Natural Inductive Biases For Artificial Intelligence
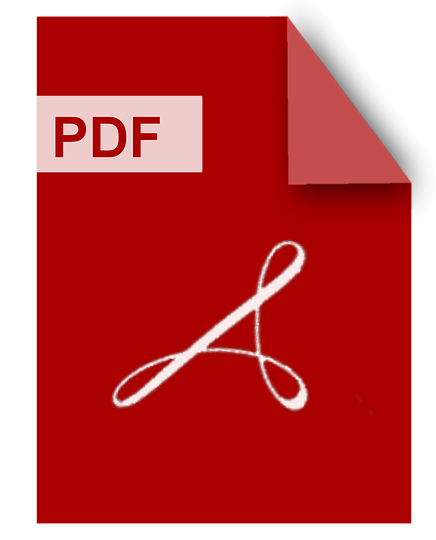
DOWNLOAD
Download Natural Inductive Biases For Artificial Intelligence PDF/ePub or read online books in Mobi eBooks. Click Download or Read Online button to get Natural Inductive Biases For Artificial Intelligence book now. This website allows unlimited access to, at the time of writing, more than 1.5 million titles, including hundreds of thousands of titles in various foreign languages. If the content not found or just blank you must refresh this page
Natural Inductive Biases For Artificial Intelligence
DOWNLOAD
Author : T. Anderson Keller
language : en
Publisher:
Release Date : 2023
Natural Inductive Biases For Artificial Intelligence written by T. Anderson Keller and has been published by this book supported file pdf, txt, epub, kindle and other format this book has been release on 2023 with categories.
"The study of inductive bias is one of the most all encompassing in all of machine learning. Inductive biases define not only the efficiency and speed of learning, but also what is ultimately possible to learn by a given machine learning system. The history of modern machine learning is intertwined with that of psychology, cognitive science and neuroscience, and therefore many of the most impactful inductive biases have come directly from these fields. Examples include convolutional neural networks, stemming from the observed organization of natural visual systems, and artificial neural networks themselves intending to model idolized abstract neural circuits. Given the dramatic successes of machine learning in recent years however, more emphasis has been placed on the engineering challenges faced by scaling up machine learning systems, with less focus on their inductive biases . This thesis will be an attempted step in the reverse direction. To do so, we will cover both naturally relevant learning algorithms, as well as natural structure inherent to neural representations. We will build artificial systems which are modeled after these natural properties, and we will demonstrate how they are both beneficial to computation, and may serve to help us better understand natural intelligence itself." --
Inductive Biases In Machine Learning For Robotics And Control
DOWNLOAD
Author : Michael Lutter
language : en
Publisher:
Release Date : 2023
Inductive Biases In Machine Learning For Robotics And Control written by Michael Lutter and has been published by this book supported file pdf, txt, epub, kindle and other format this book has been release on 2023 with categories.
One important robotics problem is "How can one program a robot to perform a task"? Classical robotics solves this problem by manually engineering modules for state estimation, planning, and control. In contrast, robot learning solely relies on black-box models and data. This book shows that these two approaches of classical engineering and black-box machine learning are not mutually exclusive. To solve tasks with robots, one can transfer insights from classical robotics to deep networks and obtain better learning algorithms for robotics and control. To highlight that incorporating existing knowledge as inductive biases in machine learning algorithms improves performance, this book covers different approaches for learning dynamics models and learning robust control policies. The presented algorithms leverage the knowledge of Newtonian Mechanics, Lagrangian Mechanics as well as the Hamilton-Jacobi-Isaacs differential equation as inductive bias and are evaluated on physical robots.
Change Of Representation And Inductive Bias
DOWNLOAD
Author : D. Paul Benjamin
language : en
Publisher: Springer
Release Date : 1989-12-31
Change Of Representation And Inductive Bias written by D. Paul Benjamin and has been published by Springer this book supported file pdf, txt, epub, kindle and other format this book has been release on 1989-12-31 with Computers categories.
Machine Learning Of Inductive Bias
DOWNLOAD
Author : Paul E. Utgoff
language : en
Publisher: Springer Science & Business Media
Release Date : 2012-12-06
Machine Learning Of Inductive Bias written by Paul E. Utgoff and has been published by Springer Science & Business Media this book supported file pdf, txt, epub, kindle and other format this book has been release on 2012-12-06 with Computers categories.
