Modes Of Parametric Statistical Inference
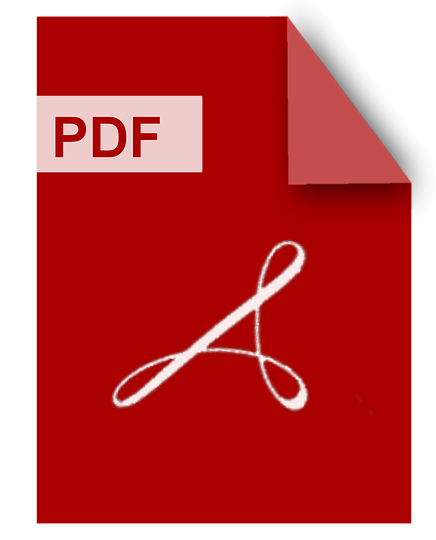
DOWNLOAD
Download Modes Of Parametric Statistical Inference PDF/ePub or read online books in Mobi eBooks. Click Download or Read Online button to get Modes Of Parametric Statistical Inference book now. This website allows unlimited access to, at the time of writing, more than 1.5 million titles, including hundreds of thousands of titles in various foreign languages. If the content not found or just blank you must refresh this page
Modes Of Parametric Statistical Inference
DOWNLOAD
Author : Seymour Geisser
language : en
Publisher: John Wiley & Sons
Release Date : 2006-01-27
Modes Of Parametric Statistical Inference written by Seymour Geisser and has been published by John Wiley & Sons this book supported file pdf, txt, epub, kindle and other format this book has been release on 2006-01-27 with Mathematics categories.
A fascinating investigation into the foundations of statistical inference This publication examines the distinct philosophical foundations of different statistical modes of parametric inference. Unlike many other texts that focus on methodology and applications, this book focuses on a rather unique combination of theoretical and foundational aspects that underlie the field of statistical inference. Readers gain a deeper understanding of the evolution and underlying logic of each mode as well as each mode's strengths and weaknesses. The book begins with fascinating highlights from the history of statistical inference. Readers are given historical examples of statistical reasoning used to address practical problems that arose throughout the centuries. Next, the book goes on to scrutinize four major modes of statistical inference: * Frequentist * Likelihood * Fiducial * Bayesian The author provides readers with specific examples and counterexamples of situations and datasets where the modes yield both similar and dissimilar results, including a violation of the likelihood principle in which Bayesian and likelihood methods differ from frequentist methods. Each example is followed by a detailed discussion of why the results may have varied from one mode to another, helping the reader to gain a greater understanding of each mode and how it works. Moreover, the author provides considerable mathematical detail on certain points to highlight key aspects of theoretical development. The author's writing style and use of examples make the text clear and engaging. This book is fundamental reading for graduate-level students in statistics as well as anyone with an interest in the foundations of statistics and the principles underlying statistical inference, including students in mathematics and the philosophy of science. Readers with a background in theoretical statistics will find the text both accessible and absorbing.
Non Parametric Statistical Diagnosis
DOWNLOAD
Author : E. Brodsky
language : en
Publisher: Springer Science & Business Media
Release Date : 2013-03-14
Non Parametric Statistical Diagnosis written by E. Brodsky and has been published by Springer Science & Business Media this book supported file pdf, txt, epub, kindle and other format this book has been release on 2013-03-14 with Mathematics categories.
Non-Parametric Statistical Diagnosis
Nonparametric Statistical Inference
DOWNLOAD
Author : Jean Dickinson Gibbons
language : en
Publisher: CRC Press
Release Date : 2020-12-21
Nonparametric Statistical Inference written by Jean Dickinson Gibbons and has been published by CRC Press this book supported file pdf, txt, epub, kindle and other format this book has been release on 2020-12-21 with Mathematics categories.
