Modeling Protein Structure Alignment Based On Markov Random Field Theory
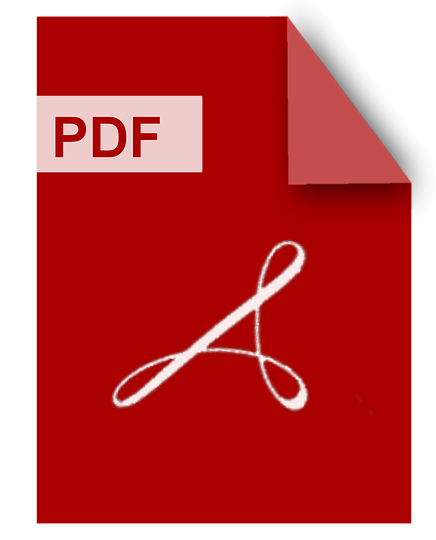
DOWNLOAD
Download Modeling Protein Structure Alignment Based On Markov Random Field Theory PDF/ePub or read online books in Mobi eBooks. Click Download or Read Online button to get Modeling Protein Structure Alignment Based On Markov Random Field Theory book now. This website allows unlimited access to, at the time of writing, more than 1.5 million titles, including hundreds of thousands of titles in various foreign languages. If the content not found or just blank you must refresh this page
Modeling Protein Structure Alignment Based On Markov Random Field Theory
DOWNLOAD
Author : Xin Sheng
language : en
Publisher:
Release Date : 1999
Modeling Protein Structure Alignment Based On Markov Random Field Theory written by Xin Sheng and has been published by this book supported file pdf, txt, epub, kindle and other format this book has been release on 1999 with categories.
Protein Homology Detection Through Alignment Of Markov Random Fields
DOWNLOAD
Author : Jinbo Xu
language : en
Publisher: Springer
Release Date : 2015-01-22
Protein Homology Detection Through Alignment Of Markov Random Fields written by Jinbo Xu and has been published by Springer this book supported file pdf, txt, epub, kindle and other format this book has been release on 2015-01-22 with Computers categories.
This work covers sequence-based protein homology detection, a fundamental and challenging bioinformatics problem with a variety of real-world applications. The text first surveys a few popular homology detection methods, such as Position-Specific Scoring Matrix (PSSM) and Hidden Markov Model (HMM) based methods, and then describes a novel Markov Random Fields (MRF) based method developed by the authors. MRF-based methods are much more sensitive than HMM- and PSSM-based methods for remote homolog detection and fold recognition, as MRFs can model long-range residue-residue interaction. The text also describes the installation, usage and result interpretation of programs implementing the MRF-based method.
Protein Structure Prediction
DOWNLOAD
Author : Mohammed Zaki
language : en
Publisher: Springer Science & Business Media
Release Date : 2007-09-12
Protein Structure Prediction written by Mohammed Zaki and has been published by Springer Science & Business Media this book supported file pdf, txt, epub, kindle and other format this book has been release on 2007-09-12 with Science categories.
This book covers elements of both the data-driven comparative modeling approach to structure prediction and also recent attempts to simulate folding using explicit or simplified models. Despite the unsolved mystery of how a protein folds, advances are being made in predicting the interactions of proteins with other molecules. Also rapidly advancing are the methods for solving the inverse folding problem, the problem of finding a sequence to fit a structure. This book focuses on the various computational methods for prediction, their successes and their limitations, from the perspective of their most well known practitioners.
Computational Methods For Protein Structure Prediction And Modeling
DOWNLOAD
Author : Ying Xu
language : en
Publisher: Springer Science & Business Media
Release Date : 2007-08-24
Computational Methods For Protein Structure Prediction And Modeling written by Ying Xu and has been published by Springer Science & Business Media this book supported file pdf, txt, epub, kindle and other format this book has been release on 2007-08-24 with Science categories.
Volume One of this two-volume sequence focuses on the basic characterization of known protein structures, and structure prediction from protein sequence information. Eleven chapters survey of the field, covering key topics in modeling, force fields, classification, computational methods, and structure prediction. Each chapter is a self contained review covering definition of the problem and historical perspective; mathematical formulation; computational methods and algorithms; performance results; existing software; strengths, pitfalls, challenges, and future research.
