Probabilistic Graphical Models For Protein Structure Prediction
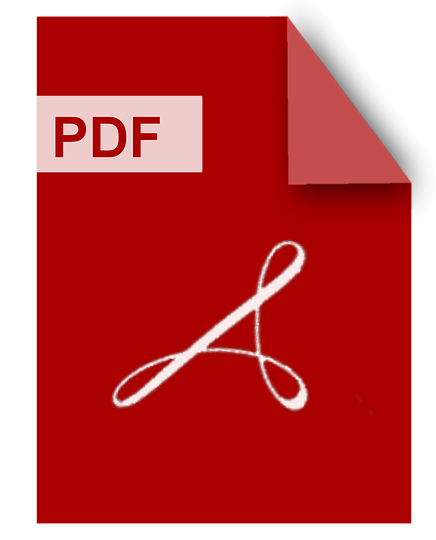
DOWNLOAD
Download Probabilistic Graphical Models For Protein Structure Prediction PDF/ePub or read online books in Mobi eBooks. Click Download or Read Online button to get Probabilistic Graphical Models For Protein Structure Prediction book now. This website allows unlimited access to, at the time of writing, more than 1.5 million titles, including hundreds of thousands of titles in various foreign languages. If the content not found or just blank you must refresh this page
Probabilistic Graphical Models For Protein Structure Prediction
DOWNLOAD
Author : Debswapna Bhattacharya
language : en
Publisher:
Release Date : 2016
Probabilistic Graphical Models For Protein Structure Prediction written by Debswapna Bhattacharya and has been published by this book supported file pdf, txt, epub, kindle and other format this book has been release on 2016 with categories.
Computationally predicting the folded and functional three-dimensional structure of a protein molecule from its amino acid sequence with high degree of accuracy is critically important in structural bioinformatics and has huge implications in understanding and curing numerous diseases caused by protein misfolding, including CJD and type II diabetes, as well as neurodegenerative diseases like Alzheimer's, Parkinson's, Huntington's, and amyotrophic lateral sclerosis. Existing computational approaches for protein structure prediction faces two key challenges: (1) difficulty in efficiently navigating the enormous conformational space accessible to proteins and (2) difficulty in accurately capturing energetics of the complex interactions of thousand of atoms in a protein molecule in silico. This dissertation attempts to address these challenges by (1) developing novel probabilistic graphical models and experimentally motivated probabilistic sampling techniques to fully capture and efficiently explore proteins' conformational space in various granularities and (2) integrating knowledge-based information into existing energy functions in order to improve their ability to discriminate correctly folded protein structures from decoys. We show that our methods outperform many traditional and state-of-the-art protein structure prediction approaches in terms of accuracy, speed and robustness. All of these methods are freely available to the scientific community.
Probabilistic Graphical Models And Algorithms For Protein Problems
DOWNLOAD
Author : Feng Jiao
language : en
Publisher:
Release Date : 2008
Probabilistic Graphical Models And Algorithms For Protein Problems written by Feng Jiao and has been published by this book supported file pdf, txt, epub, kindle and other format this book has been release on 2008 with categories.
In this thesis I present research in two fields: machine learning and computational biology. First, I develop new machine learning methods for graphical models that can be applied to protein problems. Then I apply graphical model algorithms to protein problems, obtaining improvements in protein structure prediction and protein structure alignment. First, in the machine learning work, I focus on a special kind of graphical model---conditional random fields (CRFs). Here, I present a new semi-supervised training procedure for CRFs that can be used to train sequence segmentors and labellers from a combination of labeled and unlabeled training data. Such learning algorithms can be applied to protein and gene name entity recognition problems. This work provides one of the first semi-supervised discriminative training methods for structured classification. Second, in my computational biology work, I focus mainly on protein problems. In particular, I first propose a tree decomposition method for solving the protein structure prediction and protein structure alignment problems. In so doing, I reveal why tree decomposition is a good method for many protein problems. Then, I propose a computational framework for detection of similar structures of a target protein with sparse NMR data, which can help to predict protein structure using experimental data. Finally, I propose a new machine learning approach---LS_Boost---to solve the protein fold recognition problem, which is one of the key steps in protein structure prediction. After a thorough comparison, the algorithm is proved to be both more accurate and more efficient than traditional z-Score method and other machine learning methods.
