Semantic Role Labeling
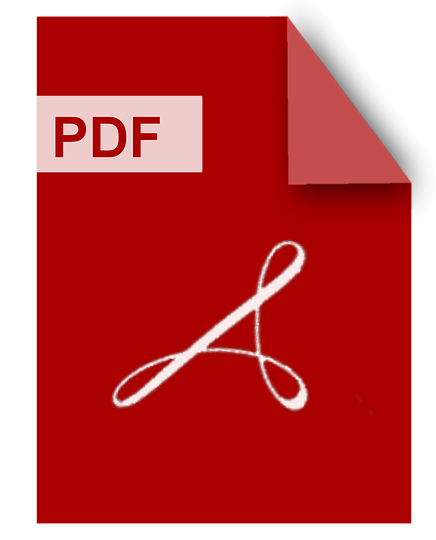
DOWNLOAD
Download Semantic Role Labeling PDF/ePub or read online books in Mobi eBooks. Click Download or Read Online button to get Semantic Role Labeling book now. This website allows unlimited access to, at the time of writing, more than 1.5 million titles, including hundreds of thousands of titles in various foreign languages. If the content not found or just blank you must refresh this page
Semantic Role Labeling
DOWNLOAD
Author : Martha Palmer
language : en
Publisher: Morgan & Claypool Publishers
Release Date : 2011-02-02
Semantic Role Labeling written by Martha Palmer and has been published by Morgan & Claypool Publishers this book supported file pdf, txt, epub, kindle and other format this book has been release on 2011-02-02 with Computers categories.
This book is aimed at providing an overview of several aspects of semantic role labeling. Chapter 1 begins with linguistic background on the definition of semantic roles and the controversies surrounding them. Chapter 2 describes how the theories have led to structured lexicons such as FrameNet, VerbNet and the PropBank Frame Files that in turn provide the basis for large scale semantic annotation of corpora. This data has facilitated the development of automatic semantic role labeling systems based on supervised machine learning techniques. Chapter 3 presents the general principles of applying both supervised and unsupervised machine learning to this task, with a description of the standard stages and feature choices, as well as giving details of several specific systems. Recent advances include the use of joint inference to take advantage of context sensitivities, and attempts to improve performance by closer integration of the syntactic parsing task with semantic role labeling. Chapter 3 also discusses the impact the granularity of the semantic roles has on system performance. Having outlined the basic approach with respect to English, Chapter 4 goes on to discuss applying the same techniques to other languages, using Chinese as the primary example. Although substantial training data is available for Chinese, this is not the case for many other languages, and techniques for projecting English role labels onto parallel corpora are also presented. Table of Contents: Preface / Semantic Roles / Available Lexical Resources / Machine Learning for Semantic Role Labeling / A Cross-Lingual Perspective / Summary
Semantic Features For Semantic Role Labeling
DOWNLOAD
Author : Liam R. McGrath
language : en
Publisher:
Release Date : 2011
Semantic Features For Semantic Role Labeling written by Liam R. McGrath and has been published by this book supported file pdf, txt, epub, kindle and other format this book has been release on 2011 with Semantics categories.
Robust Semantic Role Labeling
DOWNLOAD
Author : Yi Szu-Ting
language : en
Publisher: LAP Lambert Academic Publishing
Release Date : 2015-05-25
Robust Semantic Role Labeling written by Yi Szu-Ting and has been published by LAP Lambert Academic Publishing this book supported file pdf, txt, epub, kindle and other format this book has been release on 2015-05-25 with categories.
Correctly identifying semantic entities and successfully disambiguating the relations between them and their predicates is an important and necessary step for successful natural language processing applications, such as text summarization, question answering, and machine translation. Researchers have studied this problem, semantic role labeling (SRL), as a machine learning problem since 2000. However, after using an optimal global inference algorithm to combine several SRL systems, the growth of SRL performance seems to have reached a plateau. Syntactic parsing is the bottleneck of the task of semantic role labeling and robustness is the ultimate goal. In this book, we investigate ways to train a better syntactic parser and increase SRL system robustness. We demonstrate that parse trees augmented by semantic role markups can serve as suitable training data for training a parser for an SRL system. For system robustness, we propose that it is easier to learn a new set of semantic roles. The new roles are less verb- dependent than the original PropBank roles. As a result, the SRL system trained on the new roles achieves significantly better robustness.
