Logistic Regression With Missing Values In The Covariates
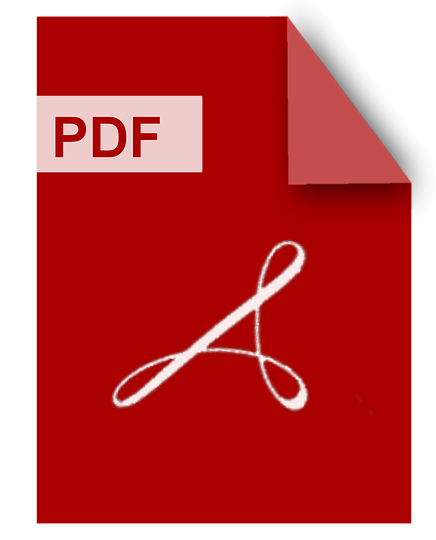
DOWNLOAD
Download Logistic Regression With Missing Values In The Covariates PDF/ePub or read online books in Mobi eBooks. Click Download or Read Online button to get Logistic Regression With Missing Values In The Covariates book now. This website allows unlimited access to, at the time of writing, more than 1.5 million titles, including hundreds of thousands of titles in various foreign languages. If the content not found or just blank you must refresh this page
Logistic Regression With Missing Values In The Covariates
DOWNLOAD
Author : Werner Vach
language : en
Publisher: Springer Science & Business Media
Release Date : 2012-12-06
Logistic Regression With Missing Values In The Covariates written by Werner Vach and has been published by Springer Science & Business Media this book supported file pdf, txt, epub, kindle and other format this book has been release on 2012-12-06 with Mathematics categories.
In many areas of science a basic task is to assess the influence of several factors on a quantity of interest. If this quantity is binary logistic, regression models provide a powerful tool for this purpose. This monograph presents an account of the use of logistic regression in the case where missing values in the variables prevent the use of standard techniques. Such situations occur frequently across a wide range of statistical applications. The emphasis of this book is on methods related to the classical maximum likelihood principle. The author reviews the essentials of logistic regression and discusses the variety of mechanisms which might cause missing values while the rest of the book covers the methods which may be used to deal with missing values and their effectiveness. Researchers across a range of disciplines and graduate students in statistics and biostatistics will find this a readable account of this.
Logistic Regression With Incompletely Observed Binary Covariates
DOWNLOAD
Author : Hai-An Hsu
language : en
Publisher:
Release Date : 1995
Logistic Regression With Incompletely Observed Binary Covariates written by Hai-An Hsu and has been published by this book supported file pdf, txt, epub, kindle and other format this book has been release on 1995 with Logistic regression analysis categories.
Logistic regression is one of the most important tools in the analysis of epidemiological and clinical data. Such data often contain missing values for one or more variables. Common practice is to eliminate all individuals for whom any information is missing. This deletion approach does not make efficient use of available information and often introduces bias.
Multiple Imputation Of Missing Data In Practice
DOWNLOAD
Author : Yulei He
language : en
Publisher: CRC Press
Release Date : 2021-11-19
Multiple Imputation Of Missing Data In Practice written by Yulei He and has been published by CRC Press this book supported file pdf, txt, epub, kindle and other format this book has been release on 2021-11-19 with Mathematics categories.
Multiple Imputation of Missing Data in Practice: Basic Theory and Analysis Strategies provides a comprehensive introduction to the multiple imputation approach to missing data problems that are often encountered in data analysis. Over the past 40 years or so, multiple imputation has gone through rapid development in both theories and applications. It is nowadays the most versatile, popular, and effective missing-data strategy that is used by researchers and practitioners across different fields. There is a strong need to better understand and learn about multiple imputation in the research and practical community. Accessible to a broad audience, this book explains statistical concepts of missing data problems and the associated terminology. It focuses on how to address missing data problems using multiple imputation. It describes the basic theory behind multiple imputation and many commonly-used models and methods. These ideas are illustrated by examples from a wide variety of missing data problems. Real data from studies with different designs and features (e.g., cross-sectional data, longitudinal data, complex surveys, survival data, studies subject to measurement error, etc.) are used to demonstrate the methods. In order for readers not only to know how to use the methods, but understand why multiple imputation works and how to choose appropriate methods, simulation studies are used to assess the performance of the multiple imputation methods. Example datasets and sample programming code are either included in the book or available at a github site (https://github.com/he-zhang-hsu/multiple_imputation_book). Key Features Provides an overview of statistical concepts that are useful for better understanding missing data problems and multiple imputation analysis Provides a detailed discussion on multiple imputation models and methods targeted to different types of missing data problems (e.g., univariate and multivariate missing data problems, missing data in survival analysis, longitudinal data, complex surveys, etc.) Explores measurement error problems with multiple imputation Discusses analysis strategies for multiple imputation diagnostics Discusses data production issues when the goal of multiple imputation is to release datasets for public use, as done by organizations that process and manage large-scale surveys with nonresponse problems For some examples, illustrative datasets and sample programming code from popular statistical packages (e.g., SAS, R, WinBUGS) are included in the book. For others, they are available at a github site (https://github.com/he-zhang-hsu/multiple_imputation_book)
Multiple Imputation Of Missing Data Using Sas
DOWNLOAD
Author : Patricia Berglund
language : en
Publisher: SAS Institute
Release Date : 2014-07-01
Multiple Imputation Of Missing Data Using Sas written by Patricia Berglund and has been published by SAS Institute this book supported file pdf, txt, epub, kindle and other format this book has been release on 2014-07-01 with Computers categories.
