Preference Learning
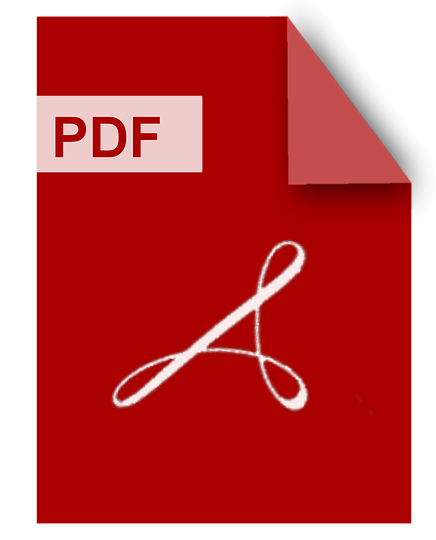
DOWNLOAD
Download Preference Learning PDF/ePub or read online books in Mobi eBooks. Click Download or Read Online button to get Preference Learning book now. This website allows unlimited access to, at the time of writing, more than 1.5 million titles, including hundreds of thousands of titles in various foreign languages. If the content not found or just blank you must refresh this page
Preference Learning
DOWNLOAD
Author : Johannes Fürnkranz
language : en
Publisher: Springer Science & Business Media
Release Date : 2010-11-19
Preference Learning written by Johannes Fürnkranz and has been published by Springer Science & Business Media this book supported file pdf, txt, epub, kindle and other format this book has been release on 2010-11-19 with Computers categories.
The topic of preferences is a new branch of machine learning and data mining, and it has attracted considerable attention in artificial intelligence research in previous years. It involves learning from observations that reveal information about the preferences of an individual or a class of individuals. Representing and processing knowledge in terms of preferences is appealing as it allows one to specify desires in a declarative way, to combine qualitative and quantitative modes of reasoning, and to deal with inconsistencies and exceptions in a flexible manner. And, generalizing beyond training data, models thus learned may be used for preference prediction. This is the first book dedicated to this topic, and the treatment is comprehensive. The editors first offer a thorough introduction, including a systematic categorization according to learning task and learning technique, along with a unified notation. The first half of the book is organized into parts on label ranking, instance ranking, and object ranking; while the second half is organized into parts on applications of preference learning in multiattribute domains, information retrieval, and recommender systems. The book will be of interest to researchers and practitioners in artificial intelligence, in particular machine learning and data mining, and in fields such as multicriteria decision-making and operations research.
Preference Learning
DOWNLOAD
Author : Johannes F Rnkranz
language : en
Publisher: Springer
Release Date : 2011-03-30
Preference Learning written by Johannes F Rnkranz and has been published by Springer this book supported file pdf, txt, epub, kindle and other format this book has been release on 2011-03-30 with Machine learning categories.
Special Issue Preference Learning And Ranking
DOWNLOAD
Author : Eyke Hüllermeier
language : en
Publisher:
Release Date : 2013
Special Issue Preference Learning And Ranking written by Eyke Hüllermeier and has been published by this book supported file pdf, txt, epub, kindle and other format this book has been release on 2013 with categories.
Individual Preference Learning With Collaborative Learning Framework
DOWNLOAD
Author : Zhu, Xi
language : en
Publisher:
Release Date : 2020
Individual Preference Learning With Collaborative Learning Framework written by Zhu, Xi and has been published by this book supported file pdf, txt, epub, kindle and other format this book has been release on 2020 with categories.
