Nonparametric Density Estimation With Applications
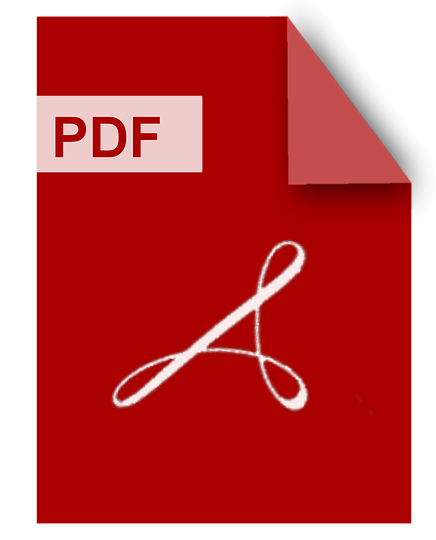
DOWNLOAD
Download Nonparametric Density Estimation With Applications PDF/ePub or read online books in Mobi eBooks. Click Download or Read Online button to get Nonparametric Density Estimation With Applications book now. This website allows unlimited access to, at the time of writing, more than 1.5 million titles, including hundreds of thousands of titles in various foreign languages. If the content not found or just blank you must refresh this page
Nonparametric Density Estimation With Applications
DOWNLOAD
Author : Steven J. Bean
language : en
Publisher:
Release Date : 1979
Nonparametric Density Estimation With Applications written by Steven J. Bean and has been published by this book supported file pdf, txt, epub, kindle and other format this book has been release on 1979 with Nonparametric statistics categories.
Nonparametric Kernel Density Estimation And Its Computational Aspects
DOWNLOAD
Author : Artur Gramacki
language : en
Publisher: Springer
Release Date : 2017-12-21
Nonparametric Kernel Density Estimation And Its Computational Aspects written by Artur Gramacki and has been published by Springer this book supported file pdf, txt, epub, kindle and other format this book has been release on 2017-12-21 with Technology & Engineering categories.
This book describes computational problems related to kernel density estimation (KDE) – one of the most important and widely used data smoothing techniques. A very detailed description of novel FFT-based algorithms for both KDE computations and bandwidth selection are presented. The theory of KDE appears to have matured and is now well developed and understood. However, there is not much progress observed in terms of performance improvements. This book is an attempt to remedy this. The book primarily addresses researchers and advanced graduate or postgraduate students who are interested in KDE and its computational aspects. The book contains both some background and much more sophisticated material, hence also more experienced researchers in the KDE area may find it interesting. The presented material is richly illustrated with many numerical examples using both artificial and real datasets. Also, a number of practical applications related to KDE are presented.
Kernel Smoothing
DOWNLOAD
Author : Sucharita Ghosh
language : en
Publisher: John Wiley & Sons
Release Date : 2018-01-09
Kernel Smoothing written by Sucharita Ghosh and has been published by John Wiley & Sons this book supported file pdf, txt, epub, kindle and other format this book has been release on 2018-01-09 with Mathematics categories.
Comprehensive theoretical overview of kernel smoothing methods with motivating examples Kernel smoothing is a flexible nonparametric curve estimation method that is applicable when parametric descriptions of the data are not sufficiently adequate. This book explores theory and methods of kernel smoothing in a variety of contexts, considering independent and correlated data e.g. with short-memory and long-memory correlations, as well as non-Gaussian data that are transformations of latent Gaussian processes. These types of data occur in many fields of research, e.g. the natural and the environmental sciences, and others. Nonparametric density estimation, nonparametric and semiparametric regression, trend and surface estimation in particular for time series and spatial data and other topics such as rapid change points, robustness etc. are introduced alongside a study of their theoretical properties and optimality issues, such as consistency and bandwidth selection. Addressing a variety of topics, Kernel Smoothing: Principles, Methods and Applications offers a user-friendly presentation of the mathematical content so that the reader can directly implement the formulas using any appropriate software. The overall aim of the book is to describe the methods and their theoretical backgrounds, while maintaining an analytically simple approach and including motivating examples—making it extremely useful in many sciences such as geophysics, climate research, forestry, ecology, and other natural and life sciences, as well as in finance, sociology, and engineering. A simple and analytical description of kernel smoothing methods in various contexts Presents the basics as well as new developments Includes simulated and real data examples Kernel Smoothing: Principles, Methods and Applications is a textbook for senior undergraduate and graduate students in statistics, as well as a reference book for applied statisticians and advanced researchers.
