Modeling High Dimensional Data Prediction Sparsity And
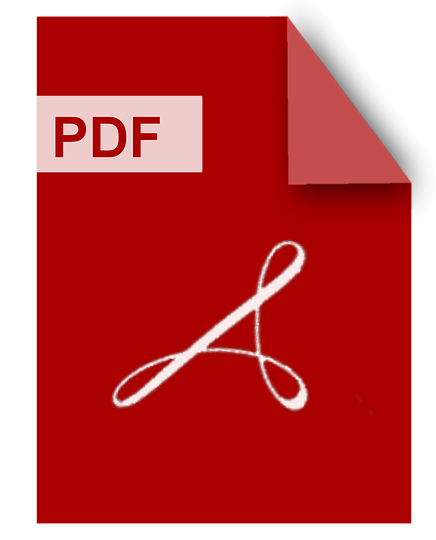
DOWNLOAD
Download Modeling High Dimensional Data Prediction Sparsity And PDF/ePub or read online books in Mobi eBooks. Click Download or Read Online button to get Modeling High Dimensional Data Prediction Sparsity And book now. This website allows unlimited access to, at the time of writing, more than 1.5 million titles, including hundreds of thousands of titles in various foreign languages. If the content not found or just blank you must refresh this page
Modeling High Dimensional Data
DOWNLOAD
Author : Chinghway Lim
language : en
Publisher:
Release Date : 2011
Modeling High Dimensional Data written by Chinghway Lim and has been published by this book supported file pdf, txt, epub, kindle and other format this book has been release on 2011 with categories.
This dissertation is on high dimensional data and their associated regularization through dimension reduction and penalization. We start with two real world problems to illustrate the practical difficulties and remedies in analyzing high dimensional data. In Chapter 1, we are tasked with modeling and predicting the U.S. stock market, where the number of stocks far exceeds the number of days relevant to the current market. Through an existing statistical arbitrage framework, we reduce the dimension of our problem with the use of correspondence analysis. We develop a data driven regression model and highlight some common statistical methods that improve our predictions. In Chapter 2, we attempt to detect and predict system anomalies in large enterprise telephony systems. We do this by processing large amounts of unstructured log files, again with dimension reduction methods, allowing effective visualization and automatic filtering of results. We then move on to more general methodology and analysis in high dimensions. In Chapter 3, we consider regularization methods, often used in dealing with high dimensional data, and tackle the problem of selecting the associated regularization parameter. We introduce SSCV, a selection criterion based on statistical stability, but also incorporating model fit, and show that it can often outperform the popular cross validation. Finally, we explore robust methods in the high dimensional setting in Chapter 4. We focus on the relative performance and distributional robustness of the estimators optimizing L1 and L2 loss functions respectively. We verify some expected results and also highlight cases where results from classical asymptotics fail, setting the stage for future theoretical work.
Sparse Boosting Based Machine Learning Methods For High Dimensional Data
DOWNLOAD
Author : Mu Yue
language : en
Publisher:
Release Date : 2020
Sparse Boosting Based Machine Learning Methods For High Dimensional Data written by Mu Yue and has been published by this book supported file pdf, txt, epub, kindle and other format this book has been release on 2020 with Electronic books categories.
In high-dimensional data, penalized regression is often used for variable selection and parameter estimation. However, these methods typically require time-consuming cross-validation methods to select tuning parameters and retain more false positives under high dimensionality. This chapter discusses sparse boosting based machine learning methods in the following high-dimensional problems. First, a sparse boosting method to select important biomarkers is studied for the right censored survival data with high-dimensional biomarkers. Then, a two-step sparse boosting method to carry out the variable selection and the model-based prediction is studied for the high-dimensional longitudinal observations measured repeatedly over time. Finally, a multi-step sparse boosting method to identify patient subgroups that exhibit different treatment effects is studied for the high-dimensional dense longitudinal observations. This chapter intends to solve the problem of how to improve the accuracy and calculation speed of variable selection and parameter estimation in high-dimensional data. It aims to expand the application scope of sparse boosting and develop new methods of high-dimensional survival analysis, longitudinal data analysis, and subgroup analysis, which has great application prospects.
Prediction And Model Selection For High Dimensional Data With Sparse Or Low Rank Structure
DOWNLOAD
Author : Rina Foygel Barber
language : en
Publisher:
Release Date : 2012
Prediction And Model Selection For High Dimensional Data With Sparse Or Low Rank Structure written by Rina Foygel Barber and has been published by this book supported file pdf, txt, epub, kindle and other format this book has been release on 2012 with categories.
For sparse regression and sparse graphical models, we consider the model selection problem, where the goal is to identify the structure of an underlying sparse model that exactly describes the distribution of the data. We analyze the extended Bayesian information criterion and its connection to the Bayesian posterior distribution over models in a high-dimensional scenario. The model selection properties of these methods are explored further with experiments on spam email filtering data and precipitation pattern data.
Sparse Modeling
DOWNLOAD
Author : Irina Rish
language : en
Publisher: CRC Press
Release Date : 2014-12-01
Sparse Modeling written by Irina Rish and has been published by CRC Press this book supported file pdf, txt, epub, kindle and other format this book has been release on 2014-12-01 with Business & Economics categories.
