Linear Causal Modeling With Structural Equations
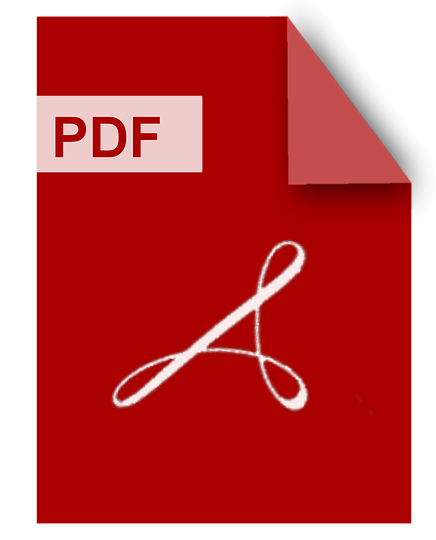
DOWNLOAD
Download Linear Causal Modeling With Structural Equations PDF/ePub or read online books in Mobi eBooks. Click Download or Read Online button to get Linear Causal Modeling With Structural Equations book now. This website allows unlimited access to, at the time of writing, more than 1.5 million titles, including hundreds of thousands of titles in various foreign languages. If the content not found or just blank you must refresh this page
Linear Causal Modeling With Structural Equations
DOWNLOAD
Author : Stanley A. Mulaik
language : en
Publisher: CRC Press
Release Date : 2009-06-16
Linear Causal Modeling With Structural Equations written by Stanley A. Mulaik and has been published by CRC Press this book supported file pdf, txt, epub, kindle and other format this book has been release on 2009-06-16 with Mathematics categories.
Emphasizing causation as a functional relationship between variables, this book provides comprehensive coverage on the basics of SEM. It takes readers through the process of identifying, estimating, analyzing, and evaluating a range of models. The author discusses the history and philosophy of causality and its place in science and presents graph theory as a tool for the design and analysis of causal models. He explains how the algorithms in SEM are derived and how they work, covers various indices and tests for evaluating the fit of structural equation models to data, and explores recent research in graph theory, path tracing rules, and model evaluation.
Handbook Of Multivariate Experimental Psychology
DOWNLOAD
Author : John R. Nesselroade
language : en
Publisher: Springer Science & Business Media
Release Date : 2013-11-11
Handbook Of Multivariate Experimental Psychology written by John R. Nesselroade and has been published by Springer Science & Business Media this book supported file pdf, txt, epub, kindle and other format this book has been release on 2013-11-11 with Psychology categories.
When the first edition of this Handbook was fields are likely to be hard reading, but anyone who wants to get in touch with the published in 1966 I scarcely gave thought to a future edition. Its whole purpose was to growing edges will find something to meet his inaugurate a radical new outlook on ex taste. perimental psychology, and if that could be Of course, this book will need teachers. As accomplished it was sufficient reward. In the it supersedes the narrow conceptions of 22 years since we have seen adequate-indeed models and statistics still taught as bivariate staggering-evidence that the growth of a new and ANOV A methods of experiment, in so branch of psychological method in science has many universities, those universities will need become established. The volume of research to expand their faculties with newly trained has grown apace in the journals and has young people. The old vicious circle of opened up new areas and a surprising increase obsoletely trained members turning out new of knowledge in methodology. obsoletely trained members has to be The credit for calling attention to the need recognized and broken. And wherever re for new guidance belongs to many members search deals with integral wholes-in per of the Society of Multivariate Experimental sonalities, processes, and groups-researchers Psychology, but the actual innervation is due will recognize the vast new future that to the skill and endurance of one man, John multivariate methods open up.
Recent Developments On Structural Equation Models
DOWNLOAD
Author : Kees van Montfort
language : en
Publisher: Springer Science & Business Media
Release Date : 2004-04-30
Recent Developments On Structural Equation Models written by Kees van Montfort and has been published by Springer Science & Business Media this book supported file pdf, txt, epub, kindle and other format this book has been release on 2004-04-30 with Psychology categories.
