Math For Machine Learning
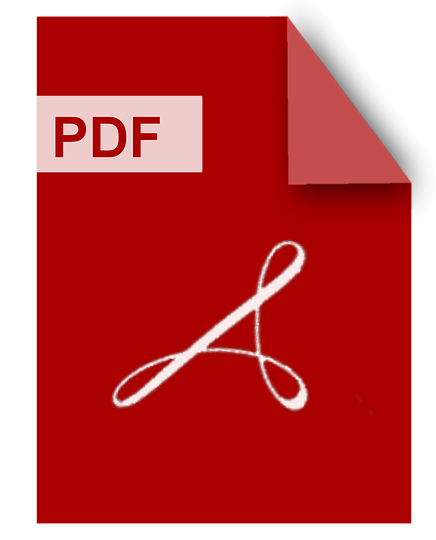
DOWNLOAD
Download Math For Machine Learning PDF/ePub or read online books in Mobi eBooks. Click Download or Read Online button to get Math For Machine Learning book now. This website allows unlimited access to, at the time of writing, more than 1.5 million titles, including hundreds of thousands of titles in various foreign languages. If the content not found or just blank you must refresh this page
Mathematics For Machine Learning
DOWNLOAD
Author : Marc Peter Deisenroth
language : en
Publisher: Cambridge University Press
Release Date : 2020-04-23
Mathematics For Machine Learning written by Marc Peter Deisenroth and has been published by Cambridge University Press this book supported file pdf, txt, epub, kindle and other format this book has been release on 2020-04-23 with Computers categories.
Distills key concepts from linear algebra, geometry, matrices, calculus, optimization, probability and statistics that are used in machine learning.
Mathematics For Machine Learning
DOWNLOAD
Author : Marc Peter Deisenroth
language : en
Publisher: Cambridge University Press
Release Date : 2020-04-23
Mathematics For Machine Learning written by Marc Peter Deisenroth and has been published by Cambridge University Press this book supported file pdf, txt, epub, kindle and other format this book has been release on 2020-04-23 with Computers categories.
The fundamental mathematical tools needed to understand machine learning include linear algebra, analytic geometry, matrix decompositions, vector calculus, optimization, probability and statistics. These topics are traditionally taught in disparate courses, making it hard for data science or computer science students, or professionals, to efficiently learn the mathematics. This self-contained textbook bridges the gap between mathematical and machine learning texts, introducing the mathematical concepts with a minimum of prerequisites. It uses these concepts to derive four central machine learning methods: linear regression, principal component analysis, Gaussian mixture models and support vector machines. For students and others with a mathematical background, these derivations provide a starting point to machine learning texts. For those learning the mathematics for the first time, the methods help build intuition and practical experience with applying mathematical concepts. Every chapter includes worked examples and exercises to test understanding. Programming tutorials are offered on the book's web site.
Math For Deep Learning
DOWNLOAD
Author : Ronald T. Kneusel
language : en
Publisher: No Starch Press
Release Date : 2021-12-07
Math For Deep Learning written by Ronald T. Kneusel and has been published by No Starch Press this book supported file pdf, txt, epub, kindle and other format this book has been release on 2021-12-07 with Computers categories.
Math for Deep Learning provides the essential math you need to understand deep learning discussions, explore more complex implementations, and better use the deep learning toolkits. With Math for Deep Learning, you'll learn the essential mathematics used by and as a background for deep learning. You’ll work through Python examples to learn key deep learning related topics in probability, statistics, linear algebra, differential calculus, and matrix calculus as well as how to implement data flow in a neural network, backpropagation, and gradient descent. You’ll also use Python to work through the mathematics that underlies those algorithms and even build a fully-functional neural network. In addition you’ll find coverage of gradient descent including variations commonly used by the deep learning community: SGD, Adam, RMSprop, and Adagrad/Adadelta.
Math And Architectures Of Deep Learning
DOWNLOAD
Author : Krishnendu Chaudhury
language : en
Publisher: Simon and Schuster
Release Date : 2024-05-21
Math And Architectures Of Deep Learning written by Krishnendu Chaudhury and has been published by Simon and Schuster this book supported file pdf, txt, epub, kindle and other format this book has been release on 2024-05-21 with Computers categories.
