Verbose Labels For Semantic Roles
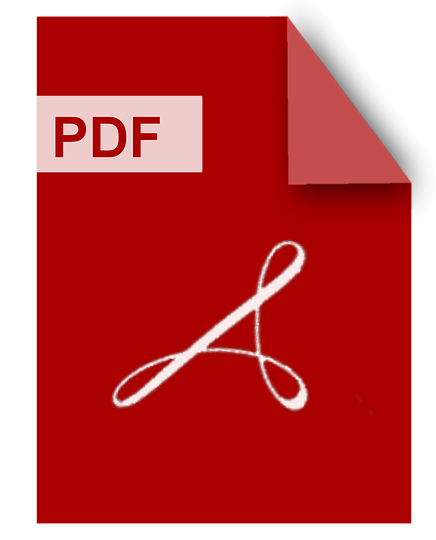
DOWNLOAD
Download Verbose Labels For Semantic Roles PDF/ePub or read online books in Mobi eBooks. Click Download or Read Online button to get Verbose Labels For Semantic Roles book now. This website allows unlimited access to, at the time of writing, more than 1.5 million titles, including hundreds of thousands of titles in various foreign languages. If the content not found or just blank you must refresh this page
Verbose Labels For Semantic Roles
DOWNLOAD
Author : Ravikiran Vadlapudi
language : en
Publisher:
Release Date : 2013
Verbose Labels For Semantic Roles written by Ravikiran Vadlapudi and has been published by this book supported file pdf, txt, epub, kindle and other format this book has been release on 2013 with Natural language processing (Computer science) categories.
We introduce a new task that takes the output of semantic role labeling and associates each of the argument slots for a predicate with a verbose description such as buyer or thing_bought to semantic role labels such as 'Arg0' and 'Arg1' for predicate like "buy". Ambiguous verb senses and syntactic alternations make this a challenging task. We adapt the frame information for each verb in the PropBank to create our training data. We propose various baseline methods and more informed models which can identify such verbose labels with 95.2% accuracy if the semantic roles have already been correctly identified. We extend our work to text visualization to illustrate the importance of verbose labeling. As a proof of concept, we built an interactive browser for human history articles from Wikipedia, called lensingWikipedia.
Semantic Role Labeling
DOWNLOAD
Author : Martha Palmer
language : en
Publisher: Morgan & Claypool Publishers
Release Date : 2011-02-02
Semantic Role Labeling written by Martha Palmer and has been published by Morgan & Claypool Publishers this book supported file pdf, txt, epub, kindle and other format this book has been release on 2011-02-02 with Computers categories.
This book is aimed at providing an overview of several aspects of semantic role labeling. Chapter 1 begins with linguistic background on the definition of semantic roles and the controversies surrounding them. Chapter 2 describes how the theories have led to structured lexicons such as FrameNet, VerbNet and the PropBank Frame Files that in turn provide the basis for large scale semantic annotation of corpora. This data has facilitated the development of automatic semantic role labeling systems based on supervised machine learning techniques. Chapter 3 presents the general principles of applying both supervised and unsupervised machine learning to this task, with a description of the standard stages and feature choices, as well as giving details of several specific systems. Recent advances include the use of joint inference to take advantage of context sensitivities, and attempts to improve performance by closer integration of the syntactic parsing task with semantic role labeling. Chapter 3 also discusses the impact the granularity of the semantic roles has on system performance. Having outlined the basic approach with respect to English, Chapter 4 goes on to discuss applying the same techniques to other languages, using Chinese as the primary example. Although substantial training data is available for Chinese, this is not the case for many other languages, and techniques for projecting English role labels onto parallel corpora are also presented. Table of Contents: Preface / Semantic Roles / Available Lexical Resources / Machine Learning for Semantic Role Labeling / A Cross-Lingual Perspective / Summary
Anaphora Resolution
DOWNLOAD
Author : Massimo Poesio
language : en
Publisher: Springer
Release Date : 2016-08-04
Anaphora Resolution written by Massimo Poesio and has been published by Springer this book supported file pdf, txt, epub, kindle and other format this book has been release on 2016-08-04 with Computers categories.
