Introduction To Probability Simulation And Gibbs Sampling With R
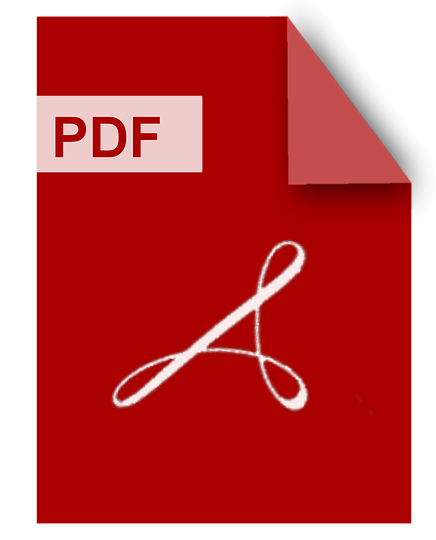
DOWNLOAD
Download Introduction To Probability Simulation And Gibbs Sampling With R PDF/ePub or read online books in Mobi eBooks. Click Download or Read Online button to get Introduction To Probability Simulation And Gibbs Sampling With R book now. This website allows unlimited access to, at the time of writing, more than 1.5 million titles, including hundreds of thousands of titles in various foreign languages. If the content not found or just blank you must refresh this page
Introduction To Probability Simulation And Gibbs Sampling With R
DOWNLOAD
Author : Eric A. Suess
language : en
Publisher: Springer Science & Business Media
Release Date : 2010-05-27
Introduction To Probability Simulation And Gibbs Sampling With R written by Eric A. Suess and has been published by Springer Science & Business Media this book supported file pdf, txt, epub, kindle and other format this book has been release on 2010-05-27 with Mathematics categories.
The first seven chapters use R for probability simulation and computation, including random number generation, numerical and Monte Carlo integration, and finding limiting distributions of Markov Chains with both discrete and continuous states. Applications include coverage probabilities of binomial confidence intervals, estimation of disease prevalence from screening tests, parallel redundancy for improved reliability of systems, and various kinds of genetic modeling. These initial chapters can be used for a non-Bayesian course in the simulation of applied probability models and Markov Chains. Chapters 8 through 10 give a brief introduction to Bayesian estimation and illustrate the use of Gibbs samplers to find posterior distributions and interval estimates, including some examples in which traditional methods do not give satisfactory results. WinBUGS software is introduced with a detailed explanation of its interface and examples of its use for Gibbs sampling for Bayesian estimation. No previous experience using R is required. An appendix introduces R, and complete R code is included for almost all computational examples and problems (along with comments and explanations). Noteworthy features of the book are its intuitive approach, presenting ideas with examples from biostatistics, reliability, and other fields; its large number of figures; and its extraordinarily large number of problems (about a third of the pages), ranging from simple drill to presentation of additional topics. Hints and answers are provided for many of the problems. These features make the book ideal for students of statistics at the senior undergraduate and at the beginning graduate levels.
Introduction To Probability Simulation And Gibbs Sampling With R
DOWNLOAD
Author : Eric A. Suess
language : en
Publisher: Springer Science & Business Media
Release Date : 2010-06-15
Introduction To Probability Simulation And Gibbs Sampling With R written by Eric A. Suess and has been published by Springer Science & Business Media this book supported file pdf, txt, epub, kindle and other format this book has been release on 2010-06-15 with Mathematics categories.
The first seven chapters use R for probability simulation and computation, including random number generation, numerical and Monte Carlo integration, and finding limiting distributions of Markov Chains with both discrete and continuous states. Applications include coverage probabilities of binomial confidence intervals, estimation of disease prevalence from screening tests, parallel redundancy for improved reliability of systems, and various kinds of genetic modeling. These initial chapters can be used for a non-Bayesian course in the simulation of applied probability models and Markov Chains. Chapters 8 through 10 give a brief introduction to Bayesian estimation and illustrate the use of Gibbs samplers to find posterior distributions and interval estimates, including some examples in which traditional methods do not give satisfactory results. WinBUGS software is introduced with a detailed explanation of its interface and examples of its use for Gibbs sampling for Bayesian estimation. No previous experience using R is required. An appendix introduces R, and complete R code is included for almost all computational examples and problems (along with comments and explanations). Noteworthy features of the book are its intuitive approach, presenting ideas with examples from biostatistics, reliability, and other fields; its large number of figures; and its extraordinarily large number of problems (about a third of the pages), ranging from simple drill to presentation of additional topics. Hints and answers are provided for many of the problems. These features make the book ideal for students of statistics at the senior undergraduate and at the beginning graduate levels.
Bayesian Computation With R
DOWNLOAD
Author : Jim Albert
language : en
Publisher: Springer Science & Business Media
Release Date : 2009-04-20
Bayesian Computation With R written by Jim Albert and has been published by Springer Science & Business Media this book supported file pdf, txt, epub, kindle and other format this book has been release on 2009-04-20 with Mathematics categories.
