parameters, model response, and the data. Solutions to most types of inverse problems require large numbers of model evaluations. In this study, we incorporate the use of surrogate models based on support vector machines to increase the number of samples used in approximating a solution to an inverse problem at a relatively low computational cost. To test the global capabilities of this type of surrogate model for quantifying uncertainties, we use a framework for constructing pullback and push-forward probability measures to study the data-to-parameter-to-prediction propagation of uncertainties under minimal statistical assumptions. Additionally, we demonstrate that it is possible to build a support vector machine using relatively low-dimensional representations of the hydraulic conductivity to propagate distributions. The numerical examples further demonstrate that we can make reliable probabilistic predictions of contaminant concentration at spatial locations corresponding to data not used in the solution to the inverse problem. This dissertation is based on the article entitled Data-driven uncertainty quantification for predictive flow and transport modeling using support vector machines by Jiachuan He, Steven Mattis, Troy Butler and Clint Dawson [32]. This material is based upon work supported by the U.S. Department of Energy Office of Science, Office of Advanced Scientific Computing Research, Applied Mathematics program under Award Number DE-SC0009286 as part of the DiaMonD Multifaceted Mathematics Integrated Capability Center
Parameter Estimation And Uncertainty Quantification In Water Resources Modeling
DOWNLOAD
Author : Philippe Renard
language : en
Publisher: Frontiers Media SA
Release Date : 2020-04-22
Parameter Estimation And Uncertainty Quantification In Water Resources Modeling written by Philippe Renard and has been published by Frontiers Media SA this book supported file pdf, txt, epub, kindle and other format this book has been release on 2020-04-22 with categories.
Numerical models of flow and transport processes are heavily employed in the fields of surface, soil, and groundwater hydrology. They are used to interpret field observations, analyze complex and coupled processes, or to support decision making related to large societal issues such as the water-energy nexus or sustainable water management and food production. Parameter estimation and uncertainty quantification are two key features of modern science-based predictions. When applied to water resources, these tasks must cope with many degrees of freedom and large datasets. Both are challenging and require novel theoretical and computational approaches to handle complex models with large number of unknown parameters.
A Pde Constrained Optimization Approach To Uncertainty Quantification In Inverse Problems With Applications To Inverse Scattering
DOWNLOAD
Author :
language : en
Publisher:
Release Date : 2010
A Pde Constrained Optimization Approach To Uncertainty Quantification In Inverse Problems With Applications To Inverse Scattering written by and has been published by this book supported file pdf, txt, epub, kindle and other format this book has been release on 2010 with categories.
This project addressed the statistical inverse problem of reconstruction of an uncertain shape of a scatterer or properties of a medium from noisy observations of scattered wavefields. The Bayesian solution of this inverse problem yields a posterior pdf, requiring the solution of the forward wave equation to evaluate the probability of any point in parameter space. The standard approach is to sample this pdf via an MCMC method and then compute statistics of the samples. However, standard MCMC methods view the underlying parameter-to-observable map as a black box, and thus do not exploit its structure, becoming prohibitive for high dimensional parameter spaces and expensive simulations. A preconditioned Langevin-accelerated MCMC method for sampling high-dimensional PDE-based probability densities was developed. The preconditioner exploits local Hessian (of the negative log posterior) information to greatly speed up sampling, leading to a stochastic version of Newton's method. Fast Hessian approximations were developed for several inverse scattering problems. Applications to model inverse medium scattering problems indicated several orders of magnitude improvement over a reference black-box MCMC method.
Optimal Calibration Uncertainty Assessment And Long Term Monitoring Using Computationally Expensive Groundwater Models
DOWNLOAD
Author : Pradeep Mugunthan
language : en
Publisher:
Release Date : 2005
Optimal Calibration Uncertainty Assessment And Long Term Monitoring Using Computationally Expensive Groundwater Models written by Pradeep Mugunthan and has been published by this book supported file pdf, txt, epub, kindle and other format this book has been release on 2005 with categories.
The Nth Order Comprehensive Adjoint Sensitivity Analysis Methodology Volume Ii
DOWNLOAD
Author : Dan Gabriel Cacuci
language : en
Publisher:
Release Date : 2023
The Nth Order Comprehensive Adjoint Sensitivity Analysis Methodology Volume Ii written by Dan Gabriel Cacuci and has been published by this book supported file pdf, txt, epub, kindle and other format this book has been release on 2023 with categories.
This text describes a comprehensive adjoint sensitivity analysis methodology (C-ASAM), developed by the author, enabling the efficient and exact computation of arbitrarily high-order functional derivatives of model responses to model parameters in large-scale systems. The C-ASAM framework is set in linearly increasing Hilbert spaces, each of state-function-dimensionality, as opposed to exponentially increasing parameter-dimensional spaces, thereby breaking the so-called "curse of dimensionality" in sensitivity and uncertainty analysis. The C-ASAM applies to any model; the larger the number of model parameters, the more efficient the C-ASAM becomes for computing arbitrarily high-order response sensitivities. The book will be helpful to those working in the fields of sensitivity analysis, uncertainty quantification, model validation, optimization, data assimilation, model calibration, sensor fusion, reduced-order modelling, inverse problems and predictive modelling. This Volume Two, the second of three, presents the large-scale application of C-ASAM to compute exactly the first-, second-, third-, and fourth-order sensitivities of the Polyethylene-Reflected Plutonium (PERP) OECD/NEO international benchmark which is modeled mathematically by the Boltzmann particle transport equation. It follows from the description of the C-ASAM framework applied to linear systems in Volume One where the PERP benchmark's response of interest is the leakage of particles through its outer boundary. The benchmark represents the largest sensitivity analysis endeavor ever carried out in the field of reactor physics and the numerical results shown in this book prove, for the first time ever, that many of the second-order sensitivities are much larger than the corresponding first-order ones. Currently, the nth-CASAM is the only known methodology which enables such large-scale computations of the exact expressions and values of the nth-order response sensitivities.