Smart, personal devices that interact with individuals make it possible to trigger desired behavioral changes with personalized incentives. Personalized incentives are the incentives that suit an individual's preferences. In this dissertation, individual preferences refer to a set of parameters describing how the individual values each influential factor in a travel alternative. To trigger behavioral changes with personalized incentives, a model that can accurately and efficiently estimate an individual's preferences from his behavior data is required. Two challenges exist in individual preference learning. For the first, the number of observations available from each individual for individual preference learning is limited. This issue causes difficulties in preference updating. For the second, the observability of the choices made is limited. This is because that it is not possible to directly observe the preference parameters -- the only information that can be observed is an individual's choice-making behavior. The two challenges prevent the use of traditional preference-learning techniques such as advanced econometric models (e.g., discrete choice models) derived from Random Utility Maximization (RUM).Other techniques such as machine learning also cannot be applied for similar reasons. New methods are needed for individual preference learning. This dissertation contributes to the existing literature in travel behavior studies by proposing individual preference learning methods such that personalized incentives could be accurately estimated to trigger behavioral changes, and proposing a design of an online experiment to collect travel behavior data. Specifically, two research questions are of interest: (1) What methodology could be used to learn an individual's preferences with only a few observations of choices made by him? (2) How to collect individuals' choice data to test the method proposed in the dissertation in terms of triggering individual behavioral changes with personalized incentives? In the dissertation, the behavior data is collected via a carefully designed online experiment utilizing the AMT (Amazon Mechanical Turk) platform. Considering the validity and reliability of the data, the dissertation contributes to the travel behavioral study in: (1) a full factorial design of a randomized experiment with two factors (commuting time and work flexibility, each with three levels) utilizing the online platform of AMT (Amazon Mechanical Turk) to collect individuals' travel choices on departure time in a sequence of hypothetical scenarios, and (2) a design of data quality control strategies, which refers to the design of some methods to reduce and identify the low-quality data collected in the experiment. These data quality control methods, such as understanding check, response consistency check, responding time record, and social desirability scale, can be applied to other online experiments and behavioral studies. To learn an individual's preference from a few choices made by him, a model structure that integrates a time-varying model and the collaborative learning model is proposed in the dissertation. The time-varying model is used to replace the original constant preference parameter to a time-dependent function, allowing an individual's preferences to fluctuate in his choice-making process. The collaborative learning model can exploit the underlying canonical structure of individuals' preference variation in a heterogeneous population. Specifically, the collaborative learning model could identify several patterns of preference changes (known as "canonical models") that exist in the population. With the canonical models, each individual's preference change can be expressed by a linear combination of all those canonical models. Considering the model's computation time, an online updating strategy for the proposed model is also proposed, such that individual preferences could be learned accurately and efficiently. Detailed specifications of two different formulations of the time-varying model are presented in the dissertation, with some explorations on model properties with simulations. The models are also applied to the real-world dataset collected in the online experiment. Results show that the proposed models can achieve higher accuracy in parameter learning and behavioral prediction than traditional preference learning models such as the logit model and the mixed logit model.
Adaptive Preference Learning With Bandit Feedback
DOWNLOAD
Author : Bangrui Chen
language : en
Publisher:
Release Date : 2017
Adaptive Preference Learning With Bandit Feedback written by Bangrui Chen and has been published by this book supported file pdf, txt, epub, kindle and other format this book has been release on 2017 with categories.
In this thesis, we study adaptive preference learning, in which a machine learning system learns users' preferences from feedback while simultaneously using these learned preferences to help them find preferred items. We study three different types of user feedback in three application setting: cardinal feedback with application in information filtering systems, ordinal feedback with application in personalized content recommender systems, and attribute feedback with application in review aggregators. We connect these settings respectively to existing work on classical multi-armed bandits, dueling bandits, and incentivizing exploration. For each type of feedback and application setting, we provide an algorithm and a theoretical analysis bounding its regret. We demonstrate through numerical experiments that our algorithms outperform existing benchmarks.
Efficient Preference Learning For Ai Powered Group Decision Making
DOWNLOAD
Author : Zhibing Zhao
language : en
Publisher:
Release Date : 2020
Efficient Preference Learning For Ai Powered Group Decision Making written by Zhibing Zhao and has been published by this book supported file pdf, txt, epub, kindle and other format this book has been release on 2020 with categories.
Learning Preference Skills
DOWNLOAD
Author : Jennifer Barnes
language : en
Publisher: Hyperion Books
Release Date : 1992
Learning Preference Skills written by Jennifer Barnes and has been published by Hyperion Books this book supported file pdf, txt, epub, kindle and other format this book has been release on 1992 with Cognitive styles categories.
Robust Preference Learning Based Reinforcement Learning
DOWNLOAD
Author : Riad Akrour
language : en
Publisher:
Release Date : 2014
Robust Preference Learning Based Reinforcement Learning written by Riad Akrour and has been published by this book supported file pdf, txt, epub, kindle and other format this book has been release on 2014 with categories.
