Sequence Based Protein Classification
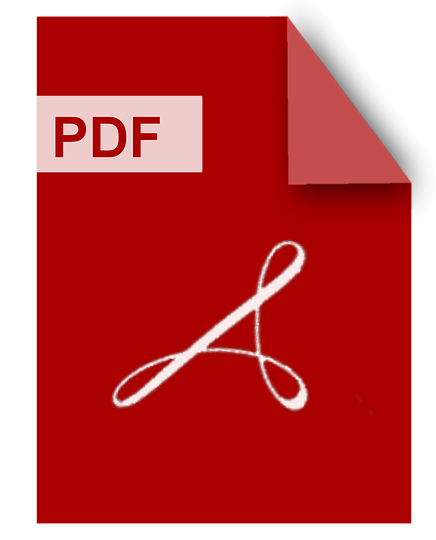
DOWNLOAD
Download Sequence Based Protein Classification PDF/ePub or read online books in Mobi eBooks. Click Download or Read Online button to get Sequence Based Protein Classification book now. This website allows unlimited access to, at the time of writing, more than 1.5 million titles, including hundreds of thousands of titles in various foreign languages. If the content not found or just blank you must refresh this page
Sequence Based Protein Classification
DOWNLOAD
Author : Stefan Mutter
language : en
Publisher:
Release Date : 2011
Sequence Based Protein Classification written by Stefan Mutter and has been published by this book supported file pdf, txt, epub, kindle and other format this book has been release on 2011 with Bioinformatics categories.
Protein Classification Based On Analysis Of Local Sequence Structure Correspondence
DOWNLOAD
Author : A. T. Zemla
language : en
Publisher:
Release Date : 2006
Protein Classification Based On Analysis Of Local Sequence Structure Correspondence written by A. T. Zemla and has been published by this book supported file pdf, txt, epub, kindle and other format this book has been release on 2006 with categories.
The goal of this project was to develop an algorithm to detect and calculate common structural motifs in compared structures, and define a set of numerical criteria to be used for fully automated motif based protein structure classification. The Protein Data Bank (PDB) contains more than 33,000 experimentally solved protein structures, and the Structural Classification of Proteins (SCOP) database, a manual classification of these structures, cannot keep pace with the rapid growth of the PDB. In our approach called STRALCP (STRucture Alignment based Clustering of Proteins), we generate detailed information about global and local similarities between given set of structures, identify similar fragments that are conserved within analyzed proteins, and use these conserved regions (detected structural motifs) to classify proteins.
Protein Classification Based On Analysis Of Local Sequence Structure Correspondence
DOWNLOAD
Author :
language : en
Publisher:
Release Date : 2006
Protein Classification Based On Analysis Of Local Sequence Structure Correspondence written by and has been published by this book supported file pdf, txt, epub, kindle and other format this book has been release on 2006 with categories.
Protein Families
DOWNLOAD
Author : Christine A. Orengo
language : en
Publisher: John Wiley & Sons
Release Date : 2014-02-03
Protein Families written by Christine A. Orengo and has been published by John Wiley & Sons this book supported file pdf, txt, epub, kindle and other format this book has been release on 2014-02-03 with Computers categories.
New insights into the evolution and nature of proteins Exploring several distinct approaches, this book describes the methods for comparing protein sequences and protein structures in order to identify homologous relationships and classify proteins and protein domains into evolutionary families. Readers will discover the common features as well as the key philosophical differences underlying the major protein classification systems, including Pfam, Panther, SCOP, and CATH. Moreover, they'll discover how these systems can be used to understand the evolution of protein families as well as understand and predict the degree to which structural and functional information are shared between relatives in a protein family. Edited and authored by leading international experts, Protein Families offers new insights into protein families that are important to medical research as well as protein families that help us understand biological systems and key biological processes such as cell signaling and the immune response. The book is divided into three sections: Section I: Concepts Underlying Protein Family Classification reviews the major strategies for identifying homologous proteins and classifying them into families. Section II: In-Depth Reviews of Protein Families focuses on some fascinating super protein families for which we have substantial amounts of sequence, structural and functional data, making it possible to trace the emergence of functionally diverse relatives. Section III: Review of Protein Families in Important Biological Systems examines protein families associated with a particular biological theme, such as the cytoskeleton. All chapters are extensively illustrated, including depictions of evolutionary relationships. References at the end of each chapter guide readers to original research papers and reviews in the field. Covering protein family classification systems alongside detailed descriptions of select protein families, this book offers biochemists, molecular biologists, protein scientists, structural biologists, and bioinformaticians new insight into the evolution and nature of proteins.