Graphical Models
DOWNLOAD
Author : Source Wikipedia
language : en
Publisher: University-Press.org
Release Date : 2013-09
Graphical Models written by Source Wikipedia and has been published by University-Press.org this book supported file pdf, txt, epub, kindle and other format this book has been release on 2013-09 with categories.
Please note that the content of this book primarily consists of articles available from Wikipedia or other free sources online. Pages: 102. Chapters: Bayesian networks, Markov models, Markov chain, Queueing theory, Snakes and ladders, Hidden Markov model, Poisson process, Reinforcement learning, Burst error, Mark V Shaney, Kalman filter, PageRank, Multiple sequence alignment, Models of DNA evolution, Forward-backward algorithm, Path dependence, Belief propagation, Structural equation modeling, Viterbi algorithm, Algorithmic composition, Part-of-speech tagging, Gene prediction, Google matrix, Markov switching multifractal, Conditional random field, Influence diagram, Markov random field, Markov chain Monte Carlo, Bayesian inference in phylogeny, Graphical models for protein structure, Queueing model, Pop music automation, Dynamic Markov compression, Subshift of finite type, Stochastic matrix, Language model, Examples of Markov chains, Hierarchical Bayes model, Factor graph, Markov property, Path analysis, Detailed balance, Bernoulli scheme, Variational message passing, Latent variable, Layered hidden Markov model, Markov partition, Hierarchical hidden Markov model, Discrete phase-type distribution, GLIMMER, Kolmogorov backward equations, Baum-Welch algorithm, Dependability state model, Plate notation, Junction tree algorithm, Variable-order Bayesian network, Iterative Viterbi decoding, Markovian discrimination, Forward algorithm, Entropy rate, Hidden semi-Markov model, Maximum entropy Markov model, Population process, Markov blanket, Collider, Soft output Viterbi algorithm, Moral graph, M-separation, Dynamics of Markovian particles, Markov chain geostatistics, Quantum Markov chain, Transiogram, Ancestral graph, Causal Markov condition, Poisson hidden Markov model, Dynamic Bayesian network.
Protein Modeling Using Hidden Markov Models
DOWNLOAD
Author :
language : en
Publisher:
Release Date : 1992
Protein Modeling Using Hidden Markov Models written by and has been published by this book supported file pdf, txt, epub, kindle and other format this book has been release on 1992 with Markov processes categories.
Abstract: "We apply Hidden Markov Models (HMMs) to the problem of statistical modeling and multiple sequence alignment of protein families. A variant of the Expectation Maximization (EM) algorithm known as the Viterbi algorithm is used to obtain the statistical model from the unaligned sequences. In a detailed series of experiments, we have taken 400 unaligned globin sequences, and produced a statistical model entirely automatically from the primary (unaligned) sequences. We use no prior knowledge of globin structure. Using this model, we obtained a multiple alignment of the 400 sequences and 225 other globin sequences that agrees almost perfectly with a structural alignment by Bashford et al. This model can also discriminate all these 625 globins from nonglobin protein sequences with greater than 99% accuracy, and can thus be used for database searches."
Introduction To Protein Structure Prediction
DOWNLOAD
Author : Huzefa Rangwala
language : en
Publisher: John Wiley & Sons
Release Date : 2011-03-16
Introduction To Protein Structure Prediction written by Huzefa Rangwala and has been published by John Wiley & Sons this book supported file pdf, txt, epub, kindle and other format this book has been release on 2011-03-16 with Science categories.
