Optimization Functions In Matlab
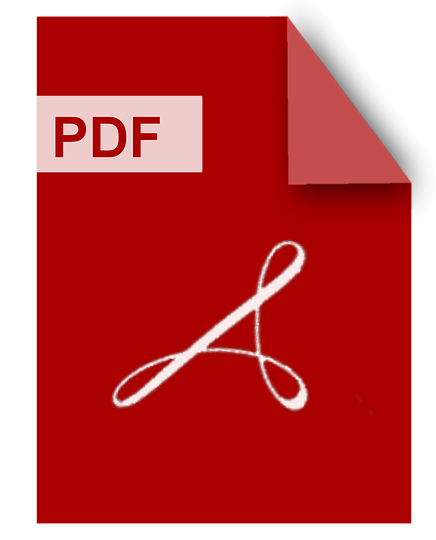
DOWNLOAD
Download Optimization Functions In Matlab PDF/ePub or read online books in Mobi eBooks. Click Download or Read Online button to get Optimization Functions In Matlab book now. This website allows unlimited access to, at the time of writing, more than 1.5 million titles, including hundreds of thousands of titles in various foreign languages. If the content not found or just blank you must refresh this page
Optimization Functions In Matlab
DOWNLOAD
Author : J Lopez
language : en
Publisher:
Release Date : 2019-07-21
Optimization Functions In Matlab written by J Lopez and has been published by this book supported file pdf, txt, epub, kindle and other format this book has been release on 2019-07-21 with categories.
Optimization Toolbox provides functions for finding parameters that minimize or maximize objectives while satisfying constraints. The toolbox includes solvers for linear programming (LP), mixed-integer linear programming (MILP), quadratic programming(QP), nonlinear programming (NLP), constrained linear least squares, nonlinear least squares, and nonlinear equations. You can define your optimization problem with functions and matrices or by specifying variable expressions that reflect the underlying mathematics. You can use the toolbox solvers to fin optimal solutions to continuous and discrete problems, perform trade of analyses, and incorporate optimization methods into algorithms and applications. The toolbox lets you perform design optimization tasks, including parameter estimation, component selection, and parameter tuning. It can be used to fin optimal solutions in applications such as portfolio optimization, resource allocation, and production planning and scheduling. This book develops the functions of Matlab for optimization through examples
Advanced Optimization Functions In Matlab
DOWNLOAD
Author : J Lopez
language : en
Publisher:
Release Date : 2019-07-08
Advanced Optimization Functions In Matlab written by J Lopez and has been published by this book supported file pdf, txt, epub, kindle and other format this book has been release on 2019-07-08 with categories.
Global Optimization Toolbox provides functions that search for global solutions to problems that contain multiple maxima or minima. Toolbox solvers include surrogate, pattern search, genetic algorithm, particle swarm, simulated annealing, multi start, and global search. You can use these solvers for optimization problems where the objective or constraint function is continuous, discontinuous, stochastic, does not possess derivatives, or includes simulations or black-box functions. For problems with multiple objectives, you can identify a Pareto front using genetic algorithm or pattern search solvers. You can improve solver effective es by adjusting options and, for applicable solvers, customizing creation, update, and search functions. You can use custom data types with the genetic algorithm and simulated annealing solvers to represent problems not easily expressed with standard data types. The hybrid function option lets you improve a solution by applying a second solver after the first.Global Optimization Toolbox functions include three direct search algorithms called the generalized pattern search (GPS) algorithm, the generating set search (GSS) algorithm, and the mesh adaptive search (MADS) algorithm. All are pattern search algorithms that compute a sequence of points that approach an optimal point. At each step, the algorithm searches a set of points, called a mesh, around the current point-the point computed at the previous step of the algorithm. The mesh is formed by adding the current point to a scalar multiple of a set of vectors called a pattern. If the pattern search algorithm finds a point in the mesh that improves the objective function at the current point, the new point becomes the current point at the next step of the algorithm.The GPS algorithm uses fixed direction vectors. The GSS algorithm is identical to the GPS algorithm, except when there are linear constraints, and when the current point is near a linear constraint boundary. The MADS algorithm uses a random selection of vectors to define the mesh.A surrogate is a function that approximates an objective function. The surrogate is useful because it takes little time to evaluate.Multiobjective optimization is concerned with the minimization of a vector of objectives F(x) that can be the subject of a number of constraints or bounds.In Big Data problems Parallel Processing is an attractive way to speed optimization algorithms. To use parallel processing, you must have a Parallel Computing Toolbox license, and have a parallel worker pool (parpool).This book develops the advanced functions of Matlab for optimization through examples
Matlab Optimization Functions And Examples
DOWNLOAD
Author : N. Foster
language : en
Publisher:
Release Date : 2016-12-29
Matlab Optimization Functions And Examples written by N. Foster and has been published by this book supported file pdf, txt, epub, kindle and other format this book has been release on 2016-12-29 with categories.
