Learning Networks And Statistics
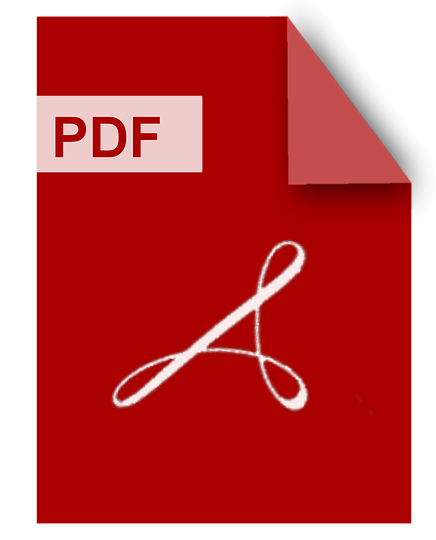
DOWNLOAD
Download Learning Networks And Statistics PDF/ePub or read online books in Mobi eBooks. Click Download or Read Online button to get Learning Networks And Statistics book now. This website allows unlimited access to, at the time of writing, more than 1.5 million titles, including hundreds of thousands of titles in various foreign languages. If the content not found or just blank you must refresh this page
Learning Networks And Statistics
DOWNLOAD
Author : Giacomo Della Riccia
language : en
Publisher: Springer
Release Date : 2014-05-04
Learning Networks And Statistics written by Giacomo Della Riccia and has been published by Springer this book supported file pdf, txt, epub, kindle and other format this book has been release on 2014-05-04 with Computers categories.
The contents of these proceedings reflect the intention of the organizers of the workshop to bring together scientists and engineers having a strong interest in interdisciplinary work in the fields of computer science, mathematics and applied statistics. Results of this collaboration are illustrated in problems dealing with neural nets, statistics and networks, classification and data mining, and (machine) learning.
Neural Networks And Statistical Learning
DOWNLOAD
Author : Ke-Lin Du
language : en
Publisher: Springer Nature
Release Date : 2019-09-12
Neural Networks And Statistical Learning written by Ke-Lin Du and has been published by Springer Nature this book supported file pdf, txt, epub, kindle and other format this book has been release on 2019-09-12 with Mathematics categories.
This book provides a broad yet detailed introduction to neural networks and machine learning in a statistical framework. A single, comprehensive resource for study and further research, it explores the major popular neural network models and statistical learning approaches with examples and exercises and allows readers to gain a practical working understanding of the content. This updated new edition presents recently published results and includes six new chapters that correspond to the recent advances in computational learning theory, sparse coding, deep learning, big data and cloud computing. Each chapter features state-of-the-art descriptions and significant research findings. The topics covered include: • multilayer perceptron; • the Hopfield network; • associative memory models;• clustering models and algorithms; • t he radial basis function network; • recurrent neural networks; • nonnegative matrix factorization; • independent component analysis; •probabilistic and Bayesian networks; and • fuzzy sets and logic. Focusing on the prominent accomplishments and their practical aspects, this book provides academic and technical staff, as well as graduate students and researchers with a solid foundation and comprehensive reference on the fields of neural networks, pattern recognition, signal processing, and machine learning.
Statistical Learning Using Neural Networks
DOWNLOAD
Author : Basilio de Bragança Pereira
language : en
Publisher: CRC Press
Release Date : 2020
Statistical Learning Using Neural Networks written by Basilio de Bragança Pereira and has been published by CRC Press this book supported file pdf, txt, epub, kindle and other format this book has been release on 2020 with Business & Economics categories.