This book is based on the author's Ph.D. dissertation[56]. The the sis research was conducted while the author was a graduate student in the Department of Computer Science at Rutgers University. The book was pre pared at the University of Massachusetts at Amherst where the author is currently an Assistant Professor in the Department of Computer and Infor mation Science. Programs that learn concepts from examples are guided not only by the examples (and counterexamples) that they observe, but also by bias that determines which concept is to be considered as following best from the ob servations. Selection of a concept represents an inductive leap because the concept then indicates the classification of instances that have not yet been observed by the learning program. Learning programs that make undesir able inductive leaps do so due to undesirable bias. The research problem addressed here is to show how a learning program can learn a desirable inductive bias.
Social Inductive Biases For Reinforcement Learning
DOWNLOAD
Author : Adjodlah, Dhaval Dhamnidhi Kumar Adjodah
language : en
Publisher:
Release Date : 2019
Social Inductive Biases For Reinforcement Learning written by Adjodlah, Dhaval Dhamnidhi Kumar Adjodah and has been published by this book supported file pdf, txt, epub, kindle and other format this book has been release on 2019 with categories.
How can we build machines that collaborate and learn more seamlessly with humans, and with each other? How do we create fairer societies? How do we minimize the impact of information manipulation campaigns, and fight back? How do we build machine learning algorithms that are more sample efficient when learning from each other's sparse data, and under time constraints? At the root of these questions is a simple one: how do agents, human or machines, learn from each other, and can we improve it and apply it to new domains? The cognitive and social sciences have provided innumerable insights into how people learn from data using both passive observation and experimental intervention. Similarly, the statistics and machine learning communities have formalized learning as a rigorous and testable computational process. There is a growing movement to apply insights from the cognitive and social sciences to improving machine learning, as well as opportunities to use machine learning as a sandbox to test, simulate and expand ideas from the cognitive and social sciences. A less researched and fertile part of this intersection is the modeling of social learning: past work has been more focused on how agents can learn from the 'environment', and there is less work that borrows from both communities to look into how agents learn from each other. This thesis presents novel contributions into the nature and usefulness of social learning as an inductive bias for reinforced learning. I start by presenting the results from two large-scale online human experiments: first, I observe Dunbar cognitive limits that shape and limit social learning in two different social trading platforms, with the additional contribution that synthetic financial bots that transcend human limitations can obtain higher profits even when using naive trading strategies. Second, I devise a novel online experiment to observe how people, at the individual level, update their belief of future financial asset prices (e.g. S&P 500 and Oil prices) from social information. I model such social learning using Bayesian models of cognition, and observe that people make strong distributional assumptions on the social data they observe (e.g. assuming that the likelihood data is unimodal). I were fortunate to collect one round of predictions during the Brexit market instability, and find that social learning leads to higher performance than when learning from the underlying price history (the environment) during such volatile times. Having observed the cognitive limits and biases people exhibit when learning from other agents, I present an motivational example of the strength of inductive biases in reinforcement learning: I implement a learning model with a relational inductive bias that pre-processes the environment state into a set of relationships between entities in the world. I observe strong improvements in performance and sample efficiency, and even observe the learned relationships to be strongly interpretable. Finally, given that most modern deep reinforcement learning algorithms are distributed (in that they have separate learning agents), I investigate the hypothesis that viewing deep reinforcement learning as a social learning distributed search problem could lead to strong improvements. I do so by creating a fully decentralized, sparsely-communicating and scalable learning algorithm, and observe strong learning improvements with lower communication bandwidth usage (between learning agents) when using communication topologies that naturally evolved due to social learning in humans. Additionally, I provide a theoretical upper bound (that agrees with our empirical results) regarding which communication topologies lead to the largest learning performance improvement. Given a future increasingly filled with decentralized autonomous machine learning systems that interact with humans, there is an increasing need to understand social learning to build resilient, scalable and effective learning systems, and this thesis provides insights into how to build such systems.
Inductive Bias In Machine Learning
DOWNLOAD
Author : Luca Rendsburg
language : en
Publisher:
Release Date : 2022
Inductive Bias In Machine Learning written by Luca Rendsburg and has been published by this book supported file pdf, txt, epub, kindle and other format this book has been release on 2022 with categories.
