Practical Guide To Logistic Regression
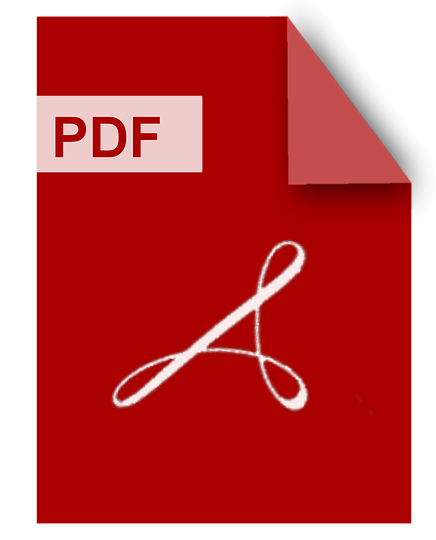
DOWNLOAD
Download Practical Guide To Logistic Regression PDF/ePub or read online books in Mobi eBooks. Click Download or Read Online button to get Practical Guide To Logistic Regression book now. This website allows unlimited access to, at the time of writing, more than 1.5 million titles, including hundreds of thousands of titles in various foreign languages. If the content not found or just blank you must refresh this page
Practical Guide To Logistic Regression
DOWNLOAD
Author : Joseph M. Hilbe
language : en
Publisher: CRC Press
Release Date : 2016-04-05
Practical Guide To Logistic Regression written by Joseph M. Hilbe and has been published by CRC Press this book supported file pdf, txt, epub, kindle and other format this book has been release on 2016-04-05 with Mathematics categories.
Practical Guide to Logistic Regression covers the key points of the basic logistic regression model and illustrates how to use it properly to model a binary response variable. This powerful methodology can be used to analyze data from various fields, including medical and health outcomes research, business analytics and data science, ecology, fishe
Practical Guide To Logistic Regression
DOWNLOAD
Author : Adjunct Professor of Statistics School of Social and Family Dynamics Joseph M Hilbe
language : en
Publisher: CRC Press
Release Date : 2018-06-28
Practical Guide To Logistic Regression written by Adjunct Professor of Statistics School of Social and Family Dynamics Joseph M Hilbe and has been published by CRC Press this book supported file pdf, txt, epub, kindle and other format this book has been release on 2018-06-28 with categories.
Practical Guide to Logistic Regression covers the key points of the basic logistic regression model and illustrates how to use it properly to model a binary response variable. This powerful methodology can be used to analyze data from various fields, including medical and health outcomes research, business analytics and data science, ecology, fisheries, astronomy, transportation, insurance, economics, recreation, and sports. By harnessing the capabilities of the logistic model, analysts can better understand their data, make appropriate predictions and classifications, and determine the odds of one value of a predictor compared to another. Drawing on his many years of teaching logistic regression, using logistic-based models in research, and writing about the subject, Professor Hilbe focuses on the most important features of the logistic model. Serving as a guide between the author and readers, the book explains how to construct a logistic model, interpret coefficients and odds ratios, predict probabilities and their standard errors based on the model, and evaluate the model as to its fit. Using a variety of real data examples, mostly from health outcomes, the author offers a basic step-by-step guide to developing and interpreting observation and grouped logistic models as well as penalized and exact logistic regression. He also gives a step-by-step guide to modeling Bayesian logistic regression. R statistical software is used throughout the book to display the statistical models while SAS and Stata codes for all examples are included at the end of each chapter. The example code can be adapted to readers� own analyses. All the code is available on the author�s website.
Modern Regression Techniques Using R
DOWNLOAD
Author : Daniel B Wright
language : en
Publisher: SAGE
Release Date : 2009-03-05
Modern Regression Techniques Using R written by Daniel B Wright and has been published by SAGE this book supported file pdf, txt, epub, kindle and other format this book has been release on 2009-03-05 with Mathematics categories.
