Robust Model Selection And Outlier Detection In Linear Regressions
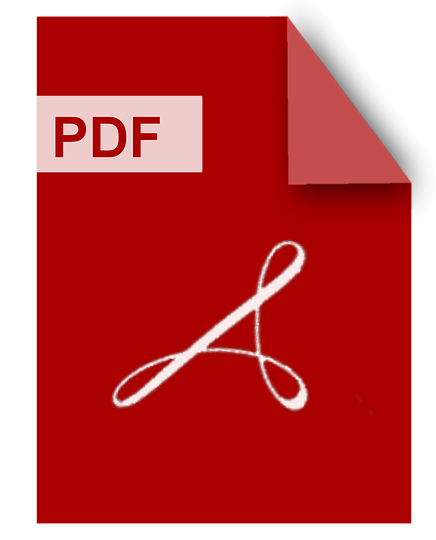
DOWNLOAD
Download Robust Model Selection And Outlier Detection In Linear Regressions PDF/ePub or read online books in Mobi eBooks. Click Download or Read Online button to get Robust Model Selection And Outlier Detection In Linear Regressions book now. This website allows unlimited access to, at the time of writing, more than 1.5 million titles, including hundreds of thousands of titles in various foreign languages. If the content not found or just blank you must refresh this page
Robust Model Selection And Outlier Detection In Linear Regressions
DOWNLOAD
Author : Lauren McCann (Ph. D.)
language : en
Publisher:
Release Date : 2006
Robust Model Selection And Outlier Detection In Linear Regressions written by Lauren McCann (Ph. D.) and has been published by this book supported file pdf, txt, epub, kindle and other format this book has been release on 2006 with categories.
(cont.) Finally, we discuss the problem of outlier detection. In addition to model selection, outliers can adversely influence many other outcomes of regression-based data analysis. We describe a new outlier diagnostic tool, which we call diagnostic data traces. This tool can be used to detect outliers and study their influence on a variety of regression statistics. We demonstrate our tool on several data sets, which are considered benchmarks in the field of outlier detection.
Robust Regression And Outlier Detection
DOWNLOAD
Author : Peter J. Rousseeuw
language : en
Publisher: John Wiley & Sons
Release Date : 2005-02-25
Robust Regression And Outlier Detection written by Peter J. Rousseeuw and has been published by John Wiley & Sons this book supported file pdf, txt, epub, kindle and other format this book has been release on 2005-02-25 with Mathematics categories.
WILEY-INTERSCIENCE PAPERBACK SERIES The Wiley-Interscience Paperback Series consists of selectedbooks that have been made more accessible to consumers in an effortto increase global appeal and general circulation. With these newunabridged softcover volumes, Wiley hopes to extend the lives ofthese works by making them available to future generations ofstatisticians, mathematicians, and scientists. "The writing style is clear and informal, and much of thediscussion is oriented to application. In short, the book is akeeper." –Mathematical Geology "I would highly recommend the addition of this book to thelibraries of both students and professionals. It is a usefultextbook for the graduate student, because it emphasizes both thephilosophy and practice of robustness in regression settings, andit provides excellent examples of precise, logical proofs oftheorems. . . .Even for those who are familiar with robustness, thebook will be a good reference because it consolidates the researchin high-breakdown affine equivariant estimators and includes anextensive bibliography in robust regression, outlier diagnostics,and related methods. The aim of this book, the authors tell us, is‘to make robust regression available for everyday statisticalpractice.’ Rousseeuw and Leroy have included all of thenecessary ingredients to make this happen." –Journal of the American Statistical Association
Multiple Outliers In Linear Regression
DOWNLOAD
Author : James Walter Wisnowski
language : en
Publisher:
Release Date : 1999
Multiple Outliers In Linear Regression written by James Walter Wisnowski and has been published by this book supported file pdf, txt, epub, kindle and other format this book has been release on 1999 with Outliers (Statistics) categories.
Multiple Outliers In Linear Regression
DOWNLOAD
Author : James Walter Wisnowski
language : en
Publisher:
Release Date : 1999
Multiple Outliers In Linear Regression written by James Walter Wisnowski and has been published by this book supported file pdf, txt, epub, kindle and other format this book has been release on 1999 with Outliers (Statistics) categories.