Probabilistic Graphical Models And Algorithms For
DOWNLOAD
Author : Feng Jiao
language : en
Publisher:
Release Date : 2008
Probabilistic Graphical Models And Algorithms For written by Feng Jiao and has been published by this book supported file pdf, txt, epub, kindle and other format this book has been release on 2008 with categories.
Bayesian Methods In Structural Bioinformatics
DOWNLOAD
Author : Thomas Hamelryck
language : en
Publisher: Springer
Release Date : 2012-03-23
Bayesian Methods In Structural Bioinformatics written by Thomas Hamelryck and has been published by Springer this book supported file pdf, txt, epub, kindle and other format this book has been release on 2012-03-23 with Medical categories.
This book is an edited volume, the goal of which is to provide an overview of the current state-of-the-art in statistical methods applied to problems in structural bioinformatics (and in particular protein structure prediction, simulation, experimental structure determination and analysis). It focuses on statistical methods that have a clear interpretation in the framework of statistical physics, rather than ad hoc, black box methods based on neural networks or support vector machines. In addition, the emphasis is on methods that deal with biomolecular structure in atomic detail. The book is highly accessible, and only assumes background knowledge on protein structure, with a minimum of mathematical knowledge. Therefore, the book includes introductory chapters that contain a solid introduction to key topics such as Bayesian statistics and concepts in machine learning and statistical physics.
Conditional Graphical Models For Protein Structure Prediction
DOWNLOAD
Author : Yan Liu
language : en
Publisher:
Release Date : 2007
Conditional Graphical Models For Protein Structure Prediction written by Yan Liu and has been published by this book supported file pdf, txt, epub, kindle and other format this book has been release on 2007 with Amino acid sequence categories.
Probabilistic Graphical Models
DOWNLOAD
Author : Daphne Koller
language : en
Publisher: MIT Press
Release Date : 2009-07-31
Probabilistic Graphical Models written by Daphne Koller and has been published by MIT Press this book supported file pdf, txt, epub, kindle and other format this book has been release on 2009-07-31 with Computers categories.
A general framework for constructing and using probabilistic models of complex systems that would enable a computer to use available information for making decisions. Most tasks require a person or an automated system to reason—to reach conclusions based on available information. The framework of probabilistic graphical models, presented in this book, provides a general approach for this task. The approach is model-based, allowing interpretable models to be constructed and then manipulated by reasoning algorithms. These models can also be learned automatically from data, allowing the approach to be used in cases where manually constructing a model is difficult or even impossible. Because uncertainty is an inescapable aspect of most real-world applications, the book focuses on probabilistic models, which make the uncertainty explicit and provide models that are more faithful to reality. Probabilistic Graphical Models discusses a variety of models, spanning Bayesian networks, undirected Markov networks, discrete and continuous models, and extensions to deal with dynamical systems and relational data. For each class of models, the text describes the three fundamental cornerstones: representation, inference, and learning, presenting both basic concepts and advanced techniques. Finally, the book considers the use of the proposed framework for causal reasoning and decision making under uncertainty. The main text in each chapter provides the detailed technical development of the key ideas. Most chapters also include boxes with additional material: skill boxes, which describe techniques; case study boxes, which discuss empirical cases related to the approach described in the text, including applications in computer vision, robotics, natural language understanding, and computational biology; and concept boxes, which present significant concepts drawn from the material in the chapter. Instructors (and readers) can group chapters in various combinations, from core topics to more technically advanced material, to suit their particular needs.
Protein Structure Prediction
DOWNLOAD
Author : Igor F. Tsigelny
language : en
Publisher: Internat'l University Line
Release Date : 2002
Protein Structure Prediction written by Igor F. Tsigelny and has been published by Internat'l University Line this book supported file pdf, txt, epub, kindle and other format this book has been release on 2002 with Science categories.