Statistical Learning using Neural Networks: A Guide for Statisticians and Data Scientists with Python introduces artificial neural networks starting from the basics and increasingly demanding more effort from readers, who can learn the theory and its applications in statistical methods with concrete Python code examples. It presents a wide range of widely used statistical methodologies, applied in several research areas with Python code examples, which are available online. It is suitable for scientists and developers as well as graduate students. Key Features: Discusses applications in several research areas Covers a wide range of widely used statistical methodologies Includes Python code examples Gives numerous neural network models This book covers fundamental concepts on Neural Networks including Multivariate Statistics Neural Networks, Regression Neural Network Models, Survival Analysis Networks, Time Series Forecasting Networks, Control Chart Networks, and Statistical Inference Results. This book is suitable for both teaching and research. It introduces neural networks and is a guide for outsiders of academia working in data mining and artificial intelligence (AI). This book brings together data analysis from statistics to computer science using neural networks.
From Statistics To Neural Networks
DOWNLOAD
Author : Vladimir Cherkassky
language : en
Publisher: Springer Science & Business Media
Release Date : 2012-12-06
From Statistics To Neural Networks written by Vladimir Cherkassky and has been published by Springer Science & Business Media this book supported file pdf, txt, epub, kindle and other format this book has been release on 2012-12-06 with Computers categories.
The NATO Advanced Study Institute From Statistics to Neural Networks, Theory and Pattern Recognition Applications took place in Les Arcs, Bourg Saint Maurice, France, from June 21 through July 2, 1993. The meeting brought to gether over 100 participants (including 19 invited lecturers) from 20 countries. The invited lecturers whose contributions appear in this volume are: L. Almeida (INESC, Portugal), G. Carpenter (Boston, USA), V. Cherkassky (Minnesota, USA), F. Fogelman Soulie (LRI, France), W. Freeman (Berkeley, USA), J. Friedman (Stanford, USA), F. Girosi (MIT, USA and IRST, Italy), S. Grossberg (Boston, USA), T. Hastie (AT&T, USA), J. Kittler (Surrey, UK), R. Lippmann (MIT Lincoln Lab, USA), J. Moody (OGI, USA), G. Palm (U1m, Germany), B. Ripley (Oxford, UK), R. Tibshirani (Toronto, Canada), H. Wechsler (GMU, USA), C. Wellekens (Eurecom, France) and H. White (San Diego, USA). The ASI consisted of lectures overviewing major aspects of statistical and neural network learning, their links to biological learning and non-linear dynamics (chaos), and real-life examples of pattern recognition applications. As a result of lively interactions between the participants, the following topics emerged as major themes of the meeting: (1) Unified framework for the study of Predictive Learning in Statistics and Artificial Neural Networks (ANNs); (2) Differences and similarities between statistical and ANN methods for non parametric estimation from examples (learning); (3) Fundamental connections between artificial learning systems and biological learning systems.
Statistical And Machine Learning Approaches For Network Analysis
DOWNLOAD
Author : Matthias Dehmer
language : en
Publisher: John Wiley & Sons
Release Date : 2012-06-26
Statistical And Machine Learning Approaches For Network Analysis written by Matthias Dehmer and has been published by John Wiley & Sons this book supported file pdf, txt, epub, kindle and other format this book has been release on 2012-06-26 with Mathematics categories.
Explore the multidisciplinary nature of complex networks through machine learning techniques Statistical and Machine Learning Approaches for Network Analysis provides an accessible framework for structurally analyzing graphs by bringing together known and novel approaches on graph classes and graph measures for classification. By providing different approaches based on experimental data, the book uniquely sets itself apart from the current literature by exploring the application of machine learning techniques to various types of complex networks. Comprised of chapters written by internationally renowned researchers in the field of interdisciplinary network theory, the book presents current and classical methods to analyze networks statistically. Methods from machine learning, data mining, and information theory are strongly emphasized throughout. Real data sets are used to showcase the discussed methods and topics, which include: A survey of computational approaches to reconstruct and partition biological networks An introduction to complex networks—measures, statistical properties, and models Modeling for evolving biological networks The structure of an evolving random bipartite graph Density-based enumeration in structured data Hyponym extraction employing a weighted graph kernel Statistical and Machine Learning Approaches for Network Analysis is an excellent supplemental text for graduate-level, cross-disciplinary courses in applied discrete mathematics, bioinformatics, pattern recognition, and computer science. The book is also a valuable reference for researchers and practitioners in the fields of applied discrete mathematics, machine learning, data mining, and biostatistics.
