Machine Learning For Cloud Management
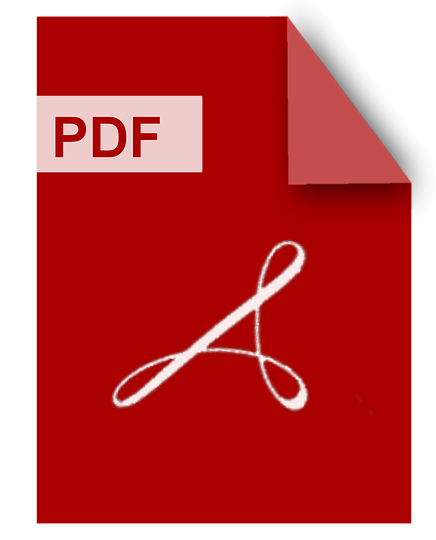
DOWNLOAD
Download Machine Learning For Cloud Management PDF/ePub or read online books in Mobi eBooks. Click Download or Read Online button to get Machine Learning For Cloud Management book now. This website allows unlimited access to, at the time of writing, more than 1.5 million titles, including hundreds of thousands of titles in various foreign languages. If the content not found or just blank you must refresh this page
Machine Learning For Cloud Management
DOWNLOAD
Author : Jitendra Kumar
language : en
Publisher: CRC Press
Release Date : 2021-11-26
Machine Learning For Cloud Management written by Jitendra Kumar and has been published by CRC Press this book supported file pdf, txt, epub, kindle and other format this book has been release on 2021-11-26 with Computers categories.
Cloud computing offers subscription-based on-demand services, and it has emerged as the backbone of the computing industry. It has enabled us to share resources among multiple users through virtualization, which creates a virtual instance of a computer system running in an abstracted hardware layer. Unlike early distributed computing models, it offers virtually limitless computing resources through its large scale cloud data centers. It has gained wide popularity over the past few years, with an ever-increasing infrastructure, a number of users, and the amount of hosted data. The large and complex workloads hosted on these data centers introduce many challenges, including resource utilization, power consumption, scalability, and operational cost. Therefore, an effective resource management scheme is essential to achieve operational efficiency with improved elasticity. Machine learning enabled solutions are the best fit to address these issues as they can analyze and learn from the data. Moreover, it brings automation to the solutions, which is an essential factor in dealing with large distributed systems in the cloud paradigm. Machine Learning for Cloud Management explores cloud resource management through predictive modelling and virtual machine placement. The predictive approaches are developed using regression-based time series analysis and neural network models. The neural network-based models are primarily trained using evolutionary algorithms, and efficient virtual machine placement schemes are developed using multi-objective genetic algorithms. Key Features: The first book to set out a range of machine learning methods for efficient resource management in a large distributed network of clouds. Predictive analytics is an integral part of efficient cloud resource management, and this book gives a future research direction to researchers in this domain. It is written by leading international researchers. The book is ideal for researchers who are working in the domain of cloud computing.
Machine Learning For Cloud Management
DOWNLOAD
Author : Jitendra Kumar
language : en
Publisher: Chapman & Hall/CRC
Release Date : 2021-11-25
Machine Learning For Cloud Management written by Jitendra Kumar and has been published by Chapman & Hall/CRC this book supported file pdf, txt, epub, kindle and other format this book has been release on 2021-11-25 with Computers categories.
Cloud computing offers subscription-based on-demand services, and it has emerged as the backbone of the computing industry. It has enabled us to share resources among multiple users through virtualization, which creates a virtual instance of a computer system running in an abstracted hardware layer. Unlike early distributed computing models, it offers virtually limitless computing resources through its large scale cloud data centers. It has gained wide popularity over the past few years, with an ever-increasing infrastructure, a number of users, and the amount of hosted data. The large and complex workloads hosted on these data centers introduce many challenges, including resource utilization, power consumption, scalability, and operational cost. Therefore, an effective resource management scheme is essential to achieve operational efficiency with improved elasticity. Machine learning enabled solutions are the best fit to address these issues as they can analyze and learn from the data. Moreover, it brings automation to the solutions, which is an essential factor in dealing with large distributed systems in the cloud paradigm. Machine Learning for Cloud Management explores cloud resource management through predictive modelling and virtual machine placement. The predictive approaches are developed using regression-based time series analysis and neural network models. The neural network-based models are primarily trained using evolutionary algorithms, and efficient virtual machine placement schemes are developed using multi-objective genetic algorithms. Key Features: The first book to set out a range of machine learning methods for efficient resource management in a large distributed network of clouds. Predictive analytics is an integral part of efficient cloud resource management, and this book gives a future research direction to researchers in this domain. It is written by leading international researchers. The book is ideal for researchers who are working in the domain of cloud computing.