In a way that is refreshingly engaging and readable, Daniel B. Wright and Kamala London describe the most useful of these techniques and provide step-by-step instructions, using the freeware R, to analyze datasets that can be located on the books’ webpage via the SAGE homepage. Techniques covered in this book include multilevel modeling, ANOVA and ANCOVA, path analysis, mediation and moderation, logistic regression (generalized linear models), generalized additive models, and robust methods. These are all tested using a range of real research examples conducted by the authors in every chapter.
Best Practices In Logistic Regression
DOWNLOAD
Author : Jason W. Osborne
language : en
Publisher: SAGE Publications
Release Date : 2014-02-26
Best Practices In Logistic Regression written by Jason W. Osborne and has been published by SAGE Publications this book supported file pdf, txt, epub, kindle and other format this book has been release on 2014-02-26 with Social Science categories.
Jason W. Osborne’s Best Practices in Logistic Regression provides students with an accessible, applied approach that communicates logistic regression in clear and concise terms. The book effectively leverages readers’ basic intuitive understanding of simple and multiple regression to guide them into a sophisticated mastery of logistic regression. Osborne’s applied approach offers students and instructors a clear perspective, elucidated through practical and engaging tools that encourage student comprehension. Best Practices in Logistic Regression explains logistic regression in a concise and simple manner that gives students the clarity they need without the extra weight of longer, high-level texts.
Survival Analysis Using Sas
DOWNLOAD
Author : Paul D. Allison
language : en
Publisher: SAS Institute
Release Date : 2010-03-29
Survival Analysis Using Sas written by Paul D. Allison and has been published by SAS Institute this book supported file pdf, txt, epub, kindle and other format this book has been release on 2010-03-29 with Computers categories.
Easy to read and comprehensive, Survival Analysis Using SAS: A Practical Guide, Second Edition, by Paul D. Allison, is an accessible, data-based introduction to methods of survival analysis. Researchers who want to analyze survival data with SAS will find just what they need with this fully updated new edition that incorporates the many enhancements in SAS procedures for survival analysis in SAS 9. Although the book assumes only a minimal knowledge of SAS, more experienced users will learn new techniques of data input and manipulation. Numerous examples of SAS code and output make this an eminently practical book, ensuring that even the uninitiated become sophisticated users of survival analysis. The main topics presented include censoring, survival curves, Kaplan-Meier estimation, accelerated failure time models, Cox regression models, and discrete-time analysis. Also included are topics not usually covered in survival analysis books, such as time-dependent covariates, competing risks, and repeated events. Survival Analysis Using SAS: A Practical Guide, Second Edition, has been thoroughly updated for SAS 9, and all figures are presented using ODS Graphics. This new edition also documents major enhancements to the STRATA statement in the LIFETEST procedure; includes a section on the PROBPLOT command, which offers graphical methods to evaluate the fit of each parametric regression model; introduces the new BAYES statement for both parametric and Cox models, which allows the user to do a Bayesian analysis using MCMC methods; demonstrates the use of the counting process syntax as an alternative method for handling time-dependent covariates; contains a section on cumulative incidence functions; and describes the use of the new GLIMMIX procedure to estimate random-effects models for discrete-time data. This book is part of the SAS Press program.
Machine Learning Essentials
DOWNLOAD
Author : Alboukadel Kassambara
language : en
Publisher: STHDA
Release Date : 2018-03-10
Machine Learning Essentials written by Alboukadel Kassambara and has been published by STHDA this book supported file pdf, txt, epub, kindle and other format this book has been release on 2018-03-10 with categories.