Find guidance on using SAS for multiple imputation and solving common missing data issues. Multiple Imputation of Missing Data Using SAS provides both theoretical background and constructive solutions for those working with incomplete data sets in an engaging example-driven format. It offers practical instruction on the use of SAS for multiple imputation and provides numerous examples that use a variety of public release data sets with applications to survey data. Written for users with an intermediate background in SAS programming and statistics, this book is an excellent resource for anyone seeking guidance on multiple imputation. The authors cover the MI and MIANALYZE procedures in detail, along with other procedures used for analysis of complete data sets. They guide analysts through the multiple imputation process, including evaluation of missing data patterns, choice of an imputation method, execution of the process, and interpretation of results. Topics discussed include how to deal with missing data problems in a statistically appropriate manner, how to intelligently select an imputation method, how to incorporate the uncertainty introduced by the imputation process, and how to incorporate the complex sample design (if appropriate) through use of the SAS SURVEY procedures. Discover the theoretical background and see extensive applications of the multiple imputation process in action. This book is part of the SAS Press program.
Flexible Imputation Of Missing Data
DOWNLOAD
Author : Stef van Buuren
language : en
Publisher: CRC Press
Release Date : 2012-03-29
Flexible Imputation Of Missing Data written by Stef van Buuren and has been published by CRC Press this book supported file pdf, txt, epub, kindle and other format this book has been release on 2012-03-29 with Mathematics categories.
Missing data form a problem in every scientific discipline, yet the techniques required to handle them are complicated and often lacking. One of the great ideas in statistical science—multiple imputation—fills gaps in the data with plausible values, the uncertainty of which is coded in the data itself. It also solves other problems, many of which are missing data problems in disguise. Flexible Imputation of Missing Data is supported by many examples using real data taken from the author's vast experience of collaborative research, and presents a practical guide for handling missing data under the framework of multiple imputation. Furthermore, detailed guidance of implementation in R using the author’s package MICE is included throughout the book. Assuming familiarity with basic statistical concepts and multivariate methods, Flexible Imputation of Missing Data is intended for two audiences: (Bio)statisticians, epidemiologists, and methodologists in the social and health sciences Substantive researchers who do not call themselves statisticians, but who possess the necessary skills to understand the principles and to follow the recipes This graduate-tested book avoids mathematical and technical details as much as possible: formulas are accompanied by a verbal statement that explains the formula in layperson terms. Readers less concerned with the theoretical underpinnings will be able to pick up the general idea, and technical material is available for those who desire deeper understanding. The analyses can be replicated in R using a dedicated package developed by the author.
Topics On Bayesian Analysis Of Missing Data
DOWNLOAD
Author : Yun Kai Jiang
language : en
Publisher:
Release Date : 2011
Topics On Bayesian Analysis Of Missing Data written by Yun Kai Jiang and has been published by this book supported file pdf, txt, epub, kindle and other format this book has been release on 2011 with categories.
This dissertation focuses on model selection in logistic regression with incompletely observed data. In particular, methods are presented for using Markov Chain Monte Carlo imputation and Bayesian variable selection to model a binary outcome. We consider multivariate missing covariates, with different types of predictors, such as continuous, counts, and categorical variables. Such type of data is considered in the analysis of Project Talent recorded from a longitudinal study. Roughly 400,000 were selected for the study from United States high school students in grades 9 through 12 during the year 1960; follow-up surveys were conducted 1, 5, and 11 years after graduation. We extend a methodology developed by Yang, Belin, and Boscardin (2005), to this Project Talent for a logistic regression model with incomplete covariates. The idea is to use data information as much as possible to fill in the missing values and study associations between a binary response variable and covariates. According to Yang, Belin, and Boscardin, one approach under a multivariate normal assumption for data, is to conduct Bayesian variable selection and missing data imputation simultaneously within one Gibbs Sampling process, called "Simultaneously Impute And Select" (SIAS). A modified strategy of SIAS is extended to a mixed data structure that allows for categorical, counts, and continuous variables. The first chapter consists of an introduction to some approaches to variable selection for missing data. The fact that missing data arise commonly in statistical analyses, leads to a variety of methods to handle missing data. The missing data mechanism needs to be considered in imputations. The multiple imputation methods and Markov Chain Mote Carlo (MCMC) algorithms are presented as general statistical approaches to missing data analysis. In the MCMC computational toolbox, various implementation methods for imputation are discussed: Metropolis-Hasting, Gibbs Sampler, and Data Augmentation. Compared to model selection methods in frequentist and likelihood inference, Bayesian inference takes an entirely different approach. The frequentist approach only looks at the current data to make inference. The Bayesian approach requires the specification of the prior distribution, which can come from historical data or expert opinion. Stochastic Search Variable Selection (SSVS) and Gibbs Variable Selection (GVS) are reviewed for model selection. Two alternative strategies, Impute Then Select (ITS) and Simultaneously Impute And Select (SIAS), are studied. In the second chapter, imputation and Bayesian variable selection methods for linear regression are extended to a binary response variable that is completely observed, but some covariates have missing values. We focus on extending SIAS strategy to logistic regression models via two alternative imputations, decomposition and Fully Conditional Specification (FCS). The decomposition method breaks a multivariate distribution into a series of univariate ones by decomposing the joint density function p(Y, X1, ..., X[p]) into the product of conditional distributions, using the factorization p(A, B) = p(A[vertical line]B)p(B). The FCS aims to involve iteratively sampling from the conditional distributions for one random variable, given all the others. These two methods are implemented in the imputation step of the SIAS procedure then applied to the Project Talent data. Simulations are also performed to validate these results and demonstrate the superiority of FCS over the decomposition method under certain circumstances. The third chapter presents a new approach for incorporating the sampling weight into imputation and Bayesian variable selection in logistic regression models. We develop the approach that extends SIAS by a Bayesian version of iterative weighted least squares algorithm to include a sampling step based on Gibbs sampler. This approach is illustrated using both simulation studies and Project Talent data.
