Machine Learning In Non Stationary Environments
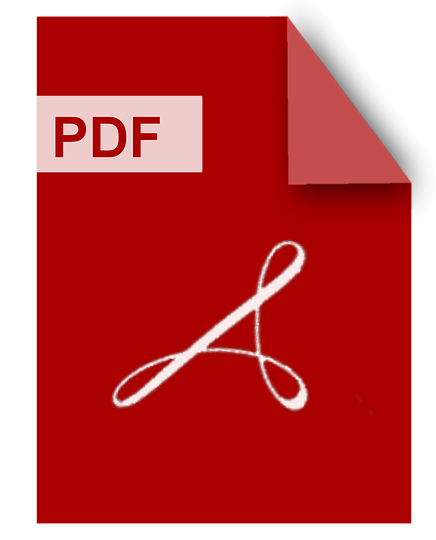
DOWNLOAD eBooks
Download Machine Learning In Non Stationary Environments PDF/ePub or read online books in Mobi eBooks. Click Download or Read Online button to get Machine Learning In Non Stationary Environments book now. This website allows unlimited access to, at the time of writing, more than 1.5 million titles, including hundreds of thousands of titles in various foreign languages. If the content not found or just blank you must refresh this page
Machine Learning In Non Stationary Environments
DOWNLOAD eBooks
Author : Masashi Sugiyama
language : en
Publisher: MIT Press
Release Date : 2012
Machine Learning In Non Stationary Environments written by Masashi Sugiyama and has been published by MIT Press this book supported file pdf, txt, epub, kindle and other format this book has been release on 2012 with Computers categories.
Dealing with non-stationarity is one of modem machine learning's greatest challenges. This book focuses on a specific non-stationary environment known as covariate shift, in which the distributions of inputs (queries) change but the conditional distribution of outputs (answers) is unchanged, and presents machine learning theory, algorithms, and applications to overcome this variety of non-stationarity.
Machine Learning In Non Stationary Environments
DOWNLOAD eBooks
Author : Masashi Sugiyama
language : en
Publisher: MIT Press
Release Date : 2012-03-30
Machine Learning In Non Stationary Environments written by Masashi Sugiyama and has been published by MIT Press this book supported file pdf, txt, epub, kindle and other format this book has been release on 2012-03-30 with Computers categories.
Theory, algorithms, and applications of machine learning techniques to overcome “covariate shift” non-stationarity. As the power of computing has grown over the past few decades, the field of machine learning has advanced rapidly in both theory and practice. Machine learning methods are usually based on the assumption that the data generation mechanism does not change over time. Yet real-world applications of machine learning, including image recognition, natural language processing, speech recognition, robot control, and bioinformatics, often violate this common assumption. Dealing with non-stationarity is one of modern machine learning's greatest challenges. This book focuses on a specific non-stationary environment known as covariate shift, in which the distributions of inputs (queries) change but the conditional distribution of outputs (answers) is unchanged, and presents machine learning theory, algorithms, and applications to overcome this variety of non-stationarity. After reviewing the state-of-the-art research in the field, the authors discuss topics that include learning under covariate shift, model selection, importance estimation, and active learning. They describe such real world applications of covariate shift adaption as brain-computer interface, speaker identification, and age prediction from facial images. With this book, they aim to encourage future research in machine learning, statistics, and engineering that strives to create truly autonomous learning machines able to learn under non-stationarity.
Learning In Non Stationary Environments
DOWNLOAD eBooks
Author : Moamar Sayed-Mouchaweh
language : en
Publisher: Springer Science & Business Media
Release Date : 2012-04-13
Learning In Non Stationary Environments written by Moamar Sayed-Mouchaweh and has been published by Springer Science & Business Media this book supported file pdf, txt, epub, kindle and other format this book has been release on 2012-04-13 with Technology & Engineering categories.