A look at the methods and algorithms used to predict protein structure A thorough knowledge of the function and structure of proteins is critical for the advancement of biology and the life sciences as well as the development of better drugs, higher-yield crops, and even synthetic bio-fuels. To that end, this reference sheds light on the methods used for protein structure prediction and reveals the key applications of modeled structures. This indispensable book covers the applications of modeled protein structures and unravels the relationship between pure sequence information and three-dimensional structure, which continues to be one of the greatest challenges in molecular biology. With this resource, readers will find an all-encompassing examination of the problems, methods, tools, servers, databases, and applications of protein structure prediction and they will acquire unique insight into the future applications of the modeled protein structures. The book begins with a thorough introduction to the protein structure prediction problem and is divided into four themes: a background on structure prediction, the prediction of structural elements, tertiary structure prediction, and functional insights. Within those four sections, the following topics are covered: Databases and resources that are commonly used for protein structure prediction The structure prediction flagship assessment (CASP) and the protein structure initiative (PSI) Definitions of recurring substructures and the computational approaches used for solving sequence problems Difficulties with contact map prediction and how sophisticated machine learning methods can solve those problems Structure prediction methods that rely on homology modeling, threading, and fragment assembly Hybrid methods that achieve high-resolution protein structures Parts of the protein structure that may be conserved and used to interact with other biomolecules How the loop prediction problem can be used for refinement of the modeled structures The computational model that detects the differences between protein structure and its modeled mutant Whether working in the field of bioinformatics or molecular biology research or taking courses in protein modeling, readers will find the content in this book invaluable.
Orlando Furioso Chor Graphie De Michel Hallet Eghoyan
DOWNLOAD
Author :
language : en
Publisher:
Release Date : 1984
Orlando Furioso Chor Graphie De Michel Hallet Eghoyan written by and has been published by this book supported file pdf, txt, epub, kindle and other format this book has been release on 1984 with categories.
Bayesian Methods In Structural Bioinformatics
DOWNLOAD
Author : Thomas Hamelryck
language : en
Publisher: Springer
Release Date : 2012-03-23
Bayesian Methods In Structural Bioinformatics written by Thomas Hamelryck and has been published by Springer this book supported file pdf, txt, epub, kindle and other format this book has been release on 2012-03-23 with Medical categories.
This book is an edited volume, the goal of which is to provide an overview of the current state-of-the-art in statistical methods applied to problems in structural bioinformatics (and in particular protein structure prediction, simulation, experimental structure determination and analysis). It focuses on statistical methods that have a clear interpretation in the framework of statistical physics, rather than ad hoc, black box methods based on neural networks or support vector machines. In addition, the emphasis is on methods that deal with biomolecular structure in atomic detail. The book is highly accessible, and only assumes background knowledge on protein structure, with a minimum of mathematical knowledge. Therefore, the book includes introductory chapters that contain a solid introduction to key topics such as Bayesian statistics and concepts in machine learning and statistical physics.
From Networks To Proteins
DOWNLOAD
Author : Yin Chen
language : en
Publisher:
Release Date : 2011
From Networks To Proteins written by Yin Chen and has been published by this book supported file pdf, txt, epub, kindle and other format this book has been release on 2011 with categories.
Abstract: Markov chain and Markov random field (MRF) theory provides a consistent way to model context-dependent entities and correlated features. It is used in conjunction with statistical decision and estimation theories to formulate objective functions in terms of established optimality principles. This dissertation applies MRF modeling and optimization in two areas: network security and protein docking. The first problem is network anomaly detection. The objective is to detect statistically significant temporal or spatial changes in either the underlying process being monitored or the network operation itself. These changes may point to faults, threats, misbehavior, or other anomalies that require intervention. A new statistical anomaly detection framework is introduced that uses Markov models to characterize the "normal" behavior of the network. A series of Markov models are developed, including a node-level model, two tree-indexed models, and a networked Markov chain model to capture temporal and spatial features of network evolution. Large deviations techniques are employed to compare the empirical distribution (estimated from past activity traces) with its most recent empirical measure. Optimal decision rules are developed for each model to identify anomalies in recent activities. Simulation results validate the effectiveness of the proposed anomaly detection algorithms. The second application lies in bioinformatics where the objective is to computationally predict the structure of a complex of two interacting proteins. In the refinement stage of a multi-stage protein docking process, side-chains are extracted from the docking interface and positioned optimally by minimizing an energy function which models interactions among atoms. This combinatorial optimization problem is formulated as a maximum weighted independent set (MWIS) problem. The MWIS problem is NP-hard, yet, the thesis develops a distributed message passing algorithm that can produce effective feasible solutions. The approach is tested on a benchmark of 17 proteins and the results indicate that optimal side-chain positioning significantly improves the binding energy landscape, increasing the correlation between energy and root mean square deviation (RMSD) from the native structure. This new side-chain positioning procedure has the potential to accelerate refinement algorithms seeking very accurate predictions of the native structure and thus serve as an important step in computational docking protocols.