Maximum Likelihood Estimation And Inference For High Dimensional Nonlinear Factor Models
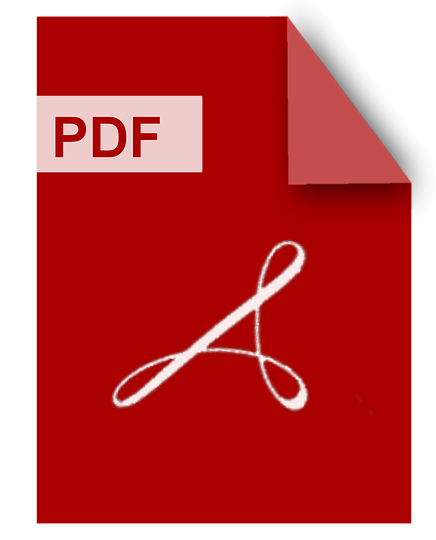
DOWNLOAD
Download Maximum Likelihood Estimation And Inference For High Dimensional Nonlinear Factor Models PDF/ePub or read online books in Mobi eBooks. Click Download or Read Online button to get Maximum Likelihood Estimation And Inference For High Dimensional Nonlinear Factor Models book now. This website allows unlimited access to, at the time of writing, more than 1.5 million titles, including hundreds of thousands of titles in various foreign languages. If the content not found or just blank you must refresh this page
Maximum Likelihood Estimation And Inference For High Dimensional Nonlinear Factor Models
DOWNLOAD
Author : Fa Wang
language : en
Publisher:
Release Date : 2017
Maximum Likelihood Estimation And Inference For High Dimensional Nonlinear Factor Models written by Fa Wang and has been published by this book supported file pdf, txt, epub, kindle and other format this book has been release on 2017 with categories.
Maximum Likelihood Estimation And Inference For High Dimensional Generalized Factor Models With Application To Factor Augmented Regressions
DOWNLOAD
Author : Fa Wang
language : en
Publisher:
Release Date : 2021
Maximum Likelihood Estimation And Inference For High Dimensional Generalized Factor Models With Application To Factor Augmented Regressions written by Fa Wang and has been published by this book supported file pdf, txt, epub, kindle and other format this book has been release on 2021 with categories.
This paper reestablishes the main results in Bai (2003) and Bai and Ng(2006) for generalized factor models, with slightly stronger conditions on therelative magnitude of N(number of subjects) and T(number of time periods).Convergence rates of the estimated factor space and loading space and asymptotic normality of the estimated factors and loadings are established under mildconditions that allow for linear, Logit, Probit, Tobit, Poisson and some othersingle-index nonlinear models. The probability density/mass function is allowed to vary across subjects and time, thus mixed models are also allowed for.For factor-augmented regressions, this paper establishes the limit distributionsof the parameter estimates, the conditional mean, and the forecast when factorsestimated from nonlinear/mixed data are used as proxies for the true factors.
Maximum Likelihood Estimation Of Time Varying Loadings In High Dimensional Factor Models
DOWNLOAD
Author : Jakob Guldbæk Mikkelsen
language : en
Publisher:
Release Date : 2015
Maximum Likelihood Estimation Of Time Varying Loadings In High Dimensional Factor Models written by Jakob Guldbæk Mikkelsen and has been published by this book supported file pdf, txt, epub, kindle and other format this book has been release on 2015 with categories.
Maximum Likelihood Estimation And Inference
DOWNLOAD
Author : Russell B. Millar
language : en
Publisher: John Wiley & Sons
Release Date : 2011-07-26
Maximum Likelihood Estimation And Inference written by Russell B. Millar and has been published by John Wiley & Sons this book supported file pdf, txt, epub, kindle and other format this book has been release on 2011-07-26 with Mathematics categories.