Praise for previous editions: "... a classic with a long history." – Statistical Papers "The fact that the first edition of this book was published in 1971 ... [is] testimony to the book’s success over a long period." – ISI Short Book Reviews "... one of the best books available for a theory course on nonparametric statistics. ... very well written and organized ... recommended for teachers and graduate students." – Biometrics "... There is no competitor for this book and its comprehensive development and application of nonparametric methods. Users of one of the earlier editions should certainly consider upgrading to this new edition." – Technometrics "... Useful to students and research workers ... a good textbook for a beginning graduate-level course in nonparametric statistics." – Journal of the American Statistical Association Since its first publication in 1971, Nonparametric Statistical Inference has been widely regarded as the source for learning about nonparametrics. The Sixth Edition carries on this tradition and incorporates computer solutions based on R. Features Covers the most commonly used nonparametric procedures States the assumptions, develops the theory behind the procedures, and illustrates the techniques using realistic examples from the social, behavioral, and life sciences Presents tests of hypotheses, confidence-interval estimation, sample size determination, power, and comparisons of competing procedures Includes an Appendix of user-friendly tables needed for solutions to all data-oriented examples Gives examples of computer applications based on R, MINITAB, STATXACT, and SAS Lists over 100 new references Nonparametric Statistical Inference, Sixth Edition, has been thoroughly revised and rewritten to make it more readable and reader-friendly. All of the R solutions are new and make this book much more useful for applications in modern times. It has been updated throughout and contains 100 new citations, including some of the most recent, to make it more current and useful for researchers.
Non Standard Parametric Statistical Inference
DOWNLOAD
Author : Russell Cheng
language : en
Publisher: Oxford University Press
Release Date : 2017-09-15
Non Standard Parametric Statistical Inference written by Russell Cheng and has been published by Oxford University Press this book supported file pdf, txt, epub, kindle and other format this book has been release on 2017-09-15 with Mathematics categories.
This book discusses the fitting of parametric statistical models to data samples. Emphasis is placed on: (i) how to recognize situations where the problem is non-standard when parameter estimates behave unusually, and (ii) the use of parametric bootstrap resampling methods in analyzing such problems. A frequentist likelihood-based viewpoint is adopted, for which there is a well-established and very practical theory. The standard situation is where certain widely applicable regularity conditions hold. However, there are many apparently innocuous situations where standard theory breaks down, sometimes spectacularly. Most of the departures from regularity are described geometrically, with only sufficient mathematical detail to clarify the non-standard nature of a problem and to allow formulation of practical solutions. The book is intended for anyone with a basic knowledge of statistical methods, as is typically covered in a university statistical inference course, wishing to understand or study how standard methodology might fail. Easy to understand statistical methods are presented which overcome these difficulties, and demonstrated by detailed examples drawn from real applications. Simple and practical model-building is an underlying theme. Parametric bootstrap resampling is used throughout for analyzing the properties of fitted models, illustrating its ease of implementation even in non-standard situations. Distributional properties are obtained numerically for estimators or statistics not previously considered in the literature because their theoretical distributional properties are too hard to obtain theoretically. Bootstrap results are presented mainly graphically in the book, providing an accessible demonstration of the sampling behaviour of estimators.
Nonparametric Statistical Inference
DOWNLOAD
Author : Jean Dickinson Gibbons
language : en
Publisher: CRC Press
Release Date : 2014-03-10
Nonparametric Statistical Inference written by Jean Dickinson Gibbons and has been published by CRC Press this book supported file pdf, txt, epub, kindle and other format this book has been release on 2014-03-10 with Mathematics categories.
Thoroughly revised and reorganized, the fourth edition presents in-depth coverage of the theory and methods of the most widely used nonparametric procedures in statistical analysis and offers example applications appropriate for all areas of the social, behavioral, and life sciences. The book presents new material on the quantiles, the calculation of exact and simulated power, multiple comparisons, additional goodness-of-fit tests, methods of analysis of count data, and modern computer applications using MINITAB, SAS, and STATXACT. It includes tabular guides for simplified applications of tests and finding P values and confidence interval estimates.
A History Of Parametric Statistical Inference From Bernoulli To Fisher 1713 1935
DOWNLOAD
Author : Anders Hald
language : en
Publisher: Springer Science & Business Media
Release Date : 2008-08-24
A History Of Parametric Statistical Inference From Bernoulli To Fisher 1713 1935 written by Anders Hald and has been published by Springer Science & Business Media this book supported file pdf, txt, epub, kindle and other format this book has been release on 2008-08-24 with Mathematics categories.
