Mixture Models Robustness And The Weighted Likelihood Methodology
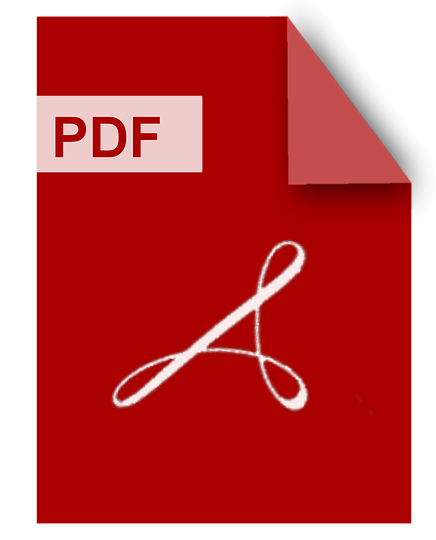
DOWNLOAD
Download Mixture Models Robustness And The Weighted Likelihood Methodology PDF/ePub or read online books in Mobi eBooks. Click Download or Read Online button to get Mixture Models Robustness And The Weighted Likelihood Methodology book now. This website allows unlimited access to, at the time of writing, more than 1.5 million titles, including hundreds of thousands of titles in various foreign languages. If the content not found or just blank you must refresh this page
Mixture Models Robustness And The Weighted Likelihood Methodology
DOWNLOAD
Author : Marianthi Markatou
language : en
Publisher:
Release Date : 1998
Mixture Models Robustness And The Weighted Likelihood Methodology written by Marianthi Markatou and has been published by this book supported file pdf, txt, epub, kindle and other format this book has been release on 1998 with categories.
Finite Mixture Models
DOWNLOAD
Author : Geoffrey McLachlan
language : en
Publisher: John Wiley & Sons
Release Date : 2004-03-22
Finite Mixture Models written by Geoffrey McLachlan and has been published by John Wiley & Sons this book supported file pdf, txt, epub, kindle and other format this book has been release on 2004-03-22 with Mathematics categories.
An up-to-date, comprehensive account of major issues in finitemixture modeling This volume provides an up-to-date account of the theory andapplications of modeling via finite mixture distributions. With anemphasis on the applications of mixture models in both mainstreamanalysis and other areas such as unsupervised pattern recognition,speech recognition, and medical imaging, the book describes theformulations of the finite mixture approach, details itsmethodology, discusses aspects of its implementation, andillustrates its application in many common statisticalcontexts. Major issues discussed in this book include identifiabilityproblems, actual fitting of finite mixtures through use of the EMalgorithm, properties of the maximum likelihood estimators soobtained, assessment of the number of components to be used in themixture, and the applicability of asymptotic theory in providing abasis for the solutions to some of these problems. The author alsoconsiders how the EM algorithm can be scaled to handle the fittingof mixture models to very large databases, as in data miningapplications. This comprehensive, practical guide: * Provides more than 800 references-40% published since 1995 * Includes an appendix listing available mixture software * Links statistical literature with machine learning and patternrecognition literature * Contains more than 100 helpful graphs, charts, and tables Finite Mixture Models is an important resource for both applied andtheoretical statisticians as well as for researchers in the manyareas in which finite mixture models can be used to analyze data.
Mixture Models And Applications
DOWNLOAD
Author : Nizar Bouguila
language : en
Publisher: Springer
Release Date : 2019-08-13
Mixture Models And Applications written by Nizar Bouguila and has been published by Springer this book supported file pdf, txt, epub, kindle and other format this book has been release on 2019-08-13 with Technology & Engineering categories.
This book focuses on recent advances, approaches, theories and applications related to mixture models. In particular, it presents recent unsupervised and semi-supervised frameworks that consider mixture models as their main tool. The chapters considers mixture models involving several interesting and challenging problems such as parameters estimation, model selection, feature selection, etc. The goal of this book is to summarize the recent advances and modern approaches related to these problems. Each contributor presents novel research, a practical study, or novel applications based on mixture models, or a survey of the literature. Reports advances on classic problems in mixture modeling such as parameter estimation, model selection, and feature selection; Present theoretical and practical developments in mixture-based modeling and their importance in different applications; Discusses perspectives and challenging future works related to mixture modeling.