Shine a spotlight into the deep learning “black box”. This comprehensive and detailed guide reveals the mathematical and architectural concepts behind deep learning models, so you can customize, maintain, and explain them more effectively. Inside Math and Architectures of Deep Learning you will find: Math, theory, and programming principles side by side Linear algebra, vector calculus and multivariate statistics for deep learning The structure of neural networks Implementing deep learning architectures with Python and PyTorch Troubleshooting underperforming models Working code samples in downloadable Jupyter notebooks The mathematical paradigms behind deep learning models typically begin as hard-to-read academic papers that leave engineers in the dark about how those models actually function. Math and Architectures of Deep Learning bridges the gap between theory and practice, laying out the math of deep learning side by side with practical implementations in Python and PyTorch. Written by deep learning expert Krishnendu Chaudhury, you’ll peer inside the “black box” to understand how your code is working, and learn to comprehend cutting-edge research you can turn into practical applications. Foreword by Prith Banerjee. About the technology Discover what’s going on inside the black box! To work with deep learning you’ll have to choose the right model, train it, preprocess your data, evaluate performance and accuracy, and deal with uncertainty and variability in the outputs of a deployed solution. This book takes you systematically through the core mathematical concepts you’ll need as a working data scientist: vector calculus, linear algebra, and Bayesian inference, all from a deep learning perspective. About the book Math and Architectures of Deep Learning teaches the math, theory, and programming principles of deep learning models laid out side by side, and then puts them into practice with well-annotated Python code. You’ll progress from algebra, calculus, and statistics all the way to state-of-the-art DL architectures taken from the latest research. What's inside The core design principles of neural networks Implementing deep learning with Python and PyTorch Regularizing and optimizing underperforming models About the reader Readers need to know Python and the basics of algebra and calculus. About the author Krishnendu Chaudhury is co-founder and CTO of the AI startup Drishti Technologies. He previously spent a decade each at Google and Adobe. Table of Contents 1 An overview of machine learning and deep learning 2 Vectors, matrices, and tensors in machine learning 3 Classifiers and vector calculus 4 Linear algebraic tools in machine learning 5 Probability distributions in machine learning 6 Bayesian tools for machine learning 7 Function approximation: How neural networks model the world 8 Training neural networks: Forward propagation and backpropagation 9 Loss, optimization, and regularization 10 Convolutions in neural networks 11 Neural networks for image classification and object detection 12 Manifolds, homeomorphism, and neural networks 13 Fully Bayes model parameter estimation 14 Latent space and generative modeling, autoencoders, and variational autoencoders A Appendix
Hands On Mathematics For Deep Learning
DOWNLOAD
Author : Jay Dawani
language : en
Publisher: Packt Publishing Ltd
Release Date : 2020-06-12
Hands On Mathematics For Deep Learning written by Jay Dawani and has been published by Packt Publishing Ltd this book supported file pdf, txt, epub, kindle and other format this book has been release on 2020-06-12 with Computers categories.
A comprehensive guide to getting well-versed with the mathematical techniques for building modern deep learning architectures Key FeaturesUnderstand linear algebra, calculus, gradient algorithms, and other concepts essential for training deep neural networksLearn the mathematical concepts needed to understand how deep learning models functionUse deep learning for solving problems related to vision, image, text, and sequence applicationsBook Description Most programmers and data scientists struggle with mathematics, having either overlooked or forgotten core mathematical concepts. This book uses Python libraries to help you understand the math required to build deep learning (DL) models. You'll begin by learning about core mathematical and modern computational techniques used to design and implement DL algorithms. This book will cover essential topics, such as linear algebra, eigenvalues and eigenvectors, the singular value decomposition concept, and gradient algorithms, to help you understand how to train deep neural networks. Later chapters focus on important neural networks, such as the linear neural network and multilayer perceptrons, with a primary focus on helping you learn how each model works. As you advance, you will delve into the math used for regularization, multi-layered DL, forward propagation, optimization, and backpropagation techniques to understand what it takes to build full-fledged DL models. Finally, you’ll explore CNN, recurrent neural network (RNN), and GAN models and their application. By the end of this book, you'll have built a strong foundation in neural networks and DL mathematical concepts, which will help you to confidently research and build custom models in DL. What you will learnUnderstand the key mathematical concepts for building neural network modelsDiscover core multivariable calculus conceptsImprove the performance of deep learning models using optimization techniquesCover optimization algorithms, from basic stochastic gradient descent (SGD) to the advanced Adam optimizerUnderstand computational graphs and their importance in DLExplore the backpropagation algorithm to reduce output errorCover DL algorithms such as convolutional neural networks (CNNs), sequence models, and generative adversarial networks (GANs)Who this book is for This book is for data scientists, machine learning developers, aspiring deep learning developers, or anyone who wants to understand the foundation of deep learning by learning the math behind it. Working knowledge of the Python programming language and machine learning basics is required.
Deep Learning
DOWNLOAD
Author : Ian Goodfellow
language : en
Publisher: MIT Press
Release Date : 2016-11-10
Deep Learning written by Ian Goodfellow and has been published by MIT Press this book supported file pdf, txt, epub, kindle and other format this book has been release on 2016-11-10 with Computers categories.