Sparse models are particularly useful in scientific applications, such as biomarker discovery in genetic or neuroimaging data, where the interpretability of a predictive model is essential. Sparsity can also dramatically improve the cost efficiency of signal processing. Sparse Modeling: Theory, Algorithms, and Applications provides an introduction to the growing field of sparse modeling, including application examples, problem formulations that yield sparse solutions, algorithms for finding such solutions, and recent theoretical results on sparse recovery. The book gets you up to speed on the latest sparsity-related developments and will motivate you to continue learning about the field. The authors first present motivating examples and a high-level survey of key recent developments in sparse modeling. The book then describes optimization problems involving commonly used sparsity-enforcing tools, presents essential theoretical results, and discusses several state-of-the-art algorithms for finding sparse solutions. The authors go on to address a variety of sparse recovery problems that extend the basic formulation to more sophisticated forms of structured sparsity and to different loss functions. They also examine a particular class of sparse graphical models and cover dictionary learning and sparse matrix factorizations.
Cross Validation And Regression Analysis In High Dimensional Sparse Linear Models
DOWNLOAD
Author : Feng Zhang
language : en
Publisher: Stanford University
Release Date : 2011
Cross Validation And Regression Analysis In High Dimensional Sparse Linear Models written by Feng Zhang and has been published by Stanford University this book supported file pdf, txt, epub, kindle and other format this book has been release on 2011 with categories.
Modern scientific research often involves experiments with at most hundreds of subjects but with tens of thousands of variables for every subject. The challenge of high dimensionality has reshaped statistical thinking and modeling. Variable selection plays a pivotal role in the high-dimensional data analysis, and the combination of sparsity and accuracy is crucial for statistical theory and practical applications. Regularization methods are attractive for tackling these sparsity and accuracy issues. The first part of this thesis studies two regularization methods. First, we consider the orthogonal greedy algorithm (OGA) used in conjunction with a high-dimensional information criterion introduced by Ing& Lai (2011). Although it has been shown to have excellent performance for weakly sparse regression models, one does not know a priori in practice that the actual model is weakly sparse, and we address this problem by developing a new cross-validation approach. OGA can be viewed as L0 regularization for weakly sparse regression models. When such sparsity fails, as revealed by the cross-validation analysis, we propose to use a new way to combine L1 and L2 penalties, which we show to have important advantages over previous regularization methods. The second part of the thesis develops a Monte Carlo Cross-Validation (MCCV) method to estimate the distribution of out-of-sample prediction errors when a training sample is used to build a regression model for prediction. Asymptotic theory and simulation studies show that the proposed MCCV method mimics the actual (but unknown) prediction error distribution even when the number of regressors exceeds the sample size. Therefore MCCV provides a useful tool for comparing the predictive performance of different regularization methods for real (rather than simulated) data sets.
Statistical Analysis For High Dimensional Data
DOWNLOAD
Author : Arnoldo Frigessi
language : en
Publisher: Springer
Release Date : 2016-02-16
Statistical Analysis For High Dimensional Data written by Arnoldo Frigessi and has been published by Springer this book supported file pdf, txt, epub, kindle and other format this book has been release on 2016-02-16 with Mathematics categories.
This book features research contributions from The Abel Symposium on Statistical Analysis for High Dimensional Data, held in Nyvågar, Lofoten, Norway, in May 2014. The focus of the symposium was on statistical and machine learning methodologies specifically developed for inference in “big data” situations, with particular reference to genomic applications. The contributors, who are among the most prominent researchers on the theory of statistics for high dimensional inference, present new theories and methods, as well as challenging applications and computational solutions. Specific themes include, among others, variable selection and screening, penalised regression, sparsity, thresholding, low dimensional structures, computational challenges, non-convex situations, learning graphical models, sparse covariance and precision matrices, semi- and non-parametric formulations, multiple testing, classification, factor models, clustering, and preselection. Highlighting cutting-edge research and casting light on future research directions, the contributions will benefit graduate students and researchers in computational biology, statistics and the machine learning community.
Sparse Graphical Modeling For High Dimensional Data
DOWNLOAD
Author : Faming Liang
language : en
Publisher: CRC Press
Release Date : 2023-08-02
Sparse Graphical Modeling For High Dimensional Data written by Faming Liang and has been published by CRC Press this book supported file pdf, txt, epub, kindle and other format this book has been release on 2023-08-02 with Mathematics categories.
A general framework for learning sparse graphical models with conditional independence tests Complete treatments for different types of data, Gaussian, Poisson, multinomial, and mixed data Unified treatments for data integration, network comparison, and covariate adjustment Unified treatments for missing data and heterogeneous data Efficient methods for joint estimation of multiple graphical models Effective methods of high-dimensional variable selection Effective methods of high-dimensional inference
Sparse Models For Sparse Data
DOWNLOAD
Author : David Gregory Purdy
language : en
Publisher:
Release Date : 2012
Sparse Models For Sparse Data written by David Gregory Purdy and has been published by this book supported file pdf, txt, epub, kindle and other format this book has been release on 2012 with categories.