Modeling Of Proteins And Protein Protein Interactions With Probabilistic Graphical Models
DOWNLOAD
Author : Bornika Ghosh
language : en
Publisher:
Release Date : 2011
Modeling Of Proteins And Protein Protein Interactions With Probabilistic Graphical Models written by Bornika Ghosh and has been published by this book supported file pdf, txt, epub, kindle and other format this book has been release on 2011 with Graphical modeling (Statistics) categories.
Introduction To Protein Structure Prediction
DOWNLOAD
Author : Huzefa Rangwala
language : en
Publisher: John Wiley & Sons
Release Date : 2011-03-16
Introduction To Protein Structure Prediction written by Huzefa Rangwala and has been published by John Wiley & Sons this book supported file pdf, txt, epub, kindle and other format this book has been release on 2011-03-16 with Science categories.
A look at the methods and algorithms used to predict protein structure A thorough knowledge of the function and structure of proteins is critical for the advancement of biology and the life sciences as well as the development of better drugs, higher-yield crops, and even synthetic bio-fuels. To that end, this reference sheds light on the methods used for protein structure prediction and reveals the key applications of modeled structures. This indispensable book covers the applications of modeled protein structures and unravels the relationship between pure sequence information and three-dimensional structure, which continues to be one of the greatest challenges in molecular biology. With this resource, readers will find an all-encompassing examination of the problems, methods, tools, servers, databases, and applications of protein structure prediction and they will acquire unique insight into the future applications of the modeled protein structures. The book begins with a thorough introduction to the protein structure prediction problem and is divided into four themes: a background on structure prediction, the prediction of structural elements, tertiary structure prediction, and functional insights. Within those four sections, the following topics are covered: Databases and resources that are commonly used for protein structure prediction The structure prediction flagship assessment (CASP) and the protein structure initiative (PSI) Definitions of recurring substructures and the computational approaches used for solving sequence problems Difficulties with contact map prediction and how sophisticated machine learning methods can solve those problems Structure prediction methods that rely on homology modeling, threading, and fragment assembly Hybrid methods that achieve high-resolution protein structures Parts of the protein structure that may be conserved and used to interact with other biomolecules How the loop prediction problem can be used for refinement of the modeled structures The computational model that detects the differences between protein structure and its modeled mutant Whether working in the field of bioinformatics or molecular biology research or taking courses in protein modeling, readers will find the content in this book invaluable.
Protein Conformational Dynamics
DOWNLOAD
Author : Ke-li Han
language : en
Publisher: Springer Science & Business Media
Release Date : 2014-01-20
Protein Conformational Dynamics written by Ke-li Han and has been published by Springer Science & Business Media this book supported file pdf, txt, epub, kindle and other format this book has been release on 2014-01-20 with Medical categories.
This book discusses how biological molecules exert their function and regulate biological processes, with a clear focus on how conformational dynamics of proteins are critical in this respect. In the last decade, the advancements in computational biology, nuclear magnetic resonance including paramagnetic relaxation enhancement, and fluorescence-based ensemble/single-molecule techniques have shown that biological molecules (proteins, DNAs and RNAs) fluctuate under equilibrium conditions. The conformational and energetic spaces that these fluctuations explore likely contain active conformations that are critical for their function. More interestingly, these fluctuations can respond actively to external cues, which introduces layers of tight regulation on the biological processes that they dictate. A growing number of studies have suggested that conformational dynamics of proteins govern their role in regulating biological functions, examples of this regulation can be found in signal transduction, molecular recognition, apoptosis, protein / ion / other molecules translocation and gene expression. On the experimental side, the technical advances have offered deep insights into the conformational motions of a number of proteins. These studies greatly enrich our knowledge of the interplay between structure and function. On the theoretical side, novel approaches and detailed computational simulations have provided powerful tools in the study of enzyme catalysis, protein / drug design, protein / ion / other molecule translocation and protein folding/aggregation, to name but a few. This work contains detailed information, not only on the conformational motions of biological systems, but also on the potential governing forces of conformational dynamics (transient interactions, chemical and physical origins, thermodynamic properties). New developments in computational simulations will greatly enhance our understanding of how these molecules function in various biological events.