The thesis contributions resolves around sequential decision taking and more precisely Reinforcement Learning (RL). Taking its root in Machine Learning in the same way as supervised and unsupervised learning, RL quickly grow in popularity within the last two decades due to a handful of achievements on both the theoretical and applicative front. RL supposes that the learning agent and its environment follow a stochastic Markovian decision process over a state and action space. The process is said of decision as the agent is asked to choose at each time step an action to take. It is said stochastic as the effect of selecting a given action in a given state does not systematically yield the same state but rather defines a distribution over the state space. It is said to be Markovian as this distribution only depends on the current state-action pair. Consequently to the choice of an action, the agent receives a reward. The RL goal is then to solve the underlying optimization problem of finding the behaviour that maximizes the sum of rewards all along the interaction of the agent with its environment. From an applicative point of view, a large spectrum of problems can be cast onto an RL one, from Backgammon (TD-Gammon, was one of Machine Learning first success giving rise to a world class player of advanced level) to decision problems in the industrial and medical world. However, the optimization problem solved by RL depends on the prevous definition of a reward function that requires a certain level of domain expertise and also knowledge of the internal quirks of RL algorithms. As such, the first contribution of the thesis was to propose a learning framework that lightens the requirements made to the user. The latter does not need anymore to know the exact solution of the problem but to only be able to choose between two behaviours exhibited by the agent, the one that matches more closely the solution. Learning is interactive between the agent and the user and resolves around the three main following points: i) The agent demonstrates a behaviour ii) The user compares it w.r.t. to the current best one iii) The agent uses this feedback to update its preference model of the user and uses it to find the next behaviour to demonstrate. To reduce the number of required interactions before finding the optimal behaviour, the second contribution of the thesis was to define a theoretically sound criterion making the trade-off between the sometimes contradicting desires of complying with the user's preferences and demonstrating sufficiently different behaviours. The last contribution was to ensure the robustness of the algorithm w.r.t. the feedback errors that the user might make. Which happens more often than not in practice, especially at the initial phase of the interaction, when all the behaviours are far from the expected solution.
Towards Long Short Term Preference Learning Fairness Aware And Conversational Recommender Systems With Graph Neural Networks
DOWNLOAD
Author : 許丞
language : en
Publisher:
Release Date : 2020
Towards Long Short Term Preference Learning Fairness Aware And Conversational Recommender Systems With Graph Neural Networks written by 許丞 and has been published by this book supported file pdf, txt, epub, kindle and other format this book has been release on 2020 with categories.
Active Preference Learning Using Trajectory Segmentation
DOWNLOAD
Author : Monica Patel
language : en
Publisher:
Release Date : 2019
Active Preference Learning Using Trajectory Segmentation written by Monica Patel and has been published by this book supported file pdf, txt, epub, kindle and other format this book has been release on 2019 with categories.
"Machine learning is currently used heavily to develop robot behaviors, giving great flexibility and power, but there remains significant burden on human designers to specify reward functions or label data for the learning target. Learning from demonstration is a very intuitive way to teach your robot a new skill. This thesis considers two improvements to modern learning from demonstrations. First, we consider a model-based imitation approach that utilizes a modern form of deep probabilistic model to predict agent behaviors in order to match them to demonstrations. We replace the non-parametric estimator utilized by an existing approach, which has the limitation of poor scaling with the amount of training data. In its place, a learned parametric model is trained to capture inherent uncertainties. Through sampling-based prediction, our approach is able to capture a distribution over likely outcomes of the given policy. This is paired with a probabilistic notion of the difference between the agent's outcome distribution and the distribution of demonstrations, to produce a gradient-based policy improvement approach. Our results show that this method is effective in imitating demonstrations in a range of scenarios.The second portion of this thesis considers the \textit{temporal credit assignment} problem within learning from demonstration. We propose an active learning framework that uses trajectory segmentation to addresses this issue. Our method uses spatiotemporal criteria to segment the trajectory. These criteria can be based upon speed, heading, or curve of the trajectory which are intuitive properties to understand user's intentions. Thus, not only does our framework make the user query interface more intuitive but the resulting approach also learns faster. We demonstrate and evaluate our approach by learning a reward function for various driving scenarios and show that our algorithm converges faster"--