This book offers a detailed history of parametric statistical inference. Covering the period between James Bernoulli and R.A. Fisher, it examines: binomial statistical inference; statistical inference by inverse probability; the central limit theorem and linear minimum variance estimation by Laplace and Gauss; error theory, skew distributions, correlation, sampling distributions; and the Fisherian Revolution. Lively biographical sketches of many of the main characters are featured throughout, including Laplace, Gauss, Edgeworth, Fisher, and Karl Pearson. Also examined are the roles played by DeMoivre, James Bernoulli, and Lagrange.
Parametric Statistical Inference
DOWNLOAD
Author : James K. Lindsey
language : en
Publisher: Oxford University Press
Release Date : 1996
Parametric Statistical Inference written by James K. Lindsey and has been published by Oxford University Press this book supported file pdf, txt, epub, kindle and other format this book has been release on 1996 with Mathematics categories.
Two unifying components of statistics are the likelihood function and the exponential family. These are brought together for the first time as the central themes in this book on statistical inference, written for advanced undergraduate and graduate students in mathematical statistics.
Nonparametric Statistical Inference
DOWNLOAD
Author : Jean Dickinson Gibbons
language : en
Publisher: CRC Press
Release Date : 2010-07-26
Nonparametric Statistical Inference written by Jean Dickinson Gibbons and has been published by CRC Press this book supported file pdf, txt, epub, kindle and other format this book has been release on 2010-07-26 with Mathematics categories.
Proven Material for a Course on the Introduction to the Theory and/or on the Applications of Classical Nonparametric Methods Since its first publication in 1971, Nonparametric Statistical Inference has been widely regarded as the source for learning about nonparametric statistics. The fifth edition carries on this tradition while thoroughly revising at least 50 percent of the material. New to the Fifth Edition Updated and revised contents based on recent journal articles in the literature A new section in the chapter on goodness-of-fit tests A new chapter that offers practical guidance on how to choose among the various nonparametric procedures covered Additional problems and examples Improved computer figures This classic, best-selling statistics book continues to cover the most commonly used nonparametric procedures. The authors carefully state the assumptions, develop the theory behind the procedures, and illustrate the techniques using realistic research examples from the social, behavioral, and life sciences. For most procedures, they present the tests of hypotheses, confidence interval estimation, sample size determination, power, and comparisons of other relevant procedures. The text also gives examples of computer applications based on Minitab, SAS, and StatXact and compares these examples with corresponding hand calculations. The appendix includes a collection of tables required for solving the data-oriented problems. Nonparametric Statistical Inference, Fifth Edition provides in-depth yet accessible coverage of the theory and methods of nonparametric statistical inference procedures. It takes a practical approach that draws on scores of examples and problems and minimizes the theorem-proof format. Jean Dickinson Gibbons was recently interviewed regarding her generous pledge to Virginia Tech.
Statistical Inference
DOWNLOAD
Author : Michael J. Panik
language : en
Publisher: John Wiley & Sons
Release Date : 2012-06-06
Statistical Inference written by Michael J. Panik and has been published by John Wiley & Sons this book supported file pdf, txt, epub, kindle and other format this book has been release on 2012-06-06 with Mathematics categories.
A concise, easily accessible introduction to descriptive and inferential techniques Statistical Inference: A Short Course offers a concise presentation of the essentials of basic statistics for readers seeking to acquire a working knowledge of statistical concepts, measures, and procedures. The author conducts tests on the assumption of randomness and normality, provides nonparametric methods when parametric approaches might not work. The book also explores how to determine a confidence interval for a population median while also providing coverage of ratio estimation, randomness, and causality. To ensure a thorough understanding of all key concepts, Statistical Inference provides numerous examples and solutions along with complete and precise answers to many fundamental questions, including: How do we determine that a given dataset is actually a random sample? With what level of precision and reliability can a population sample be estimated? How are probabilities determined and are they the same thing as odds? How can we predict the level of one variable from that of another? What is the strength of the relationship between two variables? The book is organized to present fundamental statistical concepts first, with later chapters exploring more advanced topics and additional statistical tests such as Distributional Hypotheses, Multinomial Chi-Square Statistics, and the Chi-Square Distribution. Each chapter includes appendices and exercises, allowing readers to test their comprehension of the presented material. Statistical Inference: A Short Course is an excellent book for courses on probability, mathematical statistics, and statistical inference at the upper-undergraduate and graduate levels. The book also serves as a valuable reference for researchers and practitioners who would like to develop further insights into essential statistical tools.
