Combining Related And Sparse Data In Linear Regression Models
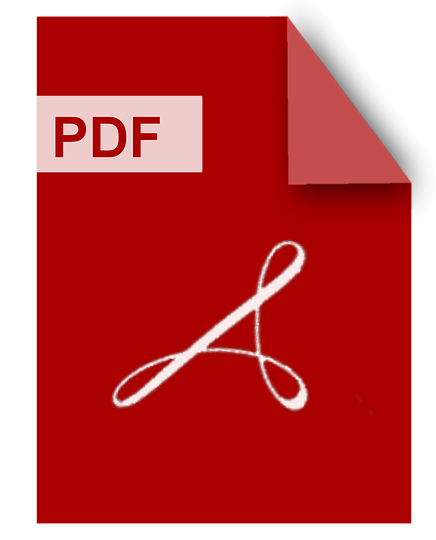
DOWNLOAD
Download Combining Related And Sparse Data In Linear Regression Models PDF/ePub or read online books in Mobi eBooks. Click Download or Read Online button to get Combining Related And Sparse Data In Linear Regression Models book now. This website allows unlimited access to, at the time of writing, more than 1.5 million titles, including hundreds of thousands of titles in various foreign languages. If the content not found or just blank you must refresh this page
Combining Related And Sparse Data In Linear Regression Models
DOWNLOAD
Author : Wilfried R. Vanhonacker
language : en
Publisher:
Release Date : 1986
Combining Related And Sparse Data In Linear Regression Models written by Wilfried R. Vanhonacker and has been published by this book supported file pdf, txt, epub, kindle and other format this book has been release on 1986 with categories.
Cross Validation And Regression Analysis In High Dimensional Sparse Linear Models
DOWNLOAD
Author : Feng Zhang
language : en
Publisher: Stanford University
Release Date : 2011
Cross Validation And Regression Analysis In High Dimensional Sparse Linear Models written by Feng Zhang and has been published by Stanford University this book supported file pdf, txt, epub, kindle and other format this book has been release on 2011 with categories.
Modern scientific research often involves experiments with at most hundreds of subjects but with tens of thousands of variables for every subject. The challenge of high dimensionality has reshaped statistical thinking and modeling. Variable selection plays a pivotal role in the high-dimensional data analysis, and the combination of sparsity and accuracy is crucial for statistical theory and practical applications. Regularization methods are attractive for tackling these sparsity and accuracy issues. The first part of this thesis studies two regularization methods. First, we consider the orthogonal greedy algorithm (OGA) used in conjunction with a high-dimensional information criterion introduced by Ing& Lai (2011). Although it has been shown to have excellent performance for weakly sparse regression models, one does not know a priori in practice that the actual model is weakly sparse, and we address this problem by developing a new cross-validation approach. OGA can be viewed as L0 regularization for weakly sparse regression models. When such sparsity fails, as revealed by the cross-validation analysis, we propose to use a new way to combine L1 and L2 penalties, which we show to have important advantages over previous regularization methods. The second part of the thesis develops a Monte Carlo Cross-Validation (MCCV) method to estimate the distribution of out-of-sample prediction errors when a training sample is used to build a regression model for prediction. Asymptotic theory and simulation studies show that the proposed MCCV method mimics the actual (but unknown) prediction error distribution even when the number of regressors exceeds the sample size. Therefore MCCV provides a useful tool for comparing the predictive performance of different regularization methods for real (rather than simulated) data sets.
Cross Validation And Regression Analysis In High Dimensional Sparse Linear Models
DOWNLOAD
Author : Feng Zhang
language : en
Publisher:
Release Date : 2011
Cross Validation And Regression Analysis In High Dimensional Sparse Linear Models written by Feng Zhang and has been published by this book supported file pdf, txt, epub, kindle and other format this book has been release on 2011 with categories.