Discovering knowledge from big multivariate data, recorded every days, requires specialized machine learning techniques. This book presents an easy to use practical guide in R to compute the most popular machine learning methods for exploring real word data sets, as well as, for building predictive models. The main parts of the book include: A) Unsupervised learning methods, to explore and discover knowledge from a large multivariate data set using clustering and principal component methods. You will learn hierarchical clustering, k-means, principal component analysis and correspondence analysis methods. B) Regression analysis, to predict a quantitative outcome value using linear regression and non-linear regression strategies. C) Classification techniques, to predict a qualitative outcome value using logistic regression, discriminant analysis, naive bayes classifier and support vector machines. D) Advanced machine learning methods, to build robust regression and classification models using k-nearest neighbors methods, decision tree models, ensemble methods (bagging, random forest and boosting). E) Model selection methods, to select automatically the best combination of predictor variables for building an optimal predictive model. These include, best subsets selection methods, stepwise regression and penalized regression (ridge, lasso and elastic net regression models). We also present principal component-based regression methods, which are useful when the data contain multiple correlated predictor variables. F) Model validation and evaluation techniques for measuring the performance of a predictive model. G) Model diagnostics for detecting and fixing a potential problems in a predictive model. The book presents the basic principles of these tasks and provide many examples in R. This book offers solid guidance in data mining for students and researchers. Key features: - Covers machine learning algorithm and implementation - Key mathematical concepts are presented - Short, self-contained chapters with practical examples.
Multivariable Analysis
DOWNLOAD
Author : Mitchell H. Katz
language : en
Publisher: Cambridge University Press
Release Date : 2006-02-06
Multivariable Analysis written by Mitchell H. Katz and has been published by Cambridge University Press this book supported file pdf, txt, epub, kindle and other format this book has been release on 2006-02-06 with Mathematics categories.
How to perform and interpret multivariable analysis, using plain language rather than complex derivations.
Understanding And Using Advanced Statistics
DOWNLOAD
Author : Jeremy J Foster
language : en
Publisher: SAGE
Release Date : 2006
Understanding And Using Advanced Statistics written by Jeremy J Foster and has been published by SAGE this book supported file pdf, txt, epub, kindle and other format this book has been release on 2006 with Social Science categories.
The spread of sophisticated computer packages and the machinery on which to run them has meant that procedures which were previously only available to experienced researchers with access to expensive machines and research students can now be carried out in a few seconds by almost every undergraduate. Understanding and Using Advanced Statistics provides the basis for gaining an understanding of what these analytic procedures do, when they should be used, and what the results provided signify. This comprehensive textbook guides students and researchers through the transition from simple statistics to more complex procedures with accessible language and illustration.
Logistic Regression Using Sas
DOWNLOAD
Author : Paul D. Allison
language : en
Publisher: SAS Institute
Release Date : 2012-03-30
Logistic Regression Using Sas written by Paul D. Allison and has been published by SAS Institute this book supported file pdf, txt, epub, kindle and other format this book has been release on 2012-03-30 with Computers categories.
If you are a researcher or student with experience in multiple linear regression and want to learn about logistic regression, Paul Allison's Logistic Regression Using SAS: Theory and Application, Second Edition, is for you! Informal and nontechnical, this book both explains the theory behind logistic regression, and looks at all the practical details involved in its implementation using SAS. Several real-world examples are included in full detail. This book also explains the differences and similarities among the many generalizations of the logistic regression model. The following topics are covered: binary logistic regression, logit analysis of contingency tables, multinomial logit analysis, ordered logit analysis, discrete-choice analysis, and Poisson regression. Other highlights include discussions on how to use the GENMOD procedure to do loglinear analysis and GEE estimation for longitudinal binary data. Only basic knowledge of the SAS DATA step is assumed. The second edition describes many new features of PROC LOGISTIC, including conditional logistic regression, exact logistic regression, generalized logit models, ROC curves, the ODDSRATIO statement (for analyzing interactions), and the EFFECTPLOT statement (for graphing nonlinear effects). Also new is coverage of PROC SURVEYLOGISTIC (for complex samples), PROC GLIMMIX (for generalized linear mixed models), PROC QLIM (for selection models and heterogeneous logit models), and PROC MDC (for advanced discrete choice models). This book is part of the SAS Press program.
Applied Mixed Model Analysis
DOWNLOAD
Author : Jos W. R. Twisk
language : en
Publisher: Cambridge University Press
Release Date : 2019-04-18
Applied Mixed Model Analysis written by Jos W. R. Twisk and has been published by Cambridge University Press this book supported file pdf, txt, epub, kindle and other format this book has been release on 2019-04-18 with Mathematics categories.
Emphasizing interpretation of results, this hands-on guide explains why, when, and how to use mixed models with your data.