Methods For Agnostic Statistical Inference
DOWNLOAD
Author : Kendrick Qijun Li
language : en
Publisher:
Release Date : 2021
Methods For Agnostic Statistical Inference written by Kendrick Qijun Li and has been published by this book supported file pdf, txt, epub, kindle and other format this book has been release on 2021 with categories.
A traditional goal of parametric statistics is to estimate some or all of a data-generating model's finite set of parameters, thereby turning data into scientific insights. Point estimates of parameters, and corresponding standard error estimates are used to quantify the information provided by the data. However, in reality the true data-generation rarely follows the assumed model. When the model assumptions are incorrect, though the point estimates' target parameters are often still meaningful quantities, the model-based standard error estimates may be difficult to interpret in any helpful way; they may also be notably biased. In light of doubts about model assumptions and the known difficulties of checking models, agnostic statistics aims to develop statistical inference with only minimal assumptions. Though agnostic statistics has become popular over the past few decades, methods of agnostic inference are yet to be developed in some fundamental application areas. One such area is meta-analysis. Meta-analysis of 2-by-2 tables is common and useful in research topics including analysis of adverse events and survey research data. Fixed-effects inference typically centers on measures of association such as the Cochran-Mantel-Haenszel statistic or Woolf's estimator, but to obtain well-calibrated inference when studies are small most methods rely on assuming exact homogeneity across studies, which is often unrealistic. By showing that estimators of several widely-used methods have meaningful estimands even in the presence of heterogeneity, we derive improved confidence intervals for them under heterogeneity. These improvements over current methods are illustrated by simulation. We find that our confidence intervals provide coverage closer to the nominal level when heterogeneity is present, in both small and large-sample settings. The conventional confidence intervals derived under homogeneity are often conservative, though anti-conservative inferences occur in some scenarios. We also apply the proposed methods to a meta-analysis of 19 randomized clinical trials on the effect of sclerotherapy in preventing first bleeding for patients with cirrhosis and esophagogastric varices. Our methods provide a more interpretable approach to meta-analyzing binary data and more accuracy in characterizing the uncertainty of the estimates. Another area lacking agnostic methods is adaptive shrinkage estimation. Shrinkage estimation attempts to increase the precision of an estimator in exchange for introducing a modest bias. Standard shrinkage estimators in linear models include the James-Stein estimator, Ridge estimator, and LASSO. However, theories regarding the optimal amount of shrinkage and statistical properties of these estimators are often based on stringent distributional assumptions. In Chapter 3, we provide a unified framework of shrinkage estimation -- penalized precision-weighted least square estimation. We demonstrate that the James-Stein estimator, Ridge and LASSO are all penalized precision-weighted least-square estimators using model-based precision weights. Using a model-agnostic precision weighting matrix, we propose three shrinkage estimators in the novel framework: Rotated James-Stein estimator, Rotated Ridge, and Rotated LASSO. As we show, the three proposed estimators have theoretical properties and empirical performance that are comparable to the standard shrinkage estimators, while rotated LASSO has improved precision in some situations. We apply these estimators in a prostate cancer example. The third area is variance estimation in Bayesian inference. Many frequentist parametric statistical methods have large sample Bayesian analogs. However, there is no general Bayesian analog of ``robust'' covariance estimates, that are widely-used in frequentist work. In Chapter 4, we propose such an analog, produced as the Bayes rule under a form of balanced loss function. This loss combines standard parametric inference's goal of accurate estimation of the truth with less-standard fidelity of the data to the model. Besides being the large-sample equivalent of its frequentist counterpart, we show by simulation that the Bayesian robust standard error can also be used to construct Wald confidence intervals that improve small-sample coverage. We demonstrate the novel standard error estimates in a Bayesian linear regression model to study the association between systolic blood pressure and age in 2017-2018 NHANES data.