Kernel Based Nonparametric Density Estimation And Regression With Statistical Applications
DOWNLOAD
Author : Peter John Foster
language : en
Publisher:
Release Date : 1990
Kernel Based Nonparametric Density Estimation And Regression With Statistical Applications written by Peter John Foster and has been published by this book supported file pdf, txt, epub, kindle and other format this book has been release on 1990 with Nonparametric statistics categories.
Applications Of Non Parametric Density Estimation
DOWNLOAD
Author : Ahmed Mohamed Mohamed Sultan
language : en
Publisher:
Release Date : 1990
Applications Of Non Parametric Density Estimation written by Ahmed Mohamed Mohamed Sultan and has been published by this book supported file pdf, txt, epub, kindle and other format this book has been release on 1990 with Density functionals categories.
The dissertation examines various methods of nonparametric density estimation, and nonparametric kernel estimation in more detail. The consequences of various kernel window width and their effect on the mean integrated square error are examined using Monte Carlo techniques. The mean and the variance of nonparametric density estimator is derived for symmetric kernels with finite mean and finite variance. The results also treat kernels with varying window parameters. The nonparametric kernel estimate was used to obtain new estimators for the three parameter Weibull distribution using distance estimation and the Cramer-von-Mises statistic. Comparison with maximum likelihood estimators using a Monte Carlo sample of size 1000 and various different parameters showed a significant improvement over the maximum likelihood estimators in the mean integrated square error between the estimated distribution and the true distribution. Several new goodness of fit tests are proposed using the nonparametric kernel estimator and the Cramer-von-Mises and the Anderson Darling statistics. Extensive Monte Carlo experiments were performed to obtain the critical values for the test and to study the power of the tests against eight alternative distributions. The tests using the Anderson Darling statistic showed greater power against almost all alternative distributions studied than the K.S. test. (kr).
Multivariate Density Estimation
DOWNLOAD
Author : David W. Scott
language : en
Publisher: John Wiley & Sons
Release Date : 2015-03-30
Multivariate Density Estimation written by David W. Scott and has been published by John Wiley & Sons this book supported file pdf, txt, epub, kindle and other format this book has been release on 2015-03-30 with Mathematics categories.
Clarifies modern data analysis through nonparametric density estimation for a complete working knowledge of the theory and methods Featuring a thoroughly revised presentation, Multivariate Density Estimation: Theory, Practice, and Visualization, Second Edition maintains an intuitive approach to the underlying methodology and supporting theory of density estimation. Including new material and updated research in each chapter, the Second Edition presents additional clarification of theoretical opportunities, new algorithms, and up-to-date coverage of the unique challenges presented in the field of data analysis. The new edition focuses on the various density estimation techniques and methods that can be used in the field of big data. Defining optimal nonparametric estimators, the Second Edition demonstrates the density estimation tools to use when dealing with various multivariate structures in univariate, bivariate, trivariate, and quadrivariate data analysis. Continuing to illustrate the major concepts in the context of the classical histogram, Multivariate Density Estimation: Theory, Practice, and Visualization, Second Edition also features: Over 150 updated figures to clarify theoretical results and to show analyses of real data sets An updated presentation of graphic visualization using computer software such as R A clear discussion of selections of important research during the past decade, including mixture estimation, robust parametric modeling algorithms, and clustering More than 130 problems to help readers reinforce the main concepts and ideas presented Boxed theorems and results allowing easy identification of crucial ideas Figures in color in the digital versions of the book A website with related data sets Multivariate Density Estimation: Theory, Practice, and Visualization, Second Edition is an ideal reference for theoretical and applied statisticians, practicing engineers, as well as readers interested in the theoretical aspects of nonparametric estimation and the application of these methods to multivariate data. The Second Edition is also useful as a textbook for introductory courses in kernel statistics, smoothing, advanced computational statistics, and general forms of statistical distributions.