Recent decades have seen rapid advances in automatization processes, supported by modern machines and computers. The result is significant increases in system complexity and state changes, information sources, the need for faster data handling and the integration of environmental influences. Intelligent systems, equipped with a taxonomy of data-driven system identification and machine learning algorithms, can handle these problems partially. Conventional learning algorithms in a batch off-line setting fail whenever dynamic changes of the process appear due to non-stationary environments and external influences. Learning in Non-Stationary Environments: Methods and Applications offers a wide-ranging, comprehensive review of recent developments and important methodologies in the field. The coverage focuses on dynamic learning in unsupervised problems, dynamic learning in supervised classification and dynamic learning in supervised regression problems. A later section is dedicated to applications in which dynamic learning methods serve as keystones for achieving models with high accuracy. Rather than rely on a mathematical theorem/proof style, the editors highlight numerous figures, tables, examples and applications, together with their explanations. This approach offers a useful basis for further investigation and fresh ideas and motivates and inspires newcomers to explore this promising and still emerging field of research.
Machine Learning In Non Stationary Environments
DOWNLOAD eBooks
Author : Motoaki Kawanabe
language : en
Publisher:
Release Date :
Machine Learning In Non Stationary Environments written by Motoaki Kawanabe and has been published by this book supported file pdf, txt, epub, kindle and other format this book has been release on with categories.
Theory, algorithms, and applications of machine learning techniques to overcome "covariate shift" non-stationarity.
Learning In Non Stationary Environments
DOWNLOAD eBooks
Author : Springer
language : en
Publisher:
Release Date : 2012-04-01
Learning In Non Stationary Environments written by Springer and has been published by this book supported file pdf, txt, epub, kindle and other format this book has been release on 2012-04-01 with categories.
Learning From Data Streams In Evolving Environments
DOWNLOAD eBooks
Author : Moamar Sayed-Mouchaweh
language : en
Publisher: Springer
Release Date : 2018-07-28
Learning From Data Streams In Evolving Environments written by Moamar Sayed-Mouchaweh and has been published by Springer this book supported file pdf, txt, epub, kindle and other format this book has been release on 2018-07-28 with Technology & Engineering categories.
This edited book covers recent advances of techniques, methods and tools treating the problem of learning from data streams generated by evolving non-stationary processes. The goal is to discuss and overview the advanced techniques, methods and tools that are dedicated to manage, exploit and interpret data streams in non-stationary environments. The book includes the required notions, definitions, and background to understand the problem of learning from data streams in non-stationary environments and synthesizes the state-of-the-art in the domain, discussing advanced aspects and concepts and presenting open problems and future challenges in this field. Provides multiple examples to facilitate the understanding data streams in non-stationary environments; Presents several application cases to show how the methods solve different real world problems; Discusses the links between methods to help stimulate new research and application directions.
Special Topics In Information Technology
DOWNLOAD eBooks
Author : Luigi Piroddi
language : en
Publisher: Springer Nature
Release Date : 2022-01-01
Special Topics In Information Technology written by Luigi Piroddi and has been published by Springer Nature this book supported file pdf, txt, epub, kindle and other format this book has been release on 2022-01-01 with Technology & Engineering categories.
This open access book presents thirteen outstanding doctoral dissertations in Information Technology from the Department of Electronics, Information and Bioengineering, Politecnico di Milano, Italy. Information Technology has always been highly interdisciplinary, as many aspects have to be considered in IT systems. The doctoral studies program in IT at Politecnico di Milano emphasizes this interdisciplinary nature, which is becoming more and more important in recent technological advances, in collaborative projects, and in the education of young researchers. Accordingly, the focus of advanced research is on pursuing a rigorous approach to specific research topics starting from a broad background in various areas of Information Technology, especially Computer Science and Engineering, Electronics, Systems and Control, and Telecommunications. Each year, more than 50 PhDs graduate from the program. This book gathers the outcomes of the thirteen best theses defended in 2020-21 and selected for the IT PhD Award. Each of the authors provides a chapter summarizing his/her findings, including an introduction, description of methods, main achievements and future work on the topic. Hence, the book provides a cutting-edge overview of the latest research trends in Information Technology at Politecnico di Milano, presented in an easy-to-read format that will also appeal to non-specialists.