Mixture Models
DOWNLOAD
Author : Bruce G. Lindsay
language : en
Publisher: IMS
Release Date : 1995
Mixture Models written by Bruce G. Lindsay and has been published by IMS this book supported file pdf, txt, epub, kindle and other format this book has been release on 1995 with Mathematics categories.
Mixture Models
DOWNLOAD
Author : Weixin Yao
language : en
Publisher: CRC Press
Release Date : 2024-04-18
Mixture Models written by Weixin Yao and has been published by CRC Press this book supported file pdf, txt, epub, kindle and other format this book has been release on 2024-04-18 with Mathematics categories.
Mixture models are a powerful tool for analyzing complex and heterogeneous datasets across many scientific fields, from finance to genomics. Mixture Models: Parametric, Semiparametric, and New Directions provides an up-to-date introduction to these models, their recent developments, and their implementation using R. It fills a gap in the literature by covering not only the basics of finite mixture models, but also recent developments such as semiparametric extensions, robust modeling, label switching, and high-dimensional modeling. Features Comprehensive overview of the methods and applications of mixture models Key topics include hypothesis testing, model selection, estimation methods, and Bayesian approaches Recent developments, such as semiparametric extensions, robust modeling, label switching, and high-dimensional modeling Examples and case studies from such fields as astronomy, biology, genomics, economics, finance, medicine, engineering, and sociology Integrated R code for many of the models, with code and data available in the R Package MixSemiRob Mixture Models: Parametric, Semiparametric, and New Directions is a valuable resource for researchers and postgraduate students from statistics, biostatistics, and other fields. It could be used as a textbook for a course on model-based clustering methods, and as a supplementary text for courses on data mining, semiparametric modeling, and high-dimensional data analysis.
Handbook Of Mixture Analysis
DOWNLOAD
Author : Sylvia Fruhwirth-Schnatter
language : en
Publisher: CRC Press
Release Date : 2019-01-04
Handbook Of Mixture Analysis written by Sylvia Fruhwirth-Schnatter and has been published by CRC Press this book supported file pdf, txt, epub, kindle and other format this book has been release on 2019-01-04 with Computers categories.
Mixture models have been around for over 150 years, and they are found in many branches of statistical modelling, as a versatile and multifaceted tool. They can be applied to a wide range of data: univariate or multivariate, continuous or categorical, cross-sectional, time series, networks, and much more. Mixture analysis is a very active research topic in statistics and machine learning, with new developments in methodology and applications taking place all the time. The Handbook of Mixture Analysis is a very timely publication, presenting a broad overview of the methods and applications of this important field of research. It covers a wide array of topics, including the EM algorithm, Bayesian mixture models, model-based clustering, high-dimensional data, hidden Markov models, and applications in finance, genomics, and astronomy. Features: Provides a comprehensive overview of the methods and applications of mixture modelling and analysis Divided into three parts: Foundations and Methods; Mixture Modelling and Extensions; and Selected Applications Contains many worked examples using real data, together with computational implementation, to illustrate the methods described Includes contributions from the leading researchers in the field The Handbook of Mixture Analysis is targeted at graduate students and young researchers new to the field. It will also be an important reference for anyone working in this field, whether they are developing new methodology, or applying the models to real scientific problems.
Probability And Statistical Models With Applications
DOWNLOAD
Author : CH. A. Charalambides
language : en
Publisher: CRC Press
Release Date : 2000-09-21
Probability And Statistical Models With Applications written by CH. A. Charalambides and has been published by CRC Press this book supported file pdf, txt, epub, kindle and other format this book has been release on 2000-09-21 with Mathematics categories.
This monograph of carefully collected articles reviews recent developments in theoretical and applied statistical science, highlights current noteworthy results and illustrates their applications; and points out possible new directions to pursue. With its enlightening account of statistical discoveries and its numerous figures and tables, Probabili
Robust Mixture Modeling
DOWNLOAD
Author : Chun Yu
language : en
Publisher:
Release Date : 2014
Robust Mixture Modeling written by Chun Yu and has been published by this book supported file pdf, txt, epub, kindle and other format this book has been release on 2014 with categories.