Data Driven Discovery Of Rules For Protein Function Classification Based On Sequence Motifs
DOWNLOAD
Author : Xiangyun Wang
language : en
Publisher:
Release Date : 2002
Data Driven Discovery Of Rules For Protein Function Classification Based On Sequence Motifs written by Xiangyun Wang and has been published by this book supported file pdf, txt, epub, kindle and other format this book has been release on 2002 with categories.
This thesis describes an approach to data-driven discovery of decision trees or rules for assigning protein sequences to functional families using sequence motifs. This method is able to capture regularities that can be described in terms of presence or absence of arbitrary combinations of motifs. A training set of peptidase sequences labeled with the corresponding MEROPS functional families or clans is used to automatically construct decision trees that capture regularities that are sufficient to assign the sequences to their respective functional families. The performance of the resulting decision tree classifiers is then evaluated on an independent test set. Results of experiments that proposed approach matches or outperforms protein function classification based on the presence of a single characteristic motif in terms of accuracy, precision, and recall. We compared the rules constructed using motifs generated by a multiple sequence alignment based motif discovery tool (MEME) with rules constructed using expert annotated ProSite motifs (patterns and profiles). Our results indicate that the former provide a potentially powerful high throughput technique for constructing protein function classifiers when adequate training data are available. Examination of the generated rules in the case of a Caspase (C14) family suggests that the proposed technique might be able to identify combinations of sequence motifs that characterize functionally significant 3-dimensional structural features of proteins.
Kernel Based Classification Of Protein Structure And Function From Amino Acid Sequences
DOWNLOAD
Author : Jonathan James Ward
language : en
Publisher:
Release Date : 2005
Kernel Based Classification Of Protein Structure And Function From Amino Acid Sequences written by Jonathan James Ward and has been published by this book supported file pdf, txt, epub, kindle and other format this book has been release on 2005 with categories.
Classifying Noisy Protein Sequence Data A Case Study Of Immunoglobulin Light Chains
DOWNLOAD
Author :
language : en
Publisher:
Release Date : 2005
Classifying Noisy Protein Sequence Data A Case Study Of Immunoglobulin Light Chains written by and has been published by this book supported file pdf, txt, epub, kindle and other format this book has been release on 2005 with categories.
The classification of protein sequences obtained from patients with various immunoglobulin-related conformational diseases may provide insight into structural correlates of pathogenicity. However, clinical data are very sparse and, in the case of antibody-related proteins, the collected sequences have large variability with only a small subset of variations relevant to the protein pathogenicity (function). On this basis, these sequences represent a model system for development of strategies to recognize the small subset of function-determining variations among the much larger number of primary structure diversifications introduced during evolution. Under such conditions, most protein classification algorithms have limited accuracy. To address this problem, we propose a support vector machine (SVM)-based classifier that combines sequence and 3D structural averaging information. Each amino acid in the sequence is represented by a set of six physicochemical properties: hydrophobicity, hydrophilicity volume, surface area, bulkiness and refractivity. Each position in the sequence is described by the properties of the amino acid at that position and the properties of its neighbors in 3D space or in the sequence. A structure template is selected to determine neighbors in 3D space and a window size is used to determine the neighbors in the sequence. The test data consist of 209 proteins of human antibody immunoglobulin light chains, each represented by aligned sequences of 120 amino acids. The methodology is applied to the classification of protein sequences collected from patients with and without amyloidosis, and indicates that the proposed modified classifiers are more robust to sequence variability than standard SVM classifiers, improving classification error between 5 and 25% and sensitivity between 9 and 17%. The classification results might also suggest possible mechanisms for the propensity of immunoglobulin light chains to amyloid formation.