Comparison Of Approaches For Handling Missingness In Covariates For Propensity Score Models
DOWNLOAD
Author : Jiangxiu Zhou
language : en
Publisher:
Release Date : 2015
Comparison Of Approaches For Handling Missingness In Covariates For Propensity Score Models written by Jiangxiu Zhou and has been published by this book supported file pdf, txt, epub, kindle and other format this book has been release on 2015 with categories.
Causal effect estimation with observational data is subject to bias due to confounding. Although potential confounders could be adjusted for by fitting a multiple regression model, a more effective way to control for confounding is to use propensity score methods. Propensity scores are most commonly estimated from logistic regression with a binary exposure; generalized propensity scores could be estimated instead using linear regression if the exposure is continuous. One unresolved issue in propensity score estimation is handling of missing values in covariates. As covariates are only used for propensity score estimation but not for later outcome analysis, missing values in covariates may need to be handled differently from missing values in outcome analysis. Several approaches have been proposed for handling covariate missingness, including multiple imputation (MI), multiple imputation with missingness pattern (MIMP) and treatment mean imputation. There are other potentially useful approaches that have not been evaluated, including single imputation, single conditional mean imputation and Generalized Boosted Modeling (GBM), which is a nonparametric approach of estimating propensity scores and missing values are automatically accounted for in the estimation.To evaluate the performance of single imputation, single conditional mean imputation and GBM in comparison to the previously proposed approaches including treatment mean imputation, MI and MIMP, a simulation study is conducted with a binary exposure. Results suggest that when all confounders are included for propensity score estimation, single imputation, single conditional mean imputation, MI and MIMP perform almost equally well and better than treatment mean imputation and GBM. To examine whether the finding could be extended to a continuous exposure setting, another simulation study is conducted. Results suggest that single imputation, single conditional imputation, MI, MIMP and GBM with single conditional mean imputation have equally good and better performance than treatment mean imputation and GBM with incomplete data under scenario A (linearity and additivity). None of the approaches perform well under scenario G (nonlinearity and nonadditivity). These approaches are further demonstrated and compared through an empirical analysis with the Adolescent Alcohol Prevention Trial (AAPT). A similar pattern of results is observed as in the simulation study. It is recommended to impute missing covariates using different approaches and similar estimates help provide more confidence in the estimates.
Model Free Curve Estimation
DOWNLOAD
Author : Michael E. Tarter
language : en
Publisher: CRC Press
Release Date : 1993-12-16
Model Free Curve Estimation written by Michael E. Tarter and has been published by CRC Press this book supported file pdf, txt, epub, kindle and other format this book has been release on 1993-12-16 with Mathematics categories.
Model-Free Curve Estimation details the Fourier series approach to density estimation and explores how model-free technology can be expanded to deal with other statistical curves, such as survival and regression functions. It also describes the implementation of some curves for exploratory data analysis, including a specialized curve for detecting and analyzing hidden subpopulations in data and a family of curves useful for finding the best transformation and model to use in a statistical analysis.
Logistic Regression Analysis With Missing Values
DOWNLOAD
Author :
language : en
Publisher:
Release Date : 2015
Logistic Regression Analysis With Missing Values written by and has been published by this book supported file pdf, txt, epub, kindle and other format this book has been release on 2015 with categories.
Logistic Regression With Missing Covariate Data
DOWNLOAD
Author : Marjorie Ireland
language : en
Publisher:
Release Date : 1995
Logistic Regression With Missing Covariate Data written by Marjorie Ireland and has been published by this book supported file pdf, txt, epub, kindle and other format this book has been release on 1995 with Regression analysis categories.