Machine Learning And Optimization Models For Optimization In Cloud
DOWNLOAD
Author : Punit Gupta
language : en
Publisher: CRC Press
Release Date : 2022-02-17
Machine Learning And Optimization Models For Optimization In Cloud written by Punit Gupta and has been published by CRC Press this book supported file pdf, txt, epub, kindle and other format this book has been release on 2022-02-17 with Computers categories.
Machine Learning and Models for Optimization in Cloud’s main aim is to meet the user requirement with high quality of service, least time for computation and high reliability. With increase in services migrating over cloud providers, the load over the cloud increases resulting in fault and various security failure in the system results in decreasing reliability. To fulfill this requirement cloud system uses intelligent metaheuristic and prediction algorithm to provide resources to the user in an efficient manner to manage the performance of the system and plan for upcoming requests. Intelligent algorithm helps the system to predict and find a suitable resource for a cloud environment in real time with least computational complexity taking into mind the system performance in under loaded and over loaded condition. This book discusses the future improvements and possible intelligent optimization models using artificial intelligence, deep learning techniques and other hybrid models to improve the performance of cloud. Various methods to enhance the directivity of cloud services have been presented which would enable cloud to provide better services, performance and quality of service to user. It talks about the next generation intelligent optimization and fault model to improve security and reliability of cloud. Key Features · Comprehensive introduction to cloud architecture and its service models. · Vulnerability and issues in cloud SAAS, PAAS and IAAS · Fundamental issues related to optimizing the performance in Cloud Computing using meta-heuristic, AI and ML models · Detailed study of optimization techniques, and fault management techniques in multi layered cloud. · Methods to improve reliability and fault in cloud using nature inspired algorithms and artificial neural network. · Advanced study of algorithms using artificial intelligence for optimization in cloud · Method for power efficient virtual machine placement using neural network in cloud · Method for task scheduling using metaheuristic algorithms. · A study of machine learning and deep learning inspired resource allocation algorithm for cloud in fault aware environment. This book aims to create a research interest & motivation for graduates degree or post-graduates. It aims to present a study on optimization algorithms in cloud for researchers to provide them with a glimpse of future of cloud computing in the era of artificial intelligence.
Applications Of Machine Learning In Big Data Analytics And Cloud Computing
DOWNLOAD
Author : Subhendu Kumar Pani
language : en
Publisher: CRC Press
Release Date : 2022-09-01
Applications Of Machine Learning In Big Data Analytics And Cloud Computing written by Subhendu Kumar Pani and has been published by CRC Press this book supported file pdf, txt, epub, kindle and other format this book has been release on 2022-09-01 with Technology & Engineering categories.
Cloud Computing and Big Data technologies have become the new descriptors of the digital age. The global amount of digital data has increased more than nine times in volume in just five years and by 2030 its volume may reach a staggering 65 trillion gigabytes. This explosion of data has led to opportunities and transformation in various areas such as healthcare, enterprises, industrial manufacturing and transportation. New Cloud Computing and Big Data tools endow researchers and analysts with novel techniques and opportunities to collect, manage and analyze the vast quantities of data. In Cloud and Big Data Analytics, the two areas of Swarm Intelligence and Deep Learning are a developing type of Machine Learning techniques that show enormous potential for solving complex business problems. Deep Learning enables computers to analyze large quantities of unstructured and binary data and to deduce relationships without requiring specific models or programming instructions. This book introduces the state-of-the-art trends and advances in the use of Machine Learning in Cloud and Big Data Analytics. The book will serve as a reference for Data Scientists, systems architects, developers, new researchers and graduate level students in Computer and Data science. The book will describe the concepts necessary to understand current Machine Learning issues, challenges and possible solutions as well as upcoming trends in Big Data Analytics.