Reinforcement Learning Second Edition
DOWNLOAD eBooks
Author : Richard S. Sutton
language : en
Publisher: MIT Press
Release Date : 2018-11-13
Reinforcement Learning Second Edition written by Richard S. Sutton and has been published by MIT Press this book supported file pdf, txt, epub, kindle and other format this book has been release on 2018-11-13 with Computers categories.
The significantly expanded and updated new edition of a widely used text on reinforcement learning, one of the most active research areas in artificial intelligence. Reinforcement learning, one of the most active research areas in artificial intelligence, is a computational approach to learning whereby an agent tries to maximize the total amount of reward it receives while interacting with a complex, uncertain environment. In Reinforcement Learning, Richard Sutton and Andrew Barto provide a clear and simple account of the field's key ideas and algorithms. This second edition has been significantly expanded and updated, presenting new topics and updating coverage of other topics. Like the first edition, this second edition focuses on core online learning algorithms, with the more mathematical material set off in shaded boxes. Part I covers as much of reinforcement learning as possible without going beyond the tabular case for which exact solutions can be found. Many algorithms presented in this part are new to the second edition, including UCB, Expected Sarsa, and Double Learning. Part II extends these ideas to function approximation, with new sections on such topics as artificial neural networks and the Fourier basis, and offers expanded treatment of off-policy learning and policy-gradient methods. Part III has new chapters on reinforcement learning's relationships to psychology and neuroscience, as well as an updated case-studies chapter including AlphaGo and AlphaGo Zero, Atari game playing, and IBM Watson's wagering strategy. The final chapter discusses the future societal impacts of reinforcement learning.
Statistical Reinforcement Learning
DOWNLOAD eBooks
Author : Masashi Sugiyama
language : en
Publisher: CRC Press
Release Date : 2015-03-16
Statistical Reinforcement Learning written by Masashi Sugiyama and has been published by CRC Press this book supported file pdf, txt, epub, kindle and other format this book has been release on 2015-03-16 with Business & Economics categories.
Reinforcement learning is a mathematical framework for developing computer agents that can learn an optimal behavior by relating generic reward signals with its past actions. With numerous successful applications in business intelligence, plant control, and gaming, the RL framework is ideal for decision making in unknown environments with large amo
Metaheuristics
DOWNLOAD eBooks
Author : Mauricio G.C. Resende
language : en
Publisher: Springer Science & Business Media
Release Date : 2003-11-30
Metaheuristics written by Mauricio G.C. Resende and has been published by Springer Science & Business Media this book supported file pdf, txt, epub, kindle and other format this book has been release on 2003-11-30 with Computers categories.
Combinatorial optimization is the process of finding the best, or optimal, so lution for problems with a discrete set of feasible solutions. Applications arise in numerous settings involving operations management and logistics, such as routing, scheduling, packing, inventory and production management, lo cation, logic, and assignment of resources. The economic impact of combi natorial optimization is profound, affecting sectors as diverse as transporta tion (airlines, trucking, rail, and shipping), forestry, manufacturing, logistics, aerospace, energy (electrical power, petroleum, and natural gas), telecommu nications, biotechnology, financial services, and agriculture. While much progress has been made in finding exact (provably optimal) so lutions to some combinatorial optimization problems, using techniques such as dynamic programming, cutting planes, and branch and cut methods, many hard combinatorial problems are still not solved exactly and require good heuristic methods. Moreover, reaching "optimal solutions" is in many cases meaningless, as in practice we are often dealing with models that are rough simplifications of reality. The aim of heuristic methods for combinatorial op timization is to quickly produce good-quality solutions, without necessarily providing any guarantee of solution quality. Metaheuristics are high level procedures that coordinate simple heuristics, such as local search, to find solu tions that are of better quality than those found by the simple heuristics alone: Modem metaheuristics include simulated annealing, genetic algorithms, tabu search, GRASP, scatter search, ant colony optimization, variable neighborhood search, and their hybrids.