There has been dramatic growth in the development and application of Bayesian inference in statistics. Berger (2000) documents the increase in Bayesian activity by the number of published research articles, the number of books,andtheextensivenumberofapplicationsofBayesianarticlesinapplied disciplines such as science and engineering. One reason for the dramatic growth in Bayesian modeling is the availab- ity of computational algorithms to compute the range of integrals that are necessary in a Bayesian posterior analysis. Due to the speed of modern c- puters, it is now possible to use the Bayesian paradigm to ?t very complex models that cannot be ?t by alternative frequentist methods. To ?t Bayesian models, one needs a statistical computing environment. This environment should be such that one can: write short scripts to de?ne a Bayesian model use or write functions to summarize a posterior distribution use functions to simulate from the posterior distribution construct graphs to illustrate the posterior inference An environment that meets these requirements is the R system. R provides a wide range of functions for data manipulation, calculation, and graphical d- plays. Moreover, it includes a well-developed, simple programming language that users can extend by adding new functions. Many such extensions of the language in the form of packages are easily downloadable from the Comp- hensive R Archive Network (CRAN).
Mathematical Statistics With Resampling And R
DOWNLOAD
Author : Laura M. Chihara
language : en
Publisher: John Wiley & Sons
Release Date : 2012-09-05
Mathematical Statistics With Resampling And R written by Laura M. Chihara and has been published by John Wiley & Sons this book supported file pdf, txt, epub, kindle and other format this book has been release on 2012-09-05 with Mathematics categories.
This book bridges the latest software applications with the benefits of modern resampling techniques Resampling helps students understand the meaning of sampling distributions, sampling variability, P-values, hypothesis tests, and confidence intervals. This groundbreaking book shows how to apply modern resampling techniques to mathematical statistics. Extensively class-tested to ensure an accessible presentation, Mathematical Statistics with Resampling and R utilizes the powerful and flexible computer language R to underscore the significance and benefits of modern resampling techniques. The book begins by introducing permutation tests and bootstrap methods, motivating classical inference methods. Striking a balance between theory, computing, and applications, the authors explore additional topics such as: Exploratory data analysis Calculation of sampling distributions The Central Limit Theorem Monte Carlo sampling Maximum likelihood estimation and properties of estimators Confidence intervals and hypothesis tests Regression Bayesian methods Throughout the book, case studies on diverse subjects such as flight delays, birth weights of babies, and telephone company repair times illustrate the relevance of the real-world applications of the discussed material. Key definitions and theorems of important probability distributions are collected at the end of the book, and a related website is also available, featuring additional material including data sets, R scripts, and helpful teaching hints. Mathematical Statistics with Resampling and R is an excellent book for courses on mathematical statistics at the upper-undergraduate and graduate levels. It also serves as a valuable reference for applied statisticians working in the areas of business, economics, biostatistics, and public health who utilize resampling methods in their everyday work.
Probability And Statistics With R
DOWNLOAD
Author : Maria Dolores Ugarte
language : en
Publisher: CRC Press
Release Date : 2015-07-21
Probability And Statistics With R written by Maria Dolores Ugarte and has been published by CRC Press this book supported file pdf, txt, epub, kindle and other format this book has been release on 2015-07-21 with Mathematics categories.
Since the publication of the popular first edition, the contributed R packages on CRAN have increased from around 1,000 to over 6,000. This second edition explores how some of these new packages make analysis easier and more intuitive as well as create more visually pleasing graphs. Along with adding new examples and exercises, this edition improves the existing examples, problems, concepts, data, and functions. Data sets, R functions, and more are available online.
A Course In Statistics With R
DOWNLOAD
Author : Prabhanjan N. Tattar
language : en
Publisher: John Wiley & Sons
Release Date : 2016-05-02
A Course In Statistics With R written by Prabhanjan N. Tattar and has been published by John Wiley & Sons this book supported file pdf, txt, epub, kindle and other format this book has been release on 2016-05-02 with Computers categories.
Integrates the theory and applications of statistics using R A Course in Statistics with R has been written to bridge the gap between theory and applications and explain how mathematical expressions are converted into R programs. The book has been primarily designed as a useful companion for a Masters student during each semester of the course, but will also help applied statisticians in revisiting the underpinnings of the subject. With this dual goal in mind, the book begins with R basics and quickly covers visualization and exploratory analysis. Probability and statistical inference, inclusive of classical, nonparametric, and Bayesian schools, is developed with definitions, motivations, mathematical expression and R programs in a way which will help the reader to understand the mathematical development as well as R implementation. Linear regression models, experimental designs, multivariate analysis, and categorical data analysis are treated in a way which makes effective use of visualization techniques and the related statistical techniques underlying them through practical applications, and hence helps the reader to achieve a clear understanding of the associated statistical models. Key features: Integrates R basics with statistical concepts Provides graphical presentations inclusive of mathematical expressions Aids understanding of limit theorems of probability with and without the simulation approach Presents detailed algorithmic development of statistical models from scratch Includes practical applications with over 50 data sets
Introducing Monte Carlo Methods With R
DOWNLOAD
Author : Christian Robert
language : en
Publisher: Springer Science & Business Media
Release Date : 2010
Introducing Monte Carlo Methods With R written by Christian Robert and has been published by Springer Science & Business Media this book supported file pdf, txt, epub, kindle and other format this book has been release on 2010 with Computers categories.