Neural Networks And Statistical Learning
DOWNLOAD
Author : K.-L. Du
language : en
Publisher:
Release Date : 2019
Neural Networks And Statistical Learning written by K.-L. Du and has been published by this book supported file pdf, txt, epub, kindle and other format this book has been release on 2019 with Computational intelligence categories.
This book provides a broad yet detailed introduction to neural networks and machine learning in a statistical framework. A single, comprehensive resource for study and further research, it explores the major popular neural network models and statistical learning approaches with examples and exercises and allows readers to gain a practical working understanding of the content. This updated new edition presents recently published results and includes six new chapters that correspond to the recent advances in computational learning theory, sparse coding, deep learning, big data and cloud computing. Each chapter features state-of-the-art descriptions and significant research findings. The topics covered include: • multilayer perceptron; • the Hopfield network; • associative memory models; • clustering models and algorithms; • t he radial basis function network; • recurrent neural networks; • nonnegative matrix factorization; • independent component analysis; •probabilistic and Bayesian networks; and • fuzzy sets and logic. Focusing on the prominent accomplishments and their practical aspects, this book provides academic and technical staff, as well as graduate students and researchers with a solid foundation and comprehensive reference on the fields of neural networks, pattern recognition, signal processing, and machine learning.
Statistics For Data Science
DOWNLOAD
Author : James D. Miller
language : en
Publisher: Packt Publishing Ltd
Release Date : 2017-11-17
Statistics For Data Science written by James D. Miller and has been published by Packt Publishing Ltd this book supported file pdf, txt, epub, kindle and other format this book has been release on 2017-11-17 with Computers categories.
Get your statistics basics right before diving into the world of data science About This Book No need to take a degree in statistics, read this book and get a strong statistics base for data science and real-world programs; Implement statistics in data science tasks such as data cleaning, mining, and analysis Learn all about probability, statistics, numerical computations, and more with the help of R programs Who This Book Is For This book is intended for those developers who are willing to enter the field of data science and are looking for concise information of statistics with the help of insightful programs and simple explanation. Some basic hands on R will be useful. What You Will Learn Analyze the transition from a data developer to a data scientist mindset Get acquainted with the R programs and the logic used for statistical computations Understand mathematical concepts such as variance, standard deviation, probability, matrix calculations, and more Learn to implement statistics in data science tasks such as data cleaning, mining, and analysis Learn the statistical techniques required to perform tasks such as linear regression, regularization, model assessment, boosting, SVMs, and working with neural networks Get comfortable with performing various statistical computations for data science programmatically In Detail Data science is an ever-evolving field, which is growing in popularity at an exponential rate. Data science includes techniques and theories extracted from the fields of statistics; computer science, and, most importantly, machine learning, databases, data visualization, and so on. This book takes you through an entire journey of statistics, from knowing very little to becoming comfortable in using various statistical methods for data science tasks. It starts off with simple statistics and then move on to statistical methods that are used in data science algorithms. The R programs for statistical computation are clearly explained along with logic. You will come across various mathematical concepts, such as variance, standard deviation, probability, matrix calculations, and more. You will learn only what is required to implement statistics in data science tasks such as data cleaning, mining, and analysis. You will learn the statistical techniques required to perform tasks such as linear regression, regularization, model assessment, boosting, SVMs, and working with neural networks. By the end of the book, you will be comfortable with performing various statistical computations for data science programmatically. Style and approach Step by step comprehensive guide with real world examples
The Elements Of Statistical Learning
DOWNLOAD
Author : Trevor Hastie
language : en
Publisher: Springer Science & Business Media
Release Date : 2013-11-11
The Elements Of Statistical Learning written by Trevor Hastie and has been published by Springer Science & Business Media this book supported file pdf, txt, epub, kindle and other format this book has been release on 2013-11-11 with Mathematics categories.
