Machine Learning In Insurance
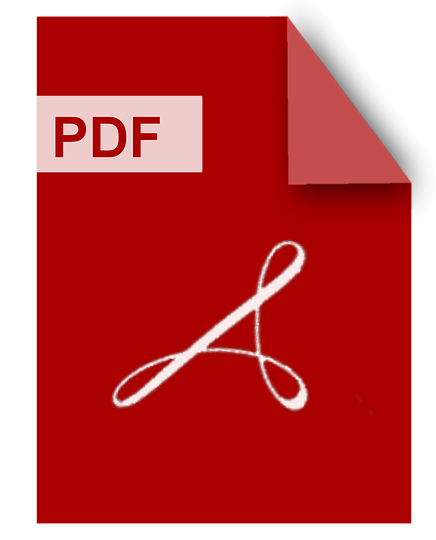
DOWNLOAD
Download Machine Learning In Insurance PDF/ePub or read online books in Mobi eBooks. Click Download or Read Online button to get Machine Learning In Insurance book now. This website allows unlimited access to, at the time of writing, more than 1.5 million titles, including hundreds of thousands of titles in various foreign languages. If the content not found or just blank you must refresh this page
Machine Learning In Insurance
DOWNLOAD
Author : Jens Perch Nielsen
language : en
Publisher: MDPI
Release Date : 2020-12-02
Machine Learning In Insurance written by Jens Perch Nielsen and has been published by MDPI this book supported file pdf, txt, epub, kindle and other format this book has been release on 2020-12-02 with Business & Economics categories.
Machine learning is a relatively new field, without a unanimous definition. In many ways, actuaries have been machine learners. In both pricing and reserving, but also more recently in capital modelling, actuaries have combined statistical methodology with a deep understanding of the problem at hand and how any solution may affect the company and its customers. One aspect that has, perhaps, not been so well developed among actuaries is validation. Discussions among actuaries’ “preferred methods” were often without solid scientific arguments, including validation of the case at hand. Through this collection, we aim to promote a good practice of machine learning in insurance, considering the following three key issues: a) who is the client, or sponsor, or otherwise interested real-life target of the study? b) The reason for working with a particular data set and a clarification of the available extra knowledge, that we also call prior knowledge, besides the data set alone. c) A mathematical statistical argument for the validation procedure.
Data Science And Machine Learning In Insurance A Gentle Introduction For Actuaries
DOWNLOAD
Author : Marco Aleandri
language : en
Publisher:
Release Date : 2019
Data Science And Machine Learning In Insurance A Gentle Introduction For Actuaries written by Marco Aleandri and has been published by this book supported file pdf, txt, epub, kindle and other format this book has been release on 2019 with Mathematics categories.
Artificial Intelligence In Insurance And Finance
DOWNLOAD
Author : Glenn Fung
language : en
Publisher: Frontiers Media SA
Release Date : 2022-01-04
Artificial Intelligence In Insurance And Finance written by Glenn Fung and has been published by Frontiers Media SA this book supported file pdf, txt, epub, kindle and other format this book has been release on 2022-01-04 with Science categories.
Luisa Fernanda Polania Cabrera is an Experienced Professional at Target Corporation (United States). Victor Wu is a Product Manager at GitLab Inc, San Francisco, United States. Sou-Cheng Choi is a Consulting Principle Data Scientist at Allstate Corporation. Lawrence Kwan Ho Ma is the Founder, Director and Chief Scientist of Valigo Limited and Founder, CEO and Chief Scientist of EMALI.IO Limited. Glenn M. Fung is the Chief Research Scientist at American Family Insurance.
Big Data For Insurance Companies
DOWNLOAD
Author : Marine Corlosquet-Habart
language : en
Publisher: John Wiley & Sons
Release Date : 2018-01-19
Big Data For Insurance Companies written by Marine Corlosquet-Habart and has been published by John Wiley & Sons this book supported file pdf, txt, epub, kindle and other format this book has been release on 2018-01-19 with Business & Economics categories.
This book will be a “must” for people who want good knowledge of big data concepts and their applications in the real world, particularly in the field of insurance. It will be useful to people working in finance and to masters students using big data tools. The authors present the bases of big data: data analysis methods, learning processes, application to insurance and position within the insurance market. Individual chapters a will be written by well-known authors in this field.
Big Data
DOWNLOAD
Author : Kiran Sood
language : en
Publisher: Emerald Group Publishing
Release Date : 2022-07-19
Big Data written by Kiran Sood and has been published by Emerald Group Publishing this book supported file pdf, txt, epub, kindle and other format this book has been release on 2022-07-19 with Business & Economics categories.
Striking a balance between the technical characteristics of the subject and the practical aspects of decision making, spanning from fraud analytics in claims management, to customer analytics, to risk analytics in solvency, the comprehensive coverage presented makes Big Data an invaluable resource for any insurance professional.
Machine Learning In Insurance
DOWNLOAD
Author : Jens Perch Nielsen
language : en
Publisher:
Release Date : 2020
Machine Learning In Insurance written by Jens Perch Nielsen and has been published by this book supported file pdf, txt, epub, kindle and other format this book has been release on 2020 with categories.