Inductive bias describes the preference for solutions that a machine learning algorithm holds before seeing any data. It is a necessary ingredient for the goal of machine learning, which is to generalize from a set of examples to unseen data points. Yet, the inductive bias of learning algorithms is often not specified explicitly in practice, which prevents a theoretical understanding and undermines trust in machine learning. This issue is most prominently visible in the contemporary case of deep learning, which is widely successful in applications but relies on many poorly understood techniques and heuristics. This thesis aims to uncover the hidden inductive biases of machine learning algorithms. In the first part of the thesis, we uncover the implicit inductive bias of NetGAN, a complex graph generative model with seemingly no prior preferences. We find that the root of its generalization properties does not lie in the GAN architecture but in an inconspicuous low-rank approximation. We then use this insight to strip NetGAN of all unnecessary parts, including the GAN, and obtain a highly simplified reformulation. Next, we present a generic algorithm that reverse-engineers hidden inductive bias in approximate Bayesian inference. While the inductive bias is completely described by the prior distribution in full Bayesian inference, real-world applications often resort to approximate techniques that can make uncontrollable errors. By reframing the problem in terms of incompatible conditional distributions, we arrive at a generic algorithm based on pseudo-Gibbs sampling that attributes the change in inductive bias to a change in the prior distribution. The last part of the thesis concerns a common inductive bias in causal learning, the assumption of independent causal mechanisms. Under this assumption, we consider estimators for confounding strength, which governs the generalization ability from observational distribution to the underlying causal model. We show that an existing estimator is generally inconsistent and propose a consistent estimator based on tools from random matrix theory.
Machine Learning Q And Ai
DOWNLOAD
Author : Sebastian Raschka
language : en
Publisher: No Starch Press
Release Date : 2024-04-16
Machine Learning Q And Ai written by Sebastian Raschka and has been published by No Starch Press this book supported file pdf, txt, epub, kindle and other format this book has been release on 2024-04-16 with Computers categories.
Learn the answers to 30 cutting-edge questions in machine learning and AI and level up your expertise in the field. If you’re ready to venture beyond introductory concepts and dig deeper into machine learning, deep learning, and AI, the question-and-answer format of Machine Learning Q and AI will make things fast and easy for you, without a lot of mucking about. Born out of questions often fielded by author Sebastian Raschka, the direct, no-nonsense approach of this book makes advanced topics more accessible and genuinely engaging. Each brief, self-contained chapter journeys through a fundamental question in AI, unraveling it with clear explanations, diagrams, and hands-on exercises. WHAT'S INSIDE: FOCUSED CHAPTERS: Key questions in AI are answered concisely, and complex ideas are broken down into easily digestible parts. WIDE RANGE OF TOPICS: Raschka covers topics ranging from neural network architectures and model evaluation to computer vision and natural language processing. PRACTICAL APPLICATIONS: Learn techniques for enhancing model performance, fine-tuning large models, and more. You’ll also explore how to: • Manage the various sources of randomness in neural network training • Differentiate between encoder and decoder architectures in large language models • Reduce overfitting through data and model modifications • Construct confidence intervals for classifiers and optimize models with limited labeled data • Choose between different multi-GPU training paradigms and different types of generative AI models • Understand performance metrics for natural language processing • Make sense of the inductive biases in vision transformers If you’ve been on the hunt for the perfect resource to elevate your understanding of machine learning, Machine Learning Q and AI will make it easy for you to painlessly advance your knowledge beyond the basics.
Change Of Representation And Inductive Bias
DOWNLOAD
Author : D. Paul Benjamin
language : en
Publisher:
Release Date : 1989-12-31
Change Of Representation And Inductive Bias written by D. Paul Benjamin and has been published by this book supported file pdf, txt, epub, kindle and other format this book has been release on 1989-12-31 with categories.
Inductive Biases In Machine Learning For Robotics And Control
DOWNLOAD
Author : Michael Lutter
language : en
Publisher: Springer Nature
Release Date : 2023-07-31
Inductive Biases In Machine Learning For Robotics And Control written by Michael Lutter and has been published by Springer Nature this book supported file pdf, txt, epub, kindle and other format this book has been release on 2023-07-31 with Technology & Engineering categories.
One important robotics problem is “How can one program a robot to perform a task”? Classical robotics solves this problem by manually engineering modules for state estimation, planning, and control. In contrast, robot learning solely relies on black-box models and data. This book shows that these two approaches of classical engineering and black-box machine learning are not mutually exclusive. To solve tasks with robots, one can transfer insights from classical robotics to deep networks and obtain better learning algorithms for robotics and control. To highlight that incorporating existing knowledge as inductive biases in machine learning algorithms improves performance, this book covers different approaches for learning dynamics models and learning robust control policies. The presented algorithms leverage the knowledge of Newtonian Mechanics, Lagrangian Mechanics as well as the Hamilton-Jacobi-Isaacs differential equation as inductive bias and are evaluated on physical robots.
Inductive Biases In A Reinforcement Learner
DOWNLOAD
Author : Helen G. Cobb
language : en
Publisher:
Release Date : 1992
Inductive Biases In A Reinforcement Learner written by Helen G. Cobb and has been published by this book supported file pdf, txt, epub, kindle and other format this book has been release on 1992 with categories.