After Karl Jöreskog's first presentation in 1970, Structural Equation Modelling or SEM has become a main statistical tool in many fields of science. It is the standard approach of factor analytic and causal modelling in such diverse fields as sociology, education, psychology, economics, management and medical sciences. In addition to an extension of its application area, Structural Equation Modelling also features a continual renewal and extension of its theoretical background. The sixteen contributions to this book, written by experts from many countries, present important new developments and interesting applications in Structural Equation Modelling. The book addresses methodologists and statisticians professionally dealing with Structural Equation Modelling to enhance their knowledge of the type of models covered and the technical problems involved in their formulation. In addition, the book offers applied researchers new ideas about the use of Structural Equation Modeling in solving their problems. Finally, methodologists, mathematicians and applied researchers alike are addressed, who simply want to update their knowledge of recent approaches in data analysis and mathematical modelling.
Ecological Statistics
DOWNLOAD
Author : Gordon A. Fox
language : en
Publisher: Oxford University Press
Release Date : 2015
Ecological Statistics written by Gordon A. Fox and has been published by Oxford University Press this book supported file pdf, txt, epub, kindle and other format this book has been release on 2015 with Computers categories.
The application and interpretation of statistics are central to ecological study and practice. Ecologists are now asking more sophisticated questions than in the past. These new questions, together with the continued growth of computing power and the availability of new software, have created a new generation of statistical techniques. These have resulted in major recent developments in both our understanding and practice of ecological statistics. This novel book synthesizes a number of these changes, addressing key approaches and issues that tend to be overlooked in other books such as missing/censored data, correlation structure of data, heterogeneous data, and complex causal relationships. These issues characterize a large proportion of ecological data, but most ecologists' training in traditional statistics simply does not provide them with adequate preparation to handle the associated challenges. Uniquely, Ecological Statistics highlights the underlying links among many statistical approaches that attempt to tackle these issues. In particular, it gives readers an introduction to approaches to inference, likelihoods, generalized linear (mixed) models, spatially or phylogenetically-structured data, and data synthesis, with a strong emphasis on conceptual understanding and subsequent application to data analysis. Written by a team of practicing ecologists, mathematical explanations have been kept to the minimum necessary. This user-friendly textbook will be suitable for graduate students, researchers, and practitioners in the fields of ecology, evolution, environmental studies, and computational biology who are interested in updating their statistical tool kits. A companion web site provides example data sets and commented code in the R language.
Cause And Correlation In Biology
DOWNLOAD
Author : Bill Shipley
language : en
Publisher: Cambridge University Press
Release Date : 2002-08
Cause And Correlation In Biology written by Bill Shipley and has been published by Cambridge University Press this book supported file pdf, txt, epub, kindle and other format this book has been release on 2002-08 with Mathematics categories.
This book goes beyond the truism that 'correlation does not imply causation' and explores the logical and methodological relationships between correlation and causation. It presents a series of statistical methods that can test, and potentially discover, cause-effect relationships between variables in situations in which it is not possible to conduct randomised or experimentally controlled experiments. Many of these methods are quite new and most are generally unknown to biologists. In addition to describing how to conduct these statistical tests, the book also puts the methods into historical context and explains when they can and cannot justifiably be used to test or discover causal claims. Written in a conversational style that minimises technical jargon, the book is aimed at practising biologists and advanced students, and assumes only a very basic knowledge of introductory statistics.
Handbook Of Structural Equation Modeling
DOWNLOAD
Author : Rick H. Hoyle
language : en
Publisher: Guilford Publications
Release Date : 2023-02-17
Handbook Of Structural Equation Modeling written by Rick H. Hoyle and has been published by Guilford Publications this book supported file pdf, txt, epub, kindle and other format this book has been release on 2023-02-17 with Business & Economics categories.