Modern scientific research often involves experiments with at most hundreds of subjects but with tens of thousands of variables for every subject. The challenge of high dimensionality has reshaped statistical thinking and modeling. Variable selection plays a pivotal role in the high-dimensional data analysis, and the combination of sparsity and accuracy is crucial for statistical theory and practical applications. Regularization methods are attractive for tackling these sparsity and accuracy issues. The first part of this thesis studies two regularization methods. First, we consider the orthogonal greedy algorithm (OGA) used in conjunction with a high-dimensional information criterion introduced by Ing & Lai (2011). Although it has been shown to have excellent performance for weakly sparse regression models, one does not know a priori in practice that the actual model is weakly sparse, and we address this problem by developing a new cross-validation approach. OGA can be viewed as L0 regularization for weakly sparse regression models. When such sparsity fails, as revealed by the cross-validation analysis, we propose to use a new way to combine L1 and L2 penalties, which we show to have important advantages over previous regularization methods. The second part of the thesis develops a Monte Carlo Cross-Validation (MCCV) method to estimate the distribution of out-of-sample prediction errors when a training sample is used to build a regression model for prediction. Asymptotic theory and simulation studies show that the proposed MCCV method mimics the actual (but unknown) prediction error distribution even when the number of regressors exceeds the sample size. Therefore MCCV provides a useful tool for comparing the predictive performance of different regularization methods for real (rather than simulated) data sets.
Combining Soft Computing And Statistical Methods In Data Analysis
DOWNLOAD
Author : Christian Borgelt
language : en
Publisher: Springer Science & Business Media
Release Date : 2010-10-12
Combining Soft Computing And Statistical Methods In Data Analysis written by Christian Borgelt and has been published by Springer Science & Business Media this book supported file pdf, txt, epub, kindle and other format this book has been release on 2010-10-12 with Technology & Engineering categories.
Over the last forty years there has been a growing interest to extend probability theory and statistics and to allow for more flexible modelling of imprecision, uncertainty, vagueness and ignorance. The fact that in many real-life situations data uncertainty is not only present in the form of randomness (stochastic uncertainty) but also in the form of imprecision/fuzziness is but one point underlining the need for a widening of statistical tools. Most such extensions originate in a "softening" of classical methods, allowing, in particular, to work with imprecise or vague data, considering imprecise or generalized probabilities and fuzzy events, etc. About ten years ago the idea of establishing a recurrent forum for discussing new trends in the before-mentioned context was born and resulted in the first International Conference on Soft Methods in Probability and Statistics (SMPS) that was held in Warsaw in 2002. In the following years the conference took place in Oviedo (2004), in Bristol (2006) and in Toulouse (2008). In the current edition the conference returns to Oviedo. This edited volume is a collection of papers presented at the SMPS 2010 conference held in Mieres and Oviedo. It gives a comprehensive overview of current research into the fusion of soft methods with probability and statistics.
Post Shrinkage Strategies In Statistical And Machine Learning For High Dimensional Data
DOWNLOAD
Author : Syed Ejaz Ahmed
language : en
Publisher: CRC Press
Release Date : 2023-05-25
Post Shrinkage Strategies In Statistical And Machine Learning For High Dimensional Data written by Syed Ejaz Ahmed and has been published by CRC Press this book supported file pdf, txt, epub, kindle and other format this book has been release on 2023-05-25 with Business & Economics categories.
This book presents some post-estimation and predictions strategies for the host of useful statistical models with applications in data science. It combines statistical learning and machine learning techniques in a unique and optimal way. It is well-known that machine learning methods are subject to many issues relating to bias, and consequently the mean squared error and prediction error may explode. For this reason, we suggest shrinkage strategies to control the bias by combining a submodel selected by a penalized method with a model with many features. Further, the suggested shrinkage methodology can be successfully implemented for high dimensional data analysis. Many researchers in statistics and medical sciences work with big data. They need to analyse this data through statistical modelling. Estimating the model parameters accurately is an important part of the data analysis. This book may be a repository for developing improve estimation strategies for statisticians. This book will help researchers and practitioners for their teaching and advanced research, and is an excellent textbook for advanced undergraduate and graduate courses involving shrinkage, statistical, and machine learning. The book succinctly reveals the bias inherited in machine learning method and successfully provides tools, tricks and tips to deal with the bias issue. Expertly sheds light on the fundamental reasoning for model selection and post estimation using shrinkage and related strategies. This presentation is fundamental, because shrinkage and other methods appropriate for model selection and estimation problems and there is a growing interest in this area to fill the gap between competitive strategies. Application of these strategies to real life data set from many walks of life. Analytical results are fully corroborated by numerical work and numerous worked examples are included in each chapter with numerous graphs for data visualization. The presentation and style of the book clearly makes it accessible to a broad audience. It offers rich, concise expositions of each strategy and clearly describes how to use each estimation strategy for the problem at hand. This book emphasizes that statistics/statisticians can play a dominant role in solving Big Data problems, and will put them on the precipice of scientific discovery. The book contributes novel methodologies for HDDA and will open a door for continued research in this hot area. The practical impact of the proposed work stems from wide applications. The developed computational packages will aid in analyzing a broad range of applications in many walks of life.