MATLAB Optimization Toolbox provides widely used algorithms for and large-scale optimization. These algorithms solve constrained and unconstrained continuous and discrete problems. The toolbox, developed in this book, includes functions for linear programming, quadratic programming, binary integer programming, nonlinear optimization, nonlinear least squares, systems of nonlinear equations, and multiobjective optimization. You can use them to find optimal solutions, perform tradeoff analyses, balance multiple design alternatives, and incorporate optimization methods into algorithms and models. This books develops the optimization functions in MATLAB and presents examples.
Optimization Functions In Matlab And Examples
DOWNLOAD
Author : Foster N.
language : en
Publisher: Createspace Independent Publishing Platform
Release Date : 2016-11-12
Optimization Functions In Matlab And Examples written by Foster N. and has been published by Createspace Independent Publishing Platform this book supported file pdf, txt, epub, kindle and other format this book has been release on 2016-11-12 with categories.
MATLAB Optimization Toolbox provides widely used algorithms for and large-scale optimization. These algorithms solve constrained and unconstrained continuous and discrete problems. The toolbox, developed in this book, includes functions for linear programming, quadratic programming, binary integer programming, nonlinear optimization, nonlinear least squares, systems of nonlinear equations, and multiobjective optimization. You can use them to find optimal solutions, perform tradeoff analyses, balance multiple design alternatives, and incorporate optimization methods into algorithms and models. This books develops the optimization functions in MATLAB and presents examples.The more important features are the next:* Interactive tools for defining and solving optimization problems and monitoring solution progress* Solvers for nonlinear and multiobjective optimization * Solvers for nonlinear least squares, data fitting, and nonlinear equations* Methods for solving quadratic and linear programming problems * Methods for solving binary integer programming problems* Parallel computing support in selected constrained nonlinear solvers
Operations Research Optimization Functions In Matlab For Linear And Nonlinear Programming
DOWNLOAD
Author : Perez C.
language : en
Publisher:
Release Date : 2017-08-16
Operations Research Optimization Functions In Matlab For Linear And Nonlinear Programming written by Perez C. and has been published by this book supported file pdf, txt, epub, kindle and other format this book has been release on 2017-08-16 with categories.