During the past decade there has been an explosion in computation and information technology. With it have come vast amounts of data in a variety of fields such as medicine, biology, finance, and marketing. The challenge of understanding these data has led to the development of new tools in the field of statistics, and spawned new areas such as data mining, machine learning, and bioinformatics. Many of these tools have common underpinnings but are often expressed with different terminology. This book describes the important ideas in these areas in a common conceptual framework. While the approach is statistical, the emphasis is on concepts rather than mathematics. Many examples are given, with a liberal use of color graphics. It should be a valuable resource for statisticians and anyone interested in data mining in science or industry. The book’s coverage is broad, from supervised learning (prediction) to unsupervised learning. The many topics include neural networks, support vector machines, classification trees and boosting---the first comprehensive treatment of this topic in any book. This major new edition features many topics not covered in the original, including graphical models, random forests, ensemble methods, least angle regression & path algorithms for the lasso, non-negative matrix factorization, and spectral clustering. There is also a chapter on methods for “wide” data (p bigger than n), including multiple testing and false discovery rates. Trevor Hastie, Robert Tibshirani, and Jerome Friedman are professors of statistics at Stanford University. They are prominent researchers in this area: Hastie and Tibshirani developed generalized additive models and wrote a popular book of that title. Hastie co-developed much of the statistical modeling software and environment in R/S-PLUS and invented principal curves and surfaces. Tibshirani proposed the lasso and is co-author of the very successful An Introduction to the Bootstrap. Friedman is the co-inventor of many data-mining tools including CART, MARS, projection pursuit and gradient boosting.
Learning From Data
DOWNLOAD
Author : Doug Fisher
language : en
Publisher: Springer Science & Business Media
Release Date : 2012-12-06
Learning From Data written by Doug Fisher and has been published by Springer Science & Business Media this book supported file pdf, txt, epub, kindle and other format this book has been release on 2012-12-06 with Mathematics categories.
Ten years ago Bill Gale of AT&T Bell Laboratories was primary organizer of the first Workshop on Artificial Intelligence and Statistics. In the early days of the Workshop series it seemed clear that researchers in AI and statistics had common interests, though with different emphases, goals, and vocabularies. In learning and model selection, for example, a historical goal of AI to build autonomous agents probably contributed to a focus on parameter-free learning systems, which relied little on an external analyst's assumptions about the data. This seemed at odds with statistical strategy, which stemmed from a view that model selection methods were tools to augment, not replace, the abilities of a human analyst. Thus, statisticians have traditionally spent considerably more time exploiting prior information of the environment to model data and exploratory data analysis methods tailored to their assumptions. In statistics, special emphasis is placed on model checking, making extensive use of residual analysis, because all models are 'wrong', but some are better than others. It is increasingly recognized that AI researchers and/or AI programs can exploit the same kind of statistical strategies to good effect. Often AI researchers and statisticians emphasized different aspects of what in retrospect we might now regard as the same overriding tasks.
Dynamics On And Of Complex Networks Iii
DOWNLOAD
Author : Fakhteh Ghanbarnejad
language : en
Publisher: Springer
Release Date : 2019-05-13
Dynamics On And Of Complex Networks Iii written by Fakhteh Ghanbarnejad and has been published by Springer this book supported file pdf, txt, epub, kindle and other format this book has been release on 2019-05-13 with Science categories.
This book bridges the gap between advances in the communities of computer science and physics--namely machine learning and statistical physics. It contains diverse but relevant topics in statistical physics, complex systems, network theory, and machine learning. Examples of such topics are: predicting missing links, higher-order generative modeling of networks, inferring network structure by tracking the evolution and dynamics of digital traces, recommender systems, and diffusion processes. The book contains extended versions of high-quality submissions received at the workshop, Dynamics On and Of Complex Networks (doocn.org), together with new invited contributions. The chapters will benefit a diverse community of researchers. The book is suitable for graduate students, postdoctoral researchers and professors of various disciplines including sociology, physics, mathematics, and computer science.