Sparse Models For Sparse Data
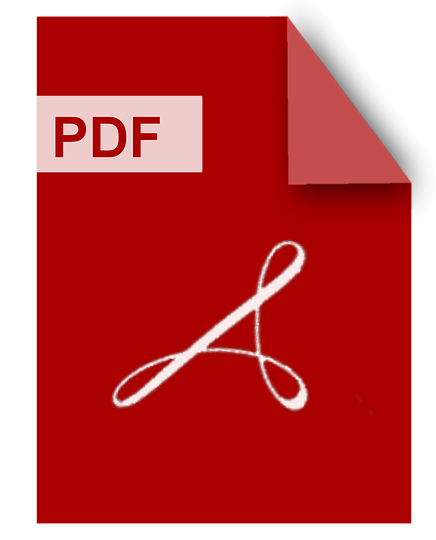
DOWNLOAD
Download Sparse Models For Sparse Data PDF/ePub or read online books in Mobi eBooks. Click Download or Read Online button to get Sparse Models For Sparse Data book now. This website allows unlimited access to, at the time of writing, more than 1.5 million titles, including hundreds of thousands of titles in various foreign languages. If the content not found or just blank you must refresh this page
Sparse Models For Sparse Data
DOWNLOAD
Author : David Gregory Purdy
language : en
Publisher:
Release Date : 2012
Sparse Models For Sparse Data written by David Gregory Purdy and has been published by this book supported file pdf, txt, epub, kindle and other format this book has been release on 2012 with categories.
Significant recent advances in many areas of data collection and processing have introduced many challenges for modeling such data. Data sets have exploded in the number of observations and dimensionality. The explosion in dimensionality has led to advances in the modeling of high dimensional data with regularized and sparse models. One of the more interesting and challenging varieties of high dimensional data are sparse data sets. Sparse data sets arise from many important areas involving human-computer interaction, such as text and language processing, and human-human interaction, such as social networks. Our motivation in this thesis is to explore the use of sparse models for applications involving sparse data. In some cases, we have made improvements over previous methods that fundamentally involved dense models fitted on, and applied to, sparse data. In other cases, we have adapted sparse models developed for dense data sets. Along the way, we have encountered a recurring issue: due to both subsampling and regularization, we are faced with a problem that sparse models may not adequately capture the full dimensionality of such data and may be inadequate for prediction on test data. The utility of sparse models have been demonstrated in contexts with very high dimensional dense data. In this dissertation, we shall examine two applications and modeling methods involving sparse linear models and sparse matrix decompositions. Our first application involves natural language processing and ranking, the second involves recommendation systems and matrix factorization. In Chapter 2, we developed a novel and powerful visualization system. We named our system Bonsai as it enables a curated process of developing trees that partition the joint space of data and models. By exploring the product space of the space of training data, the space of modeling parameters, and the space of test data, we can explore how our models are developed based on the constraints imposed and the data they attempt to model or predict. More generally, we believe we have introduced a very fruitful means of exploring a multiplicity of models and a multiplicity of data samples. Chapter 3 is based on our work in the Netflix Prize competition. In contrast to others' use of dense models for this sparse data, we sought to introduce modeling methods with tunable sparsity. In this work, we found striking difficulties in modeling the data with sparse models, and identified concerns about the utility of sparse models for sparse data. In conclusion, this thesis presents several methods, and limitations of such methods, for modeling sparse data with sparse models. These limitations are suggestive of new directions to pursue. In particular, we are optimistic that future research in modeling methods may find new ways to tune models for density, when applied to sparse data, just as much research on models for dense data has involved tuning models for sparsity.
Sparse Modeling
DOWNLOAD
Author : Irina Rish
language : en
Publisher: CRC Press
Release Date : 2014-12-01
Sparse Modeling written by Irina Rish and has been published by CRC Press this book supported file pdf, txt, epub, kindle and other format this book has been release on 2014-12-01 with Business & Economics categories.