This book takes a fresh look at the popular and well-established method of maximum likelihood for statistical estimation and inference. It begins with an intuitive introduction to the concepts and background of likelihood, and moves through to the latest developments in maximum likelihood methodology, including general latent variable models and new material for the practical implementation of integrated likelihood using the free ADMB software. Fundamental issues of statistical inference are also examined, with a presentation of some of the philosophical debates underlying the choice of statistical paradigm. Key features: Provides an accessible introduction to pragmatic maximum likelihood modelling. Covers more advanced topics, including general forms of latent variable models (including non-linear and non-normal mixed-effects and state-space models) and the use of maximum likelihood variants, such as estimating equations, conditional likelihood, restricted likelihood and integrated likelihood. Adopts a practical approach, with a focus on providing the relevant tools required by researchers and practitioners who collect and analyze real data. Presents numerous examples and case studies across a wide range of applications including medicine, biology and ecology. Features applications from a range of disciplines, with implementation in R, SAS and/or ADMB. Provides all program code and software extensions on a supporting website. Confines supporting theory to the final chapters to maintain a readable and pragmatic focus of the preceding chapters. This book is not just an accessible and practical text about maximum likelihood, it is a comprehensive guide to modern maximum likelihood estimation and inference. It will be of interest to readers of all levels, from novice to expert. It will be of great benefit to researchers, and to students of statistics from senior undergraduate to graduate level. For use as a course text, exercises are provided at the end of each chapter.
Maximum Likelihood Estimation Of Time Varying Loadings In High Dimensional Factor Models
DOWNLOAD
Author :
language : en
Publisher:
Release Date : 2017
Maximum Likelihood Estimation Of Time Varying Loadings In High Dimensional Factor Models written by and has been published by this book supported file pdf, txt, epub, kindle and other format this book has been release on 2017 with categories.
Maximum Likelihood Estimation For Dynamic Factor Models With Missing Data
DOWNLOAD
Author : Borus Jungbacker
language : en
Publisher:
Release Date : 2011
Maximum Likelihood Estimation For Dynamic Factor Models With Missing Data written by Borus Jungbacker and has been published by this book supported file pdf, txt, epub, kindle and other format this book has been release on 2011 with categories.
This paper concerns estimating parameters in a high-dimensional dynamic factor model by the method of maximum likelihood. To accommodate missing data in the analysis, we propose a new model representation for the dynamic factor model. It allows the Kalman filter and related smoothing methods to evaluate the likelihood function and to produce optimal factor estimates in a computationally efficient way when missing data is present. The implementation details of our methods for signal extraction and maximum likelihood estimation are discussed. The computational gains of the new devices are presented based on simulated data sets with varying numbers of missing entries.
Quasi Maximum Likelihood Analysis Of High Dimensional Constrained Factor Models
DOWNLOAD
Author : Kunpeng Li
language : en
Publisher:
Release Date : 2019
Quasi Maximum Likelihood Analysis Of High Dimensional Constrained Factor Models written by Kunpeng Li and has been published by this book supported file pdf, txt, epub, kindle and other format this book has been release on 2019 with categories.
Factor models have been widely used in practice. However, an undesirable feature of a high dimensional factor model is that the model has too many parameters. An effective way to address this issue, proposed in a seminal work by Tsai and Tsay (2010), is to decompose the loadings matrix by a high-dimensional known matrix multiplying with a low-dimensional unknown matrix, which Tsai and Tsay (2010) name the constrained factor models. This paper investigates the estimation and inferential theory of constrained factor models under large-N and large-T setup, where N denotes the number of cross sectional units and T the time periods. We propose using the quasi maximum likelihood method to estimate the model and investigate the asymptotic properties of the quasi maximum likelihood estimators, including consistency, rates of convergence and limiting distributions. A new statistic is proposed for testing the null hypothesis of constrained factor models against the alternative of standard factor models. Partially constrained factor models are also investigated. Monte carlo simulations confirm our theoretical results and show that the quasi maximum likelihood estimators and the proposed new statistic perform well in finite samples. We also consider the extension to an approximate constrained factor model where the idiosyncratic errors are allowed to be weakly dependent processes.