Empirical evidence suggests unusual or outlying observations in data sets are much more prevalent than one might expect; 5 to 10% on average for many industries. This research addresses multiple outliers in the linear regression model. Although reliable for a single or a few outliers, standard diagnostic techniques from an ordinary least squares (OLS) fit can fail to identify multiple outliers. The parameter estimates, diagnostic quantities and model inferences from the contaminated data set can be significantly different from those obtained with the clean data. The researcher requires a dependable method to identify and accommodate these multiple outliers. This research tests both direct methods from algorithms and indirect methods from robust regression estimators to identify multiple outliers. A comprehensive Monte Carlo simulation study evaluates the impact that outlier density and geometry, regressor variable dimension, and outlying distance have on numerous published methods. The performance study focuses on outlier configurations likely to be encountered in practice and uses a designed experiment approach. The results for each scenario provide insight and limitations in performance for each technique. Recommendations are given for each technique. OLS is the optimal regression estimator under a set of assumptions on the distribution of the error term and predictor variables. Compound robust regression estimators have been proposed as alternatives when some OLS assumptions fail. Compound estimators can accommodate multiple outliers and limit the influence of the observations with remote levels of predictor variables. This research proposes a new compound estimator that is more effective for extreme observations in X space and high dimension than currently published methods. This research also addresses the variable selection problem for compound robust regression estimators.
Essays On Robust Model Selection And Model Averaging For Linear Models
DOWNLOAD
Author : Le Chang
language : en
Publisher:
Release Date : 2017
Essays On Robust Model Selection And Model Averaging For Linear Models written by Le Chang and has been published by this book supported file pdf, txt, epub, kindle and other format this book has been release on 2017 with categories.
Model selection is central to all applied statistical work. Selecting the variables for use in a regression model is one important example of model selection. This thesis is a collection of essays on robust model selection procedures and model averaging for linear regression models. In the first essay, we propose robust Akaike information criteria (AIC) for MM-estimation and an adjusted robust scale based AIC for M and MM-estimation. Our proposed model selection criteria can maintain their robust properties in the presence of a high proportion of outliers and the outliers in the covariates. We compare our proposed criteria with other robust model selection criteria discussed in previous literature. Our simulation studies demonstrate a significant outperformance of robust AIC based on MM-estimation in the presence of outliers in the covariates. The real data example also shows a better performance of robust AIC based on MM-estimation. The second essay focuses on robust versions of the "Least Absolute Shrinkage and Selection Operator" (lasso). The adaptive lasso is a method for performing simultaneous parameter estimation and variable selection. The adaptive weights used in its penalty term mean that the adaptive lasso achieves the oracle property. In this essay, we propose an extension of the adaptive lasso named the Tukey-lasso. By using Tukey's biweight criterion, instead of squared loss, the Tukey-lasso is resistant to outliers in both the response and covariates. Importantly, we demonstrate that the Tukey-lasso also enjoys the oracle property. A fast accelerated proximal gradient (APG) algorithm is proposed and implemented for computing the Tukey-lasso. Our extensive simulations show that the Tukey-lasso, implemented with the APG algorithm, achieves very reliable results, including for high-dimensional data where p>n. In the presence of outliers, the Tukey-lasso is shown to offer substantial improvements in performance compared to the adaptive lasso and other robust implementations of the lasso. Real data examples further demonstrate the utility of the Tukey-lasso. In many statistical analyses, a single model is used for statistical inference, ignoring the process that leads to the model being selected. To account for this model uncertainty, many model averaging procedures have been proposed. In the last essay, we propose an extension of a bootstrap model averaging approach, called bootstrap lasso averaging (BLA). BLA utilizes the lasso for model selection. This is in contrast to other forms of bootstrap model averaging that use AIC or Bayesian information criteria (BIC). The use of the lasso improves the computation speed and allows BLA to be applied even when the number of variables p is larger than the sample size n. Extensive simulations confirm that BLA has outstanding finite sample performance, in terms of both variable and prediction accuracies, compared with traditional model selection and model averaging methods. Several real data examples further demonstrate an improved out-of-sample predictive performance of BLA.