Machine learning is a relatively new field, without a unanimous definition. In many ways, actuaries have been machine learners. In both pricing and reserving, but also more recently in capital modelling, actuaries have combined statistical methodology with a deep understanding of the problem at hand and how any solution may affect the company and its customers. One aspect that has, perhaps, not been so well developed among actuaries is validation. Discussions among actuaries' “preferred methods” were often without solid scientific arguments, including validation of the case at hand. Through this collection, we aim to promote a good practice of machine learning in insurance, considering the following three key issues: a) who is the client, or sponsor, or otherwise interested real-life target of the study? b) The reason for working with a particular data set and a clarification of the available extra knowledge, that we also call prior knowledge, besides the data set alone. c) A mathematical statistical argument for the validation procedure.
Risk Prediction In Life Insurance Industry Using Machine Learning Algorithms
DOWNLOAD
Author : BOODHUN NOORHANNAH (TP031392)
language : en
Publisher:
Release Date : 2017
Risk Prediction In Life Insurance Industry Using Machine Learning Algorithms written by BOODHUN NOORHANNAH (TP031392) and has been published by this book supported file pdf, txt, epub, kindle and other format this book has been release on 2017 with categories.
The Application Of Emerging Technology And Blockchain In The Insurance Industry
DOWNLOAD
Author : Kiran Sood
language : en
Publisher: CRC Press
Release Date : 2024-02-20
The Application Of Emerging Technology And Blockchain In The Insurance Industry written by Kiran Sood and has been published by CRC Press this book supported file pdf, txt, epub, kindle and other format this book has been release on 2024-02-20 with Computers categories.
This book is a unique guide to the disruptions, innovations, and opportunities that technology provides the insurance sector and acts as an academic/industry-specific guide for creating operational effectiveness, managing risk, improving financials, and retaining customers. It also contains the current philosophy and actionable strategies from a wide range of contributors who are experts on the topic. It logically explains why traditional ways of doing business will soon become irrelevant and therefore provides an alternative choice by embracing technology. Practitioners and students alike will find value in the support for understanding practical implications of how technology has brought innovation and modern methods to measure, control, and evaluation price risk in the insurance business. It will help insurers reduce operational costs, strengthen customer interactions, target potential customers to provide usage-based insurance, and optimize the overall business. Retailers and industry giants have made significant strides in adopting digital platforms to deliver a satisfying customer experience. Insurance companies must adjust their business models and strategies to remain competitive and take advantage of technology. Insurance companies are increasingly investing in IT and related technologies to improve customer experience and reduce operational costs. Innovation through new technologies is a key driver of change in the financial sector which is often accompanied by uncertainty and doubt. This book will play a pivotal role in risk management through fraud detection, regulatory compliances, and claim settlement leading to overall satisfaction of customers.
The Digital Journey Of Banking And Insurance Volume Ii
DOWNLOAD
Author : Volker Liermann
language : en
Publisher: Springer Nature
Release Date : 2021-10-27
The Digital Journey Of Banking And Insurance Volume Ii written by Volker Liermann and has been published by Springer Nature this book supported file pdf, txt, epub, kindle and other format this book has been release on 2021-10-27 with Business & Economics categories.
This book, the second one of three volumes, gives practical examples by a number of use cases showing how to take first steps in the digital journey of banks and insurance companies. The angle shifts over the volumes from a business-driven approach in “Disruption and DNA” to a strong technical focus in “Data Storage, Processing and Analysis”, leaving “Digitalization and Machine Learning Applications” with the business and technical aspects in-between. This second volume mainly emphasizes use cases as well as the methods and technologies applied to drive digital transformation (such as processes, leveraging computational power and machine learning models).
Predicting Risk Level For Life Insurance Using Machine Learning Algorithms
DOWNLOAD
Author : WANG BAOLING (TP051988)
language : en
Publisher:
Release Date : 2019
Predicting Risk Level For Life Insurance Using Machine Learning Algorithms written by WANG BAOLING (TP051988) and has been published by this book supported file pdf, txt, epub, kindle and other format this book has been release on 2019 with Computer algorithms categories.
In the era of big data expansion, how to use data to improve risk assessment is a key point for insurance companies. The underwriting process is the starting step of an insurance policy and the first customer touch point. The life insurance underwriters currently face the problem of how to find a solution to improve the accuracy of risk assessment and service efficiency at the same time. This article proposes a solution with three research objectives for underwriters to overcome this predicament. As the high dimensional dataset became the common challenge for insurance dataset, the first objective would be demonstrating the impact between different dimension reduction techniques. One filter method and one wrapper method of feature selection will be applied in this research. The second objective would be identifying the most key risk factors for risk assessment in underwriting, in order to improve the quality of data collection for better risk management. And the last objective is comparing the performance between different machine learning algorithms. In this research, Multiple Linear Regression (MLR), XBoost, Support Vector Regression (SVR) and Stacking Ensemble model be trained according to those two feature selection methods. As the results, overall the models built based on wrapper method have the better performance, meanwhile, Stacking Ensemble model achieved the best performance with RMSE as 1.92 and MAE as 1.45, respectively. Furthermore, this study also analysed the most significant factors that influence the risk level most according t the feature selection methods and models.