Personal Knowledge Base Construction Using Semantic Role Labeling
DOWNLOAD
Author : 楊宗翰
language : en
Publisher:
Release Date : 2018
Personal Knowledge Base Construction Using Semantic Role Labeling written by 楊宗翰 and has been published by this book supported file pdf, txt, epub, kindle and other format this book has been release on 2018 with categories.
A Study On The Fundamentals Of Semantic Role Labeling
DOWNLOAD
Author : Tin Wing (Winona) Wu
language : en
Publisher:
Release Date : 2010
A Study On The Fundamentals Of Semantic Role Labeling written by Tin Wing (Winona) Wu and has been published by this book supported file pdf, txt, epub, kindle and other format this book has been release on 2010 with Computational linguistics categories.
The natural language processing (NLP) community has recently experienced a growing interest in semantic role labeling (SRL) - the process of assigning a WHO did WHAT to WHOM, WHEN, WHERE, WHY and HOW structure to text. The increased availability of annotated resources enables the development of statistical approaches specifically for SRL. This holds potential impact in NLP applications. In this project, we describe the linguistic background of the SRL problem, major resources that are used and an overview of general approaches in computational systems. We reproduce the approaches to SRL based on Pradhan's ASSERT system extending the work of Gildea and Jurafsky. We examine the system and its individual components, including its annotated resources, parser, classification system, and the features used. We then examine the results obtained by the system and its components. We also assess the challenges in SRL and identify the opportunities for useful further research in SRL.
Predicate Informed Syntax Guidance For Semantic Role Labeling
DOWNLOAD
Author : Sijia Wang
language : en
Publisher:
Release Date : 2020
Predicate Informed Syntax Guidance For Semantic Role Labeling written by Sijia Wang and has been published by this book supported file pdf, txt, epub, kindle and other format this book has been release on 2020 with Electronic dissertations categories.
In this thesis, we consider neural network approaches to the semantic role labeling task in seman-tic parsing. Recent state-of-the-art results for semantic role labeling are achieved by combiningLSTM neural networks and pre-trained features. This work offers a simple BERT-based modelwhich shows that, contrary to the popular belief that more complexity means better performance,removing LSTM improves the state of the art for span-based semantic role labeling. This modelhas improved F1 scores on both the test set of CoNLL-2012, and the Brown test set of CoNLL-2005 by at least 3 percentage points.In addition to this refinement of existing architectures, we also propose a new mechanism. Therehas been an active line of research focusing on incorporating syntax information into the atten-tion mechanism for semantic parsing. However, the existing models do not make use of whichsub-clause a given token belongs to or where the boundary of the sub-clause lies. In this thesis,we propose a predicate-aware attention mechanism that explicitly incorporates the portion of theparsing spanning from the predicate. The proposed Syntax-Guidance (SG) mechanism further improves the model performance. We compare the predicate informed method with three other SG mechanisms in detailed error analysis, showing the advantage and potential research directions ofthe proposed method.
Semantic Role Labeling Using Rich Morphological Features
DOWNLOAD
Author :
language : en
Publisher:
Release Date : 2013
Semantic Role Labeling Using Rich Morphological Features written by and has been published by this book supported file pdf, txt, epub, kindle and other format this book has been release on 2013 with categories.
Semantic Role Labeling Using Lexicalized Tree Adjoining Grammars
DOWNLOAD
Author : Yudong Liu
language : en
Publisher:
Release Date : 2009
Semantic Role Labeling Using Lexicalized Tree Adjoining Grammars written by Yudong Liu and has been published by this book supported file pdf, txt, epub, kindle and other format this book has been release on 2009 with Computational linguistics categories.