Multivariate Long Memory Processes And Nonparametric Density Estimation With Applications To Ridge Detection
DOWNLOAD
Author : Klaus Telkmann
language : en
Publisher:
Release Date : 2018
Multivariate Long Memory Processes And Nonparametric Density Estimation With Applications To Ridge Detection written by Klaus Telkmann and has been published by this book supported file pdf, txt, epub, kindle and other format this book has been release on 2018 with Memory categories.
Nonparametric Density Estimation Using Correlated Data With Application To Screening Problems
DOWNLOAD
Author : Junjun Qin
language : en
Publisher:
Release Date : 2013
Nonparametric Density Estimation Using Correlated Data With Application To Screening Problems written by Junjun Qin and has been published by this book supported file pdf, txt, epub, kindle and other format this book has been release on 2013 with categories.
To detect a rare phenomenon with a higher probability by means of some other variable(s) which is (are) highly related but easier in technology or cheaper in expenditure is termed as "screening". It has come to be realized in recent years that current methodologies are of limited practical value. They are either built on a parametric framework which in fact we have little knowledge about or little involved with "multivariate screening" and "multi-stage screening", which are more frequent and more important in practice. Therefore, the alternative method discussed here suggests that we consider a nonparametric density estimation using correlated data. This one derives from and greatly formulates a rough idea that screening in nature is a kind of problems about how to solve probabilities and thus we intuitively look for estimating the probability density function (pdf). Since we have little information about data in a regular setting, nonparametric estimation seems a best candidate. In this study, the approach is presented by firstly making nonparametric density estimation in the case where random variables X and Y are both continuous and in the case where X is continuous but Y is discrete respectively. The behaviors under the large sample setting are explored and the performance of the proposed method is evaluated through simulations. The proposed method is then applied to a subsequent screening problem. The comparison with some current method is also presented.
Nonparametric Curve Estimation
DOWNLOAD
Author : Sam Efromovich
language : en
Publisher: Springer Science & Business Media
Release Date : 2008-01-19
Nonparametric Curve Estimation written by Sam Efromovich and has been published by Springer Science & Business Media this book supported file pdf, txt, epub, kindle and other format this book has been release on 2008-01-19 with Mathematics categories.
This book gives a systematic, comprehensive, and unified account of modern nonparametric statistics of density estimation, nonparametric regression, filtering signals, and time series analysis. The companion software package, available over the Internet, brings all of the discussed topics into the realm of interactive research. Virtually every claim and development mentioned in the book is illustrated with graphs which are available for the reader to reproduce and modify, making the material fully transparent and allowing for complete interactivity.
Density Estimation For Statistics And Data Analysis
DOWNLOAD
Author : Bernard. W. Silverman
language : en
Publisher: Routledge
Release Date : 2018-02-19
Density Estimation For Statistics And Data Analysis written by Bernard. W. Silverman and has been published by Routledge this book supported file pdf, txt, epub, kindle and other format this book has been release on 2018-02-19 with Mathematics categories.
Although there has been a surge of interest in density estimation in recent years, much of the published research has been concerned with purely technical matters with insufficient emphasis given to the technique's practical value. Furthermore, the subject has been rather inaccessible to the general statistician. The account presented in this book places emphasis on topics of methodological importance, in the hope that this will facilitate broader practical application of density estimation and also encourage research into relevant theoretical work. The book also provides an introduction to the subject for those with general interests in statistics. The important role of density estimation as a graphical technique is reflected by the inclusion of more than 50 graphs and figures throughout the text. Several contexts in which density estimation can be used are discussed, including the exploration and presentation of data, nonparametric discriminant analysis, cluster analysis, simulation and the bootstrap, bump hunting, projection pursuit, and the estimation of hazard rates and other quantities that depend on the density. This book includes general survey of methods available for density estimation. The Kernel method, both for univariate and multivariate data, is discussed in detail, with particular emphasis on ways of deciding how much to smooth and on computation aspects. Attention is also given to adaptive methods, which smooth to a greater degree in the tails of the distribution, and to methods based on the idea of penalized likelihood.