This book lays out a path leading from the linguistic and cognitive basics, to classical rule-based and machine learning algorithms, to today’s state-of-the-art approaches, which use advanced empirically grounded techniques, automatic knowledge acquisition, and refined linguistic modeling to make a real difference in real-world applications. Anaphora and coreference resolution both refer to the process of linking textual phrases (and, consequently, the information attached to them) within as well as across sentence boundaries, and to the same discourse referent. The book offers an overview of recent research advances, focusing on practical, operational approaches and their applications. In part I (Background), it provides a general introduction, which succinctly summarizes the linguistic, cognitive, and computational foundations of anaphora processing and the key classical rule- and machine-learning-based anaphora resolution algorithms. Acknowledging the central importance of shared resources, part II (Resources) covers annotated corpora, formal evaluation, preprocessing technology, and off-the-shelf anaphora resolution systems. Part III (Algorithms) provides a thorough description of state-of-the-art anaphora resolution algorithms, covering enhanced machine learning methods as well as techniques for accomplishing important subtasks such as mention detection and acquisition of relevant knowledge. Part IV (Applications) deals with a selection of important anaphora and coreference resolution applications, discussing particular scenarios in diverse domains and distilling a best-practice model for systematically approaching new application cases. In the concluding part V (Outlook), based on a survey conducted among the contributing authors, the prospects of the research field of anaphora processing are discussed, and promising new areas of interdisciplinary cooperation and emerging application scenarios are identified. Given the book’s design, it can be used both as an accompanying text for advanced lectures in computational linguistics, natural language engineering, and computer science, and as a reference work for research and independent study. It addresses an audience that includes academic researchers, university lecturers, postgraduate students, advanced undergraduate students, industrial researchers, and software engineers.
Using Semantic Role Labels To Reorder Statistical Machine Translation Output
DOWNLOAD
Author : Chi Kiu Lo
language : en
Publisher:
Release Date : 2009
Using Semantic Role Labels To Reorder Statistical Machine Translation Output written by Chi Kiu Lo and has been published by this book supported file pdf, txt, epub, kindle and other format this book has been release on 2009 with Machine translating categories.
Generalizing Semantic Role Annotations Across Syntactically Similar Verbs
DOWNLOAD
Author :
language : en
Publisher:
Release Date : 2007
Generalizing Semantic Role Annotations Across Syntactically Similar Verbs written by and has been published by this book supported file pdf, txt, epub, kindle and other format this book has been release on 2007 with categories.
Large corpora of parsed sentences with semantic role labels (e.g. PropBank) provide training data for use in the creation of high-performance automatic semantic role labeling systems. Despite the size of these corpora, individual verbs (or rolesets) often have only a handful of instances in these corpora, and only a fraction of English verbs have even a single annotation. In this paper, we describe an approach for dealing with this sparse data problem, enabling accurate semantic role labeling for novel verbs (rolesets) with only a single training example. Our approach involves the identification of syntactically similar verbs found in Prop-Bank, the alignment of arguments in their corresponding rolesets, and the use of their corresponding annotations in Prop-Bank as surrogate training data.
Semantic Features For Semantic Role Labeling
DOWNLOAD
Author : Liam R. McGrath
language : en
Publisher:
Release Date : 2011
Semantic Features For Semantic Role Labeling written by Liam R. McGrath and has been published by this book supported file pdf, txt, epub, kindle and other format this book has been release on 2011 with Semantics categories.
Semantic Role Labeling Using Lexicalized Tree Adjoining Grammars
DOWNLOAD
Author : Yudong Liu
language : en
Publisher:
Release Date : 2009
Semantic Role Labeling Using Lexicalized Tree Adjoining Grammars written by Yudong Liu and has been published by this book supported file pdf, txt, epub, kindle and other format this book has been release on 2009 with Computational linguistics categories.
The predicate-argument structure (PAS) of a natural language sentence is a useful representation that can be used for a deeper analysis of the underlying meaning of the sentence or directly used in various natural language processing (NLP) applications. The task of semantic role labeling (SRL) is to identify the predicate-argument structures and label the relations between the predicate and each of its arguments. Researchers have been studying SRL as a machine learning problem in the past six years, after large-scale semantically annotated corpora such as FrameNet and PropBank were released to the research community. Lexicalized Tree Adjoining Grammars (LTAGs), a tree rewriting formalism, are often a convenient representation for capturing locality of predicate-argument relations. Our work in this thesis is focused on the development and learning of the state of the art discriminative SRL systems with LTAGs. Our contributions to this field include: We apply to the SRL task a variant of the LTAG formalism called LTAG-spinal and the associated LTAG-spinal Treebank (the formalism and the Treebank were created by Libin Shen). Predicate-argument relations that are either implicit or absent from the original Penn Treebank are made explicit and accessible in the LTAG-spinal Treebank, which we show to be a useful resource for SRL. We propose the use of the LTAGs as an important additional source of features for the SRL task. Our experiments show that, compared with the best-known set of features that are used in state of the art SRL systems, LTAG-based features can improve SRL performance significantly. We treat multiple LTAG derivation trees as latent features for SRL and introduce a novel learning framework -- Latent Support Vector Machines (LSVMs) to the SRL task using these latent features. This method significantly outperforms state of the art SRL systems. In addition, we adapt an SRL framework to a real-world ternary relation extraction task in the biomedical domain. Our experiments show that the use of SRL related features significantly improves performance over the system using only shallow word-based features.
Constructions Collocations Patterns
DOWNLOAD
Author : Thomas Herbst
language : en
Publisher: Walter de Gruyter GmbH & Co KG
Release Date : 2014-10-14
Constructions Collocations Patterns written by Thomas Herbst and has been published by Walter de Gruyter GmbH & Co KG this book supported file pdf, txt, epub, kindle and other format this book has been release on 2014-10-14 with Language Arts & Disciplines categories.