The predicate-argument structure (PAS) of a natural language sentence is a useful representation that can be used for a deeper analysis of the underlying meaning of the sentence or directly used in various natural language processing (NLP) applications. The task of semantic role labeling (SRL) is to identify the predicate-argument structures and label the relations between the predicate and each of its arguments. Researchers have been studying SRL as a machine learning problem in the past six years, after large-scale semantically annotated corpora such as FrameNet and PropBank were released to the research community. Lexicalized Tree Adjoining Grammars (LTAGs), a tree rewriting formalism, are often a convenient representation for capturing locality of predicate-argument relations. Our work in this thesis is focused on the development and learning of the state of the art discriminative SRL systems with LTAGs. Our contributions to this field include: We apply to the SRL task a variant of the LTAG formalism called LTAG-spinal and the associated LTAG-spinal Treebank (the formalism and the Treebank were created by Libin Shen). Predicate-argument relations that are either implicit or absent from the original Penn Treebank are made explicit and accessible in the LTAG-spinal Treebank, which we show to be a useful resource for SRL. We propose the use of the LTAGs as an important additional source of features for the SRL task. Our experiments show that, compared with the best-known set of features that are used in state of the art SRL systems, LTAG-based features can improve SRL performance significantly. We treat multiple LTAG derivation trees as latent features for SRL and introduce a novel learning framework -- Latent Support Vector Machines (LSVMs) to the SRL task using these latent features. This method significantly outperforms state of the art SRL systems. In addition, we adapt an SRL framework to a real-world ternary relation extraction task in the biomedical domain. Our experiments show that the use of SRL related features significantly improves performance over the system using only shallow word-based features.
Verbose Labels For Semantic Roles
DOWNLOAD
Author : Ravikiran Vadlapudi
language : en
Publisher:
Release Date : 2013
Verbose Labels For Semantic Roles written by Ravikiran Vadlapudi and has been published by this book supported file pdf, txt, epub, kindle and other format this book has been release on 2013 with Natural language processing (Computer science) categories.
We introduce a new task that takes the output of semantic role labeling and associates each of the argument slots for a predicate with a verbose description such as buyer or thing_bought to semantic role labels such as 'Arg0' and 'Arg1' for predicate like "buy". Ambiguous verb senses and syntactic alternations make this a challenging task. We adapt the frame information for each verb in the PropBank to create our training data. We propose various baseline methods and more informed models which can identify such verbose labels with 95.2% accuracy if the semantic roles have already been correctly identified. We extend our work to text visualization to illustrate the importance of verbose labeling. As a proof of concept, we built an interactive browser for human history articles from Wikipedia, called lensingWikipedia.
Hands On Natural Language Processing With Python
DOWNLOAD
Author : Rajesh Arumugam
language : en
Publisher: Packt Publishing Ltd
Release Date : 2018-07-18
Hands On Natural Language Processing With Python written by Rajesh Arumugam and has been published by Packt Publishing Ltd this book supported file pdf, txt, epub, kindle and other format this book has been release on 2018-07-18 with Computers categories.
Foster your NLP applications with the help of deep learning, NLTK, and TensorFlow Key Features Weave neural networks into linguistic applications across various platforms Perform NLP tasks and train its models using NLTK and TensorFlow Boost your NLP models with strong deep learning architectures such as CNNs and RNNs Book Description Natural language processing (NLP) has found its application in various domains, such as web search, advertisements, and customer services, and with the help of deep learning, we can enhance its performances in these areas. Hands-On Natural Language Processing with Python teaches you how to leverage deep learning models for performing various NLP tasks, along with best practices in dealing with today’s NLP challenges. To begin with, you will understand the core concepts of NLP and deep learning, such as Convolutional Neural Networks (CNNs), recurrent neural networks (RNNs), semantic embedding, Word2vec, and more. You will learn how to perform each and every task of NLP using neural networks, in which you will train and deploy neural networks in your NLP applications. You will get accustomed to using RNNs and CNNs in various application areas, such as text classification and sequence labeling, which are essential in the application of sentiment analysis, customer service chatbots, and anomaly detection. You will be equipped with practical knowledge in order to implement deep learning in your linguistic applications using Python's popular deep learning library, TensorFlow. By the end of this book, you will be well versed in building deep learning-backed NLP applications, along with overcoming NLP challenges with best practices developed by domain experts. What you will learn Implement semantic embedding of words to classify and find entities Convert words to vectors by training in order to perform arithmetic operations Train a deep learning model to detect classification of tweets and news Implement a question-answer model with search and RNN models Train models for various text classification datasets using CNN Implement WaveNet a deep generative model for producing a natural-sounding voice Convert voice-to-text and text-to-voice Train a model to convert speech-to-text using DeepSpeech Who this book is for Hands-on Natural Language Processing with Python is for you if you are a developer, machine learning or an NLP engineer who wants to build a deep learning application that leverages NLP techniques. This comprehensive guide is also useful for deep learning users who want to extend their deep learning skills in building NLP applications. All you need is the basics of machine learning and Python to enjoy the book.