Pragmatic Ai
DOWNLOAD
Author : Noah Gift
language : en
Publisher: Addison-Wesley Professional
Release Date : 2018-07-12
Pragmatic Ai written by Noah Gift and has been published by Addison-Wesley Professional this book supported file pdf, txt, epub, kindle and other format this book has been release on 2018-07-12 with Computers categories.
Master Powerful Off-the-Shelf Business Solutions for AI and Machine Learning Pragmatic AI will help you solve real-world problems with contemporary machine learning, artificial intelligence, and cloud computing tools. Noah Gift demystifies all the concepts and tools you need to get results—even if you don’t have a strong background in math or data science. Gift illuminates powerful off-the-shelf cloud offerings from Amazon, Google, and Microsoft, and demonstrates proven techniques using the Python data science ecosystem. His workflows and examples help you streamline and simplify every step, from deployment to production, and build exceptionally scalable solutions. As you learn how machine language (ML) solutions work, you’ll gain a more intuitive understanding of what you can achieve with them and how to maximize their value. Building on these fundamentals, you’ll walk step-by-step through building cloud-based AI/ML applications to address realistic issues in sports marketing, project management, product pricing, real estate, and beyond. Whether you’re a business professional, decision-maker, student, or programmer, Gift’s expert guidance and wide-ranging case studies will prepare you to solve data science problems in virtually any environment. Get and configure all the tools you’ll need Quickly review all the Python you need to start building machine learning applications Master the AI and ML toolchain and project lifecycle Work with Python data science tools such as IPython, Pandas, Numpy, Juypter Notebook, and Sklearn Incorporate a pragmatic feedback loop that continually improves the efficiency of your workflows and systems Develop cloud AI solutions with Google Cloud Platform, including TPU, Colaboratory, and Datalab services Define Amazon Web Services cloud AI workflows, including spot instances, code pipelines, boto, and more Work with Microsoft Azure AI APIs Walk through building six real-world AI applications, from start to finish Register your book for convenient access to downloads, updates, and/or corrections as they become available. See inside book for details.
An Optimized Deep Machine Learning And Micro Services Architecture Based Proactive Elastic Cloud Framework
DOWNLOAD
Author : Tariq Daradkeh
language : en
Publisher:
Release Date : 2022
An Optimized Deep Machine Learning And Micro Services Architecture Based Proactive Elastic Cloud Framework written by Tariq Daradkeh and has been published by this book supported file pdf, txt, epub, kindle and other format this book has been release on 2022 with categories.
To achieve elasticity in cloud environment a holistic solution must be considered that measures all running applications and resources performance, including its cloud management system. Cloud resources and applications are continuously changing in their capacity and behavior, which implies a dynamic change in the cloud management system architecture and characteristics. The new era of application modeling is to decouple its components and make them as standalone cooperated modules following micro-service pattern architecture. This design gives an application a fast adaptation agility to change in requirements by customizing the application operation modules to match new tasks. The proposed elastic framework is achieved using multiple tasks as a sequence of steps. First, cloud resources monitoring, and workload changes are tracked. Second, workloads clustering using custom K-means method is used to categorize unlabeled workload sets. Third, workload demands, and datacenter configuration are predicted, classified, and labeled using deep machine learning techniques. Fourth, resources are scaled and scheduled based on workload characteristics and scaling dimension conditions. Fifth, a micro-service pattern based elastic framework is implemented for dynamic resources management and operation. First task, monitoring system must provide needed information to cloud manager to describe cloud dynamic state by reading cloud-generated logs and sending them to cloud manager. Log updates should be accurate, instantaneous, and sent with minimum time delay. Data sources vary from low to high level of cloud infrastructure resources, or they can be generated from workload demands. Logs are used to discover cloud system status, which is input for future actions in cloud management resources orchestration. Point Estimator (PE) log tracker is proposed that can dynamically adapt to the type of workload providing accurate fresh logs values to cloud manager with minimum number of data transactions. Second task, dynamic K-Means clustering using kernel density estimator is proposed to analyze and characterize both workloads and datacenter configurations. This method