Information Bounds And Nonparametric Maximum Likelihood Estimation
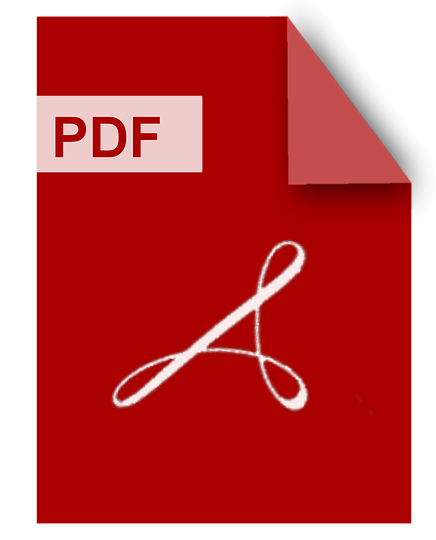
DOWNLOAD
Download Information Bounds And Nonparametric Maximum Likelihood Estimation PDF/ePub or read online books in Mobi eBooks. Click Download or Read Online button to get Information Bounds And Nonparametric Maximum Likelihood Estimation book now. This website allows unlimited access to, at the time of writing, more than 1.5 million titles, including hundreds of thousands of titles in various foreign languages. If the content not found or just blank you must refresh this page
Information Bounds And Nonparametric Maximum Likelihood Estimation
DOWNLOAD
Author : P. Groeneboom
language : en
Publisher: Birkhäuser
Release Date : 2012-12-06
Information Bounds And Nonparametric Maximum Likelihood Estimation written by P. Groeneboom and has been published by Birkhäuser this book supported file pdf, txt, epub, kindle and other format this book has been release on 2012-12-06 with Mathematics categories.
This book contains the lecture notes for a DMV course presented by the authors at Gunzburg, Germany, in September, 1990. In the course we sketched the theory of information bounds for non parametric and semiparametric models, and developed the theory of non parametric maximum likelihood estimation in several particular inverse problems: interval censoring and deconvolution models. Part I, based on Jon Wellner's lectures, gives a brief sketch of information lower bound theory: Hajek's convolution theorem and extensions, useful minimax bounds for parametric problems due to Ibragimov and Has'minskii, and a recent result characterizing differentiable functionals due to van der Vaart (1991). The differentiability theorem is illustrated with the examples of interval censoring and deconvolution (which are pursued from the estimation perspective in part II). The differentiability theorem gives a way of clearly distinguishing situations in which 1 2 the parameter of interest can be estimated at rate n / and situations in which this is not the case. However it says nothing about which rates to expect when the functional is not differentiable. Even the casual reader will notice that several models are introduced, but not pursued in any detail; many problems remain. Part II, based on Piet Groeneboom's lectures, focuses on non parametric maximum likelihood estimates (NPMLE's) for certain inverse problems. The first chapter deals with the interval censoring problem.
Information Bounds And Nonparametric Maximum Likelihood Estimation
DOWNLOAD
Author : P. Groeneboom
language : en
Publisher: Springer Science & Business Media
Release Date : 1992-07-31
Information Bounds And Nonparametric Maximum Likelihood Estimation written by P. Groeneboom and has been published by Springer Science & Business Media this book supported file pdf, txt, epub, kindle and other format this book has been release on 1992-07-31 with Mathematics categories.
This book contains the lecture notes for a DMV course presented by the authors at Gunzburg, Germany, in September, 1990. In the course we sketched the theory of information bounds for non parametric and semiparametric models, and developed the theory of non parametric maximum likelihood estimation in several particular inverse problems: interval censoring and deconvolution models. Part I, based on Jon Wellner's lectures, gives a brief sketch of information lower bound theory: Hajek's convolution theorem and extensions, useful minimax bounds for parametric problems due to Ibragimov and Has'minskii, and a recent result characterizing differentiable functionals due to van der Vaart (1991). The differentiability theorem is illustrated with the examples of interval censoring and deconvolution (which are pursued from the estimation perspective in part II). The differentiability theorem gives a way of clearly distinguishing situations in which 1 2 the parameter of interest can be estimated at rate n / and situations in which this is not the case. However it says nothing about which rates to expect when the functional is not differentiable. Even the casual reader will notice that several models are introduced, but not pursued in any detail; many problems remain. Part II, based on Piet Groeneboom's lectures, focuses on non parametric maximum likelihood estimates (NPMLE's) for certain inverse problems. The first chapter deals with the interval censoring problem.
Information Bounds And Nonparametric Maximum Likelihood Estimation
DOWNLOAD
Author : P. Groeneboom
language : en
Publisher: Birkhauser
Release Date : 1992
Information Bounds And Nonparametric Maximum Likelihood Estimation written by P. Groeneboom and has been published by Birkhauser this book supported file pdf, txt, epub, kindle and other format this book has been release on 1992 with Mathematics categories.
Maximum Likelihood Estimation
DOWNLOAD
Author : Scott R. Eliason
language : en
Publisher: SAGE
Release Date : 1993
Maximum Likelihood Estimation written by Scott R. Eliason and has been published by SAGE this book supported file pdf, txt, epub, kindle and other format this book has been release on 1993 with Mathematics categories.