In the simplest case, an optimization problem consists of maximizing or minimizing a real function by systematically choosing input values from within an allowed set and computing the value of the function. The generalization of optimization theory and techniques to other formulations comprises a large area of applied mathematics. More generally, optimization includes finding "best available" values of some objective function given a defined domain (or input), including a variety of different types of objective functions and different types of domains.MATLAB Optimization Toolbox provides functions for finding parameters that minimize or maximize objectives while satisfying constraints. The toolbox includes solvers for linear programming, mixed-integer linear programming, quadratic programming, nonlinear optimization, and nonlinear least squares. You can use these solvers to find optimal solutions to continuous and discrete problems, perform tradeoff analyses, and incorporate optimization methods into algorithms and applications.Adding more than one objective to an optimization problem adds complexity. For example, to optimize a structural design, one would desire a design that is both light and rigid. When two objectives conflict, a trade-off must be created. There may be one lightest design, one stiffest design, and an infinite number of designs that are some compromise of weight and rigidity. The set of trade-off designs that cannot be improved upon according to one criterion without hurting another criterion is known as the Pareto set. The curve created plotting weight against stiffness of the best designs is known as the Pareto frontier.Also MATLAB Optimization Toolbox provides functions for MULTIOBJECTIVE, QUADRATIC and MIXED DATA PROGRAMMING
Advanced Optimization With Matlab
DOWNLOAD
Author : J Lopez
language : en
Publisher:
Release Date : 2019-06-18
Advanced Optimization With Matlab written by J Lopez and has been published by this book supported file pdf, txt, epub, kindle and other format this book has been release on 2019-06-18 with categories.
Optimization Toolbox provides functions for finding parameters that minimize or maximize objectives while satisfying constraints. The toolbox includes solvers for linear programming (LP), mixed-integer linear programming (MILP), quadratic programming(QP), nonlinear programming (NLP), constrained linear least squares, nonlinear least squares, and nonlinear equations. You can define your optimization problem with functions and matrices or by specifying variable expressions that reflect the underlying mathematics. You can use the toolbox solvers to fin optimal solutions to continuous and discrete problems, perform trade of analyses, and incorporate optimization methods into algorithms and applications. The toolbox lets you perform design optimization tasks, including parameter estimation, component selection, and parameter tuning. It can be used to fin optimal solutions in applications such as portfolio optimization, resource allocation, and production planning and scheduling.Global Optimization Toolbox provides functions that search for global solutions to problems that contain multiple maxima or minima. Toolbox solvers include surrogate, pattern search, genetic algorithm, particle swarm, simulated annealing, multi start, and global search. You can use these solvers for optimization problems where the objective or constraint function is continuous, discontinuous, stochastic, does not possess derivatives, or includes simulations or black-box functions. For problems with multiple objectives, you can identify a Pareto front using genetic algorithm or pattern search solvers. You can improve solver effective es by adjusting options and, for applicable solvers, customizing creation, update, and search functions. You can use custom data types with the genetic algorithm and simulated annealing solvers to represent problems not easily expressed with standard data types. The hybrid function option lets you improve a solution by applying a second solver after the first.
Advanced Optimization With Matlab Using Big Data Techniques
DOWNLOAD
Author : J Lopez
language : en
Publisher:
Release Date : 2019-07-07
Advanced Optimization With Matlab Using Big Data Techniques written by J Lopez and has been published by this book supported file pdf, txt, epub, kindle and other format this book has been release on 2019-07-07 with categories.
Global Optimization Toolbox provides functions that search for global solutions to problems that contain multiple maxima or minima. Toolbox solvers include surrogate, pattern search, genetic algorithm, particle swarm, simulated annealing, multi start, and global search. You can use these solvers for optimization problems where the objective or constraint function is continuous, discontinuous, stochastic, does not possess derivatives, or includes simulations or black-box functions. For problems with multiple objectives, you can identify a Pareto front using genetic algorithm or pattern search solvers. You can improve solver effective es by adjusting options and, for applicable solvers, customizing creation, update, and search functions. You can use custom data types with the genetic algorithm and simulated annealing solvers to represent problems not easily expressed with standard data types. The hybrid function option lets you improve a solution by applying a second solver after the first.Simulated annealing is a method for solving unconstrained and bound-constrained optimization problems. The