Data Driven Uncertainty Quantification For Large Scale Simulations
DOWNLOAD
Author : Fabian Franzelin
language : en
Publisher:
Release Date : 2018
Data Driven Uncertainty Quantification For Large Scale Simulations written by Fabian Franzelin and has been published by this book supported file pdf, txt, epub, kindle and other format this book has been release on 2018 with categories.
Active Subspaces
DOWNLOAD
Author : Paul G. Constantine
language : en
Publisher: SIAM
Release Date : 2015-03-17
Active Subspaces written by Paul G. Constantine and has been published by SIAM this book supported file pdf, txt, epub, kindle and other format this book has been release on 2015-03-17 with Computers categories.
Scientists and engineers use computer simulations to study relationships between a model's input parameters and its outputs. However, thorough parameter studies are challenging, if not impossible, when the simulation is expensive and the model has several inputs. To enable studies in these instances, the engineer may attempt to reduce the dimension of the model's input parameter space. Active subspaces are an emerging set of dimension reduction tools that identify important directions in the parameter space. This book describes techniques for discovering a model's active subspace and proposes methods for exploiting the reduced dimension to enable otherwise infeasible parameter studies. Readers will find new ideas for dimension reduction, easy-to-implement algorithms, and several examples of active subspaces in action.
Large Scale Inverse Problems
DOWNLOAD
Author : Mike Cullen
language : en
Publisher: Walter de Gruyter
Release Date : 2013-08-29
Large Scale Inverse Problems written by Mike Cullen and has been published by Walter de Gruyter this book supported file pdf, txt, epub, kindle and other format this book has been release on 2013-08-29 with Mathematics categories.
This book is thesecond volume of a three volume series recording the "Radon Special Semester 2011 on Multiscale Simulation & Analysis in Energy and the Environment" that took placein Linz, Austria, October 3-7, 2011. This volume addresses the common ground in the mathematical and computational procedures required for large-scale inverse problems and data assimilation in forefront applications. The solution of inverse problems is fundamental to a wide variety of applications such as weather forecasting, medical tomography, and oil exploration. Regularisation techniques are needed to ensure solutions of sufficient quality to be useful, and soundly theoretically based. This book addresses the common techniques required for all the applications, and is thus truly interdisciplinary. Thiscollection of surveyarticlesfocusses onthe large inverse problems commonly arising in simulation and forecasting in the earth sciences. For example, operational weather forecasting models have between 107 and 108 degrees of freedom. Even so, these degrees of freedom represent grossly space-time averaged properties of the atmosphere. Accurate forecasts require accurate initial conditions. With recent developments in satellite data, there are between 106 and 107 observations each day. However, while these also represent space-time averaged properties, the averaging implicit in the measurements is quite different from that used in the models. In atmosphere and ocean applications, there is a physically-based model available which can be used to regularise the problem. We assume that there is a set of observations with known error characteristics available over a period of time. The basic deterministic technique is to fit a model trajectory to the observations over a period of time to within the observation error. Since the model is not perfect the model trajectory has to be corrected, which defines the data assimilation problem. The stochastic view can be expressed by using an ensemble of model trajectories, and calculating corrections to both the mean value and the spread which allow the observations to be fitted by each ensemble member. In other areas of earth science, only the structure of the model formulation itself is known and the aim is to use the past observation history to determine the unknown model parameters. The book records the achievements of Workshop2 "Large-Scale Inverse Problems and Applications in the Earth Sciences". Itinvolves experts in the theory of inverse problems together with experts working on both theoretical and practical aspects of the techniques by which large inverse problems arise in the earth sciences.
Multiple Point Geostatistics
DOWNLOAD
Author : Professor Gregoire Mariethoz
language : en
Publisher: John Wiley & Sons
Release Date : 2014-10-16
Multiple Point Geostatistics written by Professor Gregoire Mariethoz and has been published by John Wiley & Sons this book supported file pdf, txt, epub, kindle and other format this book has been release on 2014-10-16 with Science categories.
This book provides a comprehensive introduction to multiple-point geostatistics, where spatial continuity is described using training images. Multiple-point geostatistics aims at bridging the gap between physical modelling/realism and spatio-temporal stochastic modelling. The book provides an overview of this new field in three parts. Part I presents a conceptual comparison between traditional random function theory and stochastic modelling based on training images, where random function theory is not always used. Part II covers in detail various algorithms and methodologies starting from basic building blocks in statistical science and computer science. Concepts such as non-stationary and multi-variate modeling, consistency between data and model, the construction of training images and inverse modelling are treated. Part III covers three example application areas, namely, reservoir modelling, mineral resources modelling and climate model downscaling. This book will be an invaluable reference for students, researchers and practitioners of all areas of the Earth Sciences where forecasting based on spatio-temporal data is performed.