enhances K-Means clustering by automatically determining optimum number of classes and finding the mean centroids for the clusters. In addition, it improves the accuracy and the time complexity of standard K-Means clustering model, by best correlating between clustering attributes using statistical correlation methods. Third task, cloud workload prediction is a very critical task for elastic scaling, because cloud manager decides what configuration sequence is to be considered for resource provisioning. Predicting workload demands to optimize datacenter configuration, such that increasing/decreasing datacenter resources provides an accurate and efficient configuration. Three methods of deep machine learning (namely NN, CNN and LSTM) are used and compared with analytical approach to model workload and datacenter actions. Analytical model is used as predictor to evaluate and test optimization solution set and find the best configuration and scaling actions before applying it on the real datacenter. Deep machine learning together with analytical approach is used to find the best prediction values of workload demands and evaluate the scaling and resources capacity required to be provisioned. Deep machine learning is used to find optimal configuration and to solve the elasticity scaling boundaries values. %Matching the demand guarantees Service Level Agreement (SLA) conditions and Quality of Service (QoS) performance. Fourth task, resources scaling and scheduling in cloud elasticity involves timely provisioning and de-provisioning of computing resources and adjusting resources size to meet the dynamic workload demand. This requires fast and accurate resource scaling methods at minimum cost (e.g. pay as you go) that match with workload demands. Two dynamic changing parameters must be defined in an elastic model, the workload resource demand classes, and the data center resource reconfiguration classes. These parameters are not labeled for cloud management system while data center logs are being captured. A deep machine learning method is used to label datacenter configuration. Fifth task, micro-service pattern architecture with open standard API is used to integrate between all elastic cloud framework components. Full stack micro-service based elastic cloud management system is implemented considering elastic scaling and management requirements of all resources. The model focuses on elasticity scaling performance by analyzing cloud micro-service management modules in different aspects: interactions, end to end delay, and communication. It also focuses on optimizing decoupling of system components and optimizing orchestration scheduling for elastic scaling.
Machine Learning Empowered Intelligent Data Center Networking
DOWNLOAD
Author : Ting Wang
language : en
Publisher: Springer Nature
Release Date : 2023-02-21
Machine Learning Empowered Intelligent Data Center Networking written by Ting Wang and has been published by Springer Nature this book supported file pdf, txt, epub, kindle and other format this book has been release on 2023-02-21 with Computers categories.
An Introduction to the Machine Learning Empowered Intelligent Data Center Networking Fundamentals of Machine Learning in Data Center Networks. This book reviews the common learning paradigms that are widely used in data centernetworks, and offers an introduction to data collection and data processing in data centers. Additionally, it proposes a multi-dimensional and multi-perspective solution quality assessment system called REBEL-3S. The book offers readers a solid foundation for conducting research in the field of AI-assisted data center networks. Comprehensive Survey of AI-assisted Intelligent Data Center Networks. This book comprehensively investigates the peer-reviewed literature published in recent years. The wide range of machine learning techniques is fully reflected to allow fair comparisons. In addition, the book provides in-depth analysis and enlightening discussions on the effectiveness of AI in DCNs from various perspectives, covering flow prediction, flow classification, load balancing, resource management, energy management, routing optimization, congestion control, fault management, and network security. Provides a Broad Overview with Key Insights. This book introduces several novel intelligent networking concepts pioneered by real-world industries, such as Knowledge Defined Networks, Self-Driving Networks, Intent-driven Networks and Intent-based Networks. Moreover, it shares unique insights into the technological evolution of the fusion of artificial intelligence and data center networks, together with selected challenges and future research opportunities.
Intelligent And Cloud Computing
DOWNLOAD
Author : Debahuti Mishra
language : en
Publisher: Springer Nature
Release Date : 2022-04-22
Intelligent And Cloud Computing written by Debahuti Mishra and has been published by Springer Nature this book supported file pdf, txt, epub, kindle and other format this book has been release on 2022-04-22 with Technology & Engineering categories.