method models the physical process of heating a material and then slowly lowering the temperature to decrease defects, thus minimizing the system energy. At each iteration of the simulated annealing algorithm, a new point is randomly generated. The distance of the new point from the current point, or the extent of the search, is based on a probability distribution with a scale proportional to the temperature. The algorithm accepts all new points that lower the objective, but also, with a certain probability, points that raise the objective. By accepting points that raise the objective, the algorithm avoids being trapped in local minima, and is able to explore globally for more possible solutions. An annealing schedule is selected to systematically decrease the temperature as the algorithm proceeds. As the temperature decreases, the algorithm reduces the extent of its search to converge to a minimum.You might need to formulate problems with more than one objective, since a single objective with several constraints may not adequately represent the problem being faced. If so, there is a vector of objectives, F(x) = [F1(x), F2(x), ..., Fm(x)], that must be traded off in some way. The relative importance of these objectives is not generally known until the system's best capabilities are determined and tradeoffs between the objectives fully understood. As the number of objectives increases, tradeoffs are likely to become complex and less easily quantified. The designer must rely on his or her intuition and ability to express preferences throughout the optimization cycle. Thus, requirements for a multiobjective design strategy must enable a natural problema formulation to be expressed, and be able to solve the problem and enter preferences into a numerically tractable and realistic design proble
Practical Optimization With Matlab
DOWNLOAD
Author : Mircea Ancău
language : en
Publisher: Cambridge Scholars Publishing
Release Date : 2019-10-03
Practical Optimization With Matlab written by Mircea Ancău and has been published by Cambridge Scholars Publishing this book supported file pdf, txt, epub, kindle and other format this book has been release on 2019-10-03 with Mathematics categories.
This easy-to-follow guide provides academics and industrial engineers with a state-of-the-art numerical approach to the most frequent technical and economical optimization methods. In an engaging manner, it provides the reader with not only a systematic and comprehensive study, but also with necessary and directly implementable code written in the versatile and readily available platform Matlab. The book offers optimization methods for univariate and multivariate constrained or unconstrained functions, general optimization methods and multicriteria optimization methods; provides intuitively, step-by-step explained sample Matlab code, that can be easily adjusted to meet individual requirements; and uses a clear, concise presentation style, which will be suited to readers even without a programming background, as well as to students preparing for examinations in optimization methods.
Project Optimization
DOWNLOAD
Author : Reyolando M.L.R.F. Brasil
language : en
Publisher: Walter de Gruyter GmbH & Co KG
Release Date : 2021-10-04
Project Optimization written by Reyolando M.L.R.F. Brasil and has been published by Walter de Gruyter GmbH & Co KG this book supported file pdf, txt, epub, kindle and other format this book has been release on 2021-10-04 with Technology & Engineering categories.
A comprehensive and easy to understand introduction to a wide range of tools to help designers to optimize their projects. The authors are engineers and therefore many of the examples are on engineering applications, but the techniques presented are common to various areas of knowledge and pervade disciplinary divisions. The book describes the fundamental ideas, mathematical and graphic methods and shows how to use Matlab and EXCEL for optimization.
Introduction To Nonlinear Optimization
DOWNLOAD
Author : Amir Beck
language : en
Publisher: SIAM
Release Date : 2014-10-27
Introduction To Nonlinear Optimization written by Amir Beck and has been published by SIAM this book supported file pdf, txt, epub, kindle and other format this book has been release on 2014-10-27 with Mathematics categories.
This book provides the foundations of the theory of nonlinear optimization as well as some related algorithms and presents a variety of applications from diverse areas of applied sciences. The author combines three pillars of optimization?theoretical and algorithmic foundation, familiarity with various applications, and the ability to apply the theory and algorithms on actual problems?and rigorously and gradually builds the connection between theory, algorithms, applications, and implementation. Readers will find more than 170 theoretical, algorithmic, and numerical exercises that deepen and enhance the reader's understanding of the topics. The author includes offers several subjects not typically found in optimization books?for example, optimality conditions in sparsity-constrained optimization, hidden convexity, and total least squares. The book also offers a large number of applications discussed theoretically and algorithmically, such as circle fitting, Chebyshev center, the Fermat?Weber problem, denoising, clustering, total least squares, and orthogonal regression and theoretical and algorithmic topics demonstrated by the MATLAB? toolbox CVX and a package of m-files that is posted on the book?s web site.