This book covers the main tools used in statistical simulation from a programmer’s point of view, explaining the R implementation of each simulation technique and providing the output for better understanding and comparison.
Primer To Analysis Of Genomic Data Using R
DOWNLOAD
Author : Cedric Gondro
language : en
Publisher: Springer
Release Date : 2015-05-18
Primer To Analysis Of Genomic Data Using R written by Cedric Gondro and has been published by Springer this book supported file pdf, txt, epub, kindle and other format this book has been release on 2015-05-18 with Medical categories.
Through this book, researchers and students will learn to use R for analysis of large-scale genomic data and how to create routines to automate analytical steps. The philosophy behind the book is to start with real world raw datasets and perform all the analytical steps needed to reach final results. Though theory plays an important role, this is a practical book for graduate and undergraduate courses in bioinformatics and genomic analysis or for use in lab sessions. How to handle and manage high-throughput genomic data, create automated workflows and speed up analyses in R is also taught. A wide range of R packages useful for working with genomic data are illustrated with practical examples. The key topics covered are association studies, genomic prediction, estimation of population genetic parameters and diversity, gene expression analysis, functional annotation of results using publically available databases and how to work efficiently in R with large genomic datasets. Important principles are demonstrated and illustrated through engaging examples which invite the reader to work with the provided datasets. Some methods that are discussed in this volume include: signatures of selection, population parameters (LD, FST, FIS, etc); use of a genomic relationship matrix for population diversity studies; use of SNP data for parentage testing; snpBLUP and gBLUP for genomic prediction. Step-by-step, all the R code required for a genome-wide association study is shown: starting from raw SNP data, how to build databases to handle and manage the data, quality control and filtering measures, association testing and evaluation of results, through to identification and functional annotation of candidate genes. Similarly, gene expression analyses are shown using microarray and RNAseq data. At a time when genomic data is decidedly big, the skills from this book are critical. In recent years R has become the de facto tool for analysis of gene expression data, in addition to its prominent role in analysis of genomic data. Benefits to using R include the integrated development environment for analysis, flexibility and control of the analytic workflow. Included topics are core components of advanced undergraduate and graduate classes in bioinformatics, genomics and statistical genetics. This book is also designed to be used by students in computer science and statistics who want to learn the practical aspects of genomic analysis without delving into algorithmic details. The datasets used throughout the book may be downloaded from the publisher’s website.
Bayesian Models For Astrophysical Data
DOWNLOAD
Author : Joseph M. Hilbe
language : en
Publisher: Cambridge University Press
Release Date : 2017-04-27
Bayesian Models For Astrophysical Data written by Joseph M. Hilbe and has been published by Cambridge University Press this book supported file pdf, txt, epub, kindle and other format this book has been release on 2017-04-27 with Mathematics categories.
A hands-on guide to Bayesian models with R, JAGS, Python, and Stan code, for a wide range of astronomical data types.
Marketing Data Science
DOWNLOAD
Author : Thomas W. Miller
language : en
Publisher: FT Press
Release Date : 2015-05-02
Marketing Data Science written by Thomas W. Miller and has been published by FT Press this book supported file pdf, txt, epub, kindle and other format this book has been release on 2015-05-02 with Business & Economics categories.
Now, a leader of Northwestern University's prestigious analytics program presents a fully-integrated treatment of both the business and academic elements of marketing applications in predictive analytics. Writing for both managers and students, Thomas W. Miller explains essential concepts, principles, and theory in the context of real-world applications. Building on Miller's pioneering program, Marketing Data Science thoroughly addresses segmentation, target marketing, brand and product positioning, new product development, choice modeling, recommender systems, pricing research, retail site selection, demand estimation, sales forecasting, customer retention, and lifetime value analysis. Starting where Miller's widely-praised Modeling Techniques in Predictive Analytics left off, he integrates crucial information and insights that were previously segregated in texts on web analytics, network science, information technology, and programming. Coverage includes: The role of analytics in delivering effective messages on the web Understanding the web by understanding its hidden structures Being recognized on the web – and watching your own competitors Visualizing networks and understanding communities within them Measuring sentiment and making recommendations Leveraging key data science methods: databases/data preparation, classical/Bayesian statistics, regression/classification, machine learning, and text analytics Six complete case studies address exceptionally relevant issues such as: separating legitimate email from spam; identifying legally-relevant information for lawsuit discovery; gleaning insights from anonymous web surfing data, and more. This text's extensive set of web and network problems draw on rich public-domain data sources; many are accompanied by solutions in Python and/or R. Marketing Data Science will be an invaluable resource for all students, faculty, and professional marketers who want to use business analytics to improve marketing performance.