Sparse models are particularly useful in scientific applications, such as biomarker discovery in genetic or neuroimaging data, where the interpretability of a predictive model is essential. Sparsity can also dramatically improve the cost efficiency of signal processing. Sparse Modeling: Theory, Algorithms, and Applications provides an introduction to the growing field of sparse modeling, including application examples, problem formulations that yield sparse solutions, algorithms for finding such solutions, and recent theoretical results on sparse recovery. The book gets you up to speed on the latest sparsity-related developments and will motivate you to continue learning about the field. The authors first present motivating examples and a high-level survey of key recent developments in sparse modeling. The book then describes optimization problems involving commonly used sparsity-enforcing tools, presents essential theoretical results, and discusses several state-of-the-art algorithms for finding sparse solutions. The authors go on to address a variety of sparse recovery problems that extend the basic formulation to more sophisticated forms of structured sparsity and to different loss functions. They also examine a particular class of sparse graphical models and cover dictionary learning and sparse matrix factorizations.
Sparse Modeling
DOWNLOAD
Author : Irina Rish
language : en
Publisher:
Release Date : 2014
Sparse Modeling written by Irina Rish and has been published by this book supported file pdf, txt, epub, kindle and other format this book has been release on 2014 with categories.
Sparse models are particularly useful in scientific applications, such as biomarker discovery in genetic or neuroimaging data, where the interpretability of a predictive model is essential. Sparsity can also dramatically improve the cost efficiency of signal processing. Sparse Modeling: Theory, Algorithms, and Applications provides an introduction to the growing field of sparse modeling, including application examples, problem formulations that yield sparse solutions, algorithms for finding such solutions, and recent theoretical results on sparse recovery. The book gets you up to speed on the latest sparsity-related developments and will motivate you to continue learning about the field. The authors first present motivating examples and a high-level survey of key recent developments in sparse modeling. The book then describes optimization problems involving commonly used sparsity-enforcing tools, presents essential theoretical results, and discusses several state-of-the-art algorithms for finding sparse solutions. The authors go on to address a variety of sparse recovery problems that extend the basic formulation to more sophisticated forms of structured sparsity and to different loss functions. They also examine a particular class of sparse graphical models and cover dictionary learning and sparse matrix factorizations.
Practical Applications Of Sparse Modeling
DOWNLOAD
Author : Irina Rish
language : en
Publisher: MIT Press
Release Date : 2014-09-12
Practical Applications Of Sparse Modeling written by Irina Rish and has been published by MIT Press this book supported file pdf, txt, epub, kindle and other format this book has been release on 2014-09-12 with Computers categories.
"Sparse modeling is a rapidly developing area at the intersection of statistical learning and signal processing, motivated by the age-old statistical problem of selecting a small number of predictive variables in high-dimensional data sets. This collection describes key approaches in sparse modeling, focusing on its applications in such fields as neuroscience, computational biology, and computer vision. Sparse modeling methods can improve the interpretability of predictive models and aid efficient recovery of high-dimensional unobserved signals from a limited number of measurements. Yet despite significant advances in the field, a number of open issues remain when sparse modeling meets real-life applications. The book discusses a range of practical applications and state-of-the-art approaches for tackling the challenges presented by these applications. Topics considered include the choice of method in genomics applications; analysis of protein mass-spectrometry data; the stability of sparse models in brain imaging applications; sequential testing approaches; algorithmic aspects of sparse recovery; and learning sparse latent models"--Jacket.
Sparse Modeling For Image And Vision Processing
DOWNLOAD
Author : Julien Mairal
language : en
Publisher:
Release Date : 2014
Sparse Modeling For Image And Vision Processing written by Julien Mairal and has been published by this book supported file pdf, txt, epub, kindle and other format this book has been release on 2014 with Computer graphics categories.
In recent years, a large amount of multi-disciplinary research has been conducted on sparse models and their applications. In statistics and machine learning, the sparsity principle is used to perform model selection -- that is, automatically selecting a simple model among a large collection of them. In signal processing, sparse coding consists of representing data with linear combinations of a few dictionary elements. Subsequently, the corresponding tools have been widely adopted by several scientific communities such as neuroscience, bioinformatics, or computer vision. The goal of this monograph is to offer a self-contained view of sparse modeling for visual recognition and image processing. More specifically, we focus on applications where the dictionary is learned and adapted to data, yielding a compact representation that has been successful in various contexts.