Application Of Model Driven Meta Analysis And Latent Variable Framework In Synthesizing Studies Using Diverse Measures
DOWNLOAD
Author : Soyeon Ahn
language : en
Publisher:
Release Date : 2008
Application Of Model Driven Meta Analysis And Latent Variable Framework In Synthesizing Studies Using Diverse Measures written by Soyeon Ahn and has been published by this book supported file pdf, txt, epub, kindle and other format this book has been release on 2008 with Latent variables categories.
Statistical Learning With Sparsity
DOWNLOAD
Author : Trevor Hastie
language : en
Publisher: CRC Press
Release Date : 2015-05-07
Statistical Learning With Sparsity written by Trevor Hastie and has been published by CRC Press this book supported file pdf, txt, epub, kindle and other format this book has been release on 2015-05-07 with Business & Economics categories.
Discover New Methods for Dealing with High-Dimensional DataA sparse statistical model has only a small number of nonzero parameters or weights; therefore, it is much easier to estimate and interpret than a dense model. Statistical Learning with Sparsity: The Lasso and Generalizations presents methods that exploit sparsity to help recover the underl
Statistical Theory And Method Abstracts
DOWNLOAD
Author :
language : en
Publisher:
Release Date : 2001
Statistical Theory And Method Abstracts written by and has been published by this book supported file pdf, txt, epub, kindle and other format this book has been release on 2001 with Statistics categories.
Interpretable Machine Learning
DOWNLOAD
Author : Christoph Molnar
language : en
Publisher: Lulu.com
Release Date : 2020
Interpretable Machine Learning written by Christoph Molnar and has been published by Lulu.com this book supported file pdf, txt, epub, kindle and other format this book has been release on 2020 with Artificial intelligence categories.
This book is about making machine learning models and their decisions interpretable. After exploring the concepts of interpretability, you will learn about simple, interpretable models such as decision trees, decision rules and linear regression. Later chapters focus on general model-agnostic methods for interpreting black box models like feature importance and accumulated local effects and explaining individual predictions with Shapley values and LIME. All interpretation methods are explained in depth and discussed critically. How do they work under the hood? What are their strengths and weaknesses? How can their outputs be interpreted? This book will enable you to select and correctly apply the interpretation method that is most suitable for your machine learning project.
Tutorials In Biostatistics Statistical Methods In Clinical Studies
DOWNLOAD
Author : Ralph B. D'Agostino
language : en
Publisher: John Wiley & Sons
Release Date : 2005-09-27
Tutorials In Biostatistics Statistical Methods In Clinical Studies written by Ralph B. D'Agostino and has been published by John Wiley & Sons this book supported file pdf, txt, epub, kindle and other format this book has been release on 2005-09-27 with Mathematics categories.
The Tutorials in Biostatistics have become a very popular feature of the prestigious Wiley journal, Statistics in Medicine (SIM). The introductory style and practical focus make them accessible to a wide audience including medical practitioners with limited statistical knowledge. This book represents the first of two volumes presenting the best tutorials published in SIM, focusing on statistical methods in clinical studies. Topics include the design and analysis of clinical trials, epidemiology, survival analysis, and data monitoring. Each tutorial is focused on a medical problem, has been fully peer-reviewed and edited, and is authored by leading researchers in biostatistics. Many articles include an appendix on the latest developments since publication in the journal and additional references. This will appeal to statisticians working in medical research, as well as statistically-minded clinicians, biologists, epidemiologists and geneticists. It will also appeal to graduate students of biostatistics.