Ordinary least-squares (OLS) estimators for a linear model are very sensitive to unusual values in the design space or outliers among y values. Even one single atypical value may have a large effect on the parameter estimates. In this proposal, we first review and describe some available and popular robust techniques, including some recent developed ones, and compare them in terms of breakdown point and efficiency. In addition, we also use a simulation study and a real data application to compare the performance of existing robust methods under different scenarios. Finite mixture models are widely applied in a variety of random phenomena. However, inference of mixture models is a challenging work when the outliers exist in the data. The traditional maximum likelihood estimator (MLE) is sensitive to outliers. In this proposal, we propose a Robust Mixture via Mean shift penalization (RMM) in mixture models and Robust Mixture Regression via Mean shift penalization (RMRM) in mixture regression, to achieve simultaneous outlier detection and parameter estimation. A mean shift parameter is added to the mixture models, and penalized by a nonconvex penalty function. With this model setting, we develop an iterative thresholding embedded EM algorithm to maximize the penalized objective function. Comparing with other existing robust methods, the proposed methods show outstanding performance in both identifying outliers and estimating the parameters.
Mixture Model Based Classification
DOWNLOAD
Author : Paul D. McNicholas
language : en
Publisher: CRC Press
Release Date : 2016-10-04
Mixture Model Based Classification written by Paul D. McNicholas and has been published by CRC Press this book supported file pdf, txt, epub, kindle and other format this book has been release on 2016-10-04 with Mathematics categories.
"This is a great overview of the field of model-based clustering and classification by one of its leading developers. McNicholas provides a resource that I am certain will be used by researchers in statistics and related disciplines for quite some time. The discussion of mixtures with heavy tails and asymmetric distributions will place this text as the authoritative, modern reference in the mixture modeling literature." (Douglas Steinley, University of Missouri) Mixture Model-Based Classification is the first monograph devoted to mixture model-based approaches to clustering and classification. This is both a book for established researchers and newcomers to the field. A history of mixture models as a tool for classification is provided and Gaussian mixtures are considered extensively, including mixtures of factor analyzers and other approaches for high-dimensional data. Non-Gaussian mixtures are considered, from mixtures with components that parameterize skewness and/or concentration, right up to mixtures of multiple scaled distributions. Several other important topics are considered, including mixture approaches for clustering and classification of longitudinal data as well as discussion about how to define a cluster Paul D. McNicholas is the Canada Research Chair in Computational Statistics at McMaster University, where he is a Professor in the Department of Mathematics and Statistics. His research focuses on the use of mixture model-based approaches for classification, with particular attention to clustering applications, and he has published extensively within the field. He is an associate editor for several journals and has served as a guest editor for a number of special issues on mixture models.
Essays On Finite Mixture Models
DOWNLOAD
Author : Abram van Dijk
language : en
Publisher: Rozenberg Publishers
Release Date : 2009
Essays On Finite Mixture Models written by Abram van Dijk and has been published by Rozenberg Publishers this book supported file pdf, txt, epub, kindle and other format this book has been release on 2009 with categories.
Finite mixture distributions are a weighted average of a finite number of distributions. The latter are usually called the mixture components. The weights are usually described by a multinomial distribution and are sometimes called mixing proportions. The mixture components may be the same type of distributions with di®erent parameter values but they may also be completely different distributions. Therefore, finite mixture distributions are very °exible for modeling data. They are frequently used as a building block within many modern econometric models. The specification of the mixture distribution depends on the modeling problem at hand. In this thesis, we introduce new applications of finite mixtures to deal with several di®erent modeling issues. Each chapter of the thesis focusses on a specific modeling issue. The parameters of some of the resulting models can be estimated using standard techniques but for some of the chapters we need to develop new estimation and inference methods. To illustrate how the methods can be applied, we analyze at least one empirical data set for each approach. These data sets cover a wide range of research fields, such as macroeconomics, marketing, and political science. We show the usefulness of the methods and, in some cases, the improvement over previous methods in the literature.