Estimation And Inference In High Dimensional Models
DOWNLOAD
Author : Mojtaba Sahraee Ardakan
language : en
Publisher:
Release Date : 2022
Estimation And Inference In High Dimensional Models written by Mojtaba Sahraee Ardakan and has been published by this book supported file pdf, txt, epub, kindle and other format this book has been release on 2022 with categories.
A wide variety of problems that are encountered in different fields can be formulated as an inference problem. Common examples of such inference problems include estimating parameters of a model from some observations, inverse problems where an unobserved signal is to be estimated based on a given model and some measurements, or a combination of the two where hidden signals along with some parameters of the model are to be estimated jointly. For example, various tasks in machine learning such as image inpainting and super-resolution can be cast as an inverse problem over deep neural networks. Similarly, in computational neuroscience, a common task is to estimate the parameters of a nonlinear dynamical system from neuronal activities. Despite wide application of different models and algorithms to solve these problems, our theoretical understanding of how these algorithms work is often incomplete. In this work, we try to bridge the gap between theory and practice by providing theoretical analysis of three different estimation problems. First, we consider the problem of estimating the input and hidden layer signals in a given multi-layer stochastic neural network with all the signals being matrix valued. Various problems such as multitask regression and classification, and inverse problems that use deep generative priors can be modeled as inference problem over multi-layer neural networks. We consider different types of estimators for such problems and exactly analyze the performance of these estimators in a certain high-dimensional regime known as the large system limit. Our analysis allows us to obtain the estimation error of all the hidden signals in the deep neural network as expectations over low-dimensional random variables that are characterized via a set of equations called the state evolution. Next, we analyze the problem of estimating a signal from convolutional observations via ridge estimation. Such convolutional inverse problems arise naturally in several fields such as imaging and seismology. The shared weights of the convolution operator introduces dependencies in the observations that makes analysis of such estimators difficult. By looking at the problem in the Fourier domain and using results about Fourier transform of a class of random processes, we show that this problem can be reduced to analysis of multiple ordinary ridge estimators, one for each frequency. This allows us to write the estimation error of the ridge estimator as an integral that depends on the spectrum of the underlying random process that generates the input features. Finally, we conclude this work by considering the problem of estimating the parameters of a multi-dimensional autoregressive generalized linear model with discrete values. Such processes take a linear combination of the past outputs of the process as the mean parameter of a generalized linear model that generates the future values. The coefficients of the linear combination are the parameters of the model and we seek to estimate these parameters under the assumption that they are sparse. This model can be used for example to model the spiking activity of neurons. In this problem, we obtain a high-probability upper bound for the estimation error of the parameters. Our experiments further support these theoretical results.
Maximum Likelihood Estimation
DOWNLOAD
Author : Scott R. Eliason
language : en
Publisher: SAGE
Release Date : 1993
Maximum Likelihood Estimation written by Scott R. Eliason and has been published by SAGE this book supported file pdf, txt, epub, kindle and other format this book has been release on 1993 with Mathematics categories.
This is a short introduction to Maximum Likelihood (ML) Estimation. It provides a general modeling framework that utilizes the tools of ML methods to outline a flexible modeling strategy that accommodates cases from the simplest linear models (such as the normal error regression model) to the most complex nonlinear models linking endogenous and exogenous variables with non-normal distributions. Using examples to illustrate the techniques of finding ML estimators and estimates, the author discusses what properties are desirable in an estimator, basic techniques for finding maximum likelihood solutions, the general form of the covariance matrix for ML estimates, the sampling distribution of ML estimators; the use of ML in the normal as well as other distributions, and some useful illustrations of likelihoods.
Maximum Likelihood Estimation Of Nonlinear Factor Analysis Model Using Mcecm Algorithm
DOWNLOAD
Author : Mei Long
language : en
Publisher:
Release Date : 2005
Maximum Likelihood Estimation Of Nonlinear Factor Analysis Model Using Mcecm Algorithm written by Mei Long and has been published by this book supported file pdf, txt, epub, kindle and other format this book has been release on 2005 with Estimation theory categories.