This volume, which has textbook character, is intended to provide an in-depth introduction to different theoretical and methodological research frameworks concerned with the role of item-specific grammatical and lexical behaviour.
Learning Structured Probabilistic Models For Semantic Role Labeling
DOWNLOAD
Author : David Terrell Vickrey
language : en
Publisher:
Release Date : 2010
Learning Structured Probabilistic Models For Semantic Role Labeling written by David Terrell Vickrey and has been published by this book supported file pdf, txt, epub, kindle and other format this book has been release on 2010 with categories.
Teaching a computer to read is one of the most interesting and important artificial intelligence tasks. In this thesis, we focus on semantic role labeling (SRL), one important processing step on the road from raw text to a full semantic representation. Given an input sentence and a target verb in that sentence, the SRL task is to label the semantic arguments, or roles, of that verb. For example, in the sentence "Tom eats an apple, " the verb "eat" has two roles, Eater = "Tom" and Thing Eaten = "apple". Most SRL systems, including the ones presented in this thesis, take as input a syntactic analysis built by an automatic syntactic parser. SRL systems rely heavily on path features constructed from the syntactic parse, which capture the syntactic relationship between the target verb and the phrase being classified. However, there are several issues with these path features. First, the path feature does not always contain all relevant information for the SRL task. Second, the space of possible path features is very large, resulting in very sparse features that are hard to learn. In this thesis, we consider two ways of addressing these issues. First, we experiment with a number of variants of the standard syntactic features for SRL. We include a large number of syntactic features suggested by previous work, many of which are designed to reduce sparsity of the path feature. We also suggest several new features, most of which are designed to capture additional information about the sentence not included in the standard path feature. We build an SRL model using the best of these new and old features, and show that this model achieves performance competitive with current state-of-the-art. The second method we consider is a new methodology for SRL based on labeling canonical forms. A canonical form is a representation of a verb and its arguments that is abstracted away from the syntax of the input sentence. For example, "A car hit Bob" and "Bob was hit by a car" have the same canonical form, {Verb = "hit", Deep Subject = "a car", Deep Object = "a car"}. Labeling canonical forms makes it much easier to generalize between sentences with different syntax. To label canonical forms, we first need to automatically extract them given an input parse. We develop a system based on a combination of hand-coded rules and machine learning. This allows us to include a large amount of linguistic knowledge and also have the robustness of a machine learning system. Our system improves significantly over a strong baseline, demonstrating the viability of this new approach to SRL. This latter method involves learning a large, complex probabilistic model. In the model we present, exact learning is tractable, but there are several natural extensions to the model for which exact learning is not possible. This is quite a general issue; in many different application domains, we would like to use probabilistic models that cannot be learned exactly. We propose a new method for learning these kinds of models based on contrastive objectives. The main idea is to learn by comparing only a few possible values of the model, instead of all possible values. This method generalizes a standard learning method, pseudo-likelihood, and is closely related to another, contrastive divergence. Previous work has mostly focused on comparing nearby sets of values; we focus on non-local contrastive objectives, which compare arbitrary sets of values. We prove several theoretical results about our model, showing that contrastive objectives attempt to enforce probability ratio constraints between the compared values. Based on this insight, we suggest several methods for constructing contrastive objectives, including contrastive constraint generation (CCG), a cutting-plane style algorithm that iteratively builds a good contrastive objective based on finding high-scoring values. We evaluate CCG on a machine vision task, showing that it significantly outperforms pseudo-likelihood, contrastive divergence, as well as a state-of-the-art max-margin cutting-plane algorithm.
Natural Language Processing With Python
DOWNLOAD
Author : Steven Bird
language : en
Publisher: "O'Reilly Media, Inc."
Release Date : 2009-06-12
Natural Language Processing With Python written by Steven Bird and has been published by "O'Reilly Media, Inc." this book supported file pdf, txt, epub, kindle and other format this book has been release on 2009-06-12 with Computers categories.
This book offers a highly accessible introduction to natural language processing, the field that supports a variety of language technologies, from predictive text and email filtering to automatic summarization and translation. With it, you'll learn how to write Python programs that work with large collections of unstructured text. You'll access richly annotated datasets using a comprehensive range of linguistic data structures, and you'll understand the main algorithms for analyzing the content and structure of written communication. Packed with examples and exercises, Natural Language Processing with Python will help you: Extract information from unstructured text, either to guess the topic or identify "named entities" Analyze linguistic structure in text, including parsing and semantic analysis Access popular linguistic databases, including WordNet and treebanks Integrate techniques drawn from fields as diverse as linguistics and artificial intelligence This book will help you gain practical skills in natural language processing using the Python programming language and the Natural Language Toolkit (NLTK) open source library. If you're interested in developing web applications, analyzing multilingual news sources, or documenting endangered languages -- or if you're simply curious to have a programmer's perspective on how human language works -- you'll find Natural Language Processing with Python both fascinating and immensely useful.