An introduction to a broad range of topics in deep learning, covering mathematical and conceptual background, deep learning techniques used in industry, and research perspectives. “Written by three experts in the field, Deep Learning is the only comprehensive book on the subject.” —Elon Musk, cochair of OpenAI; cofounder and CEO of Tesla and SpaceX Deep learning is a form of machine learning that enables computers to learn from experience and understand the world in terms of a hierarchy of concepts. Because the computer gathers knowledge from experience, there is no need for a human computer operator to formally specify all the knowledge that the computer needs. The hierarchy of concepts allows the computer to learn complicated concepts by building them out of simpler ones; a graph of these hierarchies would be many layers deep. This book introduces a broad range of topics in deep learning. The text offers mathematical and conceptual background, covering relevant concepts in linear algebra, probability theory and information theory, numerical computation, and machine learning. It describes deep learning techniques used by practitioners in industry, including deep feedforward networks, regularization, optimization algorithms, convolutional networks, sequence modeling, and practical methodology; and it surveys such applications as natural language processing, speech recognition, computer vision, online recommendation systems, bioinformatics, and videogames. Finally, the book offers research perspectives, covering such theoretical topics as linear factor models, autoencoders, representation learning, structured probabilistic models, Monte Carlo methods, the partition function, approximate inference, and deep generative models. Deep Learning can be used by undergraduate or graduate students planning careers in either industry or research, and by software engineers who want to begin using deep learning in their products or platforms. A website offers supplementary material for both readers and instructors.
Machine Learning Math
DOWNLOAD
Author : ML and AI Academy
language : en
Publisher:
Release Date : 2021-02-14
Machine Learning Math written by ML and AI Academy and has been published by this book supported file pdf, txt, epub, kindle and other format this book has been release on 2021-02-14 with categories.
!! 55% OFF for Bookstores!! NOW at 29,95 instead of 39.95 !! Buy it NOW and let your customers get addicted to this awesome book!
Mathematical Theories Of Machine Learning Theory And Applications
DOWNLOAD
Author : Bin Shi
language : en
Publisher: Springer
Release Date : 2019-06-12
Mathematical Theories Of Machine Learning Theory And Applications written by Bin Shi and has been published by Springer this book supported file pdf, txt, epub, kindle and other format this book has been release on 2019-06-12 with Technology & Engineering categories.
This book studies mathematical theories of machine learning. The first part of the book explores the optimality and adaptivity of choosing step sizes of gradient descent for escaping strict saddle points in non-convex optimization problems. In the second part, the authors propose algorithms to find local minima in nonconvex optimization and to obtain global minima in some degree from the Newton Second Law without friction. In the third part, the authors study the problem of subspace clustering with noisy and missing data, which is a problem well-motivated by practical applications data subject to stochastic Gaussian noise and/or incomplete data with uniformly missing entries. In the last part, the authors introduce an novel VAR model with Elastic-Net regularization and its equivalent Bayesian model allowing for both a stable sparsity and a group selection.
Mathematics And Programming For Machine Learning With R
DOWNLOAD
Author : William B. Claster
language : en
Publisher:
Release Date : 2020
Mathematics And Programming For Machine Learning With R written by William B. Claster and has been published by this book supported file pdf, txt, epub, kindle and other format this book has been release on 2020 with Machine learning categories.
Based on the author's experience teaching data science for more than 10 years, Mathematics and R Programming for Machine Learningreveals how machine learning algorithms do their magic and explains how logic can be implemented in code. It is designed to give students an understanding of the logic behind machine learning algorithms as well as how to program these algorithms. Written for novice programmers, the book goes step-by-step to develop coding skills needed to implement algorithms in R. The text begins with simple implementations and fundamental concepts of logic, sets, and probability before moving to coverage of powerful deep learning algorithms. The first eight chapters deal with probability-based machine learning algorithms, and the last eight chapters deal with artificial neural network-based machine learning. The first half of the text does not require mathematical sophistication, although familiarity with probability and statistics is helpful. The second half is written for students who have taken one semester of calculus. The book guides students, who are novice R programmers, through algorithms and their application to improve the ability to code and confidence in programming R and tackling advance R programming challenges. Highlights of the book include: More than 400 exercises A strong emphasis on improving programming skills and guiding beginners on implementing full-fledged algorithms. Coverage of fundamental computer and mathematical concepts including logic, sets, and probability In-depth explanations of the heart of AI and machine learning as well as the mechanisms that underly machine learning algorithms
Statistical Learning With Math And Python
DOWNLOAD
Author : Joe Suzuki
language : en
Publisher: Springer Nature
Release Date : 2021-08-03
Statistical Learning With Math And Python written by Joe Suzuki and has been published by Springer Nature this book supported file pdf, txt, epub, kindle and other format this book has been release on 2021-08-03 with Computers categories.
The most crucial ability for machine learning and data science is mathematical logic for grasping their essence rather than knowledge and experience. This textbook approaches the essence of machine learning and data science by considering math problems and building Python programs. As the preliminary part, Chapter 1 provides a concise introduction to linear algebra, which will help novices read further to the following main chapters. Those succeeding chapters present essential topics in statistical learning: linear regression, classification, resampling, information criteria, regularization, nonlinear regression, decision trees, support vector machines, and unsupervised learning. Each chapter mathematically formulates and solves machine learning problems and builds the programs. The body of a chapter is accompanied by proofs and programs in an appendix, with exercises at the end of the chapter. Because the book is carefully organized to provide the solutions to the exercises in each chapter, readers can solve the total of 100 exercises by simply following the contents of each chapter. This textbook is suitable for an undergraduate or graduate course consisting of about 12 lectures. Written in an easy-to-follow and self-contained style, this book will also be perfect material for independent learning.