This is a short introduction to Maximum Likelihood (ML) Estimation. It provides a general modeling framework that utilizes the tools of ML methods to outline a flexible modeling strategy that accommodates cases from the simplest linear models (such as the normal error regression model) to the most complex nonlinear models linking endogenous and exogenous variables with non-normal distributions. Using examples to illustrate the techniques of finding ML estimators and estimates, the author discusses what properties are desirable in an estimator, basic techniques for finding maximum likelihood solutions, the general form of the covariance matrix for ML estimates, the sampling distribution of ML estimators; the use of ML in the normal as well as other distributions, and some useful illustrations of likelihoods.
Maximum Penalized Likelihood Estimation
DOWNLOAD
Author : P.P.B. Eggermont
language : en
Publisher: Springer Nature
Release Date : 2020-12-15
Maximum Penalized Likelihood Estimation written by P.P.B. Eggermont and has been published by Springer Nature this book supported file pdf, txt, epub, kindle and other format this book has been release on 2020-12-15 with Mathematics categories.
This book deals with parametric and nonparametric density estimation from the maximum (penalized) likelihood point of view, including estimation under constraints. The focal points are existence and uniqueness of the estimators, almost sure convergence rates for the L1 error, and data-driven smoothing parameter selection methods, including their practical performance. The reader will gain insight into technical tools from probability theory and applied mathematics.
Exact Maximum Likelihood Estimation Of Observation Driven Econometric Models
DOWNLOAD
Author : Francis X. Diebold
language : en
Publisher:
Release Date : 1996
Exact Maximum Likelihood Estimation Of Observation Driven Econometric Models written by Francis X. Diebold and has been published by this book supported file pdf, txt, epub, kindle and other format this book has been release on 1996 with Econometric models categories.
The possibility of exact maximum likelihood estimation of many observation-driven models remains an open question. Often only approximate maximum likelihood estimation is attempted, because the unconditional density needed for exact estimation is not known in closed form. Using simulation and nonparametric density estimation techniques that facilitate empirical likelihood evaluation, we develop an exact maximum likelihood procedure. We provide an illustrative application to the estimation of ARCH models, in which we compare the sampling properties of the exact estimator to those of several competitors. We find that, especially in situations of small samples and high persistence, efficiency gains are obtained. We conclude with a discussion of directions for future research, including application of our methods to panel data models.
Empirical Likelihood
DOWNLOAD
Author : Art B. Owen
language : en
Publisher: CRC Press
Release Date : 2001-05-18
Empirical Likelihood written by Art B. Owen and has been published by CRC Press this book supported file pdf, txt, epub, kindle and other format this book has been release on 2001-05-18 with Mathematics categories.
Empirical likelihood provides inferences whose validity does not depend on specifying a parametric model for the data. Because it uses a likelihood, the method has certain inherent advantages over resampling methods: it uses the data to determine the shape of the confidence regions, and it makes it easy to combined data from multiple sources. It al
Nonparametric Maximum Likelihood Estimator Based On Doubly Censored Data
DOWNLOAD
Author : Ting Li
language : en
Publisher:
Release Date : 1993
Nonparametric Maximum Likelihood Estimator Based On Doubly Censored Data written by Ting Li and has been published by this book supported file pdf, txt, epub, kindle and other format this book has been release on 1993 with categories.
Maximum Likelihood Estimation And Inference
DOWNLOAD
Author : Russell B. Millar
language : en
Publisher: John Wiley & Sons
Release Date : 2011-07-26
Maximum Likelihood Estimation And Inference written by Russell B. Millar and has been published by John Wiley & Sons this book supported file pdf, txt, epub, kindle and other format this book has been release on 2011-07-26 with Mathematics categories.
This book takes a fresh look at the popular and well-established method of maximum likelihood for statistical estimation and inference. It begins with an intuitive introduction to the concepts and background of likelihood, and moves through to the latest developments in maximum likelihood methodology, including general latent variable models and new material for the practical implementation of integrated likelihood using the free ADMB software. Fundamental issues of statistical inference are also examined, with a presentation of some of the philosophical debates underlying the choice of statistical paradigm. Key features: Provides an accessible introduction to pragmatic maximum likelihood modelling. Covers more advanced topics, including general forms of latent variable models (including non-linear and non-normal mixed-effects and state-space models) and the use of maximum likelihood variants, such as estimating equations, conditional likelihood, restricted likelihood and integrated likelihood. Adopts a practical approach, with a focus on providing the relevant tools required by researchers and practitioners who collect and analyze real data. Presents numerous examples and case studies across a wide range of applications including medicine, biology and ecology. Features applications from a range of disciplines, with implementation in R, SAS and/or ADMB. Provides all program code and software extensions on a supporting website. Confines supporting theory to the final chapters to maintain a readable and pragmatic focus of the preceding chapters. This book is not just an accessible and practical text about maximum likelihood, it is a comprehensive guide to modern maximum likelihood estimation and inference. It will be of interest to readers of all levels, from novice to expert. It will be of great benefit to researchers, and to students of statistics from senior undergraduate to graduate level. For use as a course text, exercises are provided at the end of each chapter.
Maximum Likelihood Estimation A Practical Theorem On Consistency Of The Nonparametric Maximum Likelihood Estimates With Applications
DOWNLOAD
Author :
language : en
Publisher:
Release Date : 1967
Maximum Likelihood Estimation A Practical Theorem On Consistency Of The Nonparametric Maximum Likelihood Estimates With Applications written by and has been published by this book supported file pdf, txt, epub, kindle and other format this book has been release on 1967 with categories.
Sufficient conditions for consistency of a nonparametric maximum likelihood estimate are given which are applicable to those problems where a class of distribution functions is specified only in terms of its graphs. Consistency is proven and applications are given. (Author).