A Synonymous Dictionary Based Approach For Protein Sequence Analysis And Classification
DOWNLOAD
Author : 信男·林
language : en
Publisher:
Release Date : 2010
A Synonymous Dictionary Based Approach For Protein Sequence Analysis And Classification written by 信男·林 and has been published by this book supported file pdf, txt, epub, kindle and other format this book has been release on 2010 with categories.
Protein Bioinformatics
DOWNLOAD
Author : Ingvar Eidhammer
language : en
Publisher: John Wiley & Sons
Release Date : 2004-02-13
Protein Bioinformatics written by Ingvar Eidhammer and has been published by John Wiley & Sons this book supported file pdf, txt, epub, kindle and other format this book has been release on 2004-02-13 with Mathematics categories.
Pairwise global alignment of sequences. Pairwise local alignment and database search. Statical analysis. Multiple global alignment and phylogenetic trees. Scoring matrices. Profiles. Sequence patterns. Structures and structure descriptions. Superposition and Dynamic programming. Geometric techniques. Clustering: Combining local similarities. Significance and assessment of structure comparisons. Multiple structure comparison. Protein structure classification. Structure prediction: Threading. Basics in mathematics, probability and algorithms. Introduction to molecular biology.
Clustering And Classification Of Multi Domain Proteins
DOWNLOAD
Author : Neethu Shah
language : en
Publisher:
Release Date : 2013
Clustering And Classification Of Multi Domain Proteins written by Neethu Shah and has been published by this book supported file pdf, txt, epub, kindle and other format this book has been release on 2013 with categories.
Rapid development of next-generation sequencing technology has led to an unprecedented growth in protein sequence data repositories over the last decade. Majority of these proteins lack structural and functional characterization. This necessitates design and development of fast, efficient, and sensitive computational tools and algorithms that can classify these proteins into functionally coherent groups. Domains are fundamental units of protein structure and function. Multi-domain proteins are extremely complex as opposed to proteins that have single or no domains. They exhibit network-like complex evolutionary events such as domain shuffling, domain loss, and domain gain. These events therefore, cannot be represented in the conventional protein clustering algorithms like phylogenetic reconstruction and Markov clustering. In this thesis, a multi-domain protein classification system is developed primarily based on the domain composition of protein sequences. Using the principle of co-clustering (biclustering), both proteins and domains are simultaneously clustered, where each bicluster contains a subset of proteins and domains forming a complete bipartite graph. These clusters are then converted into a network of biclusters based on the domains shared between the clusters, thereby classifying the proteins into similar protein families. We applied our biclustering network approach on a multi-domain protein family, Regulator of G-protein Signalling (RGS) proteins, where heterogeneous domain composition exists among subfamilies. Our approach showed mostly consistent clustering with the existing RGS subfamilies. The average maximum Jaccard Index scores for the clusters obtained by Markov Clustering and phylogenetic clustering methods against the biclusters were 0.64 and 0.60, respectively. Compared to other clustering methods, our approach uses auxiliary domain information of each protein, and therefore, generates more functionally coherent protein clusters and differentiates each protein subfamily from each other. Biclustered networks on complete nine proteomes showed that the number of multi-domain proteins included in connected biclusters rapidly increased with genome complexity, 48.5% in bacteria to 80% in eukaryotes. Protein clustering and classification, incorporating such wealth of additonal domain information on protein networks has wide applications and would impact functional analysis and characterization of novel proteins.