This book features a collection of high-quality research papers presented at the International Conference on Intelligent and Cloud Computing (ICICC 2021), held at Siksha 'O' Anusandhan (Deemed to be University), Bhubaneswar, India, during October 22–23, 2021. The book includes contributions on system and network design that can support existing and future applications and services. It covers topics such as cloud computing system and network design, optimization for cloud computing, networking, and applications, green cloud system design, cloud storage design and networking, storage security, cloud system models, big data storage, intra-cloud computing, mobile cloud system design, real-time resource reporting and monitoring for cloud management, machine learning, data mining for cloud computing, data-driven methodology and architecture, and networking for machine learning systems.
Intelligent And Cloud Computing
DOWNLOAD
Author : Debahuti Mishra
language : en
Publisher: Springer Nature
Release Date : 2020-08-28
Intelligent And Cloud Computing written by Debahuti Mishra and has been published by Springer Nature this book supported file pdf, txt, epub, kindle and other format this book has been release on 2020-08-28 with Technology & Engineering categories.
This book features a collection of high-quality research papers presented at the International Conference on Intelligent and Cloud Computing (ICICC 2019), held at Siksha 'O' Anusandhan (Deemed to be University), Bhubaneswar, India, on December 20, 2019. Including contributions on system and network design that can support existing and future applications and services, it covers topics such as cloud computing system and network design, optimization for cloud computing, networking, and applications, green cloud system design, cloud storage design and networking, storage security, cloud system models, big data storage, intra-cloud computing, mobile cloud system design, real-time resource reporting and monitoring for cloud management, machine learning, data mining for cloud computing, data-driven methodology and architecture, and networking for machine learning systems.
Hands On Machine Learning On Google Cloud Platform
DOWNLOAD
Author : Giuseppe Ciaburro
language : en
Publisher: Packt Publishing Ltd
Release Date : 2018-04-30
Hands On Machine Learning On Google Cloud Platform written by Giuseppe Ciaburro and has been published by Packt Publishing Ltd this book supported file pdf, txt, epub, kindle and other format this book has been release on 2018-04-30 with Computers categories.
Unleash Google's Cloud Platform to build, train and optimize machine learning models Key Features Get well versed in GCP pre-existing services to build your own smart models A comprehensive guide covering aspects from data processing, analyzing to building and training ML models A practical approach to produce your trained ML models and port them to your mobile for easy access Book Description Google Cloud Machine Learning Engine combines the services of Google Cloud Platform with the power and flexibility of TensorFlow. With this book, you will not only learn to build and train different complexities of machine learning models at scale but also host them in the cloud to make predictions. This book is focused on making the most of the Google Machine Learning Platform for large datasets and complex problems. You will learn from scratch how to create powerful machine learning based applications for a wide variety of problems by leveraging different data services from the Google Cloud Platform. Applications include NLP, Speech to text, Reinforcement learning, Time series, recommender systems, image classification, video content inference and many other. We will implement a wide variety of deep learning use cases and also make extensive use of data related services comprising the Google Cloud Platform ecosystem such as Firebase, Storage APIs, Datalab and so forth. This will enable you to integrate Machine Learning and data processing features into your web and mobile applications. By the end of this book, you will know the main difficulties that you may encounter and get appropriate strategies to overcome these difficulties and build efficient systems. What you will learn Use Google Cloud Platform to build data-based applications for dashboards, web, and mobile Create, train and optimize deep learning models for various data science problems on big data Learn how to leverage BigQuery to explore big datasets Use Google’s pre-trained TensorFlow models for NLP, image, video and much more Create models and architectures for Time series, Reinforcement Learning, and generative models Create, evaluate, and optimize TensorFlow and Keras models for a wide range of applications Who this book is for This book is for data scientists, machine learning developers and AI developers who want to learn Google Cloud Platform services to build machine learning applications. Since the interaction with the Google ML platform is mostly done via the command line, the reader is supposed to have some familiarity with the bash shell and Python scripting. Some understanding of machine learning and data science concepts will be handy