Contributions To Robust Regression Using The L Norm Criterion And Mixed Integer Linear Programming
DOWNLOAD
Author : Pieter Marthinus Du Plessis
language : en
Publisher:
Release Date : 2010
Contributions To Robust Regression Using The L Norm Criterion And Mixed Integer Linear Programming written by Pieter Marthinus Du Plessis and has been published by this book supported file pdf, txt, epub, kindle and other format this book has been release on 2010 with categories.
Model selection -- Variable selection -- Outlier detection -- Data screening -- Mixed integer linear programming model -- Integer programming -- Robust regression -- Model seleksie -- Veranderlike seleksie -- Uitskieters -- Gemengde heeltallige line?ere programmering -- Heeltallige programmering -- Robuuste regressie.
Regression And Time Series Model Selection
DOWNLOAD
Author : Allan D. R. McQuarrie
language : en
Publisher: World Scientific
Release Date : 1998
Regression And Time Series Model Selection written by Allan D. R. McQuarrie and has been published by World Scientific this book supported file pdf, txt, epub, kindle and other format this book has been release on 1998 with Mathematics categories.
This important book describes procedures for selecting a model from a large set of competing statistical models. It includes model selection techniques for univariate and multivariate regression models, univariate and multivariate autoregressive models, nonparametric (including wavelets) and semiparametric regression models, and quasi-likelihood and robust regression models. Information-based model selection criteria are discussed, and small sample and asymptotic properties are presented. The book also provides examples and large scale simulation studies comparing the performances of information-based model selection criteria, bootstrapping, and cross-validation selection methods over a wide range of models.
Basics Of Modern Mathematical Statistics
DOWNLOAD
Author : Vladimir Spokoiny
language : en
Publisher: Springer
Release Date : 2014-10-25
Basics Of Modern Mathematical Statistics written by Vladimir Spokoiny and has been published by Springer this book supported file pdf, txt, epub, kindle and other format this book has been release on 2014-10-25 with Mathematics categories.
This textbook provides a unified and self-contained presentation of the main approaches to and ideas of mathematical statistics. It collects the basic mathematical ideas and tools needed as a basis for more serious study or even independent research in statistics. The majority of existing textbooks in mathematical statistics follow the classical asymptotic framework. Yet, as modern statistics has changed rapidly in recent years, new methods and approaches have appeared. The emphasis is on finite sample behavior, large parameter dimensions, and model misspecifications. The present book provides a fully self-contained introduction to the world of modern mathematical statistics, collecting the basic knowledge, concepts and findings needed for doing further research in the modern theoretical and applied statistics. This textbook is primarily intended for graduate and postdoc students and young researchers who are interested in modern statistical methods.
Mixed Integer Linear Programming Robust Regression With Feature Selection
DOWNLOAD
Author : Oleksii Omelchenko
language : en
Publisher:
Release Date : 2014
Mixed Integer Linear Programming Robust Regression With Feature Selection written by Oleksii Omelchenko and has been published by this book supported file pdf, txt, epub, kindle and other format this book has been release on 2014 with categories.
We introduce a Mixed-Integer Linear Programming approach for building Regression models. These models can detect potential outliers and have a built-in Feature Selection technique. We demonstrate how to build a linear regression model as well as a multidimensional piece-wise linear regression model that can simulate non-linear models. We compare our techniques with the existing statistical approaches for building regression models with different feature selection algorithms by comparing the results of predictions for 3 real-world data sets. All experiments show that our approach is useful in case where the number of training instances is less than the number of predictors, more stable and provides better results than Stepwise regression, which is the most used linear regression technique in cases when we deal with too many features in the model while having fewer observations.
Applied Linear Regression
DOWNLOAD
Author : Sanford Weisberg
language : en
Publisher:
Release Date : 1985-08-14
Applied Linear Regression written by Sanford Weisberg and has been published by this book supported file pdf, txt, epub, kindle and other format this book has been release on 1985-08-14 with Mathematics categories.
Simple linear regression; Multiple regression; Drawing conclusions; Weighted least squares, testing for lack of fit, general F-tests, and confidence ellipsoids; Diagnostics I, residuals and influence; Diagnostics II, symptoms and remedies; Model building I, defining new predictors; Model building I, collinearity and variable selection; Prediction; Incomplete data; Contents; Nonleast squares estimation; Generalizations of linear regression.