Significant recent advances in many areas of data collection and processing have introduced many challenges for modeling such data. Data sets have exploded in the number of observations and dimensionality. The explosion in dimensionality has led to advances in the modeling of high dimensional data with regularized and sparse models. One of the more interesting and challenging varieties of high dimensional data are sparse data sets. Sparse data sets arise from many important areas involving human-computer interaction, such as text and language processing, and human-human interaction, such as social networks. Our motivation in this thesis is to explore the use of sparse models for applications involving sparse data. In some cases, we have made improvements over previous methods that fundamentally involved dense models fitted on, and applied to, sparse data. In other cases, we have adapted sparse models developed for dense data sets. Along the way, we have encountered a recurring issue: due to both subsampling and regularization, we are faced with a problem that sparse models may not adequately capture the full dimensionality of such data and may be inadequate for prediction on test data. The utility of sparse models have been demonstrated in contexts with very high dimensional dense data. In this dissertation, we shall examine two applications and modeling methods involving sparse linear models and sparse matrix decompositions. Our first application involves natural language processing and ranking, the second involves recommendation systems and matrix factorization. In Chapter 2, we developed a novel and powerful visualization system. We named our system Bonsai as it enables a curated process of developing trees that partition the joint space of data and models. By exploring the product space of the space of training data, the space of modeling parameters, and the space of test data, we can explore how our models are developed based on the constraints imposed and the data they attempt to model or predict. More generally, we believe we have introduced a very fruitful means of exploring a multiplicity of models and a multiplicity of data samples. Chapter 3 is based on our work in the Netflix Prize competition. In contrast to others' use of dense models for this sparse data, we sought to introduce modeling methods with tunable sparsity. In this work, we found striking difficulties in modeling the data with sparse models, and identified concerns about the utility of sparse models for sparse data. In conclusion, this thesis presents several methods, and limitations of such methods, for modeling sparse data with sparse models. These limitations are suggestive of new directions to pursue. In particular, we are optimistic that future research in modeling methods may find new ways to tune models for density, when applied to sparse data, just as much research on models for dense data has involved tuning models for sparsity.
Practical Applications Of Sparse Modeling
DOWNLOAD
Author : Irina Rish
language : en
Publisher: MIT Press
Release Date : 2014-09-12
Practical Applications Of Sparse Modeling written by Irina Rish and has been published by MIT Press this book supported file pdf, txt, epub, kindle and other format this book has been release on 2014-09-12 with Computers categories.
"Sparse modeling is a rapidly developing area at the intersection of statistical learning and signal processing, motivated by the age-old statistical problem of selecting a small number of predictive variables in high-dimensional data sets. This collection describes key approaches in sparse modeling, focusing on its applications in such fields as neuroscience, computational biology, and computer vision. Sparse modeling methods can improve the interpretability of predictive models and aid efficient recovery of high-dimensional unobserved signals from a limited number of measurements. Yet despite significant advances in the field, a number of open issues remain when sparse modeling meets real-life applications. The book discusses a range of practical applications and state-of-the-art approaches for tackling the challenges presented by these applications. Topics considered include the choice of method in genomics applications; analysis of protein mass-spectrometry data; the stability of sparse models in brain imaging applications; sequential testing approaches; algorithmic aspects of sparse recovery; and learning sparse latent models"--Jacket.
High Dimensional Covariance Estimation
DOWNLOAD
Author : Mohsen Pourahmadi
language : en
Publisher: John Wiley & Sons
Release Date : 2013-05-28
High Dimensional Covariance Estimation written by Mohsen Pourahmadi and has been published by John Wiley & Sons this book supported file pdf, txt, epub, kindle and other format this book has been release on 2013-05-28 with Mathematics categories.
Methods for estimating sparse and large covariance matrices Covariance and correlation matrices play fundamental roles in every aspect of the analysis of multivariate data collected from a variety of fields including business and economics, health care, engineering, and environmental and physical sciences. High-Dimensional Covariance Estimation provides accessible and comprehensive coverage of the classical and modern approaches for estimating covariance matrices as well as their applications to the rapidly developing areas lying at the intersection of statistics and machine learning. Recently, the classical sample covariance methodologies have been modified and improved upon to meet the needs of statisticians and researchers dealing with large correlated datasets. High-Dimensional Covariance Estimation focuses on the methodologies based on shrinkage, thresholding, and penalized likelihood with applications to Gaussian graphical models, prediction, and mean-variance portfolio management. The book relies heavily on regression-based ideas and interpretations to connect and unify many existing methods and algorithms for the task. High-Dimensional Covariance Estimation features chapters on: Data, Sparsity, and Regularization Regularizing the Eigenstructure Banding, Tapering, and Thresholding Covariance Matrices Sparse Gaussian Graphical Models Multivariate Regression The book is an ideal resource for researchers in statistics, mathematics, business and economics, computer sciences, and engineering, as well as a useful text or supplement for graduate-level courses in multivariate analysis, covariance estimation, statistical learning, and high-dimensional data analysis.