"This accessible volume presents both the mechanics of structural equation modeling (SEM) and specific SEM strategies and applications. The editor, along with an international group of contributors, and editorial advisory board are leading methodologists who have organized the book to move from simpler material to more statistically complex modeling approaches. Sections cover the foundations of SEM; statistical underpinnings, from assumptions to model modifications; steps in implementation, from data preparation through writing the SEM report; and basic and advanced applications, including new and emerging topics in SEM. Each chapter provides conceptually oriented descriptions, fully explicated analyses, and engaging examples that reveal modeling possibilities for use with readers' data. Many of the chapters also include access to data and syntax files at the companion website, allowing readers to try their hands at reproducing the authors' results"--
Introduction To Structural Equation Models
DOWNLOAD
Author : Otis Dudley Duncan
language : en
Publisher: Elsevier
Release Date : 2014-06-28
Introduction To Structural Equation Models written by Otis Dudley Duncan and has been published by Elsevier this book supported file pdf, txt, epub, kindle and other format this book has been release on 2014-06-28 with Mathematics categories.
Introduction to Structural Equation Models prepares the reader to understand the recent sociological literature on the use of structural equation models in research, and discusses methodological questions pertaining to such models. The material in first seven chapters is almost entirely standard, with the remaining four introducing progressively more open-ended issues, seducing the reader into beginning to think for himself about the properties of models or even to suggest problems that may intrigue the advanced student.
A Beginner S Guide To Structural Equation Modeling
DOWNLOAD
Author : Tiffany A. Whittaker
language : en
Publisher: Routledge
Release Date : 2022-04-27
A Beginner S Guide To Structural Equation Modeling written by Tiffany A. Whittaker and has been published by Routledge this book supported file pdf, txt, epub, kindle and other format this book has been release on 2022-04-27 with Psychology categories.
A Beginner’s Guide to Structural Equation Modeling, fifth edition, has been redesigned with consideration of a true beginner in structural equation modeling (SEM) in mind. The book covers introductory through intermediate topics in SEM in more detail than in any previous edition. All of the chapters that introduce models in SEM have been expanded to include easy-to-follow, step-by-step guidelines that readers can use when conducting their own SEM analyses. These chapters also include examples of tables to include in results sections that readers may use as templates when writing up the findings from their SEM analyses. The models that are illustrated in the text will allow SEM beginners to conduct, interpret, and write up analyses for observed variable path models to full structural models, up to testing higher order models as well as multiple group modeling techniques. Updated information about methodological research in relevant areas will help students and researchers be more informed readers of SEM research. The checklist of SEM considerations when conducting and reporting SEM analyses is a collective set of requirements that will help improve the rigor of SEM analyses. This book is intended for true beginners in SEM and is designed for introductory graduate courses in SEM taught in psychology, education, business, and the social and healthcare sciences. This book also appeals to researchers and faculty in various disciplines. Prerequisites include correlation and regression methods.
Longitudinal Structural Equation Modeling
DOWNLOAD
Author : Todd D. Little
language : en
Publisher: Guilford Press
Release Date : 2013-02-26
Longitudinal Structural Equation Modeling written by Todd D. Little and has been published by Guilford Press this book supported file pdf, txt, epub, kindle and other format this book has been release on 2013-02-26 with Psychology categories.
This book has been replaced by Longitudinal Structural Equation Modeling, Second Edition, ISBN 978-1-4625-5314-3.
Handbook Of Causal Analysis For Social Research
DOWNLOAD
Author : Stephen L. Morgan
language : en
Publisher: Springer Science & Business Media
Release Date : 2013-04-22
Handbook Of Causal Analysis For Social Research written by Stephen L. Morgan and has been published by Springer Science & Business Media this book supported file pdf, txt, epub, kindle and other format this book has been release on 2013-04-22 with Social Science categories.
What constitutes a causal explanation, and must an explanation be causal? What warrants a causal inference, as opposed to a descriptive regularity? What techniques are available to detect when causal effects are present, and when can these techniques be used to identify the relative importance of these effects? What complications do the interactions of individuals create for these techniques? When can mixed methods of analysis be used to deepen causal accounts? Must causal claims include generative mechanisms, and how effective are empirical methods designed to discover them? The Handbook of Causal Analysis for Social Research tackles these questions with nineteen chapters from leading scholars in sociology, statistics, public health, computer science, and human development.