Deep Learning Through Sparse And Low Rank Modeling
DOWNLOAD
Author : Zhangyang Wang
language : en
Publisher: Academic Press
Release Date : 2019-04-26
Deep Learning Through Sparse And Low Rank Modeling written by Zhangyang Wang and has been published by Academic Press this book supported file pdf, txt, epub, kindle and other format this book has been release on 2019-04-26 with Computers categories.
Deep Learning through Sparse Representation and Low-Rank Modeling bridges classical sparse and low rank models-those that emphasize problem-specific Interpretability-with recent deep network models that have enabled a larger learning capacity and better utilization of Big Data. It shows how the toolkit of deep learning is closely tied with the sparse/low rank methods and algorithms, providing a rich variety of theoretical and analytic tools to guide the design and interpretation of deep learning models. The development of the theory and models is supported by a wide variety of applications in computer vision, machine learning, signal processing, and data mining. This book will be highly useful for researchers, graduate students and practitioners working in the fields of computer vision, machine learning, signal processing, optimization and statistics. Combines classical sparse and low-rank models and algorithms with the latest advances in deep learning networks Shows how the structure and algorithms of sparse and low-rank methods improves the performance and interpretability of Deep Learning models Provides tactics on how to build and apply customized deep learning models for various applications
Combining Related And Sparse Data In Linear Regression Models
DOWNLOAD
Author : Wilfried R. Vanhonacker
language : en
Publisher:
Release Date : 1986
Combining Related And Sparse Data In Linear Regression Models written by Wilfried R. Vanhonacker and has been published by this book supported file pdf, txt, epub, kindle and other format this book has been release on 1986 with categories.
Learning Sparse Data Models Via Geometric Optimization With Applications To Image Processing
DOWNLOAD
Author : Simon Alois Hawe
language : en
Publisher:
Release Date : 2013
Learning Sparse Data Models Via Geometric Optimization With Applications To Image Processing written by Simon Alois Hawe and has been published by this book supported file pdf, txt, epub, kindle and other format this book has been release on 2013 with categories.
Sparse Modeling With Universal Priors And Learned Incoherent Dictionaries Preprint
DOWNLOAD
Author :
language : en
Publisher:
Release Date : 2009
Sparse Modeling With Universal Priors And Learned Incoherent Dictionaries Preprint written by and has been published by this book supported file pdf, txt, epub, kindle and other format this book has been release on 2009 with categories.
Sparse data models have gained considerable attention in recent years, and their use has led to state-of-the-art results in many signal and image processing tasks. The learning of sparse models has been mostly concerned with adapting the dictionary to tasks such as classification and reconstruction, optimizing extrinsic properties of the trained dictionaries. In this work, we first propose a learning method aimed at enhancing both extrinsic and intrinsic properties of the dictionaries, such as the mutual and cumulative coherence and the Gram matrix norm, characteristics known to improve the efficiency and performance of sparse coding algorithms. We then use tools from information theory to propose a sparsity regularization term which has several desirable theoretical and practical advantages over the more standard '0 or '1 ones. These new sparse modeling components lead to improved coding performance and accuracy in reconstruction tasks.
Statistics For High Dimensional Data
DOWNLOAD
Author : Peter Bühlmann
language : en
Publisher: Springer Science & Business Media
Release Date : 2011-06-08
Statistics For High Dimensional Data written by Peter Bühlmann and has been published by Springer Science & Business Media this book supported file pdf, txt, epub, kindle and other format this book has been release on 2011-06-08 with Mathematics categories.
Modern statistics deals with large and complex data sets, and consequently with models containing a large number of parameters. This book presents a detailed account of recently developed approaches, including the Lasso and versions of it for various models, boosting methods, undirected graphical modeling, and procedures controlling false positive selections. A special characteristic of the book is that it contains comprehensive mathematical theory on high-dimensional statistics combined with methodology, algorithms and illustrations with real data examples. This in-depth approach highlights the methods’ great potential and practical applicability in a variety of settings. As such, it is a valuable resource for researchers, graduate students and experts in statistics, applied mathematics and computer science.